Don't wanna be here? Send us removal request.
Text
Revolutionizing Business Operations with Generative AI
Introduction
The digital transformation era has introduced groundbreaking technologies that are reshaping the way businesses operate. One such technology is Generative AI, which is revolutionizing industries by automating processes, enhancing decision-making, and driving innovation. Companies that integrate generative AI into their workflows gain a competitive advantage by improving efficiency, reducing costs, and delivering superior customer experiences.
Understanding Generative AI
Generative AI refers to artificial intelligence models capable of creating new content, including text, images, music, and code, based on existing data. Unlike traditional AI systems that analyze and classify information, generative AI can generate original, high-quality outputs, making it an essential tool for businesses looking to optimize their operations.
Key Applications of Generative AI in Business Operations
1. Automating Content Creation
Generative AI streamlines content production by automatically generating high-quality text, reports, marketing materials, and even personalized customer communications. Businesses can use AI-powered content generation to enhance productivity and maintain consistent branding.
2. Enhancing Customer Support with AI Chatbots
AI-driven chatbots powered by generative AI provide human-like interactions, resolving customer queries efficiently and improving user experience. These AI assistants can handle inquiries, process orders, and offer recommendations, reducing the workload for human support teams.
3. Optimizing Data Analysis and Insights
Generative AI helps businesses analyze vast amounts of data, identify trends, and generate actionable insights. By automating data interpretation, businesses can make informed decisions quickly and stay ahead of the competition.
4. Personalizing Marketing and Sales Strategies
Generative AI enables hyper-personalized marketing by analyzing customer behavior and generating tailored content, product recommendations, and dynamic advertisements. This increases engagement, conversion rates, and customer satisfaction.
5. Improving Software Development and Code Generation
Developers can leverage generative AI to generate code snippets, debug programs, and automate repetitive coding tasks. This accelerates software development, reduces errors, and enhances productivity in IT operations.
Benefits of Implementing Generative AI
Increased Efficiency and Productivity
By automating repetitive tasks, businesses can allocate resources to more strategic initiatives, improving overall productivity and operational efficiency.
Cost Reduction
Generative AI reduces labor-intensive tasks, minimizing operational costs while maintaining high-quality output. Businesses can streamline processes without compromising performance.
Enhanced Creativity and Innovation
AI-generated content and design suggestions help businesses develop innovative products, campaigns, and strategies that align with market demands.
Data-Driven Decision Making
Generative AI enhances business intelligence by processing and visualizing complex data sets, allowing leaders to make more accurate and strategic decisions.
Challenges in Adopting Generative AI
Despite its advantages, integrating generative AI into business operations presents challenges:
Data Privacy Concerns: AI systems require vast datasets, raising concerns about data security and compliance with privacy regulations.
Implementation Costs: Initial investments in AI infrastructure, training, and integration may be high.
Ethical Considerations: Businesses must ensure AI-generated content is accurate, unbiased, and ethically responsible.
Skill Gaps: Organizations may need to train employees to work effectively with AI technologies.
Future Trends in Generative AI
Generative AI continues to evolve, shaping the future of business operations:
Advanced AI Models: Next-generation AI models will improve accuracy, creativity, and adaptability.
AI-Powered Workflows: Businesses will integrate AI across departments to automate end-to-end processes.
Voice and Video Generation: AI will generate realistic voiceovers, video content, and virtual avatars for enhanced communication.
Regulatory Developments: Governments will introduce policies to ensure responsible AI usage and ethical standards.
Conclusion
Generative AI is transforming business operations by automating tasks, improving efficiency, and enhancing decision-making. Organizations that embrace AI-driven innovation can optimize workflows, personalize customer interactions, and stay ahead in a rapidly evolving digital landscape. As generative AI technology advances, businesses that leverage its potential today will be well-equipped for future success.
0 notes
Text
Embracing Innovation with Artificial Intelligence Solutions
Introduction
Artificial Intelligence (AI) has emerged as a transformative force, revolutionizing industries and driving business growth. Companies across various sectors are leveraging Artificial Intelligence Solutions to automate processes, enhance decision-making, and create personalized customer experiences. With rapid advancements in AI, businesses must adopt future-proof technologies to stay competitive in the digital age.
This article explores the benefits, applications, and implementation strategies of Artificial Intelligence Solutions and how they empower businesses to achieve sustainable success.
Understanding Artificial Intelligence Solutions
Artificial Intelligence Solutions refer to a suite of technologies that enable machines to learn, reason, and make decisions with minimal human intervention. These solutions encompass:
Machine Learning (ML) – Algorithms that improve performance over time based on data.
Natural Language Processing (NLP) – AI’s ability to understand and generate human language.
Computer Vision – Technology that enables AI to interpret and analyze images or videos.
Predictive Analytics – AI-driven forecasting based on historical data.
Robotic Process Automation (RPA) – Software bots that automate repetitive tasks.
The Role of Artificial Intelligence Solutions in Business Transformation
Businesses adopting Artificial Intelligence Solutions gain a competitive edge by optimizing efficiency, improving customer experience, and reducing operational costs. Here’s how AI is reshaping industries:
1. Enhancing Business Intelligence and Decision-Making
AI-driven data analytics allows businesses to:
Identify patterns and trends in massive datasets.
Make real-time, data-driven decisions.
Predict future outcomes with advanced forecasting models.
2. Automating Workflows for Increased Efficiency
By integrating Artificial Intelligence Solutions, organizations can:
Streamline operations with AI-powered workflow automation.
Minimize human errors in repetitive tasks with Robotic Process Automation (RPA).
Improve productivity with AI-driven insights and recommendations.
3. Improving Customer Experience with AI
AI is revolutionizing customer engagement through:
Personalized marketing campaigns based on customer behavior analysis.
AI chatbots that offer 24/7 customer support and assistance.
Voice recognition systems that enhance interactions via digital assistants.
4. Optimizing Supply Chain Management
AI is playing a crucial role in supply chain efficiency by:
Predicting demand and optimizing inventory management.
Automating logistics with AI-powered route optimization.
Enhancing risk management by detecting potential disruptions in real-time.
5. Strengthening Cybersecurity Measures
AI-powered security solutions help businesses detect and prevent cyber threats by:
Identifying vulnerabilities in networks and systems.
Detecting unusual patterns in user behavior that may indicate fraud.
Automating threat detection and response to mitigate risks.
Industry Use Cases of Artificial Intelligence Solutions
The adoption of AI is transforming industries across the board. Here’s how different sectors are leveraging Artificial Intelligence Solutions:
1. Healthcare & Life Sciences
AI-driven diagnostic tools that detect diseases with high accuracy.
Predictive analytics for patient health monitoring and early diagnosis.
AI-powered drug discovery and personalized treatment recommendations.
2. Retail & E-Commerce
Personalized product recommendations based on AI-driven analytics.
AI chatbots for real-time customer support and order management.
Automated inventory tracking and demand forecasting.
3. Financial Services & Banking
AI-driven fraud detection and risk assessment in real time.
Algorithm-based credit scoring and loan approval processes.
AI-powered robo-advisors for investment management.
4. Manufacturing & Industry 4.0
Predictive maintenance to prevent equipment failures.
AI-driven quality control systems for defect detection.
Smart factories using AI for optimized production processes.
5. Education & E-Learning
AI-powered adaptive learning platforms for personalized education.
Automated grading and assessment systems.
AI-driven virtual tutors to enhance student engagement.
Best Practices for Implementing Artificial Intelligence Solutions
Successfully integrating Artificial Intelligence Solutions requires a strategic approach. Here are key best practices for AI adoption:
1. Define Business Objectives and AI Goals
Identify specific challenges AI can address.
Align AI initiatives with overall business strategy.
2. Ensure Data Quality and Availability
AI systems rely on high-quality, well-structured data.
Implement data governance policies for accuracy and security.
3. Adopt Scalable AI Technologies
Invest in cloud-based AI platforms for scalability and flexibility.
Use modular AI models that can adapt to business growth.
4. Prioritize Ethical AI and Compliance
Ensure AI solutions comply with data privacy regulations.
Develop transparent AI systems that minimize bias and ensure fairness.
5. Upskill Workforce for AI Readiness
Provide AI training programs for employees.
Encourage cross-functional collaboration between AI teams and business units.
Overcoming Challenges in AI Adoption
While Artificial Intelligence Solutions offer significant advantages, businesses may face challenges such as:
1. Data Privacy Concerns
Ensure compliance with GDPR, CCPA, and other regulations.
Use encryption and anonymization techniques for data security.
2. High Initial Investment Costs
Start with pilot AI projects before full-scale implementation.
Consider AI-as-a-Service (AIaaS) models for cost-effective adoption.
3. Integration with Legacy Systems
Develop a phased AI implementation strategy.
Use APIs and middleware to connect AI with existing infrastructure.
The Future of Artificial Intelligence Solutions
AI is constantly evolving, with emerging trends set to shape the future of business operations:
Generative AI for creating high-quality content and simulations.
AI-driven automation for fully autonomous business processes.
Quantum AI computing for solving complex computational problems.
As AI continues to advance, businesses that embrace Artificial Intelligence Solutions will gain a competitive edge in innovation, efficiency, and customer engagement.
Conclusion
The adoption of Artificial Intelligence Solutions is no longer optional—it is essential for businesses to thrive in the digital age. By leveraging AI-powered automation, data analytics, and machine learning, organizations can enhance productivity, optimize operations, and create unparalleled customer experiences.
Companies that strategically implement AI will unlock new opportunities, improve decision-making, and future-proof their operations. The key to success lies in integrating AI with a clear vision, ethical considerations, and a robust data strategy. As technology evolves, AI will continue to be the driving force behind digital transformation, reshaping industries and paving the way for a smarter future.
0 notes
Text
Optimizing Data Operations with Databricks Services
Introduction
In today’s data-driven world, businesses generate vast amounts of information that must be processed, analyzed, and stored efficiently. Managing such complex data environments requires advanced tools and expert guidance. Databricks Services offer comprehensive solutions to streamline data operations, enhance analytics, and drive AI-powered decision-making.
This article explores how Databricks Services accelerate data operations, their key benefits, and best practices for maximizing their potential.
What are Databricks Services?
Databricks Services encompass a suite of cloud-based solutions and consulting offerings that help organizations optimize their data processing, machine learning, and analytics workflows. These services include:
Data Engineering and ETL: Automating data ingestion, transformation, and storage.
Big Data Processing with Apache Spark: Optimizing large-scale distributed computing.
Machine Learning and AI Integration: Leveraging Databricks for predictive analytics.
Data Governance and Security: Implementing policies to ensure data integrity and compliance.
Cloud Migration and Optimization: Transitioning from legacy systems to modern Databricks environments on AWS, Azure, or Google Cloud.
How Databricks Services Enhance Data Operations
Organizations that leverage Databricks Services benefit from a unified platform designed for scalability, efficiency, and AI-driven insights.
1. Efficient Data Ingestion and Integration
Seamless data integration is essential for real-time analytics and business intelligence. Databricks Services help organizations:
Automate ETL pipelines using Databricks Auto Loader.
Integrate data from multiple sources, including cloud storage, on-premise databases, and streaming data.
Improve data reliability with Delta Lake, ensuring consistency and schema evolution.
2. Accelerating Data Processing and Performance
Handling massive data volumes efficiently requires optimized computing resources. Databricks Services enable businesses to:
Utilize Apache Spark clusters for distributed data processing.
Improve query speed with Photon Engine, designed for high-performance analytics.
Implement caching, indexing, and query optimization techniques for better efficiency.
3. Scaling AI and Machine Learning Capabilities
Databricks Services provide the infrastructure and expertise to develop, train, and deploy machine learning models. These services include:
MLflow for end-to-end model lifecycle management.
AutoML capabilities for automated model tuning and selection.
Deep learning frameworks like TensorFlow and PyTorch for advanced AI applications.
4. Enhancing Security and Compliance
Data security and regulatory compliance are critical concerns for enterprises. Databricks Services ensure:
Role-based access control (RBAC) with Unity Catalog for data governance.
Encryption and data masking to protect sensitive information.
Compliance with GDPR, HIPAA, CCPA, and other industry regulations.
5. Cloud Migration and Modernization
Transitioning from legacy databases to modern cloud platforms can be complex. Databricks Services assist organizations with:
Seamless migration from Hadoop, Oracle, and Teradata to Databricks.
Cloud-native architecture design tailored for AWS, Azure, and Google Cloud.
Performance tuning and cost optimization for cloud computing environments.
Key Benefits of Databricks Services
Organizations that invest in Databricks Services unlock several advantages, including:
1. Faster Time-to-Insight
Pre-built data engineering templates accelerate deployment.
Real-time analytics improve decision-making and operational efficiency.
2. Cost Efficiency and Resource Optimization
Serverless compute options minimize infrastructure costs.
Automated scaling optimizes resource utilization based on workload demand.
3. Scalability and Flexibility
Cloud-native architecture ensures businesses can scale operations effortlessly.
Multi-cloud and hybrid cloud support enable flexibility in deployment.
4. AI-Driven Business Intelligence
Advanced analytics and AI models uncover hidden patterns in data.
Predictive insights improve forecasting and business strategy.
5. Robust Security and Governance
Enforces best-in-class data governance frameworks.
Ensures compliance with industry-specific regulatory requirements.
Industry Use Cases for Databricks Services
Many industries leverage Databricks Services to drive innovation and operational efficiency. Below are some key applications:
1. Financial Services
Fraud detection using AI-powered transaction analysis.
Regulatory compliance automation for banking and fintech.
Real-time risk assessment for investment portfolios.
2. Healthcare & Life Sciences
Predictive analytics for patient care optimization.
Drug discovery acceleration through genomic research.
HIPAA-compliant data handling for secure medical records.
3. Retail & E-Commerce
Personalized customer recommendations using AI.
Supply chain optimization with predictive analytics.
Demand forecasting to improve inventory management.
4. Manufacturing & IoT
Anomaly detection in IoT sensor data for predictive maintenance.
AI-enhanced quality control systems to reduce defects.
Real-time analytics for production line efficiency.
Best Practices for Implementing Databricks Services
To maximize the value of Databricks Services, organizations should follow these best practices:
1. Define Clear Objectives
Set measurable KPIs to track data operation improvements.
Align data strategies with business goals and revenue targets.
2. Prioritize Data Governance and Quality
Implement data validation and cleansing processes.
Leverage Unity Catalog for centralized metadata management.
3. Automate for Efficiency
Use Databricks automation tools to streamline ETL and machine learning workflows.
Implement real-time data streaming for faster insights.
4. Strengthen Security Measures
Enforce multi-layered security policies for data access control.
Conduct regular audits and compliance assessments.
5. Invest in Continuous Optimization
Update data pipelines and ML models to maintain peak performance.
Provide ongoing training for data engineers and analysts.
Conclusion
Databricks Services provide businesses with the expertise, tools, and technology needed to accelerate data operations, enhance AI-driven insights, and improve overall efficiency. Whether an organization is modernizing its infrastructure, implementing real-time analytics, or strengthening data governance, Databricks Services offer tailored solutions to meet these challenges.
By partnering with Databricks experts, companies can unlock the full potential of big data, AI, and cloud-based analytics, ensuring they stay ahead in today’s competitive digital landscape.
0 notes
Text
Enhancing Business Intelligence with Data Engineering Services
Introduction
In today's data-driven world, organizations rely on high-quality data to make informed decisions and drive innovation. However, managing and processing vast amounts of data can be a complex challenge. Data Engineering Services play a crucial role in optimizing data workflows, ensuring data quality, and enabling advanced analytics. By leveraging robust data engineering strategies, businesses can transform raw data into actionable insights and gain a competitive edge.
Understanding Data Engineering Services
Data Engineering Services focus on designing, building, and managing data infrastructure to ensure seamless data processing. These services help businesses handle structured and unstructured data efficiently, ensuring it is clean, organized, and ready for analysis.
Key components of Data Engineering Services include:
Data Pipeline Development Automating data extraction, transformation, and loading (ETL) processes for improved efficiency.
Big Data Processing Leveraging cloud-based and distributed computing solutions to manage large datasets effectively.
Data Storage and Integration Implementing scalable data storage solutions that integrate with existing business systems.
Data Quality and Governance Ensuring data accuracy, consistency, and compliance with regulatory requirements.
Real-Time Data Streaming Enabling businesses to process and analyze data in real time for timely decision-making.
The Role of Data Engineering Services in Business Growth
A strong Data Engineering Services strategy helps businesses optimize operations, improve customer experiences, and enhance decision-making. These services ensure that data is efficiently structured and accessible for analytics and AI-driven insights.
Some widely adopted Data Engineering Services solutions include:
Cloud-Based Data Warehousing: Storing and managing data securely in cloud platforms like AWS, Google Cloud, and Azure.
ETL Automation: Reducing manual data processing efforts through automated workflows.
Data Lake Implementation: Centralizing structured and unstructured data for better accessibility and analysis.
AI-Powered Data Transformation: Using machine learning to enhance data classification and anomaly detection.
Benefits of Implementing Data Engineering Services
Organizations that invest in Data Engineering Services gain significant advantages, including:
Improved Data Accuracy and Consistency Ensuring high-quality data for analytics and business intelligence.
Scalable Data Solutions Adapting to growing data needs with flexible infrastructure and cloud-based services.
Faster Decision-Making Accelerating data processing to enable real-time business insights.
Cost Optimization Reducing operational costs through automated and efficient data workflows.
Enhanced Data Security and Compliance Implementing robust data governance policies to protect sensitive information.
Challenges in Adopting Data Engineering Services
Despite the benefits, businesses may face challenges when implementing Data Engineering Services, such as:
Data Complexity and Integration Issues: Managing multiple data sources and formats can be complex.
High Implementation Costs: Initial investments in infrastructure and expertise may be required.
Security and Privacy Concerns: Ensuring data protection in compliance with industry regulations.
Skilled Workforce Requirement: Hiring or training professionals with data engineering expertise.
The Future of Data Engineering Services
As technology advances, Data Engineering Services will continue to evolve to meet new business demands. Future trends in data engineering include:
AI-Driven Data Engineering: Automating data workflows and improving processing efficiency.
Serverless Data Pipelines: Reducing infrastructure management through serverless computing.
Real-Time Data Analytics: Enabling businesses to gain insights instantly from continuous data streams.
Blockchain for Data Security: Enhancing data integrity and transparency in transactions.
Conclusion
Data Engineering Services are critical for businesses looking to maximize the value of their data assets. By implementing effective data engineering strategies, organizations can enhance data accessibility, improve analytics capabilities, and make data-driven decisions with confidence. Investing in Data Engineering Services ensures that businesses stay competitive, agile, and ready for the future of data-driven innovation.
0 notes
Text
Building the Future with Generative AI: A Guide to Gen AI Frameworks
Introduction
As artificial intelligence continues to evolve, businesses and innovators are leveraging generative AI to create new possibilities in content creation, automation, and decision-making. Gen AI Frameworks are at the core of this transformation, providing the necessary tools and architectures to build advanced AI models that drive efficiency, creativity, and personalization. By implementing generative AI solutions, organizations can revolutionize industries such as marketing, design, healthcare, and software development.
Understanding Generative AI and Its Applications
Generative AI refers to artificial intelligence models capable of generating human-like content, including text, images, videos, and even code. Unlike traditional AI systems that analyze and classify data, generative AI learns patterns from vast datasets and produces new, original content. The adoption of Gen AI Frameworks enables businesses to harness the power of generative models to enhance automation, innovation, and user experience.
Key applications of generative AI include:
Content Creation and Personalization AI-powered tools generate unique written content, graphics, and multimedia assets tailored to specific audiences.
Chatbots and Virtual Assistants AI-driven assistants offer real-time, intelligent responses, improving customer service and engagement.
Automated Software Development Generative AI streamlines code generation, bug detection, and software optimization.
Healthcare and Drug Discovery AI models assist in medical research, patient diagnostics, and the creation of new pharmaceuticals.
Design and Creativity Enhancement AI-based tools help artists, designers, and musicians craft unique and innovative projects.
The Role of Gen AI Frameworks
A Gen AI Framework is a set of tools, libraries, and pre-trained models that simplify the development and deployment of generative AI solutions. These frameworks provide the foundation for AI researchers and developers to build, fine-tune, and integrate AI models into real-world applications.
Popular Gen AI frameworks include:
TensorFlow and Keras: Open-source frameworks widely used for deep learning and AI model training.
PyTorch: A flexible and scalable AI framework favored by researchers and developers.
Hugging Face Transformers: A robust library for natural language processing and AI model fine-tuning.
OpenAI’s GPT and DALL·E: Leading-edge AI models that power text and image generation.
Stable Diffusion and MidJourney: Tools for high-quality AI-generated art and design.
Benefits of Implementing a Gen AI Framework
Integrating a Gen AI Framework into business operations provides numerous advantages, including:
Enhanced Productivity Automating content creation and data analysis reduces manual efforts and accelerates workflows.
Cost Efficiency AI-driven solutions minimize operational costs by automating tasks traditionally performed by human workers.
Scalability Generative AI frameworks support the rapid scaling of AI models to meet growing business needs.
Personalization and Customer Engagement AI-powered recommendations and custom content boost user engagement and satisfaction.
Innovative Edge Businesses leveraging generative AI stay ahead of the competition by producing unique, AI-enhanced products and services.
Challenges in Adopting Generative AI and Solutions
Despite its benefits, adopting Gen AI Frameworks presents certain challenges. Companies must navigate issues related to:
Data Privacy and Security: AI-generated content may involve sensitive data, requiring robust security measures.
Ethical Considerations: Generative AI can create misleading or biased content, necessitating ethical guidelines.
Computational Costs: Training and running AI models require significant computing resources. Solution: Cloud-based AI services can mitigate costs.
Integration Complexity: AI implementation may be complex. Solution: Adopting user-friendly AI frameworks simplifies deployment.
Future Trends in Generative AI
As generative AI continues to advance, emerging trends include:
AI-Generated Virtual Worlds: AI will play a major role in gaming, simulations, and metaverse environments.
Multimodal AI Models: Future AI systems will integrate text, image, and video generation for more comprehensive solutions.
AI-Driven Scientific Discoveries: AI will accelerate research in medicine, climate science, and material innovation.
Explainable AI: Enhancing transparency and trust in AI-generated content.
Conclusion
Generative AI is reshaping industries by enabling automation, creativity, and personalization at scale. By leveraging Gen AI Frameworks, businesses can develop powerful AI solutions that drive efficiency and innovation. Investing in generative AI ensures organizations stay competitive, future-proof their operations, and unlock new opportunities in the evolving digital landscape.
0 notes
Text
Enhance Data Management with Databricks: A Comprehensive Approach to Lakehouse Architecture
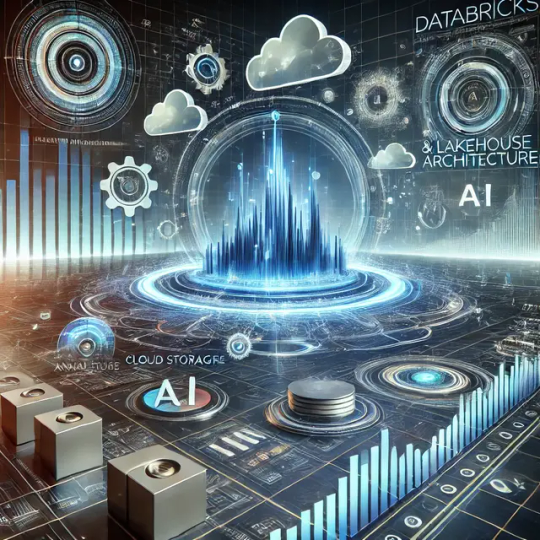
In the modern data landscape, organizations are faced with increasing data complexity and the need for efficient operations to stay ahead of the competition. This calls for innovative solutions that streamline data management while providing scalability and performance. One such solution is Databricks, a unified data analytics platform that accelerates data-driven decision-making. With the power of lakehouse architecture, Databricks offers a comprehensive approach to managing and analyzing large-scale data, blending the best of data lakes and data warehouses.
What is Lakehouse Architecture?
Before diving into how Databricks accelerates data operations with lakehouse architecture, it’s important to understand the concept of lakehouse itself. Traditional data architectures typically rely on either data lakes or data warehouses. While data lakes are ideal for storing large volumes of unstructured data, and data warehouses are optimized for structured data and high-performance queries, each of these models has its limitations.
Lakehouse architecture bridges this gap by combining the best features of both. It allows organizations to store massive amounts of raw, unstructured data in a data lake while enabling the management, performance, and analytics capabilities typically found in a data warehouse. The result is a highly flexible, cost-effective platform for managing both structured and unstructured data.
Lakehouse architecture facilitates real-time analytics and machine learning, providing unified governance and security controls while offering powerful tools for querying, transforming, and processing data. Databricks, built with lakehouse principles at its core, provides an environment where businesses can gain insights quickly, irrespective of data complexity or format.
The Role of Databricks in Accelerating Data Operations
Databricks has emerged as one of the leading platforms in the field of big data analytics. It simplifies the complexities of working with vast datasets by offering an integrated environment that leverages the lakehouse architecture. The key features of Databricks that contribute to faster and more efficient data operations include:
Unified Data Platform: Databricks offers a unified platform that allows organizations to manage their data across multiple environments. Whether the data is structured, semi-structured, or unstructured, Databricks ensures that all of it can be processed, analyzed, and stored in a single framework. This eliminates the need for disparate systems and the complexity of managing multiple data sources, making operations more streamlined and faster.
Scalable Performance: One of the key advantages of lakehouse architecture is its scalability, and Databricks ensures this by offering a highly scalable environment. With cloud-native capabilities, Databricks provides elastic compute resources, allowing organizations to scale their data operations up or down based on demand. This dynamic resource allocation helps maintain optimal performance, even as data volumes grow exponentially.
Delta Lake for Reliable Data Management: Delta Lake, an open-source storage layer developed by Databricks, enhances lakehouse architecture by adding transactional integrity to data lakes. It provides ACID (Atomicity, Consistency, Isolation, Durability) properties to your data, which ensures that data operations such as inserts, updates, and deletes are reliable and consistent. Delta Lake simplifies data engineering workflows by enabling schema enforcement, time travel (data versioning), and easy auditing, ensuring data accuracy and quality.
Real-Time Data Analytics: Databricks empowers organizations to perform real-time analytics on streaming data, which is essential for fast decision-making. The platform enables the continuous ingestion of data and facilitates real-time processing, so organizations can gain actionable insights without delay. This capability is critical for industries such as finance, retail, and manufacturing, where timely data analysis can lead to competitive advantages.
Collaborative Data Science Environment: Another key benefit of Databricks is its collaborative nature. The platform allows data scientists, data engineers, and business analysts to work together on the same projects in real-time. With shared notebooks and integrated workflows, teams can streamline the development of machine learning models and data pipelines, fostering collaboration and enhancing productivity.
Simplified Machine Learning Operations (MLOps): Databricks provides a robust environment for deploying, monitoring, and maintaining machine learning models. This is where the lakehouse architecture shines, as it enables data scientists to work with large datasets, conduct training in parallel across multiple clusters, and deploy models quickly into production. By integrating machine learning with the unified data lakehouse environment, Databricks accelerates the deployment of AI-driven insights.
Benefits of Databricks and Lakehouse Architecture
Databricks, with its integration of lakehouse architecture, offers several benefits that enhance data operations. These benefits include:
Cost Efficiency: Traditional data warehouses often come with high storage and compute costs, especially when handling large datasets. Databricks reduces the cost of data management by utilizing the storage capabilities of data lakes, which are more cost-effective. It optimizes data processing workflows, making it easier to analyze large datasets without incurring hefty infrastructure costs.
Improved Data Governance and Security: As organizations manage larger and more complex datasets, maintaining strong governance and security becomes crucial. Lakehouse architecture, supported by Databricks, ensures that data is properly governed with built-in security features such as role-based access control (RBAC), auditing, and data lineage tracking. These features help businesses comply with regulations and manage sensitive data securely.
Faster Time to Insights: The integration of real-time analytics and machine learning within Databricks ensures that organizations can gain insights faster than traditional data systems allow. By leveraging lakehouse principles, Databricks enables the processing of both historical and streaming data simultaneously, which accelerates the decision-making process. This is particularly beneficial for organizations aiming to stay ahead in rapidly changing industries.
Seamless Integration with Cloud Providers: Databricks supports major cloud platforms, including Amazon Web Services (AWS), Microsoft Azure, and Google Cloud. This makes it easier for businesses to leverage their existing cloud infrastructure and take advantage of the scalability and flexibility that cloud computing offers. With seamless cloud integration, organizations can ensure that their data operations are highly available, resilient, and globally distributed.
Conclusion
In an era where data-driven decision-making is crucial, organizations need platforms that allow them to operate more efficiently and effectively. Databricks, combined with lakehouse architecture, is a powerful solution for transforming how businesses manage, analyze, and gain insights from their data. By combining the scalability of data lakes with the performance of data warehouses, Databricks enables organizations to accelerate their data operations, reduce costs, and ensure data quality and governance.
Whether you're looking to streamline data engineering workflows, implement real-time analytics, or accelerate machine learning model deployment, Databricks offers the tools and capabilities needed to optimize data management. With its robust, unified platform and focus on lakehouse architecture, Databricks empowers businesses to stay competitive in an increasingly data-driven world.
0 notes
Text
Optimizing Operations with Industrial AI Services: Revolutionizing Manufacturing Efficiency
The rapid evolution of technology has transformed nearly every industry, and manufacturing is no exception. With the growing demand for faster production, cost-effectiveness, and innovation, manufacturers are increasingly turning to Industrial AI services to stay competitive. By integrating artificial intelligence (AI) into industrial processes, companies can unlock new levels of efficiency, reduce costs, enhance product quality, and improve decision-making. As AI technology continues to evolve, Industrial AI services are becoming essential tools for businesses looking to streamline their operations and achieve long-term success.
In this article, we will explore how Industrial AI services are revolutionizing manufacturing operations, the benefits they bring, and how companies can leverage them to optimize their manufacturing processes.
What are Industrial AI Services?
Industrial AI services refer to the application of artificial intelligence technologies in industrial sectors, such as manufacturing, supply chain management, and logistics. These services leverage AI, machine learning (ML), deep learning, computer vision, and natural language processing (NLP) to solve complex problems and optimize operations. By using AI to analyze vast amounts of data from sensors, machines, and production systems, companies can make smarter decisions, automate tasks, and identify inefficiencies in real-time.
Industrial AI services help businesses transform their traditional manufacturing operations into data-driven, smart processes. These services can be applied across a wide range of areas, from predictive maintenance and quality control to production optimization and resource management.
Key Benefits of Industrial AI Services in Manufacturing
1. Predictive Maintenance
One of the most significant advantages of Industrial AI services is the ability to predict equipment failures before they occur. In a traditional manufacturing environment, unexpected machine breakdowns can lead to significant downtime, lost production, and costly repairs. However, with AI-driven predictive maintenance, manufacturers can monitor the health of machines in real-time by analyzing data from sensors embedded in the equipment.
By using machine learning algorithms to detect patterns and anomalies, AI systems can predict when a machine is likely to fail, allowing maintenance teams to address issues before they lead to costly breakdowns. This reduces downtime, minimizes repair costs, and extends the lifespan of machinery.
For example, Industrial AI services can monitor the vibrations, temperature, and sound levels of machines, alerting operators to potential issues like bearing failures or overheating. By catching these issues early, manufacturers can schedule maintenance during off-peak times, ensuring that production runs smoothly without interruptions.
2. Improved Quality Control
In manufacturing, maintaining high product quality is crucial to customer satisfaction and brand reputation. Industrial AI services play a vital role in ensuring that products meet the required quality standards by automating quality control processes. Traditional quality control methods rely on manual inspections, which are often time-consuming and prone to human error.
With Industrial AI services, manufacturers can implement AI-powered quality control systems that automatically inspect products for defects, inconsistencies, or flaws. For example, computer vision algorithms can be used to scan products on production lines, detecting any imperfections that might go unnoticed by the human eye. AI can also analyze sensor data to identify deviations from predefined quality parameters, such as temperature or pressure thresholds.
By automating quality control, manufacturers can increase the accuracy of inspections, reduce the risk of defective products reaching customers, and lower costs associated with rework and scrap. Furthermore, AI-powered systems can continuously learn from the data they collect, improving their accuracy over time and adapting to new quality standards.
3. Production Optimization
Optimizing production processes is another area where Industrial AI services are making a significant impact. Manufacturing companies face the challenge of meeting customer demands while minimizing production costs and maintaining efficiency. AI can help manufacturers optimize production scheduling, resource allocation, and workflow management.
AI-powered systems can analyze data from various sources, such as inventory levels, production schedules, and machine performance, to create the most efficient production plans. These systems can also predict demand fluctuations, allowing manufacturers to adjust production levels accordingly. This helps prevent overproduction or underproduction, reducing waste and ensuring that products are available when customers need them.
In addition, AI can be used to optimize energy consumption and reduce waste in the production process. By analyzing real-time data on energy usage and production efficiency, AI systems can suggest ways to minimize energy consumption without compromising output, leading to significant cost savings and a smaller environmental footprint.
4. Supply Chain Optimization
A well-managed supply chain is essential for efficient manufacturing, and Industrial AI services can enhance supply chain performance by providing better visibility and improving decision-making. AI can be used to predict demand, monitor inventory levels, and optimize logistics, ensuring that materials and components are available when needed without overstocking.
For example, AI systems can forecast demand patterns based on historical data, seasonal trends, and market conditions. By using this data, manufacturers can plan their supply chain activities more accurately, reducing lead times, minimizing stockouts, and preventing excess inventory. Furthermore, AI can help optimize transportation routes, lowering shipping costs and improving delivery times.
AI can also be used to monitor the performance of suppliers and identify potential risks in the supply chain. By analyzing factors such as supplier reliability, lead times, and quality, AI systems can flag any potential issues before they impact production schedules, allowing manufacturers to take corrective action.
5. Enhanced Decision-Making
Industrial AI services enable manufacturers to make data-driven decisions by providing real-time insights into various aspects of their operations. AI can process large volumes of data from sensors, production lines, and other sources to identify trends, patterns, and potential issues. This allows decision-makers to act quickly and make more informed choices.
For instance, AI-powered analytics can provide real-time reports on production performance, allowing managers to monitor key performance indicators (KPIs) such as throughput, cycle time, and downtime. By having access to these insights, manufacturers can make adjustments to optimize production processes, improve resource allocation, and reduce inefficiencies.
AI can also assist in strategic decision-making, such as identifying new markets, optimizing pricing strategies, or evaluating the financial viability of new product lines. By leveraging AI-driven insights, manufacturers can stay ahead of the competition and make decisions that drive long-term growth and profitability.
How to Implement Industrial AI Services
To successfully integrate Industrial AI services into manufacturing operations, businesses need to take a strategic approach. Here are some key steps to consider:
Identify Key Areas for AI Integration: Start by assessing which parts of your manufacturing process could benefit the most from AI. This could include areas like predictive maintenance, quality control, production optimization, or supply chain management.
Collect and Prepare Data: AI systems rely on high-quality data to function effectively. Ensure that your business has access to accurate, real-time data from sensors, machines, and other sources. Cleaning and organizing this data is crucial for ensuring that AI models can make accurate predictions.
Choose the Right AI Solutions: There are various AI tools and platforms available, each tailored to different industrial applications. Work with an AI provider that understands your specific industry needs and can help you implement solutions that align with your business objectives.
Train Employees: While AI can automate many tasks, it's essential to ensure that your workforce is equipped to work alongside AI-powered systems. Provide training to employees on how to use AI tools and interpret the insights they generate. This will help ensure a smooth transition and maximize the benefits of AI integration.
Monitor and Optimize: Once AI services are integrated, continuously monitor their performance and make adjustments as needed. AI systems should be regularly updated and optimized to ensure that they remain effective and continue to provide value.
Conclusion
Industrial AI services are transforming the manufacturing sector by enabling businesses to optimize their operations, reduce costs, and improve efficiency. From predictive maintenance and quality control to production optimization and supply chain management, AI is empowering manufacturers to make smarter decisions and stay ahead of the competition.
As the demand for more efficient and cost-effective manufacturing processes continues to rise, adopting Industrial AI services will be crucial for businesses looking to thrive in the future. By embracing AI, manufacturers can unlock new levels of productivity, innovation, and growth, positioning themselves for long-term success in an increasingly data-driven world.
0 notes
Text
Shaping Tomorrow: The Power of Generative AI in Innovation
In the rapidly evolving world of technology, artificial intelligence (AI) has emerged as a key driver of innovation, reshaping industries and redefining how humans interact with digital ecosystems. Among the various branches of AI, Generative AI stands out as one of the most transformative forces, enabling machines to create content, design products, and generate solutions that were previously thought to be the exclusive domain of human intelligence. This article explores the profound impact of Generative AI on technological advancement and how it is shaping the future across multiple industries.
Understanding Generative AI
Generative AI refers to artificial intelligence systems that can create new content, including text, images, music, and even complex data models. Unlike traditional AI models, which primarily analyze and classify existing data, Generative AI learns patterns and structures from vast datasets to generate original and meaningful outputs. Technologies like Generative Adversarial Networks (GANs), transformers, and large language models (LLMs) such as GPT-4 have made significant strides in enhancing the creative and problem-solving capabilities of AI.
The applications of Generative AI extend far beyond simple automation. It is now being leveraged to design artwork, write essays, develop code, and even simulate human-like conversations. This ability to generate new and unique outputs has unlocked immense possibilities, making Generative AI a valuable tool for businesses, artists, researchers, and innovators alike.
Transforming Industries with Generative AI
Generative AI is not confined to a single domain; it has found applications across multiple industries, driving efficiency, creativity, and productivity.
1. Healthcare and Drug Discovery
Generative AI is revolutionizing healthcare by accelerating drug discovery, personalizing treatment plans, and enhancing medical imaging. AI-driven models can simulate molecular interactions and generate potential drug candidates in a fraction of the time it takes through traditional research methods. Additionally, AI-powered diagnostic tools help doctors detect diseases earlier and provide more accurate medical assessments.
2. Content Creation and Media
From journalism to entertainment, Generative AI is reshaping how content is produced. AI-generated articles, scripts, and music compositions are becoming more prevalent, enabling creators to automate routine tasks and focus on more strategic storytelling elements. AI models like DALL·E and MidJourney are creating visually stunning images, while platforms like ChatGPT assist writers in drafting compelling content.
3. Software Development and Code Generation
Generative AI is making software development more efficient by automating coding processes. AI-driven tools can generate, debug, and optimize code, reducing the time and effort required for software engineering projects. Platforms like GitHub Copilot use AI to suggest code snippets and help developers write efficient programs, significantly improving productivity.
4. Marketing and Customer Engagement
Businesses are increasingly using Generative AI to enhance their marketing strategies and customer engagement efforts. AI-driven chatbots and virtual assistants provide personalized interactions, while AI-generated advertisements and promotional content help brands reach their audiences more effectively. Predictive analytics powered by AI also enable businesses to anticipate customer preferences and tailor their marketing campaigns accordingly.
5. Education and Personalized Learning
The education sector is benefiting from Generative AI through personalized learning experiences and automated tutoring systems. AI-driven platforms analyze students' learning patterns and generate customized study plans, making education more adaptive and accessible. Interactive AI tutors can also provide real-time feedback, helping students grasp complex concepts more effectively.
Ethical Considerations and Challenges
While Generative AI offers numerous benefits, it also raises ethical concerns and challenges that must be addressed. Issues such as misinformation, deepfakes, and biased AI outputs pose significant risks. Ensuring transparency, accountability, and responsible AI development is crucial to mitigating these challenges.
Organizations and policymakers must establish guidelines for ethical AI use, promote diversity in training datasets, and implement mechanisms to detect and prevent malicious AI-generated content. By addressing these challenges proactively, we can harness the power of Generative AI while minimizing its potential risks.
The Future of Generative AI
As Generative AI continues to evolve, its impact on society and industries will only deepen. Advancements in AI research, increased computing power, and improved machine learning algorithms will further enhance the capabilities of Generative AI systems. We can expect AI to become an even more integral part of creativity, automation, and decision-making processes in the coming years.
To stay ahead in this AI-driven future, businesses and individuals must embrace continuous learning and adaptation. Leveraging Generative AI effectively will require a combination of technical expertise, ethical considerations, and innovative thinking.
1 note
·
View note