#predictivemodelling
Explore tagged Tumblr posts
Photo
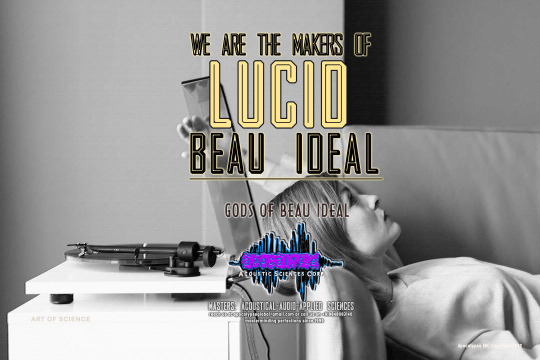
#Truthful #Tuesdays
#Life is all #about #being #Alive
So is #your #room or #space #alive?
So is your room or space alive or #dead. #know #more.
Answers @Apocalypse, we are the makers of perfection.
One of Apocalypse’s primary #approaches to #acoustical #design is to #physically #shape the #room or #space so that #sound #waves #bounce off the #walls in a #way that #sounds #good when they #hit your #eardrums. We call this #Room #Shaping. Ever heard of this #shaping for #your room or space.
#Wakeup #humankind, this is not the #chore of amateurs. It’s the #sapience of the #pros.
However, it does #take more than #slapping a #few #acoustic #panels or #designer #shapes and #colors up on the #walls to #fully #shape the #acoustical #response of a #room or a #space. We need a #right balance between #apotheoses with #hypothesis.
#distinct #discrete #decided #adept #perfections #accessible @apocalypse acoustics
Call us +919848083140 or email us at [email protected] to know the #veracity of #science.
Kindly Note: We do provide #redemptive #perfections on #conditional #relations. #Apply #Now.
https://apocalypsesite.wordpress.com/2022/04/05/so-is-your-room-or-space-alive/
#acousticalsciences#acousticalengineering#acoustical consultants#acoustic consultants#predictivemodelling#audiovisual#audioengineering#mediaengineering#appliedengineering#audiosciences#officeacoustics#openplanoffice
1 note
·
View note
Text
#Nuggets in Sound
#Sound vs. #Acoustics
#Mondays #Lateral #Reflections
#Lateral #reflections also #increase #perceived #loudness more than #sound #reflections #coming from other #directions.
#distinct #discrete #decided #adept #perfections #accessible @apocalypseglobal

#architecturalacoustics#electroacoustics#predictivemodelling#acoustic treatment#acousticpredictions#soundmodelling#noisemapping#acousticalsciences#audiosciences#appliedsciences#acousticalengineering#audioengineering#appliedengineering#mediaengineering#churchpa#churchacoustics#livesound#installations#integration#consultants#audiovisual#audioschool
1 note
·
View note
Text
Lessons Learned: Kaggle Titanic Competition using R
I've attempted my first Kaggle competition in December 2014 and achieved top 5% as of the date of this post. Although the competition is one of the entry level ones, it's a big step for me to move towards the world of data science :)
In this post, I'd like to document some of the achievements, share a few lessons learned for future reference, and provide my codes in Github.
Achievement as of February 01, 2015:
Submission History as of February 01, 2015:
Summary of my key result improvement with different algorithms and features selections
Key take-away
1. More data (dimensions) does not always mean better. So we need to be critical about Big, aka more data, in Big Data. Relating back to my previous post of Jeremy Howard's quote, the prediction algorithm works quite well in fact with small sets of data (the training data set is around 61kb).
2. Feature engineering, a process that develop new dimension based on existing ones, is both science and art; It requires logical scientific thinking as well as creative trial & error
3. The right algorithm and feature selection has to be applied in order to deliver performance
Next Steps: Revisit the code once I come across more advanced modelling techniques
My codes: https://github.com/ianxxiao/kaggle-titanic
0 notes
Text
#Mondays #Healthcare
#Sound vs. #Acoustics
#Healthcare #acoustics #pose a #wide #range of #complex and #diverse #challenges for #hospital and #healthcare #facilities #managers. Our #acoustical and AV #design #consultants #specialize in healthcare acoustics, providing #design #solutions for #new #construction, #remodelling, #Isolation #Wards, and #retrofit #healthcare #projects.
#Freedom from #physical #psychological #unwellness #illness #viruses or #nausea
#Successive #solutions for #direct or #indirect #Isolations in #noise @acousticsguru


1 note
·
View note