#small-target-cnn-architectures
Explore tagged Tumblr posts
Text
HyperTransformer: G Additional Tables and Figures
Subscribe .tade0b48c-87dc-4ecb-b3d3-8877dcf7e4d8 { color: #fff; background: #222; border: 1px solid transparent; border-radius: undefinedpx; padding: 8px 21px; } .tade0b48c-87dc-4ecb-b3d3-8877dcf7e4d8.place-top { margin-top: -10px; } .tade0b48c-87dc-4ecb-b3d3-8877dcf7e4d8.place-top::before { content: “”; background-color: inherit; position: absolute; z-index: 2; width: 20px; height: 12px; }…
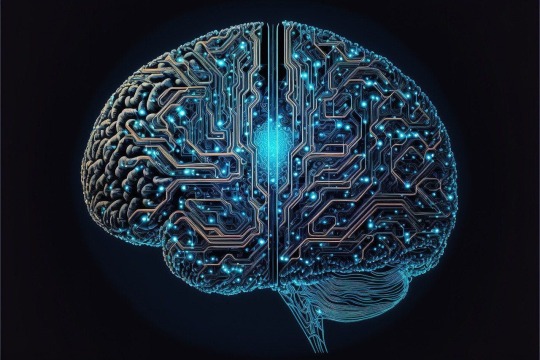
View On WordPress
#conventional-machine-learning#convolutional-neural-network#few-shot-learning#hypertransformer#parametric-model#small-target-cnn-architectures#supervised-model-generation#task-independent-embedding
0 notes
Text
Application of Hybrid CTC/2D-Attention end-to-end Model in Speech Recognition During the COVID-19 Pandemic
Application of Hybrid CTC/2D-Attention end-to-end Model in Speech Recognition During the COVID-19 Pandemic in Biomedical Journal of Scientific & Technical Research
Speech recognition technology is one of the important research directions in the field of artificial intelligence and other emerging technologies. Its main function is to convert a speech signal directly into a corresponding text. Yu Dong, et al. [1] proposed deep neural network and hidden Markov model, which has achieved better recognition effect than GMM-HMM system in continuous speech recognition task [1]. Then, Based on Recurrent Neural Networks (RNN) [2,3] and Convolutional Neural Networks (CNN) [4-9], deep learning algorithms are gradually coming into the mainstream in speech recognition tasks. And in the actual task they have achieved a very good effect. Recent studies have shown that endto- end speech recognition frameworks have greater potential than traditional frameworks. The first is the Connectionist Temporal Classification (CTC) [10], which enables us to learn each sequence directly from the end-to-end model in this way. It is unnecessary to label the mapping relationship between input sequence and output sequence in the training data in advance so that the endto- end model can achieve better results in the sequential learning tasks such as speech recognition. The second is the encodedecoder model based on the attention mechanism. Transformer [11] is a common model based on the attention mechanism. Currently, many researchers are trying to apply Transformer to the ASR field. Linhao Dong, et al. [12] introduced the Attention mechanism from both the time domain and frequency domain by applying 2D-attention, which converged with a small training cost and achieved a good effect.
And Abdelrahman Mohamed [13] both used the characterization extracted from the convolutional network to replace the previous absolute position coding representation, thus making the feature length as close as possible to the target output length, thus saving calculation, and alleviating the mismatch between the length of the feature sequence and the target sequence. Although the effect is not as good as the RNN model [14], the word error rate is the lowest in the method without language model. Shigeki Karita, et al. [15] made a complete comparison between RNN and Transformer in multiple languages, and the performance of Transformer has certain advantages in every task. Yang Wei, et al. [16] proposed that the hybrid architecture of CTC+attention has certain advancement in the task of Mandarin recognition with accent. In this paper, a hybrid end-to-end architecture model combining Transformer model and CTC is proposed. By adopting joint training and joint decoding, 2DAttention mechanism is introduced from the perspectives of time domain and frequency domain, and the training process of Aishell dataset is studied in the shallow encoder-decoder network.
For more articles in Journals on Biomedical Sciences click here bjstr
Follow on Twitter : https://twitter.com/Biomedres01 Follow on Blogger : https://biomedres01.blogspot.com/ Like Our Pins On : https://www.pinterest.com/biomedres/
#Journals on Infectious Diseases Addiction Science and clinical pathology#Open Access Clinical and Medical Journal#Journals on Medical Drug and Therapeutics#journal of biomedical research and reviews impact factor#Journals on Biomedical Engineering
0 notes
Text
Sensors, Vol. 23, Pages 2219: An Optimized Ensemble Deep Learning Model for Predicting Plant #miRNA–I#ncRNA Based on Artificial Gorilla Troops Algorithm
Micro#RNAs (#miRNA) are small, non-coding regulatory molecules whose effective alteration might result in abnormal gene manifestation in the downstream pathway of their target. #miRNA gene variants can impact #miRNA transcription, maturation, or target selectivity, impairing their usefulness in plant growth and stress responses. Simple Sequence Repeat (SSR) based on #miRNA is a newly introduced functional marker that has recently been used in plant breeding. #microRNA and long non-coding #RNA (l#ncRNA) are two examples of non-coding #RNA (#ncRNA) that play a vital role in controlling the biological processes of animals and plants. According to recent studies, the major objective for decoding their functional activities is predicting the relationship between l#ncRNA and #miRNA. Traditional feature-based classification systems’ prediction accuracy and reliability are frequently harmed because of the small data size, human factors’ limits, and huge quantity of noise. This paper proposes an optimized deep learning model built with Independently Recurrent Neural Networks (IndRNNs) and Convolutional Neural Networks (CNNs) to predict the interaction in plants between l#ncRNA and #miRNA. The deep learning ensemble model automatically investigates the function characteristics of genetic sequences. The proposed model’s main advantage is the enhanced accuracy in plant #miRNA–I#ncRNA prediction due to optimal hyperparameter tuning, which is performed by the artificial Gorilla Troops Algorithm and the proposed intelligent preying algorithm. IndRNN is adapted to derive the representation of learned sequence dependencies and sequence features by overcoming the inaccuracies of natural factors in traditional feature architecture. Working with large-scale data, the suggested model outperforms the current deep learning model and shallow machine learning, notably for extended sequences, according to the findings of the experiments, where we obtained an accuracy of 97.7% in the proposed method. https://www.mdpi.com/1424-8220/23/4/2219?utm_source=dlvr.it&utm_medium=tumblr
0 notes
Text
WordPress Help, Support: Can Need Support, Help of WordPress Enterprise?
WordPress actually powers 30% of all websites. It's an incredible figure, but it's sometimes overlooked by larger corporations — and with good reason. It's common knowledge that those figures are fabricated by teeny-tiny blogs and a website produced by, well, just about anyone. But here's the thing: while the above figure may be easily ignored by businesses looking for a CMS, WordPress remains a key player in the world's top echelon of website. WordPress controls roughly 26% of the top 10,000 websites on the internet, according to BuiltWith, and 14 of the top 100 websites in the world are powered by WordPress.
Big-name companies like CNN, Forbes, and UPS use WordPress as the foundation of their online presence. Is that confidence, however, misplaced? Is WordPress capable of supporting some of the industry's biggest digital players?
The Case for WordPress Help as a Business Platform

CMS -Grade
First and foremost, consider why such well-known brands have chosen WordPress as their web content management system.
1. Add-ons
There are over 50,000 plugins in the WordPress Plugin Directory. These plugins will help businesses add a variety of features and functionality to their WordPress site. “Plugins help you ensure good SEO, protection, social media sharing options, and so much more,” said Andrew Stanten, president of Emmaus, PA-based Altitude Marketing. When compared to other enterprise CMS solutions, he said the broad range of available WordPress plugins allows businesses to customize and extend the functionality of their website with relative ease.
2. Capabilities for integration
WordPress, like a more conventional enterprise-grade CMS, will interact with "several business-critical platforms," such as CRM systems and marketing automation platforms, according to Staten. “By streamlining the website and other business-operational resources, the company and its sales [teams] are able to get information into the sales cycle faster and more efficiently than they could with disparate systems,” he said.
3. Capabilities without a head
Though WordPress does not come with a built-in headless CMS, the advent of the WordPress REST API means it can be used as one. Enterprises who want to stay important in the IoT era need headless content management capabilities, as platforms grow with the advent of smart voice assistants, smart wearables, and more. Matt Brooks, CEO of SEOteric in Watkinsville, GA, discusses how WordPress can be used as a coupled or headless solution. “The Wordpress REST API can be used to populate content on almost any platform that supports APIs. WordPress may be used as a headed CMS solution with custom views for various content types, depending on the application. It can also be used as a headless CMS, with data being funneled to other applications via API.” Nevena Tomovic, business development manager at Human Made in Derbyshire, England, described how the company uses WordPress' headless capabilities to create scalable, enterprise sites. “We use open source technology to build out digital products that scale because the core of what we do is focused on WordPress. “Basically, we create custom workflows for major media companies like TechCrunch so they can publish and manage content in real time,” she explained. Human Made assisted TechCrunch in adopting the WordPress REST API to decentralize its publishing experience. As a result, TechCrunch was able to keep the WordPress backend's simplicity while still using the REST API to build a user-friendly frontend on any platform or touchpoint.
4. Usability
The ease of use of WordPress in almost all aspects of the backend interface is a major reason for its success. Sure, things can get complicated, but as Stanten points out, WordPress appeals to advertisers and non-technical users because they can quickly "update the website after its launch."
The Case Against WordPress as a Business-Grade Content Management System
WordPress isn't just sunshine and rainbows in the business world. Indeed, WordPress's obvious limitations and challenges keep it from being a truly ideal fit for enterprise businesses, especially those outside of the publishing industry.
1. The ability to scale
Despite headless capabilities that help businesses scale their content delivery platforms, Matthew Baier, COO of Contentstack in San Francisco, sees WordPress' scalability problems as a disadvantage: “The issue arises as the website begins to scale, which occurs when you add more content [and] resources to the equation. When you start relying on a website for a critical part of your business, your needs for a CMS will change drastically, and what started out as a simple and inexpensive way to develop your site can easily spiral into a highly complicated and costly environment to manage down the road,” Baier explained. Baier went on to say that even with a simple WordPress website with only a few plugins, you'll quickly notice that "something goes bump in the night." Almost every single night.” “At other words, you wake up every morning with a new collection of problems in your CMS that need to be addressed. Third-party tools and open source are great for innovation, but troubleshooting such a specialized software stack — let alone getting enterprise-grade support — can be difficult, according to Baier.
The plugin-based architecture of WordPress, according to Christian Gainsbrugh, founder of Seattle-based LearningCart, can quickly become a disadvantage for enterprise-scale sites and applications. “Because WordPress is capable of so many things, the temptation is to use it for everything. This works well on a smaller scale, but the data structure that makes Wordpress so flexible becomes horribly inefficient to query at the enterprise level,” he said. “When you try to use WordPress for things like powering an ecommerce store or using plugins to capture data like complex feedback forms, this becomes an issue. In those cases, you'll have to rely heavily on metadata tables to track custom fields, which means that looking up a single record could take up to 50 queries, according to Gainsbrugh.
2. Support of WordPress: Safety and security
The open-source nature of themes, widgets, and plugins, according to Shawn Moore, Global CTO at Orlando, Fla.-based Solodev, can make WordPress extremely vulnerable to cyber attacks. “Many of these plugins aren't well-supported by their developers (and, in many cases, come from untrustworthy sources), and may contain exploitable code or 'backdoors' that make them vulnerable to malicious code, malware injections, spyware, and other threats.” “Security is another area where WordPress falls short for enterprises,” Baier added on this front. WordPress-powered websites have an automatic target on their back due to the CMS's widespread use. Yes, you can secure a WordPress environment, but it takes diligence and vigilance, and even the tiniest lapse in updating can have immediate and disastrous consequences."
3. Issue with the Americans with Disabilities Act (ADA)
Moore continued his anti-WordPress statement by claiming that certain WordPress plugins and widgets placed businesses at risk of non-ADA enforcement. “Large corporations such as Target, Five Guys Burgers and Fries, Charles Schwab, and Safeway have all spent millions of dollars settling litigation after their websites were found to be in violation of the Web Content Accessibility Guidelines 2.0. “WordPress [plugins and widgets] aren't kept to ADA guidelines, but the company website that uses the widget or plugin bears the responsibility, not the developers,” Moore explained.
4. A lack of business support
To ensure that their digital presence is live, stable, and performing well, businesses need round-the-clock support. “Despite having a large group of development experts, there is no transparency or guarantee for its results, and no way to reach a dedicated support individual during a crisis,” Moore said of WordPress's lack of enterprise-level support. Enterprise companies are forced to troubleshoot on their own or find an expensive third-party option because of inconsistent documentation,” he said.
Is WordPress Appropriate for Enterprise Use?
Is WordPress capable of meeting the digital needs of a large corporation? Yes, to put it simply. It can easily integrate with third-party tools, is simple to use, and can even handle content without the need for human intervention. But, more importantly, do you use WordPress to power your large-scale web presence? This is all up in the air. One thing is certain: as WordPress grows in size, it becomes a pain to manage. Also small website owners can testify to this fact.
Updates fail, plugins break, developers abandon themes, hackers attack your web, and you can quickly end up with a patchwork digital presence that resembles a house of cards rather than an enterprise-grade CMS. For businesses that rely on using WordPress, the solution seems to be working with a WordPress-centric organization that can keep a close eye on their WordPress-powered environment — anything less will likely result in the house of cards collapsing.
We're your own personal WordPress helpdesk, support.
WordPress Support Services Available On-Demand No matter who designed it or where it's hosted, you'll get comprehensive support for your WordPress website.
The Best WordPress Support Service in the World
Our clients adore us!
WHAT WE DO ONCE-ONLY REPAIR
Website Management Mobile Websites Custom Development PageSpeed SEO Security PageSpeed Search Engine HOW DOES IT WORK? LOG IN TO THE BLOG PRICING
We're your own personal WordPress helpdesk.
WordPress Support Services Available On-Demand
No matter who designed it or where it's hosted, you'll get comprehensive support for your WordPress website.
WHAT WE DON'T DO
ONE-TIME REPAIR
Exactly what we do
WPHelp Center relieves you of the responsibility of managing your WordPress website, from friendly user support to dependable website maintenance and custom creation.
An expert in Help and troubleshooting for WordPress
Edits to the site and bug fixes Customized development Control of a website PageSpeed & Mobile-Friendly Design SEO for eCommerce WordPress's safety
We've got you covered.
Anything you'll need to keep your website in good shape. So you can focus on growing your company, we keep your website safe, healthy, and up-to-date.
Support for your website and a one-time fix
Do you need assistance with your WordPress website? We will assist you with fixing a broken site, making page edits, or creating new pages or features.
Page Speed Improvements
Are you frustrated by your low PageSpeed score? We will assist you in achieving top speed scores and lightning-fast loading times for your WordPress pages.
Optimization for mobile devices
Mobile now accounts for more than half of all internet traffic. We'll make sure that your WordPress stage is mobile-friendly.
WordPress Safety and Security
Is your WordPress account protected from hackers? Enjoy the peace of mind that comes with understanding that your website is constantly guarded against attacks.
Website Administration
Comprehensive WordPress website support, regardless of where it's hosted or who created it. We'll take care of the technology and growth. Allow our professionals to back up, upgrade, and track the security of your website while you concentrate on running your company. WPHelp.Center makes it simple to get started with WordPress support.
Tags: site, however, take care, woocommerce, take, ongoing, contact, team, requests, call us, speed, issue, error, need, job, hosting, see, necessary, one, fix, errors, request, time, free time, Wordpress, see small job for blog, need job, need experts, need experts, error fix, woocommerce info, great things about woocommerce, however take care, things, experts for error or issue fix, great woocommerce job, however contact for issue, however contact for error fix, fix speed issue, necessary team to fix the error, need strong team, request to experts to fix errors, build a free Wordpress, free request for Wordpress, WordPress errors fix, WP Website help, support, support wordpress, help, WP Website Help, free help or support of wordpress , time to fix error, website help, wordpress support for fix, see support for wordpress, take support wordpress , things see contact, help contact, take care, take care support or help, plans, small plans give for hosting, hosting plans, works for website, take care, updates of works, contact for works, free Wordpress plugins, fix Wordpress, Wordpress plan, add Wordpress team, Wordpress speed plan, fix wrodpress hosting issue, see Wordpress hosting, Wordpress service, Wordpress best site, add Wordpress best site, free Wordpress site, free Wordpress site speed check, Wordpress team for help, wordpress speed checking, support for wordpress, help for wordpress, help for free wordpress, take support of help service, wordpress site help, high speed, one chat to fix website speed test, team for wp website hosting, wordpress help,wordpress help for support, wordpress support or wordpress help, support or Help of Wordpress, free best support, help Wordpress, Chat a team for wesbiste speed,
WordPress Custom Development
WPHelp Center will take care of any custom development request, from minor tweaks to large-scale enterprise projects.
Platforms, features, and systems that are new
We specialize in developing and implementing WordPress-based a website, online utilities, and dedicated systems.
Developers who have been hand-picked
Your creation work will be completed by hand-selected, world-class WordPress experts who have gone through a rigorous submission and screening process.
You can trust our services.
Developers who are professionals
You'll task with top WordPress developers who can handle any project you throw at them.
a short processing period
We work on your requests on a regular basis, with most tasks taking 24-48 hours to complete.
Calls on Complementary Strategies
For your website, advice on SEO, technology choices, and digital strategy is available.
365 days a year, 24 hours a day
We're still on call, watching over your site and ready to assist.
Help from a Human Being
You'll be assigned a customer success manager in the United States that you can contact directly.
100% Customer Satisfaction
You'll enjoy making a website once more with WPHelp Center.
Details to Know Follow: https://wptangerine.com/wordpress-help/
Additional Resources: https://wordpress.com/learn/ https://wordpress.org/support/article/new-to-wordpress-where-to-start/ https://en.wikipedia.org/wiki/WordPress
0 notes
Link
Analysis: Volkswagen could soon steal Tesla's crown After years as the undisputed king of the electric car, Tesla (TSLA) could be matched sale for sale by Volkswagen (VLKAF) as early as 2022, according to analysts at UBS, who predict that Europe’s biggest carmaker will go on to sell 300,000 more battery electric vehicles than Tesla in 2025. Ending Tesla’s reign would be a huge milestone in Volkswagen’s transformation into an electric vehicle powerhouse. Badly burned by its diesel emissions scandal in 2015, Europe’s largest carmaker is investing €35 billion ($42 billion) in electric vehicles, staking its future on new technology and a dramatic shift away from fossil fuels. “Tesla is not only about electric vehicles. Tesla is also very strong in software. They really run the car as a device. They are making good progress on the autonomous thing. But yes … we are going to challenge Tesla,” Volkswagen CEO Herbert Diess told CNN’s Julia Chatterley on Tuesday. Volkswagen this week underscored the scale of that ambition. It said it would sell more than 2 million electric vehicles by 2025, build its own network of vast battery factories, hire 6,500 IT experts over the next five years, launch its own operating system, and become Europe’s second biggest software company behind SAP (SAP). UBS analysts told reporters last week that investors have failed to appreciate the speed at which Volkswagen is gaining ground on Tesla, and how much money the German company stands to make by going “all in” on electric cars before other established carmakers including Toyota (TM) and General Motors (GM). UBS has hiked its target price for Volkswagen shares by 50% to €300 ($358). “We have more confidence than ever that Volkswagen will deliver the unique combination of volume growth, making them the world’s largest [electric] carmaker, together with Tesla, as soon as next year, while their margins will be stable or even grow from here. That is something that is totally unappreciated,” said UBS analyst Patrick Hummel. Volkswagen, which owns Porsche, Audi, Skoda and SEAT, sold 231,600 battery electric vehicles in 2020. That’s less than half the number of sales Tesla made, but it represents an increase of 214% on the previous year. Rapid growth is expected to continue as Volkswagen launches 70 electric vehicles before the end of the decade. It will operate eight electric vehicle plants by 2022, producing models in nearly every segment — from small cars to SUVs and luxury sedans. The global race to electric car market domination will come down to the Californian upstart and the German industrial giant, according to UBS. It predicts that Volkswagen will exceed its own goal by producing 2.6 million electric vehicles in 2025, followed by Tesla with 2.3 million. Toyota, which sold more cars than anyone else last year, ranks a distant third with 1.5 million electric sales (excluding hybrids). Hyundai Motor Group (HYMTF) and Nissan (NSANF) will churn out roughly 1 million vehicles, followed by General Motors with 800,000. Shifting into overdrive Volkswagen is in a better position than its rivals because of its modular production platform, or MEB. The platform, which was used to produce the ID.3, an electric compact hatchback, will allow the carmaker to quickly produce a huge number of vehicles while slashing costs. UBS estimates that manufacturing an ID.3 currently costs Volkswagen €4,000 ($4,770) more than producing an equivalent Golf powered by gasoline or diesel. But a sharp decline in the cost of battery packs — the single most expensive part of an electric vehicle — mean the difference in production costs will be eliminated by 2025, according to UBS. Volkswagen on Monday unveiled plans to open six battery-making “gigafactories” in Europe by 2030, with the aim of slashing the cost of its battery cells by as much as 50%. “Lower prices for batteries means more affordable cars, which makes electric vehicles more attractive for customers,” said Diess. The huge scale of production at Volkswagen, which sold 9.3 million cars last year, will also help reduce costs. In addition to the MEB, the group is developing a separate platform for premium brands Audi and Porsche, allowing it to launch electric vehicles across its entire product range. Investors are starting to reward the company. Shares in Volkswagen jumped 6.5% to €207 ($247) on Tuesday, bringing gains so far this year to 35%. Where Tesla leads Despite the recent rise in its share price, Volkswagen is worth significantly less than Tesla. The market value of the challenger led by Elon Musk first overtook that of Volkswagen in January 2020, and the gap has increased dramatically since then. Volkswagen has a market capitalization of €111 billion ($133 billion), compared to $680 billion for Tesla. Part of the difference can be explained by Tesla’s continued superiority in battery costs, software and the profitability of its electric cars. According to UBS, Tesla has “a more sophisticated IT hardware architecture,” and its “software organization is on a different level.” Volkswagen lags Tesla in autonomous driving technology by several years. Some investors believe that Tesla will be able to capitalize on its software advantage in a big way. In addition to delivering wireless updates to its cars — a concept the company pioneered — Tesla may soon be able to do things like charge owners a subscription fee to use its autonomous driving software. It’s much closer to changing the nature of car ownership than established carmakers such as Volkswagen. UBS reckons that the earnings potential from software accounts for roughly two thirds, or $400 billion, of Tesla’s market value. “We think the lion’s share of this [$400 billion in] value can be generated by software, mainly autonomous driving. With that, Tesla has the potential to become one of the most valuable software companies,” its analysts wrote. There could be setbacks along the way, however. Tesla recently expanded its “full self-driving” software to roughly 2,000 owners, but some drivers had their access revoked for not paying close enough attention to the road. Dan Ives, an analyst at Wedbush Securities, said earlier this year that the electric vehicle market is “Tesla’s world and everyone else is paying rent.” But with 150 carmakers all pursuing the same goal, Tesla will need to execute on its strategy, he added. “While growth will be key, its profitability profile will be under the microscope from investors going forward to better discern how quickly Tesla can ramp its margin structure, especially with higher margin sales coming out of China over the next few years,” said Ives. For both Volkswagen and Tesla, their ambition to dominate the electric car market depends on their ability to become more like the other. Volkswagen needs to quickly upgrade its software capabilities, while Tesla would benefit from the German company’s ability to churn out million upon millions of high-quality vehicles each year. Volkswagen announced earlier this month that the first wireless software updates would be delivered to the ID.3 this summer. Ives predicts that Tesla will be making 1 million cars a year in 2022, and could be approaching 5 million annually by the end of the decade. With consumers now searching out electric cars, especially in Europe, the race between Volkswagen and Tesla will accelerate quickly. According to UBS, electric cars will make up 20% of global new vehicles sales in 2015 and 50% in 2030. “We find ourselves in a new playing field — up against companies that are entering the mobility market from the world of technology,” Diess said on Tuesday. “Stock market players still regard the Volkswagen Group as part of the ‘old auto’ world. By focusing consistently on software and efficiency, we are working to change this view.” Source link Orbem News #Analysis #Business #Crown #Steal #Tesla:Volkswagencouldsoonstealtheelectriccarcrown-CNN #Teslas #Volkswagen
0 notes
Text
A Convolutional Neural Network with K Nearest Neighbor for Image Classification
Image classification forms the basis for computer vision which is a trending sub-field in Machine Learning. The Convolutional Neural Network (ConvNet) has recently achieved great success in many computer vision tasks. The common architecture of ConvNets contains many layers to recurrently extract suitable image features and feed them to the softmax function for classification which often displays low prediction performance. In this paper, we propose the use of K-Nearest Neighbor as classifier for the ConvNets and also introduce the use of Principal Component Analysis (PCA) for dimensionality reduction. When successfully implemented, the proposed system should be able to accurately classify images.
The Convolutional Neural Network (ConvNet) has recently achieved great success in many computer vision tasks. ConvNet was partially inspired by neuroscience and thus shares many of the brain’s properties. Training a ConvNet can be achieved by back-propagating the classification error, which requires a reasonable amount of training data based on the size of the network.
Image classification can be defined as the task of categorizing images into one of several predefined classes, is a fundamental problem in computer vision. Image classification forms the basis for other computer vision tasks such as localization, detection and segmentation. Image classification is an important task in the field of machine learning and image processing, which is widely used in many fields, such as computer vision, network image retrieval and military automation target identification.
ConvNets, as standard feature extractors, have been continuously used to improve computer vision in terms of accuracy. This implies expulsion of the traditional hand-crafted feature extraction techniques in computer vision problems. The features learned from ConvNets are generated using a general-purpose learning procedure. Combining both hand-crafted features and machine learned feature is increasingly becoming a hot spot.
The common architecture of ConvNets contains many layers to recurrently extract suitable image features and feed them to the softmax function (also known as multinomial logistic regression) for classification and replaced softmax with Biometric Pattern Recognition (BPR) and Support Vector Machine (SVM) respectively in order to overcome the limitation of softmax classifier which, often displays a low prediction performance.
At every stage of unsupervised learning K Nearest Neighbor (KNN) can perform better than the SVM. Principal Component Analysis (PCA) can also be used to reduce the dimension of the convoluted image. This work is aimed at proposing an improved Convolutional Neural Network (ConvNet) with reduced complexity and improves precision that can be easily trained and can adapt to different data and tasks for image classification.
To achieve this, KNN is going to be adopted as the classifier while PCA for image dimension reduction will is adopted at the fully connected layer before input into the KNN for classification as this is to reduce the complexity.
Principal Component Analysis (PCA) is one of the statistical techniques frequently used in signal processing, data dimension reduction or data decorrelation. PCA is often used in signal and image processing as it offers a powerful means for data analysis and pattern recognition which are used as a technique for data compression, dimension reduction or decorrelation. PCA represent data in a form that increases the mutual independence of influential components by means of Eigen-analysis.
KNN is a simple algorithm that can store all available cases and classifies new cases based on a similarity measure. K-Nearest Neighbor (KNN) algorithm is one of the distinctive methods used in image classification. Nearest Neighbor classification of objects is based on their similarity to the training sets and the statistics defined. KNN’s basic idea is that if the majority of the k nearest samples of an image in the feature space belong to a certain category, the image also belongs to this category. KNN consists of two main procedures: similarity computing and k nearest samples searching. Since KNN requires no learning and training phases and avoids overfitting of parameters, it has a good accuracy in dealing with classification tasks with more samples and less classes. The k nearest neighbor (KNN) classifier is based on the Euclidean distance between a test sample and the specified training samples.
When compared, the performance of unsupervised feature learning and transfer learning against simple patch initialization and random weight initialization within the same setup. They showed that pre-training helps to train CNNs from few samples and that the correct choice of the initialization scheme can push the network’s performance by up to 41% compared to random initialization. Their results show that pre-training systematically improves generalization capabilities when handling datasets with few samples. They concluded that the choice of a pre-training method depend highly on the dataset used. They used the traditional filter selection and softmax as their classifier.
On being evaluated and compared the performance of the support vector machine (SVM) and KNN classifiers is measured and the performances of the classifiers by using the confusion matrix technique. It was found that the KNN classifier was better than the SVM classifier for the discrimination of pulmonary acoustic signals.
A new class was derived, of fast algorithms for convolutional neural networks using Winograd's minimal filtering algorithms. The algorithms were derived for network layers with 3x3 as filter size for image recognition tasks. The algorithms reduced arithmetic complexity up to 4 times compared with direct convolution, while using small block sizes with limited transform overhead and high computational intensity. Work was basically concentrated on the convolution layer and did not consider other layers of the ConvNet.
The performance of different classifiers on the CIFAR-10 dataset, were studied and an ensemble of classifiers was build to reach a better performance. It was show that CIFAR-10, KNN and Convolutional Neural Network (CNN), on some classes, are mutually exclusive, and as such produced higher accuracy when combined. The concept of Principal Component Analysis (PCA) to reduce overfitting in KNN was applied, and then combined it with a CNN to increase its accuracy. The work combined the KNN with CNN for feature extraction which is different.
On investigated, a series of data augmentation techniques to progressively improve the prediction invariance of image scaling and rotation was done. SVM classifier as an alternative to softmax was used to enhance generalization ability. The recognition rate was up to 92.74% on the patch level with data augmentation and classifier boosting. The results showed that, the combined CNN-SVM model beats models of traditional features with SVM as well as the original CNN with softmax.
To deal with the problem associated with softmax classifier, proposed a new technique of combining Biometric Pattern Recognition (BPR) with ConvNets for image classification. BPR performs class recognition by a union of geometrical cover sets in a high-dimensional feature space and therefore can overcome some disadvantages of traditional pattern recognition. They evaluated the method using three image datasets: MNIST, AR, and CIFAR10. We are applying their concept but using KNN instead of the BPR.
Architecture was presented which combines a Convolutional Neural Network (CNN) and a linear SVM for image classification. They employed the use of a simple 2-Convolutional Layer with max-pooling. This was tested, architecture using Fashion-MNIST datasets and found that the CNN-softmax outperformed CNN-SVM. Hence, confess that there was no enough preprocessing of the data in the study and need to improve on the model to achieve more accurate results,
It was proposed that a Presentation Attack Detection (PAD) method called Spoof Detection for near-infrared (NIR) camera-based finger-vein recognition system using Convolutional Neural Network (CNN) to enhance the detection ability of previous handcrafted methods. This led to derive a suitable feature extractor for the PAD using ConvNet. This processed the extracted image features in order to enhance the presentation attack finger-vein image detection ability of the CNN method using Principal Component Analysis Method (PCA) for dimensionality reduction of feature space and Support Vector Machine (SVM) for classification. Through extensive experimental results, it was endorsed that this proposed method is suitable for presentation of attack finger-vein image detection and it can deliver superior detection results when compared with other ConvNets methods.
The use of fully convolutional architectures in the notable object detection systems such as Fast/Faster RCNN to replace the fully connected layer of ConvNets was presented. A general formula was derived, specifically to accurately design the input size of the various fully convolutional networks in which the convolutional layer and pooling layer are concatenated with their strides and have proposed an efficient architecture of skip connection to accelerate the training process. This compared the model with Fast RCNN and the accuracy increased by about 2%. The method was tested using a very small data set. Again, using CNN as a classifier might not be feasible because fully connected layer is able to generalize the feature extracted into the output space and also the output need to be scaler.
During the work, a k-Tree method to learn different optimal k values for different test and new samples, by involving a training stage in the kNN classification was proposed. In the training stage, k-Tree method first learns optimal k values for all training samples by a new sparse reconstruction model, and then construct a decision tree using training samples and the learned optimal k values. In the test stage, the k-Tree obtained as output the optimal k value for each tested sample, before the kNN classification was carried out using the learned optimal k value and all training samples. The model had similar running cost but higher classification accuracy, compared with traditional kNN methods but less running cost. It was also realized that similar classification accuracy, compared with the new kNN methods, which assign different k values to different test samples is possible.
Confusion Matrix (explained below)
Positive(P): Observation is positive
Negative (N): Observation not positive
True Positive (TP): Observation is positive and is predicted positive
True Negative (TN): Observation is negative and is predicted negative
False Positive (FP): observation is negative but predicted positive
False Negative (FN): Observation is positive but predicted negative
We tend to design an improved Convolutional Neural Networks (ConvNets) with improved precision and accuracy. We employ the use of Principal Component Analysis (PCA) to reduce the dimension of the image and K Nearest Neighbor (KNN) for classification. When successfully implemented, the proposed system should be able to accurately classify images based on a given training set and test set. It will be evaluated in terms of accuracy.
#Image classification Convolutional Neural Networks Principal Component Analysis K-Nearest Neighbor.
0 notes
Photo

Visit us: http://www.connectinfosoft.com/contact_us.php
Our Services: http://www.connectinfosoft.com/service.php
Connect Infosoft Technologies, as a best Machine Learning development company, Machine learning is an application of artificial intelligence (AI) that enables a machine to learn from data rather than through explicit programming systems.
Machine learning, mainly focus on the development of computer programs that can observe or access data and use it to learn for themselves. Its primary aim is to allow the machine to learn automatically without any human intervention or assistance and adjust actions accordingly. Machine learning look’s for patterns in algorithm data and makes better decisions in the future based. It learns from experience. However, machine learning is not a simple process.
SOME MACHINE LEARNING METHODS:
Supervised Machine learning, as the name indicates the presence of supervisor. It is used in the cases where the target set is known. In this machine is trained by input data and produces a predicted outcome from labeled data. Which we can later verify using cross validation between the targets set and predicted outcome to check for our model accuracy.
Supervised learning models includes below algorithms:
Regression
o Linear
o Logistic
Classification.
Unsupervised Machine Learning, algorithms are used to train a machine when the target set is unknown or not labeled which leads algorithm to act on that information without guidance. Under the unsupervised machine learning the task of machine is to group unsorted information according to similarities, patterns and differences without any prior training of data. Thus the machine is restricted to find the hidden structure in unlabeled data by its own. Unsupervised learning models includes below algorithms:
Clustering
Association.
Semi-supervised Machine Learning, algorithms fall between supervised and unsupervised learning, since it is a combination of both labeled and unlabeled data for training. Typically a small amount of labeled data and a large amount of unlabeled data is available. This method is used on those systems which are considered for improving learning accuracy.
Reinforcement Machine Learning, is a part of Machine Learning. Reinforcement is about making our model learn by taking suitable action which provides it maximum reward in a particular situation. It is done by various software and machines to find the best possible behavior or path then it should take in a specific situation with maximum reward. Combining machine learning with cognitive technologies and AI makes it even more effective in processing large amount of information. Reinforcement machine learning is further classified into CNN(Convolutional Neural Network), ANN(Artificial Neural Network), RNN(Recurrent Neural Network), Q-Learning, DRQN, DDPG etc..
ADVANTAGES OF MACHINE LEARNING:
Vastly Used In Variety Of Applications Like Banking & Financial Sector, Healthcare, Retail, Publishing & Social Media, Robot Locomotion Etc.
Google And Facebook Uses It To Push Relevant Advertisements Based On Users Past Searched Queries Behavior.
Capable To Handle Multi-Dimensional And Multi-Variety Of Data In Dynamic Or Uncertain Environments.
Requires Less Time And Efficient Utilization Of Resources.
Tools In Machine Learning Provide Continuous Quality Improvements In Large And Complex Process Environments.
Source Programs Such As Rapidminer Help To Increase Usability Of Algorithms For Various Applications.
SOME MACHINE LEARNING TOOLS:
R: R is open-source programming languages with a large number of communities; it is mainly used for statistical analysis and analytical work. R has number of tools to communicate the results. R programming language is one the right tool for data science because of its powerful communication libraries. R is extensively used in the field of data science and machine learning from a very long time. Python: Python is an object-oriented, high level programming language for making web & app development and complex applications. It offers dynamic typing and dynamic binding options for applications and also supports modules and packages. Python programming is widely used in Artificial Intelligence (AI), Machine Learning (ML), Natural Language Generation, Neural Networks and other advanced. Python had deep focus on code readability. SAS: R and SAS is another great combination for programming languages. SAS is an integrated software suite for advanced analytics, used for statistical analysis, business intelligence, data management and predictive analytics. SAS software can be used for both ways- graphical interface and programming language. It can read in data/instruction from common spreadsheets and databases and results the output of statistical analyses in tables, graphs and as RTF, HTML, PDF. The SAS runs under compilers that can be used on Microsoft Windows, Linux and mainframe computers. SAS language consists of two compilers as SAS System and World Programming System (WPS). GPU Architecture: GPU computing is the process of using GPU (graphics processing unit) as a co-processor to accelerate CPUs for general purpose, scientific and engineering computing. The GPU accelerates the running applications on CPU by offloading some of the compute-intensive and time consuming portions of the code. The rest of the application still runs on CPU. Technically application runs faster because it is using the massively parallel processing power of the GPU to boost performance. This process is known as heterogeneous or hybrid computing architecture. Check Out Are Our More Services.
Want to learn what we can do for you?
Let's talk
Contact us:
Visit us: http://www.connectinfosoft.com/contact_us.php
Our Services: http://www.connectinfosoft.com/service.php
Email: [email protected]
Phone: (225) 361-2741
Connect InfoSoft Technologies Pvt.Ltd
0 notes
Text
HyperTransformer: A Example of a Self-Attention Mechanism For Supervised Learning
Subscribe .t9ce7d96b-e3c9-448d-b1fd-97f643ade4ab { color: #fff; background: #222; border: 1px solid transparent; border-radius: undefinedpx; padding: 8px 21px; } .t9ce7d96b-e3c9-448d-b1fd-97f643ade4ab.place-top { margin-top: -10px; } .t9ce7d96b-e3c9-448d-b1fd-97f643ade4ab.place-top::before { content: “”; background-color: inherit; position: absolute; z-index: 2; width: 20px; height: 12px; }…
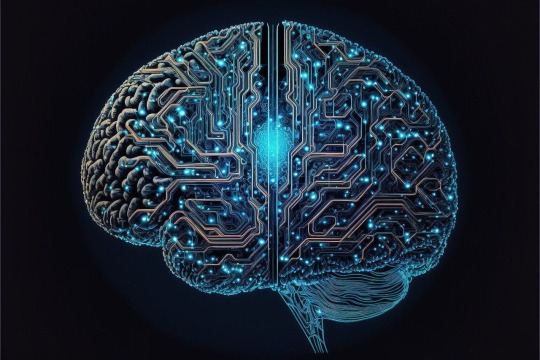
View On WordPress
#conventional-machine-learning#convolutional-neural-network#few-shot-learning#hypertransformer#parametric-model#small-target-cnn-architectures#supervised-model-generation#task-independent-embedding
0 notes
Photo
"[D] Teacher-Student training situation with CNN-FC"- Detail: I've been asked to convert a fully-trained CNN to a simple FC network with fixed architecture (it'll be used on a small chip if I remember correctly). They understand the classification performance will drop but it needs to be done anyway. I've set up the student network such that it just takes the flattened image as the input but I'm unsure what my targets are. I have the data the teacher network was trained on so I guess I can train the student using those inputs with the correspoding teacher output (rather than one-hot targets in the dataset). But my real question is can I just generate random input images and use whatever the teacher outputs as a target for the student to train on? Is that what is usually done to generate a lot of training data for the student network?. Caption by Lewba. Posted By: www.eurekaking.com
0 notes
Text
Winner Spotlight: “Highway Gallery” by Louvre Abu Dhabi & TBWA\RAAD
May 16, 2019
2018 MENA Effie Awards
GOLD – Media Innovation – Existing Channel SILVER – Travel, Tourism, and Transportation
Louvre Abu Dhabi opened in 2017 as the first universal museum in the Middle East, with a world-class collection of archaeological treasures and fine art spanning thousands of years. At launch, the museum welcomed crowds to a series of sold-out events - but just a couple of months post-celebration, visitor volume stalled.
Together, Louvre Abu Dhabi and agency partner TBWA\RAAD needed to attract locals to the museum – and the solution would need to counteract the UAE’s lagging enthusiasm for museums in general, and lack of awareness about the Louvre Abu Dhabi in particular.
Enter “Highway Gallery,” a series of masterpieces from Louvre Abu Dhabi displayed along the most highly-trafficked road in the UAE. The project integrated OOH and radio, with interpretations of each piece broadcast through the speakers of each passing car.
After successfully changing attitudes and attracting visitors, “Highway Gallery” took home a Gold and Silver Effie in the 2018 MENA Effie Awards competition.
Below, Remie Abdo, Head of Planning at TBWA\RAAD, shares insight into how she and her team got people sampling the museum and excited about the Louvre Abu Dhabi. Read on to hear how the team challenged the definition of innovation and found inspiration from unlikely sources.
What were your objectives for “Highway Gallery”?
RA: Louvre Abu Dhabi opened its doors in November 2017. As the first universal museum in the region, and with unprecedented architecture and innovative exhibitions, it ticks the ‘first’ and ‘ests’ checklist of the country’s superlatives. Add to that a string of opening events a 360 campaign, concerts and performances, global and regional celebrity visitors, a 3D laser mapping light show, and several ribbon-cutting events… and you won’t be surprised to know that opening month, tickets sold out.
However, the reality wasn’t that sweet.
Two months down the lane, once the opening hype faded, UAE residents were not that interested in visiting anymore. Fear of the ‘Eiffel Tower Syndrome’ — becoming a touristic landmark that locals don’t visit — became the new worrying reality.
The objective was as simple, and complex, as getting UAE residents to the doors of the museum.
What was the strategic insight that drove the campaign?
RA: To solve the problem at hand, we dug for the problem behind the problem. We asked, why weren’t UAE residents interested in Louvre Abu Dhabi beyond the opening ceremonies? One would have thought they’d be excited to have the Louvre in their capital city.
The UAE population consists of two major groups, the Emiratis (15% of the population) and the expats (85%). We investigated each separately.
We discovered that Emiratis believed museums ‘are not for them.’ They found museums boring and archaic, and they are more into other forms of entertainment. Their interest in Louvre Abu Dhabi was limited to their interest of having the ‘Louvre’ in their country – another prestigious milestone.
Expats were skeptical, likely to agree with the sentiments: ‘a Louvre without the Mona Lisa is not the Louvre’, ‘this will be a replica of Louvre Paris’, ‘this won’t be like the Louvre’. They were quick to compare Louvre Abu Dhabi to the Louvre in Paris, and were not interested in a doppelgänger.
Their pre-judgement wasn’t founded. Emiratis didn't know what museums were exactly, as they had never had any locally – and when they traveled, museums weren’t on their bucket lists. And expats didn’t know what Louvre Abu Dhabi could offer - and how could they love something they didn’t know?
The insight was clear: UAE residents were not into ‘Louvre Abu Dhabi’ museum, not because they didn’t love it, but because they didn’t know it.
What was your big idea? How did you bring the idea to life?
RA: Alex Likerman, author of The Undefeated Mind, said ‘Trying something new opens up the possibility for you to enjoy something new. Entire careers, entire life paths, are carved out by people dipping their baby toes into small ponds and suddenly discovering a love for something they had no idea would capture their imaginations.’
Aligned with this thinking and our insight, Louvre Abu Dhabi needed to give residents a taste of the museum in order to capture their minds and drive them to visit. In the FMCG world, the solution would have been a no-brainer: distribute free samples of the product. Borrowing from retail best practices, the strategy boiled down to one question: How do we give a sample of the museum?
We introduced The Highway Gallery: A first-ever roadside exhibition featuring 10 of Louvre Abu Dhabi’s most magnificent masterpieces on giant, can’t-miss, 9x6 meter (approx. 30x20 foot) vertical frames. Among the works featured were Leonardo da Vinci’s La Belle Ferronnière (1490), Vincent van Gogh’s Self Portrait (1887), and Gilbert Stuart’s Portrait of George Washington (1822). The frames were placed as billboards along over 100km (approx. 62 miles) of the E11 Sheikh Zayed Road, the busiest highway in the UAE with an average of 12,000 cars commuting daily and the road that leads to Louvre Abu Dhabi.
But neither the size of the exhibition nor the choice of the artworks was a rich enough sample of the museum. Louvre Abu Dhabi needed to give a sneak peek into the artworks with their corresponding stories, beyond the aesthetics. Without context, the artworks lose their value.
Hence we used old ‘FM transmitter’ technology to hijack the frequencies of the most-listened-to radio stations on the highway. The FM devices synchronized and instantaneously broadcasted the story behind each art piece through the radios of cars passing by the frames. This was the world’s first audio-visual experience of this kind.
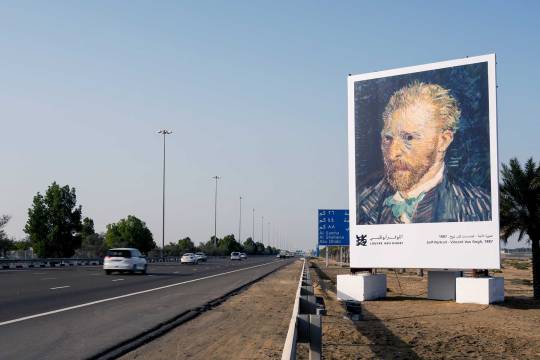
Example: When a car passed by the frame featuring Vincent Van Gogh’s Self Portrait (photo above), the passengers could hear on their radio speakers: “Say hello to Vincent Van Gogh, one of the greatest artists of the 19th century and the grandfather of modern art. He painted this Self Portrait in 1887, just three years before his death at 37. The impassioned brushstrokes reflect more than his artistic style, they reveal Vincent at his happiest and most-inspired. See them up close in our museum gallery Questioning A Modern World”.
What was the greatest challenge you faced when creating this campaign, and how did you approach that challenge?
RA: There were many challenges, but two notable ones.
The first, and easier to tackle, challenge was technical. We were innovating with an old medium, and when you’re the first to try something, it often doesn’t work quite right the first time. Until the very first day of the exhibition, we were still fixing bugs here and there. In such situations, disappointment settles in at some point, and you feel judged -- especially by those who told you ‘you can’t do it’… but the key in such situations is to use this frustration as a motive.
The second challenge was a bit bigger than us. Museums, in general, don’t appreciate creating replica of their artworks, and definitely not using these replicas as giant OOH media. We had to do a lot of selling to the client and go through multiple layers of approvals that got progressively more difficult.
How did you measure the effectiveness of the effort?
RA: The objective was to get UAE residents to the door of Louvre Abu Dhabi in the absence of all opening ceremonies. And we did just that. By the end of the Highway Gallery Exhibition, the declining numbers of visitors was a thing of the past, as the museum exceeded its monthly target x1.6 times. This time people were going to appreciate the artworks, ultimately achieving the museum’s main objective of footfall for the art.
Of course, we got some freebies along the way: Louvre Abu Dhabi followers on social media grew 4.2%; the online negative sentiment around the museum was reduced to only 1% and the positive sentiment grew 9%; Louvre Abu Dhabi brand recall registered a 14% uplift (regional average = 7%).
The Highway Gallery also got free local, regional and global coverage with CNN calling the gallery the “first of its kind in the world,” Lonely Planet stating that “Abu Dhabi became a lot more interesting,” The National regarding it as a “Highway to Heaven,” etc.
The museum became part of the conversations about Abu Dhabi through the press, but even more so through the people themselves. After stagnant Louvre Abu Dhabi online mentions during the previous months, the Highway Gallery garnered a 1,180% increase in mentions.
What are the most important learnings about marketing effectiveness that readers should take away from this case?
RA: Shifting perspective, as a means for innovation
‘Traditional media’ is a repelled expression in today’s world. Say “billboard” or “radio” twice and you will be labelled as the ‘traditional’ ‘non-digital’ ad person stuck in the old ways of doing things. With innovation, Louvre Abu Dhabi gave two traditional media a well-needed resuscitation, turning them into the most innovative and modern media combination of today.
The advertising industry witnesses changes by the minute – media channels deemed obsolete, processes reckoned too old. We naturally tend to discard the old and jump on the new to be perceived as innovative. However, this case proves that a new perspective on the old can create even more innovative solutions.
Good artists copy, great artists steal
It is unthought-of for a refined art industry to plagiarize from a mass FMCG practice. Drawing the parallel between an experience-based industry and a commodity-driven industry allowed the museum to find an unprecedented solution to its problem. Who said we can’t sample a museum?
In advertising, looking into adjacent industries is considered common practice. To create truly disruptive solutions however, looking into far-fetched industries to extract best practices can broaden our thinking, and ultimately make all the difference for the industry we are in.
Were there any unexpected long-term effects of this campaign?
RA: Last month, we launched the Tolerance Gallery, a sort of “Highway Gallery version 2” in support of the UAE’s ‘Year of Tolerance 2019.’ We placed sacred artworks representing different religions, from the Louvre Abu Dhabi’s collection, along the same Highway. This innovation is also set to be adopted soon by the Abu Dhabi government to alert drivers in cases of extreme fog to avoid road accidents. Several additional usages are being considered by different industries.
Remie Abdo is Head of Strategic Planning at TBWA\RAAD.
Remie would like to live in a world where purpose is our bread and butter, insights are our currency, storytelling is our language, common sense is more common, and free time is free.
An advocate of purpose, she tries to add sense to everything she does. On a personal level, she tailors her own clothes; grows her own vegetables and fruits, swaps consumerism with cultural-consumerism; obsesses about problem solving; and enjoys sharing ideas.
The same applies to her career. She is a firm believer that advertising is not an industry but a mean to a higher end; that of finding true solutions to real problems, influencing mindsets and shaping cultures for the better.
Her ethos: “If I am leaving my kid behind to work extra hours, I’d rather make it worthwhile”, continues to bear fruit in the shape of Cannes Lions, WARC, Effies, Dubai Lynx, Loeries, London International Awards, as well as judging global awards shows.
Remie started her career at Orange Telecom, BNP Paribas and the French Football Federation in Paris. After her Parisian adventure, she entered the agency world in Dubai making her way up from junior planner to Head of Planning at TBWA\RAAD Dubai today.
Read more Winner Spotlight interviews >
#Winner Spotlight#MENA Effies#MENA Effie Awards#TBWA#Louvre Abu Dhabi#TBWARAAD#Marketing Effectiveness Playbook
0 notes
Text
New deep learning model brings image segmentation to edge devices
A new neural network architecture designed by artificial intelligence researchers at DarwinAI and the University of Waterloo will make it possible to perform image segmentation on computing devices with low-power and -compute capacity.
Segmentation is the process of determining the boundaries and areas of objects in images. We humans perform segmentation without conscious effort, but it remains a key challenge for machine learning systems. It is vital to the functionality of mobile robots, self-driving cars, and other artificial intelligence systems that must interact and navigate the real world.
Until recently, segmentation required large, compute-intensive neural networks. This made it difficult to run these deep learning models without a connection to cloud servers.
In their latest work, the scientists at DarwinAI and the University of Waterloo have managed to create a neural network that provides near-optimal segmentation and is small enough to fit on resource-constrained devices. Called AttendSeg, the neural network is detailed in a paper that has been accepted at this year’s Conference on Computer Vision and Pattern Recognition (CVPR).
Object classification, detection, and segmentation
One of the key reasons for the growing interest in machine learning systems is the problems they can solve in computer vision. Some of the most common applications of machine learning in computer vision include image classification, object detection, and segmentation.
Image classification determines whether a certain type of object is present in an image or not. Object detection takes image classification one step further and provides the bounding box where detected objects are located.
Segmentation comes in two flavors: semantic segmentation and instance segmentation. Semantic segmentation specifies the object class of each pixel in an input image. Instance segmentation separates individual instances of each type of object. For practical purposes, the output of segmentation networks is usually presented by coloring pixels. Segmentation is by far the most complicated type of classification task.
The complexity of convolutional neural networks (CNN), the deep learning architecture commonly used in computer vision tasks, is usually measured in the number of parameters they have. The more parameters a neural network has the larger memory and computational power it will require.
RefineNet, a popular semantic segmentation neural network, contains more than 85 million parameters. At 4 bytes per parameter, it means that an application using RefineNet requires at least 340 megabytes of memory just to run the neural network. And given that the performance of neural networks is largely dependent on hardware that can perform fast matrix multiplications, it means that the model must be loaded on the graphics card or some other parallel computing unit, where memory is more scarce than the computer’s RAM.
Machine learning for edge devices
Due to their hardware requirements, most applications of image segmentation need an internet connection to send images to a cloud server that can run large deep learning models. The cloud connection can pose additional limits to where image segmentation can be used. For instance, if a drone or robot will be operating in environments where there’s no internet connection, then performing image segmentation will become a challenging task. In other domains, AI agents will be working in sensitive environments and sending images to the cloud will be subject to privacy and security constraints. The lag caused by the roundtrip to the cloud can be prohibitive in applications that require real-time response from the machine learning models. And it is worth noting that network hardware itself consumes a lot of power, and sending a constant stream of images to the cloud can be taxing for battery-powered devices.
For all these reasons (and a few more), edge AI and tiny machine learning (TinyML) have become hot areas of interest and research both in academia and in the applied AI sector. The goal of TinyML is to create machine learning models that can run on memory- and power-constrained devices without the need for a connection to the cloud.
With AttendSeg, the researchers at DarwinAI and the University of Waterloo tried to address the challenges of on-device semantic segmentation.
“The idea for AttendSeg was driven by both our desire to advance the field of TinyML and market needs that we have seen as DarwinAI,” Alexander Wong, co-founder at DarwinAI and Associate Professor at the University of Waterloo, told TechTalks. “There are numerous industrial applications for highly efficient edge-ready segmentation approaches, and that’s the kind of feedback along with market needs that I see that drives such research.”
The paper describes AttendSeg as “a low-precision, highly compact deep semantic segmentation network tailored for TinyML applications.”
The AttendSeg deep learning model performs semantic segmentation at an accuracy that is almost on-par with RefineNet while cutting down the number of parameters to 1.19 million. Interestingly, the researchers also found that lowering the precision of the parameters from 32 bits (4 bytes) to 8 bits (1 byte) did not result in a significant performance penalty while enabling them to shrink the memory footprint of AttendSeg by a factor of four. The model requires little above one megabyte of memory, which is small enough to fit on most edge devices.
“[8-bit parameters] do not pose a limit in terms of generalizability of the network based on our experiments, and illustrate that low precision representation can be quite beneficial in such cases (you only have to use as much precision as needed),” Wong said.
Attention condensers for computer vision
AttendSeg leverages “attention condensers” to reduce model size without compromising performance. Self-attention mechanisms are a series that improve the efficiency of neural networks by focusing on information that matters. Self-attention techniques have been a boon to the field of natural language processing. They have been a defining factor in the success of deep learning architectures such as Transformers. While previous architectures such as recurrent neural networks had a limited capacity on long sequences of data, Transformers used self-attention mechanisms to expand their range. Deep learning models such as GPT-3 leverage Transformers and self-attention to churn out long strings of text that (at least superficially) maintain coherence over long spans.
AI researchers have also leveraged attention mechanisms to improve the performance of convolutional neural networks. Last year, Wong and his colleagues introduced attention condensers as a very resource-efficient attention mechanism and applied them to image classifier machine learning models.
“[Attention condensers] allow for very compact deep neural network architectures that can still achieve high performance, making them very well suited for edge/TinyML applications,” Wong said.
Machine-driven design of neural networks
One of the key challenges of designing TinyML neural networks is finding the best performing architecture while also adhering to the computational budget of the target device.
To address this challenge, the researchers used “generative synthesis,” a machine learning technique that creates neural network architectures based on specified goals and constraints. Basically, instead of manually fiddling with all kinds of configurations and architectures, the researchers provide a problem space to the machine learning model and let it discover the best combination.
“The machine-driven design process leveraged here (Generative Synthesis) requires the human to provide an initial design prototype and human-specified desired operational requirements (e.g., size, accuracy, etc.) and the MD design process takes over in learning from it and generating the optimal architecture design tailored around the operational requirements and task and data at hand,” Wong said.
For their experiments, the researchers used machine-driven design to tune AttendSeg for Nvidia Jetson, hardware kits for robotics and edge AI applications. But AttendSeg is not limited to Jetson.
“Essentially, the AttendSeg neural network will run fast on most edge hardware compared to previously proposed networks in literature,” Wong said. “However, if you want to generate an AttendSeg that is even more tailored for a particular piece of hardware, the machine-driven design exploration approach can be used to create a new highly customized network for it.”
AttendSeg has obvious applications for autonomous drones, robots, and vehicles, where semantic segmentation is a key requirement for navigation. But on-device segmentation can have many more applications.
“This type of highly compact, highly efficient segmentation neural network can be used for a wide variety of things, ranging from manufacturing applications (e.g., parts inspection / quality assessment, robotic control) medical applications (e.g., cell analysis, tumor segmentation), satellite remote sensing applications (e.g., land cover segmentation), and mobile application (e.g., human segmentation for augmented reality),” Wong said.
0 notes
Text
If you did not already know
Expectation-Biasing State-of-the-art forecasting methods using Recurrent Neural Net- works (RNN) based on Long-Short Term Memory (LSTM) cells have shown exceptional performance targeting short-horizon forecasts, e.g given a set of predictor features, forecast a target value for the next few time steps in the future. However, in many applications, the performance of these methods decays as the forecasting horizon extends beyond these few time steps. This paper aims to explore the challenges of long-horizon forecasting using LSTM networks. Here, we illustrate the long-horizon forecasting problem in datasets from neuroscience and energy supply management. We then propose expectation-biasing, an approach motivated by the literature of Dynamic Belief Networks, as a solution to improve long-horizon forecasting using LSTMs. We propose two LSTM architectures along with two methods for expectation biasing that significantly outperforms standard practice. … Binary Weight and Hadamard-transformed Image Network (BWHIN) Deep learning has made significant improvements at many image processing tasks in recent years, such as image classification, object recognition and object detection. Convolutional neural networks (CNN), which is a popular deep learning architecture designed to process data in multiple array form, show great success to almost all detection \& recognition problems and computer vision tasks. However, the number of parameters in a CNN is too high such that the computers require more energy and larger memory size. In order to solve this problem, we propose a novel energy efficient model Binary Weight and Hadamard-transformed Image Network (BWHIN), which is a combination of Binary Weight Network (BWN) and Hadamard-transformed Image Network (HIN). It is observed that energy efficiency is achieved with a slight sacrifice at classification accuracy. Among all energy efficient networks, our novel ensemble model outperforms other energy efficient models. … Apache Pulsar Pulsar is a distributed pub-sub messaging platform with a very flexible messaging model and an intuitive client API. … TuckER Knowledge graphs are structured representations of real world facts. However, they typically contain only a small subset of all possible facts. Link prediction is a task of inferring missing facts based on existing ones. We propose TuckER, a relatively simple but powerful linear model based on Tucker decomposition of the binary tensor representation of knowledge graph triples. TuckER outperforms all previous state-of-the-art models across standard link prediction datasets. We prove that TuckER is a fully expressive model, deriving the bound on its entity and relation embedding dimensionality for full expressiveness which is several orders of magnitude smaller than the bound of previous state-of-the-art models ComplEx and SimplE. We further show that several previously introduced linear models can be viewed as special cases of TuckER. … https://bit.ly/3c1HP7R
0 notes
Text
Trump voter in immigration dilemma: We lied to our son – CNN Video
‘);$vidEndSlate.removeClass(‘video__end-slate–inactive’).addClass(‘video__end-slate–active’);}};CNN.autoPlayVideoExist = (CNN.autoPlayVideoExist === true) ? true : false;var configObj = {thumb: ‘none’,video: ‘politics/2019/05/29/family-separated-immigration-dilemma-kaye-dnt-ac360-vpx.cnn’,width: ‘100%’,height: ‘100%’,section: ‘domestic’,profile: ‘expansion’,network: ‘cnn’,markupId: ‘large-media_0’,adsection: ‘const-video-leaf’,frameWidth: ‘100%’,frameHeight: ‘100%’,posterImageOverride: {“mini”:{“width”:220,”type”:”jpg”,”uri”:”//cdn.cnn.com/cnnnext/dam/assets/190528210617-family-separated-immigration-dilemma-kaye-dnt-ac360-vpx-00000114-small-169.jpg”,”height”:124},”xsmall”:{“width”:307,”type”:”jpg”,”uri”:”//cdn.cnn.com/cnnnext/dam/assets/190528210617-family-separated-immigration-dilemma-kaye-dnt-ac360-vpx-00000114-medium-plus-169.jpg”,”height”:173},”small”:{“width”:460,”type”:”jpg”,”uri”:”//cdn.cnn.com/cnnnext/dam/assets/190528210617-family-separated-immigration-dilemma-kaye-dnt-ac360-vpx-00000114-large-169.jpg”,”height”:259},”medium”:{“width”:780,”type”:”jpg”,”uri”:”//cdn.cnn.com/cnnnext/dam/assets/190528210617-family-separated-immigration-dilemma-kaye-dnt-ac360-vpx-00000114-exlarge-169.jpg”,”height”:438},”large”:{“width”:1100,”type”:”jpg”,”uri”:”//cdn.cnn.com/cnnnext/dam/assets/190528210617-family-separated-immigration-dilemma-kaye-dnt-ac360-vpx-00000114-super-169.jpg”,”height”:619},”full16x9″:{“width”:1600,”type”:”jpg”,”uri”:”//cdn.cnn.com/cnnnext/dam/assets/190528210617-family-separated-immigration-dilemma-kaye-dnt-ac360-vpx-00000114-full-169.jpg”,”height”:900},”mini1x1″:{“width”:120,”type”:”jpg”,”uri”:”//cdn.cnn.com/cnnnext/dam/assets/190528210617-family-separated-immigration-dilemma-kaye-dnt-ac360-vpx-00000114-small-11.jpg”,”height”:120}}},autoStartVideo = false,isVideoReplayClicked = false,callbackObj,containerEl,currentVideoCollection = [{“descriptionPlainText”:”President Donald Trump insists there’s an immigration crisis in the United States, and while that may be true in some ways, it takes on a whole new meaning for other families. CNN’s Randi Kaye has the details.”,”imageUrl”:”//cdn.cnn.com/cnnnext/dam/assets/190528210617-family-separated-immigration-dilemma-kaye-dnt-ac360-vpx-00000114-large-169.jpg”,”title”:”Trump voter in immigration dilemma: We lied to our son”,”videoCMSUrl”:”/videos/politics/2019/05/29/family-separated-immigration-dilemma-kaye-dnt-ac360-vpx.cnn”,”videoLeafUrl”:”/videos/politics/2019/05/29/family-separated-immigration-dilemma-kaye-dnt-ac360-vpx.cnn”,”videoId”:”politics/2019/05/29/family-separated-immigration-dilemma-kaye-dnt-ac360-vpx.cnn”,”videoUrl”:”/videos/politics/2019/05/29/family-separated-immigration-dilemma-kaye-dnt-ac360-vpx.cnn”},{“descriptionPlainText”:”John Oliver revisited the Watergate scandal while tackling the prospect of impeachment on “Last Week Tonight with John Oliver.””,”imageUrl”:”//cdn.cnn.com/cnnnext/dam/assets/190617063613-john-oliver-watergate-impeachment-lnl-es-vpx-00004826-large-169.jpg”,”title”:”John Oliver takes on impeachment”,”videoCMSUrl”:”/video/data/3.0/video/media/2019/06/17/john-oliver-watergate-impeachment-lnl-es-vpx.cnn/index.xml”,”videoLeafUrl”:”/videos/media/2019/06/17/john-oliver-watergate-impeachment-lnl-es-vpx.cnn”,”videoId”:”media/2019/06/17/john-oliver-watergate-impeachment-lnl-es-vpx.cnn”,”videoUrl”:”/videos/media/2019/06/17/john-oliver-watergate-impeachment-lnl-es-vpx.cnn/video/playlists/stories-worth-watching/”},{“descriptionPlainText”:”On June 15, Target acknowledged guests were “unable to make purchases” at its stores, as shoppers complained on social that cash registers weren’t working.”,”imageUrl”:”//cdn.cnn.com/cnnnext/dam/assets/190615145101-01-target-systems-down-0615-large-169.jpg”,”title”:”Target customers waited for hours to check out after system outage”,”videoCMSUrl”:”/video/data/3.0/video/business/2019/06/15/target-outage-long-lines.cnn-business/index.xml”,”videoLeafUrl”:”/videos/business/2019/06/15/target-outage-long-lines.cnn-business”,”videoId”:”business/2019/06/15/target-outage-long-lines.cnn-business”,”videoUrl”:”/videos/business/2019/06/15/target-outage-long-lines.cnn-business/video/playlists/stories-worth-watching/”},{“descriptionPlainText”:”Late-night comedians aren’t looking the other way when it comes to Trump’s tweet in which he refers to Prince Charles as the “Prince of Whales.””,”imageUrl”:”//cdn.cnn.com/cnnnext/dam/assets/190614084605-late-night-laughs-trump-prince-of-whales-03-large-169.jpg”,”title”:”Trump’s tweet confuses an animal with a country”,”videoCMSUrl”:”/video/data/3.0/video/media/2019/06/14/late-night-laughs-trump-tweet-prince-of-whales-newday-vpx.cnn/index.xml”,”videoLeafUrl”:”/videos/media/2019/06/14/late-night-laughs-trump-tweet-prince-of-whales-newday-vpx.cnn”,”videoId”:”media/2019/06/14/late-night-laughs-trump-tweet-prince-of-whales-newday-vpx.cnn”,”videoUrl”:”/videos/media/2019/06/14/late-night-laughs-trump-tweet-prince-of-whales-newday-vpx.cnn/video/playlists/stories-worth-watching/”},{“descriptionPlainText”:”In India widespread droughts in recent years mean farmers often struggle to find enough water for their fields. Khethworks has developed a solar-powered irrigation system that doesn’t depend on seasonal rains or expensive fuel.”,”imageUrl”:”//cdn.cnn.com/cnnnext/dam/assets/190611150152-india-solar-irrigation-khethworks-02-restricted-large-169.jpg”,”title”:”Farmers are using the sun to help water their crops”,”videoCMSUrl”:”/video/data/3.0/video/business/2019/06/17/solar-irrigation-india-khethworks.cnn/index.xml”,”videoLeafUrl”:”/videos/business/2019/06/17/solar-irrigation-india-khethworks.cnn”,”videoId”:”business/2019/06/17/solar-irrigation-india-khethworks.cnn”,”videoUrl”:”/videos/business/2019/06/17/solar-irrigation-india-khethworks.cnn/video/playlists/stories-worth-watching/”},{“descriptionPlainText”:”CNN’s political analyst Brian Karem says that Sarah Sanders’ legacy will be one of obfuscation and divisiveness born out of the ending of the daily White House press briefings.”,”imageUrl”:”//cdn.cnn.com/cnnnext/dam/assets/190613195905-10-sarah-sanders-gallery-large-169.jpg”,”title”:”CNN analyst: Sarah Sanders’ legacy is divisiveness”,”videoCMSUrl”:”/video/data/3.0/video/media/2019/06/14/sarah-sanders-white-house-press-briefings-legacy-karem-cnn-today-sot.cnn/index.xml”,”videoLeafUrl”:”/videos/media/2019/06/14/sarah-sanders-white-house-press-briefings-legacy-karem-cnn-today-sot.cnn”,”videoId”:”media/2019/06/14/sarah-sanders-white-house-press-briefings-legacy-karem-cnn-today-sot.cnn”,”videoUrl”:”/videos/media/2019/06/14/sarah-sanders-white-house-press-briefings-legacy-karem-cnn-today-sot.cnn/video/playlists/stories-worth-watching/”},{“descriptionPlainText”:”Joe Crain, a local Illinois weatherman criticized his own news station’s “Code Red” weather alerts. Now he’s out of a job.”,”imageUrl”:”//cdn.cnn.com/cnnnext/dam/assets/190609114115-01-weatherman-code-red-alert-joe-crain-large-169.jpg”,”title”:”Meteorologist fired after criticizing his TV station on air”,”videoCMSUrl”:”/video/data/3.0/video/media/2019/06/10/weatherman-illinois-code-red-orig-bu.cnn/index.xml”,”videoLeafUrl”:”/videos/media/2019/06/10/weatherman-illinois-code-red-orig-bu.cnn”,”videoId”:”media/2019/06/10/weatherman-illinois-code-red-orig-bu.cnn”,”videoUrl”:”/videos/media/2019/06/10/weatherman-illinois-code-red-orig-bu.cnn/video/playlists/stories-worth-watching/”},{“descriptionPlainText”:”Airbus has annouced a 100 new orders and unveiled a new airliner at the Paris Air Show while Boeing has stalled while dealing with the grounding of their 737 Max jets. CNN’s Melissa Bell reports.”,”imageUrl”:”//cdn.cnn.com/cnnnext/dam/assets/190617123232-airbus-paris-air-show-june-17-2019-01-large-169.jpg”,”title”:”Airbus exploits Boeing’s 737 Max woes”,”videoCMSUrl”:”/video/data/3.0/video/business/2019/06/17/boeing-airbus-paris-air-show-bell-the-express.cnn/index.xml”,”videoLeafUrl”:”/videos/business/2019/06/17/boeing-airbus-paris-air-show-bell-the-express.cnn”,”videoId”:”business/2019/06/17/boeing-airbus-paris-air-show-bell-the-express.cnn”,”videoUrl”:”/videos/business/2019/06/17/boeing-airbus-paris-air-show-bell-the-express.cnn/video/playlists/stories-worth-watching/”},{“descriptionPlainText”:”Rays Power Infra is one of India’s largest solar power companies, with ambitious plans to harness the 300 days of sun that India receives each year. Co-founder and CEO Ketan Mehta describes the untapped opportunities for solar energy in the country.”,”imageUrl”:”//cdn.cnn.com/cnnnext/dam/assets/180928111730-rays-power-india-large-169.jpg”,”title”:”Harnessing India’s sunny days for power”,”videoCMSUrl”:”/video/data/3.0/video/business/2018/09/28/rays-power-india.cnn-business/index.xml”,”videoLeafUrl”:”/videos/business/2018/09/28/rays-power-india.cnn-business”,”videoId”:”business/2018/09/28/rays-power-india.cnn-business”,”videoUrl”:”/videos/business/2018/09/28/rays-power-india.cnn-business/video/playlists/stories-worth-watching/”},{“descriptionPlainText”:”A video of people abusing a robot — and later, the robot exacting revenge — went viral. Turns out, the whole video was faked.”,”imageUrl”:”//cdn.cnn.com/cnnnext/dam/assets/190617162549-04-robot-parody-grab-large-169.jpg”,”title”:”That viral video of a robot being beaten up isn’t real”,”videoCMSUrl”:”/video/data/3.0/video/business/2019/06/17/fake-robot-abuse-video-orig.cnn/index.xml”,”videoLeafUrl”:”/videos/business/2019/06/17/fake-robot-abuse-video-orig.cnn”,”videoId”:”business/2019/06/17/fake-robot-abuse-video-orig.cnn”,”videoUrl”:”/videos/business/2019/06/17/fake-robot-abuse-video-orig.cnn/video/playlists/stories-worth-watching/”},{“descriptionPlainText”:”John Stamos and his wife Caitlin McHugh gave Architectural Digest a tour of their Beverly Hills home, which they share with their 14-month-old son Billy.”,”imageUrl”:”//cdn.cnn.com/cnnnext/dam/assets/190613111806-john-stamos-home-tour-with-son-billy-large-169.jpg”,”title”:”John Stamos hosts tour of his ‘sturdy, funky, cool’ home”,”videoCMSUrl”:”/video/data/3.0/video/business/2019/06/13/john-stamos-house-tour-orig-vstop-bdk.cnn/index.xml”,”videoLeafUrl”:”/videos/business/2019/06/13/john-stamos-house-tour-orig-vstop-bdk.cnn”,”videoId”:”business/2019/06/13/john-stamos-house-tour-orig-vstop-bdk.cnn”,”videoUrl”:”/videos/business/2019/06/13/john-stamos-house-tour-orig-vstop-bdk.cnn/video/playlists/stories-worth-watching/”},{“descriptionPlainText”:”The new Bentley Flying Spur sedan looks like a limousine, but it can go over 200 miles per hour.”,”imageUrl”:”//cdn.cnn.com/cnnnext/dam/assets/190612142918-bentley-flying-spur-sedan-large-169.jpg”,”title”:”This Bentley could be the fastest sedan ever”,”videoCMSUrl”:”/video/data/3.0/video/business/2019/06/12/bentley-flying-spur-sedan-orig.cnn-business/index.xml”,”videoLeafUrl”:”/videos/business/2019/06/12/bentley-flying-spur-sedan-orig.cnn-business”,”videoId”:”business/2019/06/12/bentley-flying-spur-sedan-orig.cnn-business”,”videoUrl”:”/videos/business/2019/06/12/bentley-flying-spur-sedan-orig.cnn-business/video/playlists/stories-worth-watching/”},{“descriptionPlainText”:”In an exclusive interview at a data center in Oklahoma, Google CEO Sundar Pichai tells CNN’s Poppy Harlow why the company is investing in jobs around the country, and why tech may have to pump the brakes at times in order to best serve humanity.”,”imageUrl”:”//cdn.cnn.com/cnnnext/dam/assets/190613182415-04-google-oklahoma-large-169.jpg”,”title”:”Google CEO: We may have to slow down some ‘disruptive’ technology”,”videoCMSUrl”:”/video/data/3.0/video/business/2019/06/14/google-ceo-sundar-pichai-poppy-harlow-zw-orig.cnn/index.xml”,”videoLeafUrl”:”/videos/business/2019/06/14/google-ceo-sundar-pichai-poppy-harlow-zw-orig.cnn”,”videoId”:”business/2019/06/14/google-ceo-sundar-pichai-poppy-harlow-zw-orig.cnn”,”videoUrl”:”/videos/business/2019/06/14/google-ceo-sundar-pichai-poppy-harlow-zw-orig.cnn/video/playlists/stories-worth-watching/”},{“descriptionPlainText”:”Queen Elsa and Princess Anna are back in the trailer for “Frozen II,” which will likely mean more huge box office and merchandising returns for Disney.”,”imageUrl”:”//cdn.cnn.com/cnnnext/dam/assets/190213104122-02-frozen-2-large-169.jpg”,”title”:”Disney’s mega-hit is back with a new trailer”,”videoCMSUrl”:”/video/data/3.0/video/business/2019/02/13/frozen-ii-trailer-disney-business-orig.cnn-business/index.xml”,”videoLeafUrl”:”/videos/business/2019/02/13/frozen-ii-trailer-disney-business-orig.cnn-business”,”videoId”:”business/2019/02/13/frozen-ii-trailer-disney-business-orig.cnn-business”,”videoUrl”:”/videos/business/2019/02/13/frozen-ii-trailer-disney-business-orig.cnn-business/video/playlists/stories-worth-watching/”},{“descriptionPlainText”:”Kent International CEO Arnold Kamler tells CNN’s Clare Sebastian how trade war and tariffs have impacted his business and made things more difficult for him.”,”imageUrl”:”//cdn.cnn.com/cnnnext/dam/assets/190614105506-kent-ceo-arnold-kamler-large-169.jpg”,”title”:”CEO: Tariffs turn up pressure on my business”,”videoCMSUrl”:”/video/data/3.0/video/business/2019/06/14/tariffs-companies-warn-about-impact-sebastian-first-move-sot.cnn/index.xml”,”videoLeafUrl”:”/videos/business/2019/06/14/tariffs-companies-warn-about-impact-sebastian-first-move-sot.cnn”,”videoId”:”business/2019/06/14/tariffs-companies-warn-about-impact-sebastian-first-move-sot.cnn”,”videoUrl”:”/videos/business/2019/06/14/tariffs-companies-warn-about-impact-sebastian-first-move-sot.cnn/video/playlists/stories-worth-watching/”},{“descriptionPlainText”:”Kraft says its new dressing will encourage kids to eat their veggies. Of course, the dressing itself is far from healthy.”,”imageUrl”:”//cdn.cnn.com/cnnnext/dam/assets/190610160046-kraft-salad-frosting-large-169.jpg”,”title”:”Kraft wants to feed kids ‘Salad Frosting'”,”videoCMSUrl”:”/video/data/3.0/video/business/2019/06/11/kraft-ranch-salad-frosting.cnn-business/index.xml”,”videoLeafUrl”:”/videos/business/2019/06/11/kraft-ranch-salad-frosting.cnn-business”,”videoId”:”business/2019/06/11/kraft-ranch-salad-frosting.cnn-business”,”videoUrl”:”/videos/business/2019/06/11/kraft-ranch-salad-frosting.cnn-business/video/playlists/stories-worth-watching/”}],currentVideoCollectionId = ”,isLivePlayer = false,mediaMetadataCallbacks,mobilePinnedView = null,moveToNextTimeout,mutePlayerEnabled = false,nextVideoId = ”,nextVideoUrl = ”,turnOnFlashMessaging = false,videoPinner,videoEndSlateImpl;if (CNN.autoPlayVideoExist === false) {autoStartVideo = true;if (autoStartVideo === true) {if (turnOnFlashMessaging === true) {autoStartVideo = false;containerEl = jQuery(document.getElementById(configObj.markupId));CNN.VideoPlayer.showFlashSlate(containerEl);} else {CNN.autoPlayVideoExist = true;}}}configObj.autostart = CNN.Features.enableAutoplayBlock ? false : autoStartVideo;CNN.VideoPlayer.setPlayerProperties(configObj.markupId, autoStartVideo, isLivePlayer, isVideoReplayClicked, mutePlayerEnabled);CNN.VideoPlayer.setFirstVideoInCollection(currentVideoCollection, configObj.markupId);var embedLinkHandler = {},videoPinner,embedCodeCopy;function onVideoCarouselItemClicked(evt) {‘use strict’;var videoId,articleElem,videoPlayer,thumbImageElem,thumbImageLargeSource,overrides = {autostart: false,muteOverlayClicked: true,videoCollection: this.videoCollection},shouldStartVideo = false,playerInstance;try {articleElem = jQuery(evt.currentTarget).find(‘article’);thumbImageElem = jQuery(articleElem).find(‘.media__image’);videoId = articleElem.data().videoId;if (CNN.VideoPlayer.getLibraryName(configObj.markupId) === ‘fave’) {playerInstance = FAVE.player.getInstance(configObj.markupId);if (CNN.Utils.existsObject(playerInstance) &&typeof playerInstance.getVideoData === ‘function’ &&playerInstance.getVideoData().id !== videoId) {jQuery(articleElem).closest(‘.cn-carousel-medium-strip’).parent().find(‘script[name=”metaScript”]’).remove();playerInstance.play(videoId, overrides);}} else {videoPlayer = CNNVIDEOAPI.CNNVideoManager.getInstance().getPlayerByContainer(configObj.markupId);if (videoPlayer && videoPlayer.videoInstance) {if (!videoPlayer.videoInstance.cvp) {if (typeof thumbImageElem !== ‘undefined’ && thumbImageElem !== null) {thumbImageLargeSource = thumbImageElem.data() && thumbImageElem.data().srcLarge ? thumbImageElem.data().srcLarge : ‘none’;}overrides.thumb = thumbImageLargeSource ? thumbImageLargeSource : ‘none’;shouldStartVideo = true;}if (videoPlayer.videoInstance.config) {if (videoPlayer.videoInstance.config.video !== videoId) {jQuery(articleElem).closest(‘.cn-carousel-medium-strip’).parent().find(‘script[name=”metaScript”]’).remove();CNNVIDEOAPI.CNNVideoManager.getInstance().playVideo(configObj.markupId, videoId, overrides);}}}}} catch (error) {console.log(“error in initializing video player” + error);}}function setInitialVideoEmbed() {}function initialize(){var carousel = jQuery(document.getElementById(‘cn-current_video_collection’)).find(‘.js-owl-carousel’),owl;if (carousel) {carousel.find(‘.cn__column.carousel__content__item’).find(‘a’).removeAttr(‘href’);jQuery(carousel).on(‘click’, ‘.cn__column.carousel__content__item’, onVideoCarouselItemClicked);}}if (CNN.VideoPlayer.getLibraryName(configObj.markupId) === ‘videoLoader’) {window.CNNVideoAPILoadCompleteHandlers = window.CNNVideoAPILoadCompleteHandlers ? window.CNNVideoAPILoadCompleteHandlers : [];window.CNNVideoAPILoadCompleteHandlers.push(initialize);window.CNNVideoAPILoadCompleteHandlers.push(setInitialVideoEmbed);} else {initialize();}CNN.INJECTOR.executeFeature(‘videx’).done(function () {var initMeta = {id:”politics/2019/05/29/family-separated-immigration-dilemma-kaye-dnt-ac360-vpx.cnn”, isEmbeddable: “yes”};CNN.Videx.EmbedButton.updateCode(initMeta);}).fail(function () {throw ‘Unable to fetch the videx bundle.’;});function updateCurrentlyPlaying(videoId) {var videoCollectionId = ‘current_video_collection’,videocardContents = getCurrentVideoCardContents(videoId),carousel = jQuery(document.getElementById(‘cn-current_video_collection’)).find(‘.js-owl-carousel’),domain = CNN.Host.domain || (document.location.protocol + ‘//’ + document.location.hostname),owl,$owlFirstItem,$owlPrevItem,showDetailsSpanContent = ”,gigyaShareElement,showIndex,whatsappShareElement,$carouselContentItems = jQuery(‘.carousel__content__item’, document.getElementById(‘cn-current_video_collection’));gigyaShareElement = jQuery(‘div.js-gigya-sharebar’);if (typeof gigyaShareElement !== ‘undefined’ && CNN.Utils.existsObject(videocardContents)) {jQuery(gigyaShareElement).attr(‘data-title’, videocardContents.headlinePlainText || ”);jQuery(gigyaShareElement).attr(‘data-description’, videocardContents.descriptionPlainText || ”);jQuery(gigyaShareElement).attr(‘data-link’, domain + videocardContents.url || ”);jQuery(gigyaShareElement).attr(‘data-image-src’, (videocardContents.media && videocardContents.media.elementContents && videocardContents.media.elementContents.imageUrl) || ”);}whatsappShareElement = jQuery(‘div.share-bar-whatsapp-container’);if (typeof whatsappShareElement !== ‘undefined’) {jQuery(whatsappShareElement).attr(‘data-title’, videocardContents.headlinePlainText || ”);jQuery(whatsappShareElement).attr(‘data-storyurl’, domain + videocardContents.url || ”);}if (carousel && currentVideoCollectionContainsId(videoId)) {owl = carousel.data(‘owl.carousel’) || {};showIndex = getCurrentVideoIndex(videoId);if (typeof owl.to === ‘function’) {owl.to(showIndex);}$owlPrevItem = CNN.Utils.exists(owl.$element) ? owl.$element.find(‘.cd.cd–active’) : $carouselContentItems.find(‘.cd.cd–active’);$owlPrevItem.removeClass(‘cd–active’);$owlPrevItem.find(‘.media__over-text’).remove();$owlPrevItem.find(‘.media__icon’).show();$owlFirstItem = CNN.Utils.exists(owl._items) ? jQuery(owl._items[showIndex]) : $carouselContentItems.eq(showIndex);$owlFirstItem.find(‘.cd’).addClass(‘cd–active’);$owlFirstItem.find(‘.media a:first-child’).append(‘
Now Playing
‘);if (Modernizr && !Modernizr.phone) {$owlFirstItem.find(‘.media__icon’).hide();}}CNN.Videx.Metadata.init({dateCreated: videocardContents.dateCreated,descriptionText: videocardContents.descriptionText,duration: videocardContents.duration,sourceLink: videocardContents.sourceLink,sourceName: videocardContents.sourceName,title: videocardContents.headlineText},{videoCollectionDivId: ‘cn-qvz0x8’,videoDescriptionDivId: ‘js-video_description-qvz0x8’,videoDurationDivId: ‘js-video_duration-qvz0x8’,videoTitleDivId: ‘js-leaf-video_headline-qvz0x8’,videoSourceDivId: ‘js-video_sourceName-qvz0x8’});if (CNN.Utils.exists(videocardContents.showName)) {if (CNN.Utils.exists(videocardContents.showUrl)) {showDetailsSpanContent = ‘ ‘ + videocardContents.showName + ‘ | ‘;} else {showDetailsSpanContent = videocardContents.showName + ‘ | ‘;}}fastdom.measure(function getShowInfo() {var $show = jQuery(‘.metadata__show’),$isShowDetailsSpanExists = $show.find(‘span’).hasClass(‘metadata–show__name’),$showName = jQuery(‘.metadata–show__name’);fastdom.mutate(function updateShowInfo() {if (!$isShowDetailsSpanExists) {$show.prepend(‘ ‘ + showDetailsSpanContent + ‘‘);} else {$showName.html(showDetailsSpanContent);}});});if (typeof (history) !== ‘undefined’ && typeof (history.replaceState) !== ‘undefined’) {history.replaceState(”, ”, videocardContents.url);document.title = videocardContents.headlineText ? videocardContents.headlineText : ”;}}function getCurrentVideoCardContents(currentVideoId) {var containerContents = [{“branding”:””,”cardContents”:{“additionalSections”:[],”auxiliaryText”:””,”bannerText”:[],”bannerHasATag”:false,”bannerPosition”:””,”brandingLink”:””,”brandingImageUrl”:””,”brandingTextHead”:””,”brandingTextSub”:””,”cardSectionName”:””,”contentType”:””,”cta”:”share”,”descriptionText”:[“u003ca href=”https://cnn.it/2IO7dj6; target=”_blank”>President Donald Trump insists there’s an immigration crisis in the United Statesu003c/a>, and while that may be true in some ways, it takes on a whole new meaning for other families. CNN’s u003ca href=”https://cnn.it/2kuVaex; target=”_blank”>Randi Kayeu003c/a> has the details.”],”descriptionPlainText”:”President Donald Trump insists there’s an immigration crisis in the United States, and while that may be true in some ways, it takes on a whole new meaning for other families. CNN’s Randi Kaye has the details.”,”headlinePostText”:””,”headlinePreText”:””,”headlineText”:”Trump voter in immigration dilemma: We lied to our son”,”headlinePlainText”:”Trump voter in immigration dilemma: We lied to our son”,”iconImageUrl”:””,”iconType”:”video”,”isMobileBannerText”:false,”kickerText”:””,”maximizedBannerSize”:[],”media”:{“contentType”:”image”,”type”:”element”,”cutFormat”:”16:9″,”elementContents”:{“caption”:””,”imageAlt”:”family separated immigration dilemma kaye dnt ac360 vpx_00000114″,”imageUrl”:”//cdn.cnn.com/cnnnext/dam/assets/190528210617-family-separated-immigration-dilemma-kaye-dnt-ac360-vpx-00000114-large-169.jpg”,”label”:””,”galleryTitle”:””,”head”:””,”source”:”CNN”,”photographer”:””,”cuts”:{“mini”:{“width”:220,”type”:”jpg”,”uri”:”//cdn.cnn.com/cnnnext/dam/assets/190528210617-family-separated-immigration-dilemma-kaye-dnt-ac360-vpx-00000114-small-169.jpg”,”height”:124},”xsmall”:{“width”:307,”type”:”jpg”,”uri”:”//cdn.cnn.com/cnnnext/dam/assets/190528210617-family-separated-immigration-dilemma-kaye-dnt-ac360-vpx-00000114-medium-plus-169.jpg”,”height”:173},”small”:{“width”:460,”type”:”jpg”,”uri”:”//cdn.cnn.com/cnnnext/dam/assets/190528210617-family-separated-immigration-dilemma-kaye-dnt-ac360-vpx-00000114-large-169.jpg”,”height”:259},”medium”:{“width”:780,”type”:”jpg”,”uri”:”//cdn.cnn.com/cnnnext/dam/assets/190528210617-family-separated-immigration-dilemma-kaye-dnt-ac360-vpx-00000114-exlarge-169.jpg”,”height”:438},”large”:{“width”:1100,”type”:”jpg”,”uri”:”//cdn.cnn.com/cnnnext/dam/assets/190528210617-family-separated-immigration-dilemma-kaye-dnt-ac360-vpx-00000114-super-169.jpg”,”height”:619},”full16x9″:{“width”:1600,”type”:”jpg”,”uri”:”//cdn.cnn.com/cnnnext/dam/assets/190528210617-family-separated-immigration-dilemma-kaye-dnt-ac360-vpx-00000114-full-169.jpg”,”height”:900},”mini1x1″:{“width”:120,”type”:”jpg”,”uri”:”//cdn.cnn.com/cnnnext/dam/assets/190528210617-family-separated-immigration-dilemma-kaye-dnt-ac360-vpx-00000114-small-11.jpg”,”height”:120}},”responsiveImage”:true,”originalImageUrl”:”//cdn.cnn.com/cnnnext/dam/assets/190528210617-family-separated-immigration-dilemma-kaye-dnt-ac360-vpx-00000114.jpg”}},”noFollow”:false,”overMediaText”:””,”sectionUri”:””,”showSocialSharebar”:false,”shortUrl”:””,”statusText”:””,”statusColor”:””,”targetType”:””,”timestampDisplay”:””,”timestampUtc”:””,”lastModifiedText”:””,”lastModifiedState”:””,”type”:”card”,”url”:”/videos/politics/2019/05/29/family-separated-immigration-dilemma-kaye-dnt-ac360-vpx.cnn”,”width”:””,”webDisplayName”:”Politics”,”height”:””,”videoCMSUri”:”/videos/politics/2019/05/29/family-separated-immigration-dilemma-kaye-dnt-ac360-vpx.cnn”,”videoId”:”politics/2019/05/29/family-separated-immigration-dilemma-kaye-dnt-ac360-vpx.cnn”,”adSection”:”const-video-leaf”,”dateCreated”:”8:18 PM ET, Tue May 28, 2019″,”sourceName”:”CNN”,”sourceLink”:”http://www.cnn.com”,”showName”:”Anderson Cooper 360″,”showUrl”:”/shows/ac-360″},”contentType”:”video”,”maximizedBanner”:false,”type”:”card”,”autoStartVideo”:false},{“branding”:””,”cardContents”:{“additionalSections”:[“politics”],”auxiliaryText”:””,”bannerText”:[],”bannerHasATag”:false,”bannerPosition”:””,”brandingLink”:””,”brandingImageUrl”:””,”brandingTextHead”:””,”brandingTextSub”:””,”cardSectionName”:”media”,”contentType”:””,”cta”:”share”,”descriptionText”:[“John Oliver revisited the Watergate scandal while tackling the prospect of impeachment on “Last Week Tonight with John Oliver.””],”descriptionPlainText”:”John Oliver revisited the Watergate scandal while tackling the prospect of impeachment on “Last Week Tonight with John Oliver.””,”headlinePostText”:””,”headlinePreText”:””,”headlineText”:”John Oliver takes on impeachment”,”headlinePlainText”:”John Oliver takes on impeachment”,”iconImageUrl”:””,”iconType”:”video”,”isMobileBannerText”:false,”kickerText”:””,”maximizedBannerSize”:[],”media”:{“contentType”:”image”,”type”:”element”,”cutFormat”:”16:9″,”elementContents”:{“caption”:”john oliver watergate impeachment lnl es vpx_00004826.jpg”,”imageAlt”:”john oliver watergate impeachment lnl es vpx_00004826″,”imageUrl”:”//cdn.cnn.com/cnnnext/dam/assets/190617063613-john-oliver-watergate-impeachment-lnl-es-vpx-00004826-large-169.jpg”,”label”:””,”galleryTitle”:””,”head”:””,”source”:”HBO”,”photographer”:””,”cuts”:{“mini”:{“width”:220,”type”:”jpg”,”uri”:”//cdn.cnn.com/cnnnext/dam/assets/190617063613-john-oliver-watergate-impeachment-lnl-es-vpx-00004826-small-169.jpg”,”height”:124},”xsmall”:{“width”:307,”type”:”jpg”,”uri”:”//cdn.cnn.com/cnnnext/dam/assets/190617063613-john-oliver-watergate-impeachment-lnl-es-vpx-00004826-medium-plus-169.jpg”,”height”:173},”small”:{“width”:460,”type”:”jpg”,”uri”:”//cdn.cnn.com/cnnnext/dam/assets/190617063613-john-oliver-watergate-impeachment-lnl-es-vpx-00004826-large-169.jpg”,”height”:259},”medium”:{“width”:780,”type”:”jpg”,”uri”:”//cdn.cnn.com/cnnnext/dam/assets/190617063613-john-oliver-watergate-impeachment-lnl-es-vpx-00004826-exlarge-169.jpg”,”height”:438},”large”:{“width”:1100,”type”:”jpg”,”uri”:”//cdn.cnn.com/cnnnext/dam/assets/190617063613-john-oliver-watergate-impeachment-lnl-es-vpx-00004826-super-169.jpg”,”height”:619},”full16x9″:{“width”:1600,”type”:”jpg”,”uri”:”//cdn.cnn.com/cnnnext/dam/assets/190617063613-john-oliver-watergate-impeachment-lnl-es-vpx-00004826-full-169.jpg”,”height”:900},”mini1x1″:{“width”:120,”type”:”jpg”,”uri”:”//cdn.cnn.com/cnnnext/dam/assets/190617063613-john-oliver-watergate-impeachment-lnl-es-vpx-00004826-small-11.jpg”,”height”:120}},”responsiveImage”:true,”originalImageUrl”:”//cdn.cnn.com/cnnnext/dam/assets/190617063613-john-oliver-watergate-impeachment-lnl-es-vpx-00004826.jpg”},”duration”:”0:48″},”noFollow”:false,”overMediaText”:””,”sectionUri”:””,”showSocialSharebar”:false,”shortUrl”:””,”statusText”:””,”statusColor”:””,”targetType”:””,”timestampDisplay”:””,”timestampUtc”:””,”lastModifiedText”:””,”lastModifiedState”:””,”type”:”card”,”url”:”/videos/media/2019/06/17/john-oliver-watergate-impeachment-lnl-es-vpx.cnn/video/playlists/stories-worth-watching/”,”width”:””,”webDisplayName”:”Media”,”height”:””,”videoCMSUri”:”/video/data/3.0/video/media/2019/06/17/john-oliver-watergate-impeachment-lnl-es-vpx.cnn/index.xml”,”videoId”:”media/2019/06/17/john-oliver-watergate-impeachment-lnl-es-vpx.cnn”,”adSection”:”const-video-leaf”,”dateCreated”:”6:25 AM ET, Mon June 17, 2019″,”sourceName”:”CNN”,”sourceLink”:”http://www.cnn.com/”,”showName”:”Early Start”,”showUrl”:”/shows/early-start”,”videoCollectionUrl”:”/video/playlists/stories-worth-watching/”},”contentType”:”video”,”maximizedBanner”:false,”type”:”card”,”autoStartVideo”:false},{“branding”:””,”cardContents”:{“additionalSections”:[],”auxiliaryText”:””,”bannerText”:[],”bannerHasATag”:false,”bannerPosition”:””,”brandingLink”:””,”brandingImageUrl”:””,”brandingTextHead”:””,”brandingTextSub”:””,”cardSectionName”:”business”,”contentType”:””,”cta”:”share”,”descriptionText”:[“On June 15, Target acknowledged guests were “unable to make purchases” at its stores, as shoppers complained on social that cash registers weren’t working.”],”descriptionPlainText”:”On June 15, Target acknowledged guests were “unable to make purchases” at its stores, as shoppers complained on social that cash registers weren’t working.”,”headlinePostText”:””,”headlinePreText”:””,”headlineText”:”Target customers waited for hours to check out after system outage”,”headlinePlainText”:”Target customers waited for hours to check out after system outage”,”iconImageUrl”:””,”iconType”:”video”,”isMobileBannerText”:false,”kickerText”:””,”maximizedBannerSize”:[],”media”:{“contentType”:”image”,”type”:”element”,”cutFormat”:”16:9″,”elementContents”:{“caption”:””,”imageAlt”:””,”imageUrl”:”//cdn.cnn.com/cnnnext/dam/assets/190615145101-01-target-systems-down-0615-large-169.jpg”,”label”:””,”galleryTitle”:””,”head”:””,”source”:”Twitter”,”photographer”:”Final Cut King/Twitter”,”cuts”:{“mini”:{“width”:220,”type”:”jpg”,”uri”:”//cdn.cnn.com/cnnnext/dam/assets/190615145101-01-target-systems-down-0615-small-169.jpg”,”height”:124},”xsmall”:{“width”:307,”type”:”jpg”,”uri”:”//cdn.cnn.com/cnnnext/dam/assets/190615145101-01-target-systems-down-0615-medium-plus-169.jpg”,”height”:173},”small”:{“width”:460,”type”:”jpg”,”uri”:”//cdn.cnn.com/cnnnext/dam/assets/190615145101-01-target-systems-down-0615-large-169.jpg”,”height”:259},”medium”:{“width”:780,”type”:”jpg”,”uri”:”//cdn.cnn.com/cnnnext/dam/assets/190615145101-01-target-systems-down-0615-exlarge-169.jpg”,”height”:438},”large”:{“width”:1100,”type”:”jpg”,”uri”:”//cdn.cnn.com/cnnnext/dam/assets/190615145101-01-target-systems-down-0615-super-169.jpg”,”height”:619},”full16x9″:{“width”:1600,”type”:”jpg”,”uri”:”//cdn.cnn.com/cnnnext/dam/assets/190615145101-01-target-systems-down-0615-full-169.jpg”,”height”:900},”mini1x1″:{“width”:120,”type”:”jpg”,”uri”:”//cdn.cnn.com/cnnnext/dam/assets/190615145101-01-target-systems-down-0615-small-11.jpg”,”height”:120}},”responsiveImage”:true,”originalImageUrl”:”//cdn.cnn.com/cnnnext/dam/assets/190615145101-01-target-systems-down-0615.jpg”},”duration”:”1:02″},”noFollow”:false,”overMediaText”:””,”sectionUri”:””,”showSocialSharebar”:false,”shortUrl”:””,”statusText”:””,”statusColor”:””,”targetType”:””,”timestampDisplay”:””,”timestampUtc”:””,”lastModifiedText”:””,”lastModifiedState”:””,”type”:”card”,”url”:”/videos/business/2019/06/15/target-outage-long-lines.cnn-business/video/playlists/stories-worth-watching/”,”width”:””,”webDisplayName”:”Business”,”height”:””,”videoCMSUri”:”/video/data/3.0/video/business/2019/06/15/target-outage-long-lines.cnn-business/index.xml”,”videoId”:”business/2019/06/15/target-outage-long-lines.cnn-business”,”adSection”:”const-video-leaf”,”dateCreated”:”5:05 PM ET, Sat June 15, 2019″,”sourceName”:”CNN Business”,”sourceLink”:””,”videoCollectionUrl”:”/video/playlists/stories-worth-watching/”},”contentType”:”video”,”maximizedBanner”:false,”type”:”card”,”autoStartVideo”:false},{“branding”:””,”cardContents”:{“additionalSections”:[],”auxiliaryText”:””,”bannerText”:[],”bannerHasATag”:false,”bannerPosition”:””,”brandingLink”:””,”brandingImageUrl”:””,”brandingTextHead”:””,”brandingTextSub”:””,”cardSectionName”:”media”,”contentType”:””,”cta”:”share”,”descriptionText”:[“Late-night comedians aren’t looking the other way when it comes to Trump’s tweet in which he refers to Prince Charles as the “Prince of Whales.””],”descriptionPlainText”:”Late-night comedians aren’t looking the other way when it comes to Trump’s tweet in which he refers to Prince Charles as the “Prince of Whales.””,”headlinePostText”:””,”headlinePreText”:””,”headlineText”:”Trump’s tweet confuses an animal with a country”,”headlinePlainText”:”Trump’s tweet confuses an animal with a country”,”iconImageUrl”:””,”iconType”:”video”,”isMobileBannerText”:false,”kickerText”:””,”maximizedBannerSize”:[],”media”:{“contentType”:”image”,”type”:”element”,”cutFormat”:”16:9″,”elementContents”:{“caption”:””,”imageAlt”:””,”imageUrl”:”//cdn.cnn.com/cnnnext/dam/assets/190614084605-late-night-laughs-trump-prince-of-whales-03-large-169.jpg”,”label”:””,”galleryTitle”:””,”head”:””,”source”:”CBS/”The Late Show with Stephen Colbert””,”photographer”:”CBS/”The Late Show with Stephen Colbert””,”cuts”:{“mini”:{“width”:220,”type”:”jpg”,”uri”:”//cdn.cnn.com/cnnnext/dam/assets/190614084605-late-night-laughs-trump-prince-of-whales-03-small-169.jpg”,”height”:124},”xsmall”:{“width”:307,”type”:”jpg”,”uri”:”//cdn.cnn.com/cnnnext/dam/assets/190614084605-late-night-laughs-trump-prince-of-whales-03-medium-plus-169.jpg”,”height”:173},”small”:{“width”:460,”type”:”jpg”,”uri”:”//cdn.cnn.com/cnnnext/dam/assets/190614084605-late-night-laughs-trump-prince-of-whales-03-large-169.jpg”,”height”:259},”medium”:{“width”:780,”type”:”jpg”,”uri”:”//cdn.cnn.com/cnnnext/dam/assets/190614084605-late-night-laughs-trump-prince-of-whales-03-exlarge-169.jpg”,”height”:438},”large”:{“width”:1100,”type”:”jpg”,”uri”:”//cdn.cnn.com/cnnnext/dam/assets/190614084605-late-night-laughs-trump-prince-of-whales-03-super-169.jpg”,”height”:619},”full16x9″:{“width”:1600,”type”:”jpg”,”uri”:”//cdn.cnn.com/cnnnext/dam/assets/190614084605-late-night-laughs-trump-prince-of-whales-03-full-169.jpg”,”height”:900},”mini1x1″:{“width”:120,”type”:”jpg”,”uri”:”//cdn.cnn.com/cnnnext/dam/assets/190614084605-late-night-laughs-trump-prince-of-whales-03-small-11.jpg”,”height”:120}},”responsiveImage”:true,”originalImageUrl”:”//cdn.cnn.com/cnnnext/dam/assets/190614084605-late-night-laughs-trump-prince-of-whales-03.jpg”},”duration”:”1:09″},”noFollow”:false,”overMediaText”:””,”sectionUri”:””,”showSocialSharebar”:false,”shortUrl”:””,”statusText”:””,”statusColor”:””,”targetType”:””,”timestampDisplay”:””,”timestampUtc”:””,”lastModifiedText”:””,”lastModifiedState”:””,”type”:”card”,”url”:”/videos/media/2019/06/14/late-night-laughs-trump-tweet-prince-of-whales-newday-vpx.cnn/video/playlists/stories-worth-watching/”,”width”:””,”webDisplayName”:”Media”,”height”:””,”videoCMSUri”:”/video/data/3.0/video/media/2019/06/14/late-night-laughs-trump-tweet-prince-of-whales-newday-vpx.cnn/index.xml”,”videoId”:”media/2019/06/14/late-night-laughs-trump-tweet-prince-of-whales-newday-vpx.cnn”,”adSection”:”const-video-leaf”,”dateCreated”:”7:19 AM ET, Fri June 14, 2019″,”sourceName”:”CNN”,”sourceLink”:”https://www.cnn.com”,”showName”:”New Day”,”showUrl”:”/shows/new-day”,”videoCollectionUrl”:”/video/playlists/stories-worth-watching/”},”contentType”:”video”,”maximizedBanner”:false,”type”:”card”,”autoStartVideo”:false},{“branding”:””,”cardContents”:{“additionalSections”:[],”auxiliaryText”:””,”bannerText”:[],”bannerHasATag”:false,”bannerPosition”:””,”brandingLink”:””,”brandingImageUrl”:””,”brandingTextHead”:””,”brandingTextSub”:””,”cardSectionName”:”business”,”contentType”:””,”cta”:”share”,”descriptionText”:[“In India widespread droughts in recent years mean farmers often struggle to find enough water for their fields. Khethworks has developed a solar-powered irrigation system that doesn’t depend on seasonal rains or expensive fuel. “],”descriptionPlainText”:”In India widespread droughts in recent years mean farmers often struggle to find enough water for their fields. Khethworks has developed a solar-powered irrigation system that doesn’t depend on seasonal rains or expensive fuel.”,”headlinePostText”:””,”headlinePreText”:””,”headlineText”:”Farmers are using the sun to help water their crops”,”headlinePlainText”:”Farmers are using the sun to help water their crops”,”iconImageUrl”:””,”iconType”:”video”,”isMobileBannerText”:false,”kickerText”:””,”maximizedBannerSize”:[],”media”:{“contentType”:”image”,”type”:”element”,”cutFormat”:”16:9″,”elementContents”:{“caption”:”Water pours down an irrigation channel from a groundwater pump and well next to a field of rice growing on farmland in the Bhagpat district of Uttar Pradesh, India, on Monday, Sept. 3, 2018. Cumulative rainfall during August and September is forecast to be 95 percent of a 50-year average, according to the India Meteorological Department. The monsoon is critical to the farm sector as it accounts for more than 70 percent of India’s annual showers and irrigates more than half the country’s farmland. Photographer: Prashanth Vishwanathan/Bloomberg via Getty Images”,”imageAlt”:”Water pours down an irrigation channel from a groundwater pump and well next to a field of rice growing on farmland in the Bhagpat district of Uttar Pradesh, India, on Monday, Sept. 3, 2018. Cumulative rainfall during August and September is forecast to be 95 percent of a 50-year average, according to the India Meteorological Department. The monsoon is critical to the farm sector as it accounts for more than 70 percent of India’s annual showers and irrigates more than half the country’s farmland. Photographer: Prashanth Vishwanathan/Bloomberg via Getty Images”,”imageUrl”:”//cdn.cnn.com/cnnnext/dam/assets/190611150152-india-solar-irrigation-khethworks-02-restricted-large-169.jpg”,”label”:””,”galleryTitle”:””,”head”:””,”source”:”Getty”,”photographer”:”Prashanth Vishwanathan/Bloomberg/Getty Images”,”cuts”:{“mini”:{“width”:220,”type”:”jpg”,”uri”:”//cdn.cnn.com/cnnnext/dam/assets/190611150152-india-solar-irrigation-khethworks-02-restricted-small-169.jpg”,”height”:124},”xsmall”:{“width”:307,”type”:”jpg”,”uri”:”//cdn.cnn.com/cnnnext/dam/assets/190611150152-india-solar-irrigation-khethworks-02-restricted-medium-plus-169.jpg”,”height”:173},”small”:{“width”:460,”type”:”jpg”,”uri”:”//cdn.cnn.com/cnnnext/dam/assets/190611150152-india-solar-irrigation-khethworks-02-restricted-large-169.jpg”,”height”:259},”medium”:{“width”:780,”type”:”jpg”,”uri”:”//cdn.cnn.com/cnnnext/dam/assets/190611150152-india-solar-irrigation-khethworks-02-restricted-exlarge-169.jpg”,”height”:438},”large”:{“width”:1100,”type”:”jpg”,”uri”:”//cdn.cnn.com/cnnnext/dam/assets/190611150152-india-solar-irrigation-khethworks-02-restricted-super-169.jpg”,”height”:619},”full16x9″:{“width”:1600,”type”:”jpg”,”uri”:”//cdn.cnn.com/cnnnext/dam/assets/190611150152-india-solar-irrigation-khethworks-02-restricted-full-169.jpg”,”height”:900},”mini1x1″:{“width”:120,”type”:”jpg”,”uri”:”//cdn.cnn.com/cnnnext/dam/assets/190611150152-india-solar-irrigation-khethworks-02-restricted-small-11.jpg”,”height”:120}},”responsiveImage”:true,”originalImageUrl”:”//cdn.cnn.com/cnnnext/dam/assets/190611150152-india-solar-irrigation-khethworks-02-restricted.jpg”},”duration”:”2:16″},”noFollow”:false,”overMediaText”:””,”sectionUri”:””,”showSocialSharebar”:false,”shortUrl”:””,”statusText”:””,”statusColor”:””,”targetType”:””,”timestampDisplay”:””,”timestampUtc”:””,”lastModifiedText”:””,”lastModifiedState”:””,”type”:”card”,”url”:”/videos/business/2019/06/17/solar-irrigation-india-khethworks.cnn/video/playlists/stories-worth-watching/”,”width”:””,”webDisplayName”:”Business”,”height”:””,”videoCMSUri”:”/video/data/3.0/video/business/2019/06/17/solar-irrigation-india-khethworks.cnn/index.xml”,”videoId”:”business/2019/06/17/solar-irrigation-india-khethworks.cnn”,”adSection”:”const-video-leaf”,”dateCreated”:”10:47 AM ET, Mon June 17, 2019″,”sourceName”:”CNN”,”sourceLink”:””,”videoCollectionUrl”:”/video/playlists/stories-worth-watching/”},”contentType”:”video”,”maximizedBanner”:false,”type”:”card”,”autoStartVideo”:false},{“branding”:””,”cardContents”:{“additionalSections”:[],”auxiliaryText”:””,”bannerText”:[],”bannerHasATag”:false,”bannerPosition”:””,”brandingLink”:””,”brandingImageUrl”:””,”brandingTextHead”:””,”brandingTextSub”:””,”cardSectionName”:”media”,”contentType”:””,”cta”:”share”,”descriptionText”:[“CNN’s political analyst Brian Karem says that Sarah Sanders’ legacy will be one of obfuscation and divisiveness born out of the ending of the daily White House press briefings.”],”descriptionPlainText”:”CNN’s political analyst Brian Karem says that Sarah Sanders’ legacy will be one of obfuscation and divisiveness born out of the ending of the daily White House press briefings.”,”headlinePostText”:””,”headlinePreText”:””,”headlineText”:”CNN analyst: Sarah Sanders’ legacy is divisiveness”,”headlinePlainText”:”CNN analyst: Sarah Sanders’ legacy is divisiveness”,”iconImageUrl”:””,”iconType”:”video”,”isMobileBannerText”:false,”kickerText”:””,”maximizedBannerSize”:[],”media”:{“contentType”:”image”,”type”:”element”,”cutFormat”:”16:9″,”elementContents”:{“caption”:”WASHINGTON, DC – JULY 26: White House Press Secretary Sarah Huckabee Sanders speaks to the media during the daily press briefing at the White House on July 26, 2017 in Washington, DC. (Photo by Mark Wilson/Getty Images)”,”imageAlt”:”WASHINGTON, DC – JULY 26: White House Press Secretary Sarah Huckabee Sanders speaks to the media during the daily press briefing at the White House on July 26, 2017 in Washington, DC. (Photo by Mark Wilson/Getty Images)”,”imageUrl”:”//cdn.cnn.com/cnnnext/dam/assets/190613195905-10-sarah-sanders-gallery-large-169.jpg”,”label”:””,”galleryTitle”:””,”head”:””,”source”:”Getty Images”,”photographer”:”Mark Wilson/Getty Images”,”cuts”:{“mini”:{“width”:220,”type”:”jpg”,”uri”:”//cdn.cnn.com/cnnnext/dam/assets/190613195905-10-sarah-sanders-gallery-small-169.jpg”,”height”:124},”xsmall”:{“width”:307,”type”:”jpg”,”uri”:”//cdn.cnn.com/cnnnext/dam/assets/190613195905-10-sarah-sanders-gallery-medium-plus-169.jpg”,”height”:173},”small”:{“width”:460,”type”:”jpg”,”uri”:”//cdn.cnn.com/cnnnext/dam/assets/190613195905-10-sarah-sanders-gallery-large-169.jpg”,”height”:259},”medium”:{“width”:780,”type”:”jpg”,”uri”:”//cdn.cnn.com/cnnnext/dam/assets/190613195905-10-sarah-sanders-gallery-exlarge-169.jpg”,”height”:438},”large”:{“width”:1100,”type”:”jpg”,”uri”:”//cdn.cnn.com/cnnnext/dam/assets/190613195905-10-sarah-sanders-gallery-super-169.jpg”,”height”:619},”full16x9″:{“width”:1600,”type”:”jpg”,”uri”:”//cdn.cnn.com/cnnnext/dam/assets/190613195905-10-sarah-sanders-gallery-full-169.jpg”,”height”:900},”mini1x1″:{“width”:120,”type”:”jpg”,”uri”:”//cdn.cnn.com/cnnnext/dam/assets/190613195905-10-sarah-sanders-gallery-small-11.jpg”,”height”:120}},”responsiveImage”:true,”originalImageUrl”:”//cdn.cnn.com/cnnnext/dam/assets/190613195905-10-sarah-sanders-gallery.jpg”},”duration”:”1:13″},”noFollow”:false,”overMediaText”:””,”sectionUri”:””,”showSocialSharebar”:false,”shortUrl”:””,”statusText”:””,”statusColor”:””,”targetType”:””,”timestampDisplay”:””,”timestampUtc”:””,”lastModifiedText”:””,”lastModifiedState”:””,”type”:”card”,”url”:”/videos/media/2019/06/14/sarah-sanders-white-house-press-briefings-legacy-karem-cnn-today-sot.cnn/video/playlists/stories-worth-watching/”,”width”:””,”webDisplayName”:”Media”,”height”:””,”videoCMSUri”:”/video/data/3.0/video/media/2019/06/14/sarah-sanders-white-house-press-briefings-legacy-karem-cnn-today-sot.cnn/index.xml”,”videoId”:”media/2019/06/14/sarah-sanders-white-house-press-briefings-legacy-karem-cnn-today-sot.cnn”,”adSection”:”const-video-leaf”,”dateCreated”:”7:25 AM ET, Fri June 14, 2019″,”sourceName”:”CNN”,”sourceLink”:”https://www.cnn.com/business”,”videoCollectionUrl”:”/video/playlists/stories-worth-watching/”},”contentType”:”video”,”maximizedBanner”:false,”type”:”card”,”autoStartVideo”:false},{“branding”:””,”cardContents”:{“additionalSections”:[“business”],”auxiliaryText”:””,”bannerText”:[],”bannerHasATag”:false,”bannerPosition”:””,”brandingLink”:””,”brandingImageUrl”:””,”brandingTextHead”:””,”brandingTextSub”:””,”cardSectionName”:”media”,”contentType”:””,”cta”:”share”,”descriptionText”:[“Joe Crain, a local Illinois weatherman criticized his own news station’s “Code Red” weather alerts. Now he’s out of a job.”],”descriptionPlainText”:”Joe Crain, a local Illinois weatherman criticized his own news station’s “Code Red” weather alerts. Now he’s out of a job.”,”headlinePostText”:””,”headlinePreText”:””,”headlineText”:”Meteorologist fired after criticizing his TV station on air”,”headlinePlainText”:”Meteorologist fired after criticizing his TV station on air”,”iconImageUrl”:””,”iconType”:”video”,”isMobileBannerText”:false,”kickerText”:””,”maximizedBannerSize”:[],”media”:{“contentType”:”image”,”type”:”element”,”cutFormat”:”16:9″,”elementContents”:{“caption”:””,”imageAlt”:””,”imageUrl”:”//cdn.cnn.com/cnnnext/dam/assets/190609114115-01-weatherman-code-red-alert-joe-crain-large-169.jpg”,”label”:””,”galleryTitle”:””,”head”:””,”source”:”Twitter”,”photographer”:”217 Problems/Twitter”,”cuts”:{“mini”:{“width”:220,”type”:”jpg”,”uri”:”//cdn.cnn.com/cnnnext/dam/assets/190609114115-01-weatherman-code-red-alert-joe-crain-small-169.jpg”,”height”:124},”xsmall”:{“width”:307,”type”:”jpg”,”uri”:”//cdn.cnn.com/cnnnext/dam/assets/190609114115-01-weatherman-code-red-alert-joe-crain-medium-plus-169.jpg”,”height”:173},”small”:{“width”:460,”type”:”jpg”,”uri”:”//cdn.cnn.com/cnnnext/dam/assets/190609114115-01-weatherman-code-red-alert-joe-crain-large-169.jpg”,”height”:259},”medium”:{“width”:780,”type”:”jpg”,”uri”:”//cdn.cnn.com/cnnnext/dam/assets/190609114115-01-weatherman-code-red-alert-joe-crain-exlarge-169.jpg”,”height”:438},”large”:{“width”:1100,”type”:”jpg”,”uri”:”//cdn.cnn.com/cnnnext/dam/assets/190609114115-01-weatherman-code-red-alert-joe-crain-super-169.jpg”,”height”:619},”full16x9″:{“width”:1600,”type”:”jpg”,”uri”:”//cdn.cnn.com/cnnnext/dam/assets/190609114115-01-weatherman-code-red-alert-joe-crain-full-169.jpg”,”height”:900},”mini1x1″:{“width”:120,”type”:”jpg”,”uri”:”//cdn.cnn.com/cnnnext/dam/assets/190609114115-01-weatherman-code-red-alert-joe-crain-small-11.jpg”,”height”:120}},”responsiveImage”:true,”originalImageUrl”:”//cdn.cnn.com/cnnnext/dam/assets/190609114115-01-weatherman-code-red-alert-joe-crain.jpg”},”duration”:”1:46″},”noFollow”:false,”overMediaText”:””,”sectionUri”:””,”showSocialSharebar”:false,”shortUrl”:””,”statusText”:””,”statusColor”:””,”targetType”:””,”timestampDisplay”:””,”timestampUtc”:””,”lastModifiedText”:””,”lastModifiedState”:””,”type”:”card”,”url”:”/videos/media/2019/06/10/weatherman-illinois-code-red-orig-bu.cnn/video/playlists/stories-worth-watching/”,”width”:””,”webDisplayName”:”Media”,”height”:””,”videoCMSUri”:”/video/data/3.0/video/media/2019/06/10/weatherman-illinois-code-red-orig-bu.cnn/index.xml”,”videoId”:”media/2019/06/10/weatherman-illinois-code-red-orig-bu.cnn”,”adSection”:”const-video-leaf”,”dateCreated”:”3:07 PM ET, Mon June 10, 2019″,”sourceName”:”CNN”,”sourceLink”:”http://www.cnn.com/”,”videoCollectionUrl”:”/video/playlists/stories-worth-watching/”},”contentType”:”video”,”maximizedBanner”:false,”type”:”card”,”autoStartVideo”:false},{“branding”:””,”cardContents”:{“additionalSections”:[],”auxiliaryText”:””,”bannerText”:[],”bannerHasATag”:false,”bannerPosition”:””,”brandingLink”:””,”brandingImageUrl”:””,”brandingTextHead”:””,”brandingTextSub”:””,”cardSectionName”:”business”,”contentType”:””,”cta”:”share”,”descriptionText”:[“Airbus has annouced a 100 new orders and unveiled a new airliner at the Paris Air Show while Boeing has stalled while dealing with the grounding of their 737 Max jets. CNN’s Melissa Bell reports.”],”descriptionPlainText”:”Airbus has annouced a 100 new orders and unveiled a new airliner at the Paris Air Show while Boeing has stalled while dealing with the grounding of their 737 Max jets. CNN’s Melissa Bell reports.”,”headlinePostText”:””,”headlinePreText”:””,”headlineText”:”Airbus exploits Boeing’s 737 Max woes”,”headlinePlainText”:”Airbus exploits Boeing’s 737 Max woes”,”iconImageUrl”:””,”iconType”:”video”,”isMobileBannerText”:false,”kickerText”:””,”maximizedBannerSize”:[],”media”:{“contentType”:”image”,”type”:”element”,”cutFormat”:”16:9″,”elementContents”:{“caption”:”An Airbus A330neo aircraft flies during the inauguration of the 53rd International Paris Air Show at Le Bourget Airport near Paris, on June 17, 2019. (Photo by BENOIT TESSIER / POOL / AFP)”,”imageAlt”:”An Airbus A330neo aircraft flies during the inauguration of the 53rd International Paris Air Show at Le Bourget Airport near Paris, on June 17, 2019. (Photo by BENOIT TESSIER / POOL / AFP)”,”imageUrl”:”//cdn.cnn.com/cnnnext/dam/assets/190617123232-airbus-paris-air-show-june-17-2019-01-large-169.jpg”,”label”:””,”galleryTitle”:””,”head”:””,”source”:”AFP/Getty Images”,”photographer”:”BENOIT TESSIER/AFP/AFP/Getty Images”,”cuts”:{“mini”:{“width”:220,”type”:”jpg”,”uri”:”//cdn.cnn.com/cnnnext/dam/assets/190617123232-airbus-paris-air-show-june-17-2019-01-small-169.jpg”,”height”:124},”xsmall”:{“width”:307,”type”:”jpg”,”uri”:”//cdn.cnn.com/cnnnext/dam/assets/190617123232-airbus-paris-air-show-june-17-2019-01-medium-plus-169.jpg”,”height”:173},”small”:{“width”:460,”type”:”jpg”,”uri”:”//cdn.cnn.com/cnnnext/dam/assets/190617123232-airbus-paris-air-show-june-17-2019-01-large-169.jpg”,”height”:259},”medium”:{“width”:780,”type”:”jpg”,”uri”:”//cdn.cnn.com/cnnnext/dam/assets/190617123232-airbus-paris-air-show-june-17-2019-01-exlarge-169.jpg”,”height”:438},”large”:{“width”:1100,”type”:”jpg”,”uri”:”//cdn.cnn.com/cnnnext/dam/assets/190617123232-airbus-paris-air-show-june-17-2019-01-super-169.jpg”,”height”:619},”full16x9″:{“width”:1600,”type”:”jpg”,”uri”:”//cdn.cnn.com/cnnnext/dam/assets/190617123232-airbus-paris-air-show-june-17-2019-01-full-169.jpg”,”height”:900},”mini1x1″:{“width”:120,”type”:”jpg”,”uri”:”//cdn.cnn.com/cnnnext/dam/assets/190617123232-airbus-paris-air-show-june-17-2019-01-small-11.jpg”,”height”:120}},”responsiveImage”:true,”originalImageUrl”:”//cdn.cnn.com/cnnnext/dam/assets/190617123232-airbus-paris-air-show-june-17-2019-01.jpg”},”duration”:”1:45″},”noFollow”:false,”overMediaText”:””,”sectionUri”:””,”showSocialSharebar”:false,”shortUrl”:””,”statusText”:””,”statusColor”:””,”targetType”:””,”timestampDisplay”:””,”timestampUtc”:””,”lastModifiedText”:””,”lastModifiedState”:””,”type”:”card”,”url”:”/videos/business/2019/06/17/boeing-airbus-paris-air-show-bell-the-express.cnn/video/playlists/stories-worth-watching/”,”width”:””,”webDisplayName”:”Business”,”height”:””,”videoCMSUri”:”/video/data/3.0/video/business/2019/06/17/boeing-airbus-paris-air-show-bell-the-express.cnn/index.xml”,”videoId”:”business/2019/06/17/boeing-airbus-paris-air-show-bell-the-express.cnn”,”adSection”:”const-video-leaf”,”dateCreated”:”12:26 PM ET, Mon June 17, 2019″,”sourceName”:”CNN”,”sourceLink”:”http://www.cnn.com/”,”videoCollectionUrl”:”/video/playlists/stories-worth-watching/”},”contentType”:”video”,”maximizedBanner”:false,”type”:”card”,”autoStartVideo”:false},{“branding”:””,”cardContents”:{“additionalSections”:[],”auxiliaryText”:””,”bannerText”:[],”bannerHasATag”:false,”bannerPosition”:””,”brandingLink”:””,”brandingImageUrl”:””,”brandingTextHead”:””,”brandingTextSub”:””,”cardSectionName”:”business”,”contentType”:””,”cta”:”share”,”descriptionText”:[“Rays Power Infra is one of India’s largest solar power companies, with ambitious plans to harness the 300 days of sun that India receives each year. Co-founder and CEO Ketan Mehta describes the untapped opportunities for solar energy in the country.”],”descriptionPlainText”:”Rays Power Infra is one of India’s largest solar power companies, with ambitious plans to harness the 300 days of sun that India receives each year. Co-founder and CEO Ketan Mehta describes the untapped opportunities for solar energy in the country.”,”headlinePostText”:””,”headlinePreText”:””,”headlineText”:”Harnessing India’s sunny days for power”,”headlinePlainText”:”Harnessing India’s sunny days for power”,”iconImageUrl”:””,”iconType”:”video”,”isMobileBannerText”:false,”kickerText”:””,”maximizedBannerSize”:[],”media”:{“contentType”:”image”,”type”:”element”,”cutFormat”:”16:9″,”elementContents”:{“caption”:””,”imageAlt”:””,”imageUrl”:”//cdn.cnn.com/cnnnext/dam/assets/180928111730-rays-power-india-large-169.jpg”,”label”:””,”galleryTitle”:””,”head”:””,”source”:”CNN”,”photographer”:””,”cuts”:{“mini”:{“width”:220,”type”:”jpg”,”uri”:”//cdn.cnn.com/cnnnext/dam/assets/180928111730-rays-power-india-small-169.jpg”,”height”:124},”xsmall”:{“width”:307,”type”:”jpg”,”uri”:”//cdn.cnn.com/cnnnext/dam/assets/180928111730-rays-power-india-medium-plus-169.jpg”,”height”:173},”small”:{“width”:460,”type”:”jpg”,”uri”:”//cdn.cnn.com/cnnnext/dam/assets/180928111730-rays-power-india-large-169.jpg”,”height”:259},”medium”:{“width”:780,”type”:”jpg”,”uri”:”//cdn.cnn.com/cnnnext/dam/assets/180928111730-rays-power-india-exlarge-169.jpg”,”height”:438},”large”:{“width”:1100,”type”:”jpg”,”uri”:”//cdn.cnn.com/cnnnext/dam/assets/180928111730-rays-power-india-super-169.jpg”,”height”:619},”full16x9″:{“width”:1600,”type”:”jpg”,”uri”:”//cdn.cnn.com/cnnnext/dam/assets/180928111730-rays-power-india-full-169.jpg”,”height”:900},”mini1x1″:{“width”:120,”type”:”jpg”,”uri”:”//cdn.cnn.com/cnnnext/dam/assets/180928111730-rays-power-india-small-11.jpg”,”height”:120}},”responsiveImage”:true,”originalImageUrl”:”//cdn.cnn.com/cnnnext/dam/assets/180928111730-rays-power-india.jpg”},”duration”:”1:43″},”noFollow”:false,”overMediaText”:””,”sectionUri”:””,”showSocialSharebar”:false,”shortUrl”:””,”statusText”:””,”statusColor”:””,”targetType”:””,”timestampDisplay”:””,”timestampUtc”:””,”lastModifiedText”:””,”lastModifiedState”:””,”type”:”card”,”url”:”/videos/business/2018/09/28/rays-power-india.cnn-business/video/playlists/stories-worth-watching/”,”width”:””,”webDisplayName”:”Business”,”height”:””,”videoCMSUri”:”/video/data/3.0/video/business/2018/09/28/rays-power-india.cnn-business/index.xml”,”videoId”:”business/2018/09/28/rays-power-india.cnn-business”,”adSection”:”const-video-leaf”,”dateCreated”:”11:10 AM ET, Fri September 28, 2018″,”sourceName”:”CNN Business”,”sourceLink”:””,”videoCollectionUrl”:”/video/playlists/stories-worth-watching/”},”contentType”:”video”,”maximizedBanner”:false,”type”:”card”,”autoStartVideo”:false},{“branding”:””,”cardContents”:{“additionalSections”:[],”auxiliaryText”:””,”bannerText”:[],”bannerHasATag”:false,”bannerPosition”:””,”brandingLink”:””,”brandingImageUrl”:””,”brandingTextHead”:””,”brandingTextSub”:””,”cardSectionName”:”business”,”contentType”:””,”cta”:”share”,”descriptionText”:[“A video of people abusing a robot — and later, the robot exacting revenge — went viral. Turns out, the whole video was faked.”],”descriptionPlainText”:”A video of people abusing a robot — and later, the robot exacting revenge — went viral. Turns out, the whole video was faked.”,”headlinePostText”:””,”headlinePreText”:””,”headlineText”:”That viral video of a robot being beaten up isn’t real”,”headlinePlainText”:”That viral video of a robot being beaten up isn’t real”,”iconImageUrl”:””,”iconType”:”video”,”isMobileBannerText”:false,”kickerText”:””,”maximizedBannerSize”:[],”media”:{“contentType”:”image”,”type”:”element”,”cutFormat”:”16:9″,”elementContents”:{“caption”:””,”imageAlt”:””,”imageUrl”:”//cdn.cnn.com/cnnnext/dam/assets/190617162549-04-robot-parody-grab-large-169.jpg”,”label”:””,”galleryTitle”:””,”head”:””,”source”:”Collider/YouTube”,”photographer”:”Collider Digital/YouTube”,”cuts”:{“mini”:{“width”:220,”type”:”jpg”,”uri”:”//cdn.cnn.com/cnnnext/dam/assets/190617162549-04-robot-parody-grab-small-169.jpg”,”height”:124},”xsmall”:{“width”:307,”type”:”jpg”,”uri”:”//cdn.cnn.com/cnnnext/dam/assets/190617162549-04-robot-parody-grab-medium-plus-169.jpg”,”height”:173},”small”:{“width”:460,”type”:”jpg”,”uri”:”//cdn.cnn.com/cnnnext/dam/assets/190617162549-04-robot-parody-grab-large-169.jpg”,”height”:259},”medium”:{“width”:780,”type”:”jpg”,”uri”:”//cdn.cnn.com/cnnnext/dam/assets/190617162549-04-robot-parody-grab-exlarge-169.jpg”,”height”:438},”large”:{“width”:1100,”type”:”jpg”,”uri”:”//cdn.cnn.com/cnnnext/dam/assets/190617162549-04-robot-parody-grab-super-169.jpg”,”height”:619},”full16x9″:{“width”:1600,”type”:”jpg”,”uri”:”//cdn.cnn.com/cnnnext/dam/assets/190617162549-04-robot-parody-grab-full-169.jpg”,”height”:900},”mini1x1″:{“width”:120,”type”:”jpg”,”uri”:”//cdn.cnn.com/cnnnext/dam/assets/190617162549-04-robot-parody-grab-small-11.jpg”,”height”:120}},”responsiveImage”:true,”originalImageUrl”:”//cdn.cnn.com/cnnnext/dam/assets/190617162549-04-robot-parody-grab.jpg”},”duration”:”0:57″},”noFollow”:false,”overMediaText”:””,”sectionUri”:””,”showSocialSharebar”:false,”shortUrl”:””,”statusText”:””,”statusColor”:””,”targetType”:””,”timestampDisplay”:””,”timestampUtc”:””,”lastModifiedText”:””,”lastModifiedState”:””,”type”:”card”,”url”:”/videos/business/2019/06/17/fake-robot-abuse-video-orig.cnn/video/playlists/stories-worth-watching/”,”width”:””,”webDisplayName”:””,”height”:””,”videoCMSUri”:”/video/data/3.0/video/business/2019/06/17/fake-robot-abuse-video-orig.cnn/index.xml”,”videoId”:”business/2019/06/17/fake-robot-abuse-video-orig.cnn”,”adSection”:”const-video-leaf”,”dateCreated”:”4:14 PM ET, Mon June 17, 2019″,”sourceName”:”CNN”,”sourceLink”:””,”videoCollectionUrl”:”/video/playlists/stories-worth-watching/”},”contentType”:”video”,”maximizedBanner”:false,”type”:”card”,”autoStartVideo”:false},{“branding”:””,”cardContents”:{“additionalSections”:[],”auxiliaryText”:””,”bannerText”:[],”bannerHasATag”:false,”bannerPosition”:””,”brandingLink”:””,”brandingImageUrl”:””,”brandingTextHead”:””,”brandingTextSub”:””,”cardSectionName”:”business”,”contentType”:””,”cta”:”share”,”descriptionText”:[“John Stamos and his wife Caitlin McHugh gave Architectural Digest a tour of their Beverly Hills home, which they share with their 14-month-old son Billy.”],”descriptionPlainText”:”John Stamos and his wife Caitlin McHugh gave Architectural Digest a tour of their Beverly Hills home, which they share with their 14-month-old son Billy.”,”headlinePostText”:””,”headlinePreText”:””,”headlineText”:”John Stamos hosts tour of his ‘sturdy, funky, cool’ home”,”headlinePlainText”:”John Stamos hosts tour of his ‘sturdy, funky, cool’ home”,”iconImageUrl”:””,”iconType”:”video”,”isMobileBannerText”:false,”kickerText”:””,”maximizedBannerSize”:[],”media”:{“contentType”:”image”,”type”:”element”,”cutFormat”:”16:9″,”elementContents”:{“caption”:””,”imageAlt”:””,”imageUrl”:”//cdn.cnn.com/cnnnext/dam/assets/190613111806-john-stamos-home-tour-with-son-billy-large-169.jpg”,”label”:””,”galleryTitle”:””,”head”:””,”source”:”Architectural Digest/YouTube”,”photographer”:”Architectural Digest/YouTube”,”cuts”:{“mini”:{“width”:220,”type”:”jpg”,”uri”:”//cdn.cnn.com/cnnnext/dam/assets/190613111806-john-stamos-home-tour-with-son-billy-small-169.jpg”,”height”:124},”xsmall”:{“width”:307,”type”:”jpg”,”uri”:”//cdn.cnn.com/cnnnext/dam/assets/190613111806-john-stamos-home-tour-with-son-billy-medium-plus-169.jpg”,”height”:173},”small”:{“width”:460,”type”:”jpg”,”uri”:”//cdn.cnn.com/cnnnext/dam/assets/190613111806-john-stamos-home-tour-with-son-billy-large-169.jpg”,”height”:259},”medium”:{“width”:780,”type”:”jpg”,”uri”:”//cdn.cnn.com/cnnnext/dam/assets/190613111806-john-stamos-home-tour-with-son-billy-exlarge-169.jpg”,”height”:438},”large”:{“width”:1100,”type”:”jpg”,”uri”:”//cdn.cnn.com/cnnnext/dam/assets/190613111806-john-stamos-home-tour-with-son-billy-super-169.jpg”,”height”:619},”full16x9″:{“width”:1600,”type”:”jpg”,”uri”:”//cdn.cnn.com/cnnnext/dam/assets/190613111806-john-stamos-home-tour-with-son-billy-full-169.jpg”,”height”:900},”mini1x1″:{“width”:120,”type”:”jpg”,”uri”:”//cdn.cnn.com/cnnnext/dam/assets/190613111806-john-stamos-home-tour-with-son-billy-small-11.jpg”,”height”:120}},”responsiveImage”:true,”originalImageUrl”:”//cdn.cnn.com/cnnnext/dam/assets/190613111806-john-stamos-home-tour-with-son-billy.jpg”},”duration”:”1:18″},”noFollow”:false,”overMediaText”:””,”sectionUri”:””,”showSocialSharebar”:false,”shortUrl”:””,”statusText”:””,”statusColor”:””,”targetType”:””,”timestampDisplay”:””,”timestampUtc”:””,”lastModifiedText”:””,”lastModifiedState”:””,”type”:”card”,”url”:”/videos/business/2019/06/13/john-stamos-house-tour-orig-vstop-bdk.cnn/video/playlists/stories-worth-watching/”,”width”:””,”webDisplayName”:”Business”,”height”:””,”videoCMSUri”:”/video/data/3.0/video/business/2019/06/13/john-stamos-house-tour-orig-vstop-bdk.cnn/index.xml”,”videoId”:”business/2019/06/13/john-stamos-house-tour-orig-vstop-bdk.cnn”,”adSection”:”const-video-leaf”,”dateCreated”:”10:54 AM ET, Thu June 13, 2019″,”sourceName”:”CNN”,”sourceLink”:”https://www.cnn.com”,”videoCollectionUrl”:”/video/playlists/stories-worth-watching/”},”contentType”:”video”,”maximizedBanner”:false,”type”:”card”,”autoStartVideo”:false},{“branding”:””,”cardContents”:{“additionalSections”:[],”auxiliaryText”:””,”bannerText”:[],”bannerHasATag”:false,”bannerPosition”:””,”brandingLink”:””,”brandingImageUrl”:””,”brandingTextHead”:””,”brandingTextSub”:””,”cardSectionName”:”business”,”contentType”:””,”cta”:”share”,”descriptionText”:[“The new Bentley Flying Spur sedan looks like a limousine, but it can go over 200 miles per hour.”],”descriptionPlainText”:”The new Bentley Flying Spur sedan looks like a limousine, but it can go over 200 miles per hour.”,”headlinePostText”:””,”headlinePreText”:””,”headlineText”:”This Bentley could be the fastest sedan ever”,”headlinePlainText”:”This Bentley could be the fastest sedan ever”,”iconImageUrl”:””,”iconType”:”video”,”isMobileBannerText”:false,”kickerText”:””,”maximizedBannerSize”:[],”media”:{“contentType”:”image”,”type”:”element”,”cutFormat”:”16:9″,”elementContents”:{“caption”:””,”imageAlt”:””,”imageUrl”:”//cdn.cnn.com/cnnnext/dam/assets/190612142918-bentley-flying-spur-sedan-large-169.jpg”,”label”:””,”galleryTitle”:””,”head”:””,”source”:”Bentley”,”photographer”:””,”cuts”:{“mini”:{“width”:220,”type”:”jpg”,”uri”:”//cdn.cnn.com/cnnnext/dam/assets/190612142918-bentley-flying-spur-sedan-small-169.jpg”,”height”:124},”xsmall”:{“width”:307,”type”:”jpg”,”uri”:”//cdn.cnn.com/cnnnext/dam/assets/190612142918-bentley-flying-spur-sedan-medium-plus-169.jpg”,”height”:173},”small”:{“width”:460,”type”:”jpg”,”uri”:”//cdn.cnn.com/cnnnext/dam/assets/190612142918-bentley-flying-spur-sedan-large-169.jpg”,”height”:259},”medium”:{“width”:780,”type”:”jpg”,”uri”:”//cdn.cnn.com/cnnnext/dam/assets/190612142918-bentley-flying-spur-sedan-exlarge-169.jpg”,”height”:438},”large”:{“width”:1100,”type”:”jpg”,”uri”:”//cdn.cnn.com/cnnnext/dam/assets/190612142918-bentley-flying-spur-sedan-super-169.jpg”,”height”:619},”full16x9″:{“width”:1600,”type”:”jpg”,”uri”:”//cdn.cnn.com/cnnnext/dam/assets/190612142918-bentley-flying-spur-sedan-full-169.jpg”,”height”:900},”mini1x1″:{“width”:120,”type”:”jpg”,”uri”:”//cdn.cnn.com/cnnnext/dam/assets/190612142918-bentley-flying-spur-sedan-small-11.jpg”,”height”:120}},”responsiveImage”:true,”originalImageUrl”:”//cdn.cnn.com/cnnnext/dam/assets/190612142918-bentley-flying-spur-sedan.jpg”},”duration”:”0:52″},”noFollow”:false,”overMediaText”:””,”sectionUri”:””,”showSocialSharebar”:false,”shortUrl”:””,”statusText”:””,”statusColor”:””,”targetType”:””,”timestampDisplay”:””,”timestampUtc”:””,”lastModifiedText”:””,”lastModifiedState”:””,”type”:”card”,”url”:”/videos/business/2019/06/12/bentley-flying-spur-sedan-orig.cnn-business/video/playlists/stories-worth-watching/”,”width”:””,”webDisplayName”:”Business”,”height”:””,”videoCMSUri”:”/video/data/3.0/video/business/2019/06/12/bentley-flying-spur-sedan-orig.cnn-business/index.xml”,”videoId”:”business/2019/06/12/bentley-flying-spur-sedan-orig.cnn-business”,”adSection”:”const-video-leaf”,”dateCreated”:”2:25 PM ET, Wed June 12, 2019″,”sourceName”:”CNN Business”,”sourceLink”:””,”videoCollectionUrl”:”/video/playlists/stories-worth-watching/”},”contentType”:”video”,”maximizedBanner”:false,”type”:”card”,”autoStartVideo”:false},{“branding”:””,”cardContents”:{“additionalSections”:[],”auxiliaryText”:””,”bannerText”:[],”bannerHasATag”:false,”bannerPosition”:””,”brandingLink”:””,”brandingImageUrl”:””,”brandingTextHead”:””,”brandingTextSub”:””,”cardSectionName”:”business”,”contentType”:””,”cta”:”share”,”descriptionText”:[“In an exclusive interview at a data center in Oklahoma, Google CEO Sundar Pichai tells CNN’s Poppy Harlow why the company is investing in jobs around the country, and why tech may have to pump the brakes at times in order to best serve humanity.”],”descriptionPlainText”:”In an exclusive interview at a data center in Oklahoma, Google CEO Sundar Pichai tells CNN’s Poppy Harlow why the company is investing in jobs around the country, and why tech may have to pump the brakes at times in order to best serve humanity.”,”headlinePostText”:””,”headlinePreText”:””,”headlineText”:”Google CEO: We may have to slow down some ‘disruptive’ technology”,”headlinePlainText”:”Google CEO: We may have to slow down some ‘disruptive’ technology”,”iconImageUrl”:””,”iconType”:”video”,”isMobileBannerText”:false,”kickerText”:””,”maximizedBannerSize”:[],”media”:{“contentType”:”image”,”type”:”element”,”cutFormat”:”16:9″,”elementContents”:{“caption”:”Google CEO Sundar Pichai (C) speaks with guests during an event the Mayes County Google Data Center in Pryor, Oklahoma, June 13, 2019. Nick Oxford for CNN”,”imageAlt”:”Google CEO Sundar Pichai (C) speaks with guests during an event the Mayes County Google Data Center in Pryor, Oklahoma, June 13, 2019. Nick Oxford for CNN”,”imageUrl”:”//cdn.cnn.com/cnnnext/dam/assets/190613182415-04-google-oklahoma-large-169.jpg”,”label”:””,”galleryTitle”:””,”head”:””,”source”:”Nick Oxford for CNN”,”photographer”:”Nick Oxford for CNN”,”cuts”:{“mini”:{“width”:220,”type”:”jpg”,”uri”:”//cdn.cnn.com/cnnnext/dam/assets/190613182415-04-google-oklahoma-small-169.jpg”,”height”:124},”xsmall”:{“width”:307,”type”:”jpg”,”uri”:”//cdn.cnn.com/cnnnext/dam/assets/190613182415-04-google-oklahoma-medium-plus-169.jpg”,”height”:173},”small”:{“width”:460,”type”:”jpg”,”uri”:”//cdn.cnn.com/cnnnext/dam/assets/190613182415-04-google-oklahoma-large-169.jpg”,”height”:259},”medium”:{“width”:780,”type”:”jpg”,”uri”:”//cdn.cnn.com/cnnnext/dam/assets/190613182415-04-google-oklahoma-exlarge-169.jpg”,”height”:438},”large”:{“width”:1100,”type”:”jpg”,”uri”:”//cdn.cnn.com/cnnnext/dam/assets/190613182415-04-google-oklahoma-super-169.jpg”,”height”:619},”full16x9″:{“width”:1600,”type”:”jpg”,”uri”:”//cdn.cnn.com/cnnnext/dam/assets/190613182415-04-google-oklahoma-full-169.jpg”,”height”:900},”mini1x1″:{“width”:120,”type”:”jpg”,”uri”:”//cdn.cnn.com/cnnnext/dam/assets/190613182415-04-google-oklahoma-small-11.jpg”,”height”:120}},”responsiveImage”:true,”originalImageUrl”:”//cdn.cnn.com/cnnnext/dam/assets/190613182415-04-google-oklahoma.jpg”},”duration”:”3:47″},”noFollow”:false,”overMediaText”:””,”sectionUri”:””,”showSocialSharebar”:false,”shortUrl”:””,”statusText”:””,”statusColor”:””,”targetType”:””,”timestampDisplay”:””,”timestampUtc”:””,”lastModifiedText”:””,”lastModifiedState”:””,”type”:”card”,”url”:”/videos/business/2019/06/14/google-ceo-sundar-pichai-poppy-harlow-zw-orig.cnn/video/playlists/stories-worth-watching/”,”width”:””,”webDisplayName”:”Business”,”height”:””,”videoCMSUri”:”/video/data/3.0/video/business/2019/06/14/google-ceo-sundar-pichai-poppy-harlow-zw-orig.cnn/index.xml”,”videoId”:”business/2019/06/14/google-ceo-sundar-pichai-poppy-harlow-zw-orig.cnn”,”adSection”:”const-video-leaf”,”dateCreated”:”12:16 AM ET, Fri June 14, 2019″,”sourceName”:”CNN”,”sourceLink”:””,”videoCollectionUrl”:”/video/playlists/stories-worth-watching/”},”contentType”:”video”,”maximizedBanner”:false,”type”:”card”,”autoStartVideo”:false},{“branding”:””,”cardContents”:{“additionalSections”:[],”auxiliaryText”:””,”bannerText”:[],”bannerHasATag”:false,”bannerPosition”:””,”brandingLink”:””,”brandingImageUrl”:””,”brandingTextHead”:””,”brandingTextSub”:””,”cardSectionName”:”business”,”contentType”:””,”cta”:”share”,”descriptionText”:[“u003ca href=”https://cnn.it/2WBtomt; target=”_blank”>Queen Elsa and Princess Anna are back in the trailer for “Frozen IIu003c/a>,” which will likely mean more u003ca href=”https://cnn.it/2WzWkpV; target=”_blank”>huge box office and merchandising returns for Disneyu003c/a>.”],”descriptionPlainText”:”Queen Elsa and Princess Anna are back in the trailer for “Frozen II,” which will likely mean more huge box office and merchandising returns for Disney.”,”headlinePostText”:””,”headlinePreText”:””,”headlineText”:”Disney’s mega-hit is back with a new trailer”,”headlinePlainText”:”Disney’s mega-hit is back with a new trailer”,”iconImageUrl”:””,”iconType”:”video”,”isMobileBannerText”:false,”kickerText”:””,”maximizedBannerSize”:[],”media”:{“contentType”:”image”,”type”:”element”,”cutFormat”:”16:9″,”elementContents”:{“caption”:””,”imageAlt”:””,”imageUrl”:”//cdn.cnn.com/cnnnext/dam/assets/190213104122-02-frozen-2-large-169.jpg”,”label”:””,”galleryTitle”:””,”head”:””,”source”:”2019 Disney”,”photographer”:”2019 Disney”,”cuts”:{“mini”:{“width”:220,”type”:”jpg”,”uri”:”//cdn.cnn.com/cnnnext/dam/assets/190213104122-02-frozen-2-small-169.jpg”,”height”:124},”xsmall”:{“width”:307,”type”:”jpg”,”uri”:”//cdn.cnn.com/cnnnext/dam/assets/190213104122-02-frozen-2-medium-plus-169.jpg”,”height”:173},”small”:{“width”:460,”type”:”jpg”,”uri”:”//cdn.cnn.com/cnnnext/dam/assets/190213104122-02-frozen-2-large-169.jpg”,”height”:259},”medium”:{“width”:780,”type”:”jpg”,”uri”:”//cdn.cnn.com/cnnnext/dam/assets/190213104122-02-frozen-2-exlarge-169.jpg”,”height”:438},”large”:{“width”:1100,”type”:”jpg”,”uri”:”//cdn.cnn.com/cnnnext/dam/assets/190213104122-02-frozen-2-super-169.jpg”,”height”:619},”full16x9″:{“width”:1600,”type”:”jpg”,”uri”:”//cdn.cnn.com/cnnnext/dam/assets/190213104122-02-frozen-2-full-169.jpg”,”height”:900},”mini1x1″:{“width”:120,”type”:”jpg”,”uri”:”//cdn.cnn.com/cnnnext/dam/assets/190213104122-02-frozen-2-small-11.jpg”,”height”:120}},”responsiveImage”:true,”originalImageUrl”:”//cdn.cnn.com/cnnnext/dam/assets/190213104122-02-frozen-2.jpg”},”duration”:”1:34″},”noFollow”:false,”overMediaText”:””,”sectionUri”:””,”showSocialSharebar”:false,”shortUrl”:””,”statusText”:””,”statusColor”:””,”targetType”:””,”timestampDisplay”:””,”timestampUtc”:””,”lastModifiedText”:””,”lastModifiedState”:””,”type”:”card”,”url”:”/videos/business/2019/02/13/frozen-ii-trailer-disney-business-orig.cnn-business/video/playlists/stories-worth-watching/”,”width”:””,”webDisplayName”:”Business”,”height”:””,”videoCMSUri”:”/video/data/3.0/video/business/2019/02/13/frozen-ii-trailer-disney-business-orig.cnn-business/index.xml”,”videoId”:”business/2019/02/13/frozen-ii-trailer-disney-business-orig.cnn-business”,”adSection”:”const-video-leaf”,”dateCreated”:”11:45 AM ET, Wed February 13, 2019″,”sourceName”:”CNN Business”,”sourceLink”:”http://www.cnn.com/business”,”videoCollectionUrl”:”/video/playlists/stories-worth-watching/”},”contentType”:”video”,”maximizedBanner”:false,”type”:”card”,”autoStartVideo”:false},{“branding”:””,”cardContents”:{“additionalSections”:[],”auxiliaryText”:””,”bannerText”:[],”bannerHasATag”:false,”bannerPosition”:””,”brandingLink”:””,”brandingImageUrl”:””,”brandingTextHead”:””,”brandingTextSub”:””,”cardSectionName”:”business”,”contentType”:””,”cta”:”share”,”descriptionText”:[“Kent International CEO Arnold Kamler tells CNN’s Clare Sebastian how trade war and tariffs have impacted his business and made things more difficult for him.”],”descriptionPlainText”:”Kent International CEO Arnold Kamler tells CNN’s Clare Sebastian how trade war and tariffs have impacted his business and made things more difficult for him.”,”headlinePostText”:””,”headlinePreText”:””,”headlineText”:”CEO: Tariffs turn up pressure on my business”,”headlinePlainText”:”CEO: Tariffs turn up pressure on my business”,”iconImageUrl”:””,”iconType”:”video”,”isMobileBannerText”:false,”kickerText”:””,”maximizedBannerSize”:[],”media”:{“contentType”:”image”,”type”:”element”,”cutFormat”:”16:9″,”elementContents”:{“caption”:””,”imageAlt”:””,”imageUrl”:”//cdn.cnn.com/cnnnext/dam/assets/190614105506-kent-ceo-arnold-kamler-large-169.jpg”,”label”:””,”galleryTitle”:””,”head”:””,”source”:”CNN”,”photographer”:”CNN”,”cuts”:{“mini”:{“width”:220,”type”:”jpg”,”uri”:”//cdn.cnn.com/cnnnext/dam/assets/190614105506-kent-ceo-arnold-kamler-small-169.jpg”,”height”:124},”xsmall”:{“width”:307,”type”:”jpg”,”uri”:”//cdn.cnn.com/cnnnext/dam/assets/190614105506-kent-ceo-arnold-kamler-medium-plus-169.jpg”,”height”:173},”small”:{“width”:460,”type”:”jpg”,”uri”:”//cdn.cnn.com/cnnnext/dam/assets/190614105506-kent-ceo-arnold-kamler-large-169.jpg”,”height”:259},”medium”:{“width”:780,”type”:”jpg”,”uri”:”//cdn.cnn.com/cnnnext/dam/assets/190614105506-kent-ceo-arnold-kamler-exlarge-169.jpg”,”height”:438},”large”:{“width”:1100,”type”:”jpg”,”uri”:”//cdn.cnn.com/cnnnext/dam/assets/190614105506-kent-ceo-arnold-kamler-super-169.jpg”,”height”:619},”full16x9″:{“width”:1600,”type”:”jpg”,”uri”:”//cdn.cnn.com/cnnnext/dam/assets/190614105506-kent-ceo-arnold-kamler-full-169.jpg”,”height”:900},”mini1x1″:{“width”:120,”type”:”jpg”,”uri”:”//cdn.cnn.com/cnnnext/dam/assets/190614105506-kent-ceo-arnold-kamler-small-11.jpg”,”height”:120}},”responsiveImage”:true,”originalImageUrl”:”//cdn.cnn.com/cnnnext/dam/assets/190614105506-kent-ceo-arnold-kamler.jpg”},”duration”:”1:25″},”noFollow”:false,”overMediaText”:””,”sectionUri”:””,”showSocialSharebar”:false,”shortUrl”:””,”statusText”:””,”statusColor”:””,”targetType”:””,”timestampDisplay”:””,”timestampUtc”:””,”lastModifiedText”:””,”lastModifiedState”:””,”type”:”card”,”url”:”/videos/business/2019/06/14/tariffs-companies-warn-about-impact-sebastian-first-move-sot.cnn/video/playlists/stories-worth-watching/”,”width”:””,”webDisplayName”:”Business”,”height”:””,”videoCMSUri”:”/video/data/3.0/video/business/2019/06/14/tariffs-companies-warn-about-impact-sebastian-first-move-sot.cnn/index.xml”,”videoId”:”business/2019/06/14/tariffs-companies-warn-about-impact-sebastian-first-move-sot.cnn”,”adSection”:”const-video-leaf”,”dateCreated”:”10:49 AM ET, Fri June 14, 2019″,”sourceName”:”CNN”,”sourceLink”:”http:/www.cnn.com/”,”videoCollectionUrl”:”/video/playlists/stories-worth-watching/”},”contentType”:”video”,”maximizedBanner”:false,”type”:”card”,”autoStartVideo”:false},{“branding”:””,”cardContents”:{“additionalSections”:[],”auxiliaryText”:””,”bannerText”:[],”bannerHasATag”:false,”bannerPosition”:””,”brandingLink”:””,”brandingImageUrl”:””,”brandingTextHead”:””,”brandingTextSub”:””,”cardSectionName”:”business”,”contentType”:””,”cta”:”share”,”descriptionText”:[“Kraft says its new dressing will encourage kids to eat their veggies. Of course, the dressing itself is far from healthy.”],”descriptionPlainText”:”Kraft says its new dressing will encourage kids to eat their veggies. Of course, the dressing itself is far from healthy.”,”headlinePostText”:””,”headlinePreText”:””,”headlineText”:”Kraft wants to feed kids ‘Salad Frosting'”,”headlinePlainText”:”Kraft wants to feed kids ‘Salad Frosting'”,”iconImageUrl”:””,”iconType”:”video”,”isMobileBannerText”:false,”kickerText”:””,”maximizedBannerSize”:[],”media”:{“contentType”:”image”,”type”:”element”,”cutFormat”:”16:9″,”elementContents”:{“caption”:””,”imageAlt”:””,”imageUrl”:”//cdn.cnn.com/cnnnext/dam/assets/190610160046-kraft-salad-frosting-large-169.jpg”,”label”:””,”galleryTitle”:””,”head”:””,”source”:”Getty Images”,”photographer”:”Flashpop/Digital Vision/Getty Images”,”cuts”:{“mini”:{“width”:220,”type”:”jpg”,”uri”:”//cdn.cnn.com/cnnnext/dam/assets/190610160046-kraft-salad-frosting-small-169.jpg”,”height”:124},”xsmall”:{“width”:307,”type”:”jpg”,”uri”:”//cdn.cnn.com/cnnnext/dam/assets/190610160046-kraft-salad-frosting-medium-plus-169.jpg”,”height”:173},”small”:{“width”:460,”type”:”jpg”,”uri”:”//cdn.cnn.com/cnnnext/dam/assets/190610160046-kraft-salad-frosting-large-169.jpg”,”height”:259},”medium”:{“width”:780,”type”:”jpg”,”uri”:”//cdn.cnn.com/cnnnext/dam/assets/190610160046-kraft-salad-frosting-exlarge-169.jpg”,”height”:438},”large”:{“width”:1100,”type”:”jpg”,”uri”:”//cdn.cnn.com/cnnnext/dam/assets/190610160046-kraft-salad-frosting-super-169.jpg”,”height”:619},”full16x9″:{“width”:1600,”type”:”jpg”,”uri”:”//cdn.cnn.com/cnnnext/dam/assets/190610160046-kraft-salad-frosting-full-169.jpg”,”height”:900},”mini1x1″:{“width”:120,”type”:”jpg”,”uri”:”//cdn.cnn.com/cnnnext/dam/assets/190610160046-kraft-salad-frosting-small-11.jpg”,”height”:120}},”responsiveImage”:true,”originalImageUrl”:”//cdn.cnn.com/cnnnext/dam/assets/190610160046-kraft-salad-frosting.jpg”},”duration”:”0:46″},”noFollow”:false,”overMediaText”:””,”sectionUri”:””,”showSocialSharebar”:false,”shortUrl”:””,”statusText”:””,”statusColor”:””,”targetType”:””,”timestampDisplay”:””,”timestampUtc”:””,”lastModifiedText”:””,”lastModifiedState”:””,”type”:”card”,”url”:”/videos/business/2019/06/11/kraft-ranch-salad-frosting.cnn-business/video/playlists/stories-worth-watching/”,”width”:””,”webDisplayName”:””,”height”:””,”videoCMSUri”:”/video/data/3.0/video/business/2019/06/11/kraft-ranch-salad-frosting.cnn-business/index.xml”,”videoId”:”business/2019/06/11/kraft-ranch-salad-frosting.cnn-business”,”adSection”:”const-video-leaf”,”dateCreated”:”5:42 PM ET, Tue June 11, 2019″,”sourceName”:”CNN Business”,”sourceLink”:””,”videoCollectionUrl”:”/video/playlists/stories-worth-watching/”},”contentType”:”video”,”maximizedBanner”:false,”type”:”card”,”autoStartVideo”:false}],cardContents,i;for (i = 0; i 0) {for (i = 0; i 0) {for (i = 0; i 0) {for (i = 0; i 0) {currentVidObj = currentVideoCollection[getNextVideoIndex(currentVideoId)];nextPlay = currentVidObj.videoId;nextVideoUrl = domain + currentVidObj.videoUrl;if (nextPlay === undefined || nextPlay === null) {nextPlay = currentVideoCollection[0].videoId;}moveToNextTimeout = setTimeout(function () {overrides = {videoCollection: currentVideoCollection,autostart: true};if (CNN.VideoPlayer.getLibraryName(configObj.markupId) === ‘fave’) {FAVE.player.getInstance(configObj.markupId).play(nextPlay, overrides);} else {CNNVIDEOAPI.CNNVideoManager.getInstance().playVideo(configObj.markupId, nextPlay, overrides);}if (typeof window.recallProximic !== ‘undefined’ && nextPlay !== null) {window.recallProximic(nextVideoUrl);}}, nextVideoPlayTimeout);}}var decorateVideoApi = function(){CNN.VideoPlayer.showSpinner = function showSpinner(containerId) {if (Modernizr && !Modernizr.phone && !Modernizr.mobile && !Modernizr.tablet) {jQuery(document.getElementById((‘spinner_’ + containerId).replace(‘#’, ”))).show();}};CNN.VideoPlayer.hideSpinner = function hideSpinner(containerId) {if (Modernizr && !Modernizr.phone && !Modernizr.mobile && !Modernizr.tablet) {jQuery(document.getElementById((‘spinner_’ + containerId).replace(‘#’, ”))).hide();}};CNN.VideoPlayer.hideThumbnail = function hideThumbnail(containerId) {if (Modernizr && !Modernizr.phone && !Modernizr.mobile && !Modernizr.tablet) {jQuery(document.getElementById(containerId + ‘–thumbnail’)).hide();}};};callbackObj = {onPlayerReady: function (containerId) {CNN.INJECTOR.getNameSpaceFeature(‘CNN.VideoPlayer.showSpinner’).fail(decorateVideoApi);var containerClassId;CNN.VideoPlayer.handleAdOnCVPVisibilityChange(containerId, CNN.pageVis.isDocumentVisible());if (Modernizr && !Modernizr.phone && !Modernizr.mobile && !Modernizr.tablet) {containerClassId = ‘#’ + containerId;if (jQuery(containerClassId).parents(‘.js-pg-rail-tall__head’).length > 0) {videoPinner = new CNN.VideoPinner(containerClassId);videoPinner.setIsVideoCollection(true);videoPinner.init();} else {CNN.VideoPlayer.hideThumbnail(containerId);}}},onContentEntryLoad: function(containerId, playerId, contentid, isQueue) {CNN.VideoPlayer.showSpinner(containerId);CNN.VideoPlayer.isFirstVideoInCollection(containerId, contentid);},onAdPlay: function (containerId, cvpId, token, mode, id, duration, blockId, adType) {clearTimeout(moveToNextTimeout);CNN.VideoPlayer.hideSpinner(containerId);if (Modernizr && !Modernizr.phone && !Modernizr.mobile && !Modernizr.tablet) {if (typeof videoPinner !== ‘undefined’ && videoPinner !== null) {videoPinner.setIsPlaying(true);videoPinner.handleOnVideoPlay();videoPinner.animateDown();}}},onTrackingFullscreen: function (containerId, PlayerId, dataObj) {CNN.VideoPlayer.handleFullscreenChange(containerId, dataObj);},onContentPlay: function (containerId, cvpId, contentId) {if (CNN.companion && typeof CNN.companion.updateCompanionLayout === ‘function’) {CNN.companion.updateCompanionLayout(‘removeFreewheel’);CNN.companion.updateCompanionLayout(‘restoreEpicAds’);}clearTimeout(moveToNextTimeout);CNN.VideoPlayer.hideSpinner(containerId);if (Modernizr && !Modernizr.phone && !Modernizr.mobile && !Modernizr.tablet) {if (typeof videoPinner !== ‘undefined’ && videoPinner !== null) {videoPinner.setIsPlaying(true);videoPinner.handleOnVideoPlay();videoPinner.animateDown();}}},onContentReplayRequest: function (containerId, cvpId, contentId) {if (Modernizr && !Modernizr.phone && !Modernizr.mobile && !Modernizr.tablet) {if (typeof videoPinner !== ‘undefined’ && videoPinner !== null) {videoPinner.setIsPlaying(true);var $endSlate = jQuery(document.getElementById(containerId)).parent().find(‘.js-video__end-slate’).eq(0);if ($endSlate.length > 0) {$endSlate.removeClass(‘video__end-slate–active’).addClass(‘video__end-slate–inactive’);}}}},onContentMetadata: function (containerId, playerId, metadata, contentId, duration, width, height) {if (CNN.Utils.exists(metadata)) {try {if (CNN.VideoPlayer.getLibraryName(containerId) === ‘fave’) {CNN.Videx.EmbedButton.updateCode(metadata);} else {CNN.Videx.EmbedButton.updateCode(JSON.parse(metadata));}} catch (e) {console.log(‘Invalid video metadata JSON.’);}}},onContentBegin: function (containerId, cvpId, contentId) {CNN.VideoPlayer.reverseAutoMute(containerId);CNN.VideoPlayer.isFirstVideoInCollection(containerId, contentId);if (CNN.companion && typeof CNN.companion.updateCompanionLayout === ‘function’) {CNN.companion.updateCompanionLayout(‘removeEpicAds’);CNN.companion.updateCompanionLayout(‘restoreFreewheel’);}clearTimeout(moveToNextTimeout);fastdom.mutate(function () {if (CNN.share) {CNN.share.reloadShareBar();}});updateCurrentlyPlaying(contentId);jQuery(document).triggerVideoContentStarted();},onContentComplete: function (containerId, cvpId, contentId) {navigateToNextVideo(contentId);},onContentEnd: function (containerId, cvpId, contentId) {if (CNN.companion && typeof CNN.companion.updateCompanionLayout === ‘function’) {CNN.companion.updateCompanionLayout(‘removeEpicAds’);CNN.companion.updateCompanionLayout(��restoreFreewheel’);}if (Modernizr && !Modernizr.phone && !Modernizr.mobile && !Modernizr.tablet) {if (typeof videoPinner !== ‘undefined’ && videoPinner !== null) {videoPinner.setIsPlaying(false);}}},onCVPVisibilityChange: function (containerId, cvpId, visible) {CNN.VideoPlayer.handleAdOnCVPVisibilityChange(containerId, visible);}};mediaMetadataCallbacks = {nextTrack: function (containerId, playerId, contentId) {navigateToNextVideo(contentId);}};if (typeof configObj.context !== ‘string’ || configObj.context.length
Stories worth watching (15 Videos)
The post Trump voter in immigration dilemma: We lied to our son – CNN Video appeared first on Gyrlversion.
from WordPress http://www.gyrlversion.net/trump-voter-in-immigration-dilemma-we-lied-to-our-son-cnn-video/
0 notes
Text
How to Use Test-Time Augmentation to Improve Model Performance for Image Classification
Data augmentation is a technique often used to improve performance and reduce generalization error when training neural network models for computer vision problems.
The image data augmentation technique can also be applied when making predictions with a fit model in order to allow the model to make predictions for multiple different versions of each image in the test dataset. The predictions on the augmented images can be averaged, which can result in better predictive performance.
In this tutorial, you will discover test-time augmentation for improving the performance of models for image classification tasks.
After completing this tutorial, you will know:
Test-time augmentation is the application of data augmentation techniques normally used during training when making predictions.
How to implement test-time augmentation from scratch in Keras.
How to use test-time augmentation to improve the performance of a convolutional neural network model on a standard image classification task.
Let’s get started.
How to Use Test-Time Augmentation to Improve Model Performance for Image Classification Photo by daveynin, some rights reserved.
Tutorial Overview
This tutorial is divided into five parts; they are:
Test-Time Augmentation
Test-Time Augmentation in Keras
Dataset and Baseline Model
Example of Test-Time Augmentation
How to Tune Test-Time Augmentation Configuration
Test-Time Augmentation
Data augmentation is an approach typically used during the training of the model that expands the training set with modified copies of samples from the training dataset.
Data augmentation is often performed with image data, where copies of images in the training dataset are created with some image manipulation techniques performed, such as zooms, flips, shifts, and more.
The artificially expanded training dataset can result in a more skillful model, as often the performance of deep learning models continues to scale in concert with the size of the training dataset. In addition, the modified or augmented versions of the images in the training dataset assist the model in extracting and learning features in a way that is invariant to their position, lighting, and more.
Test-time augmentation, or TTA for short, is an application of data augmentation to the test dataset.
Specifically, it involves creating multiple augmented copies of each image in the test set, having the model make a prediction for each, then returning an ensemble of those predictions.
Augmentations are chosen to give the model the best opportunity for correctly classifying a given image, and the number of copies of an image for which a model must make a prediction is often small, such as less than 10 or 20.
Often, a single simple test-time augmentation is performed, such as a shift, crop, or image flip.
In their 2015 paper that achieved then state-of-the-art results on the ILSVRC dataset titled “Very Deep Convolutional Networks for Large-Scale Image Recognition,” the authors use horizontal flip test-time augmentation:
We also augment the test set by horizontal flipping of the images; the soft-max class posteriors of the original and flipped images are averaged to obtain the final scores for the image.
Similarly, in their 2015 paper on the inception architecture titled “Rethinking the Inception Architecture for Computer Vision,” the authors at Google use cropping test-time augmentation, which they refer to as multi-crop evaluation.
Want Results with Deep Learning for Computer Vision?
Take my free 7-day email crash course now (with sample code).
Click to sign-up and also get a free PDF Ebook version of the course.
Download Your FREE Mini-Course
Test-Time Augmentation in Keras
Test-time augmentation is not provided natively in the Keras deep learning library but can be implemented easily.
The ImageDataGenerator class can be used to configure the choice of test-time augmentation. For example, the data generator below is configured for horizontal flip image data augmentation.
# configure image data augmentation datagen = ImageDataGenerator(horizontal_flip=True)
The augmentation can then be applied to each sample in the test dataset separately.
First, the dimensions of the single image can be expanded from [rows][cols][channels] to [samples][rows][cols][channels], where the number of samples is one, for the single image. This transforms the array for the image into an array of samples with one image.
# convert image into dataset samples = expand_dims(image, 0)
Next, an iterator can be created for the sample, and the batch size can be used to specify the number of augmented images to generate, such as 10.
# prepare iterator it = datagen.flow(samples, batch_size=10)
The iterator can then be passed to the predict_generator() function of the model in order to make a prediction. Specifically, a batch of 10 augmented images will be generated and the model will make a prediction for each.
# make predictions for each augmented image yhats = model.predict_generator(it, steps=10, verbose=0)
Finally, an ensemble prediction can be made. A prediction was made for each image, and each prediction contains a probability of the image belonging to each class, in the case of image multiclass classification.
An ensemble prediction can be made using soft voting where the probabilities of each class are summed across the predictions and a class prediction is made by calculating the argmax() of the summed predictions, returning the index or class number of the largest summed probability.
# sum across predictions summed = numpy.sum(yhats, axis=0) # argmax across classes return argmax(summed)
We can tie these elements together into a function that will take a configured data generator, fit model, and single image, and will return a class prediction (integer) using test-time augmentation.
# make a prediction using test-time augmentation def tta_prediction(datagen, model, image, n_examples): # convert image into dataset samples = expand_dims(image, 0) # prepare iterator it = datagen.flow(samples, batch_size=n_examples) # make predictions for each augmented image yhats = model.predict_generator(it, steps=n_examples, verbose=0) # sum across predictions summed = numpy.sum(yhats, axis=0) # argmax across classes return argmax(summed)
Now that we know how to make predictions in Keras using test-time augmentation, let’s work through an example to demonstrate the approach.
Dataset and Baseline Model
We can demonstrate test-time augmentation using a standard computer vision dataset and a convolutional neural network.
Before we can do that, we must select a dataset and a baseline model.
We will use the CIFAR-10 dataset, comprised of 60,000 32×32 pixel color photographs of objects from 10 classes, such as frogs, birds, cats, ships, etc. CIFAR-10 is a well-understood dataset and widely used for benchmarking computer vision algorithms in the field of machine learning. The problem is “solved.” Top performance on the problem is achieved by deep learning convolutional neural networks with a classification accuracy above 96% or 97% on the test dataset.
We will also use a convolutional neural network, or CNN, model that is capable of achieving good (better than random) results, but not state-of-the-art results, on the problem. This will be sufficient to demonstrate the lift in performance that test-time augmentation can provide.
The CIFAR-10 dataset can be loaded easily via the Keras API by calling the cifar10.load_data() function, that returns a tuple with the training and test datasets split into input (images) and output (class labels) components.
# load dataset (trainX, trainY), (testX, testY) = load_data()
It is good practice to normalize the pixel values from the range 0-255 down to the range 0-1 prior to modeling. This ensures that the inputs are small and close to zero, and will, in turn, mean that the weights of the model will be kept small, leading to faster and better learning.
# normalize pixel values trainX = trainX.astype('float32') / 255 testX = testX.astype('float32') / 255
The class labels are integers and must be converted to a one hot encoding prior to modeling.
This can be achieved using the to_categorical() Keras utility function.
# one hot encode target values trainY = to_categorical(trainY) testY = to_categorical(testY)
We are now ready to define a model for this multi-class classification problem.
The model has a convolutional layer with 32 filter maps with a 3×3 kernel using the rectifier linear activation, “same” padding so the output is the same size as the input and the He weight initialization. This is followed by a batch normalization layer and a max pooling layer.
This pattern is repeated with a convolutional, batch norm, and max pooling layer, although the number of filters is increased to 64. The output is then flattened before being interpreted by a dense layer and finally provided to the output layer to make a prediction.
# define model model = Sequential() model.add(Conv2D(32, (3, 3), activation='relu', padding='same', kernel_initializer='he_uniform', input_shape=(32, 32, 3))) model.add(BatchNormalization()) model.add(MaxPooling2D((2, 2))) model.add(Conv2D(64, (3, 3), activation='relu', padding='same', kernel_initializer='he_uniform')) model.add(BatchNormalization()) model.add(MaxPooling2D((2, 2))) model.add(Flatten()) model.add(Dense(128, activation='relu', kernel_initializer='he_uniform')) model.add(BatchNormalization()) model.add(Dense(10, activation='softmax'))
The Adam variation of stochastic gradient descent is used to find the model weights.
The categorical cross entropy loss function is used, required for multi-class classification, and classification accuracy is monitored during training.
# compile model model.compile(optimizer='adam', loss='categorical_crossentropy', metrics=['accuracy'])
The model is fit for three training epochs and a large batch size of 128 images is used.
# fit model model.fit(trainX, trainY, epochs=3, batch_size=128)
Once fit, the model is evaluated on the test dataset.
# evaluate model _, acc = model.evaluate(testX, testY, verbose=0) print(acc)
The complete example is listed below and will easily run on the CPU in a few minutes.
# baseline cnn model for the cifar10 problem from keras.datasets.cifar10 import load_data from keras.utils import to_categorical from keras.models import Sequential from keras.layers import Conv2D from keras.layers import MaxPooling2D from keras.layers import Dense from keras.layers import Flatten from keras.layers import BatchNormalization # load dataset (trainX, trainY), (testX, testY) = load_data() # normalize pixel values trainX = trainX.astype('float32') / 255 testX = testX.astype('float32') / 255 # one hot encode target values trainY = to_categorical(trainY) testY = to_categorical(testY) # define model model = Sequential() model.add(Conv2D(32, (3, 3), activation='relu', padding='same', kernel_initializer='he_uniform', input_shape=(32, 32, 3))) model.add(BatchNormalization()) model.add(MaxPooling2D((2, 2))) model.add(Conv2D(64, (3, 3), activation='relu', padding='same', kernel_initializer='he_uniform')) model.add(BatchNormalization()) model.add(MaxPooling2D((2, 2))) model.add(Flatten()) model.add(Dense(128, activation='relu', kernel_initializer='he_uniform')) model.add(BatchNormalization()) model.add(Dense(10, activation='softmax')) # compile model model.compile(optimizer='adam', loss='categorical_crossentropy', metrics=['accuracy']) # fit model history = model.fit(trainX, trainY, epochs=3, batch_size=128) # evaluate model _, acc = model.evaluate(testX, testY, verbose=0) print(acc)
Running the example shows that the model is capable of learning the problem well and quickly.
A test set accuracy of about 66% is achieved, which is okay, but not terrific. The chosen model configuration has already started to overfit and could benefit from the use of regularization and further tuning. Nevertheless, this provides a good starting point for demonstrating test-time augmentation.
Epoch 1/3 50000/50000 [==============================] - 64s 1ms/step - loss: 1.2135 - acc: 0.5766 Epoch 2/3 50000/50000 [==============================] - 63s 1ms/step - loss: 0.8498 - acc: 0.7035 Epoch 3/3 50000/50000 [==============================] - 63s 1ms/step - loss: 0.6799 - acc: 0.7632 0.6679
Neural networks are stochastic algorithms and the same model fit on the same data multiple times may find a different set of weights and, in turn, have different performance each time.
In order to even out the estimate of model performance, we can change the example to re-run the fit and evaluation of the model multiple times and report the mean and standard deviation of the distribution of scores on the test dataset.
First, we can define a function named load_dataset() that will load the CIFAR-10 dataset and prepare it for modeling.
# load and return the cifar10 dataset ready for modeling def load_dataset(): # load dataset (trainX, trainY), (testX, testY) = load_data() # normalize pixel values trainX = trainX.astype('float32') / 255 testX = testX.astype('float32') / 255 # one hot encode target values trainY = to_categorical(trainY) testY = to_categorical(testY) return trainX, trainY, testX, testY
Next, we can define a function named define_model() that will define a model for the CIFAR-10 dataset, ready to be fit and then evaluated.
# define the cnn model for the cifar10 dataset def define_model(): # define model model = Sequential() model.add(Conv2D(32, (3, 3), activation='relu', padding='same', kernel_initializer='he_uniform', input_shape=(32, 32, 3))) model.add(BatchNormalization()) model.add(MaxPooling2D((2, 2))) model.add(Conv2D(64, (3, 3), activation='relu', padding='same', kernel_initializer='he_uniform')) model.add(BatchNormalization()) model.add(MaxPooling2D((2, 2))) model.add(Flatten()) model.add(Dense(128, activation='relu', kernel_initializer='he_uniform')) model.add(BatchNormalization()) model.add(Dense(10, activation='softmax')) # compile model model.compile(optimizer='adam', loss='categorical_crossentropy', metrics=['accuracy']) return model
Next, an evaluate_model() function is defined that will fit the defined model on the training dataset and then evaluate it on the test dataset, returning the estimated classification accuracy for the run.
# fit and evaluate a defined model def evaluate_model(model, trainX, trainY, testX, testY): # fit model model.fit(trainX, trainY, epochs=3, batch_size=128, verbose=0) # evaluate model _, acc = model.evaluate(testX, testY, verbose=0) return acc
Next, we can define a function with new behavior to repeatedly define, fit, and evaluate a new model and return the distribution of accuracy scores.
The repeated_evaluation() function below implements this, taking the dataset and using a default of 10 repeated evaluations.
# repeatedly evaluate model, return distribution of scores def repeated_evaluation(trainX, trainY, testX, testY, repeats=10): scores = list() for _ in range(repeats): # define model model = define_model() # fit and evaluate model accuracy = evaluate_model(model, trainX, trainY, testX, testY) # store score scores.append(accuracy) print('> %.3f' % accuracy) return scores
Finally, we can call the load_dataset() function to prepare the dataset, then repeated_evaluation() to get a distribution of accuracy scores that can be summarized by reporting the mean and standard deviation.
# load dataset trainX, trainY, testX, testY = load_dataset() # evaluate model scores = repeated_evaluation(trainX, trainY, testX, testY) # summarize result print('Accuracy: %.3f (%.3f)' % (mean(scores), std(scores)))
Tying all of this together, the complete code example of repeatedly evaluating a CNN model on the MNIST dataset is listed below.
# baseline cnn model for the cifar10 problem, repeated evaluation from numpy import mean from numpy import std from keras.datasets.cifar10 import load_data from keras.utils import to_categorical from keras.models import Sequential from keras.layers import Conv2D from keras.layers import MaxPooling2D from keras.layers import Dense from keras.layers import Flatten from keras.layers import BatchNormalization # load and return the cifar10 dataset ready for modeling def load_dataset(): # load dataset (trainX, trainY), (testX, testY) = load_data() # normalize pixel values trainX = trainX.astype('float32') / 255 testX = testX.astype('float32') / 255 # one hot encode target values trainY = to_categorical(trainY) testY = to_categorical(testY) return trainX, trainY, testX, testY # define the cnn model for the cifar10 dataset def define_model(): # define model model = Sequential() model.add(Conv2D(32, (3, 3), activation='relu', padding='same', kernel_initializer='he_uniform', input_shape=(32, 32, 3))) model.add(BatchNormalization()) model.add(MaxPooling2D((2, 2))) model.add(Conv2D(64, (3, 3), activation='relu', padding='same', kernel_initializer='he_uniform')) model.add(BatchNormalization()) model.add(MaxPooling2D((2, 2))) model.add(Flatten()) model.add(Dense(128, activation='relu', kernel_initializer='he_uniform')) model.add(BatchNormalization()) model.add(Dense(10, activation='softmax')) # compile model model.compile(optimizer='adam', loss='categorical_crossentropy', metrics=['accuracy']) return model # fit and evaluate a defined model def evaluate_model(model, trainX, trainY, testX, testY): # fit model model.fit(trainX, trainY, epochs=3, batch_size=128, verbose=0) # evaluate model _, acc = model.evaluate(testX, testY, verbose=0) return acc # repeatedly evaluate model, return distribution of scores def repeated_evaluation(trainX, trainY, testX, testY, repeats=10): scores = list() for _ in range(repeats): # define model model = define_model() # fit and evaluate model accuracy = evaluate_model(model, trainX, trainY, testX, testY) # store score scores.append(accuracy) print('> %.3f' % accuracy) return scores # load dataset trainX, trainY, testX, testY = load_dataset() # evaluate model scores = repeated_evaluation(trainX, trainY, testX, testY) # summarize result print('Accuracy: %.3f (%.3f)' % (mean(scores), std(scores)))
Running the example may take a while on modern CPU hardware and is much faster on GPU hardware.
The accuracy of the model is reported for each repeated evaluation and the final mean model performance is reported.
In this case, we can see that the mean accuracy of the chosen model configuration is about 68%, which is close to the estimate from a single model run.
> 0.690 > 0.662 > 0.698 > 0.681 > 0.686 > 0.680 > 0.697 > 0.696 > 0.689 > 0.679 Accuracy: 0.686 (0.010)
Now that we have developed a baseline model for a standard dataset, let’s look at updating the example to use test-time augmentation.
Example of Test-Time Augmentation
We can now update our repeated evaluation of the CNN model on CIFAR-10 to use test-time augmentation.
The tta_prediction() function developed in the section above on how to implement test-time augmentation in Keras can be used directly.
# make a prediction using test-time augmentation def tta_prediction(datagen, model, image, n_examples): # convert image into dataset samples = expand_dims(image, 0) # prepare iterator it = datagen.flow(samples, batch_size=n_examples) # make predictions for each augmented image yhats = model.predict_generator(it, steps=n_examples, verbose=0) # sum across predictions summed = numpy.sum(yhats, axis=0) # argmax across classes return argmax(summed)
We can develop a function that will drive the test-time augmentation by defining the ImageDataGenerator configuration and call tta_prediction() for each image in the test dataset.
It is important to consider the types of image augmentations that may benefit a model fit on the CIFAR-10 dataset. Augmentations that cause minor modifications to the photographs might be useful. This might include augmentations such as zooms, shifts, and horizontal flips.
In this example, we will only use horizontal flips.
# configure image data augmentation datagen = ImageDataGenerator(horizontal_flip=True)
We will configure the image generator to create seven photos, from which the mean prediction for each example in the test set will be made.
The tta_evaluate_model() function below configures the ImageDataGenerator then enumerates the test dataset, making a class label prediction for each image in the test dataset. The accuracy is then calculated by comparing the predicted class labels to the class labels in the test dataset. This requires that we reverse the one hot encoding performed in load_dataset() by using argmax().
# evaluate a model on a dataset using test-time augmentation def tta_evaluate_model(model, testX, testY): # configure image data augmentation datagen = ImageDataGenerator(horizontal_flip=True) # define the number of augmented images to generate per test set image n_examples_per_image = 7 yhats = list() for i in range(len(testX)): # make augmented prediction yhat = tta_prediction(datagen, model, testX[i], n_examples_per_image) # store for evaluation yhats.append(yhat) # calculate accuracy testY_labels = argmax(testY, axis=1) acc = accuracy_score(testY_labels, yhats) return acc
The evaluate_model() function can then be updated to call tta_evaluate_model() in order to get model accuracy scores.
# fit and evaluate a defined model def evaluate_model(model, trainX, trainY, testX, testY): # fit model model.fit(trainX, trainY, epochs=3, batch_size=128, verbose=0) # evaluate model using tta acc = tta_evaluate_model(model, testX, testY) return acc
Tying all of this together, the complete example of the repeated evaluation of a CNN for CIFAR-10 with test-time augmentation is listed below.
# cnn model for the cifar10 problem with test-time augmentation import numpy from numpy import argmax from numpy import mean from numpy import std from numpy import expand_dims from sklearn.metrics import accuracy_score from keras.datasets.cifar10 import load_data from keras.utils import to_categorical from keras.preprocessing.image import ImageDataGenerator from keras.models import Sequential from keras.layers import Conv2D from keras.layers import MaxPooling2D from keras.layers import Dense from keras.layers import Flatten from keras.layers import BatchNormalization # load and return the cifar10 dataset ready for modeling def load_dataset(): # load dataset (trainX, trainY), (testX, testY) = load_data() # normalize pixel values trainX = trainX.astype('float32') / 255 testX = testX.astype('float32') / 255 # one hot encode target values trainY = to_categorical(trainY) testY = to_categorical(testY) return trainX, trainY, testX, testY # define the cnn model for the cifar10 dataset def define_model(): # define model model = Sequential() model.add(Conv2D(32, (3, 3), activation='relu', padding='same', kernel_initializer='he_uniform', input_shape=(32, 32, 3))) model.add(BatchNormalization()) model.add(MaxPooling2D((2, 2))) model.add(Conv2D(64, (3, 3), activation='relu', padding='same', kernel_initializer='he_uniform')) model.add(BatchNormalization()) model.add(MaxPooling2D((2, 2))) model.add(Flatten()) model.add(Dense(128, activation='relu', kernel_initializer='he_uniform')) model.add(BatchNormalization()) model.add(Dense(10, activation='softmax')) # compile model model.compile(optimizer='adam', loss='categorical_crossentropy', metrics=['accuracy']) return model # make a prediction using test-time augmentation def tta_prediction(datagen, model, image, n_examples): # convert image into dataset samples = expand_dims(image, 0) # prepare iterator it = datagen.flow(samples, batch_size=n_examples) # make predictions for each augmented image yhats = model.predict_generator(it, steps=n_examples, verbose=0) # sum across predictions summed = numpy.sum(yhats, axis=0) # argmax across classes return argmax(summed) # evaluate a model on a dataset using test-time augmentation def tta_evaluate_model(model, testX, testY): # configure image data augmentation datagen = ImageDataGenerator(horizontal_flip=True) # define the number of augmented images to generate per test set image n_examples_per_image = 7 yhats = list() for i in range(len(testX)): # make augmented prediction yhat = tta_prediction(datagen, model, testX[i], n_examples_per_image) # store for evaluation yhats.append(yhat) # calculate accuracy testY_labels = argmax(testY, axis=1) acc = accuracy_score(testY_labels, yhats) return acc # fit and evaluate a defined model def evaluate_model(model, trainX, trainY, testX, testY): # fit model model.fit(trainX, trainY, epochs=3, batch_size=128, verbose=0) # evaluate model using tta acc = tta_evaluate_model(model, testX, testY) return acc # repeatedly evaluate model, return distribution of scores def repeated_evaluation(trainX, trainY, testX, testY, repeats=10): scores = list() for _ in range(repeats): # define model model = define_model() # fit and evaluate model accuracy = evaluate_model(model, trainX, trainY, testX, testY) # store score scores.append(accuracy) print('> %.3f' % accuracy) return scores # load dataset trainX, trainY, testX, testY = load_dataset() # evaluate model scores = repeated_evaluation(trainX, trainY, testX, testY) # summarize result print('Accuracy: %.3f (%.3f)' % (mean(scores), std(scores)))
Running the example may take some time given the repeated evaluation and the slower manual test-time augmentation used to evaluate each model.
In this case, we can see a modest lift in performance from about 68.6% on the test set without test-time augmentation to about 69.8% accuracy on the test set with test-time augmentation.
> 0.719 > 0.716 > 0.709 > 0.694 > 0.690 > 0.694 > 0.680 > 0.676 > 0.702 > 0.704 Accuracy: 0.698 (0.013)
How to Tune Test-Time Augmentation Configuration
Choosing the augmentation configurations that give the biggest lift in model performance can be challenging.
Not only are there many augmentation methods to choose from and configuration options for each, but the time to fit and evaluate a model on a single set of configuration options can take a long time, even if fit on a fast GPU.
Instead, I recommend fitting the model once and saving it to file. For example:
# save model model.save('model.h5')
Then load the model from a separate file and evaluate different test-time augmentation schemes on a small validation dataset or small subset of the test set.
For example:
... # load model model = load_model('model.h5') # evaluate model datagen = ImageDataGenerator(...) ...
Once you find a set of augmentation options that give the biggest lift, you can then evaluate the model on the whole test set or trial a repeated evaluation experiment as above.
Test-time augmentation configuration not only includes the options for the ImageDataGenerator, but also the number of images generated from which the average prediction will be made for each example in the test set.
I used this approach to choose the test-time augmentation in the previous section, discovering that seven examples worked better than three or five, and that random zooming and random shifts appeared to decrease model accuracy.
Remember, if you also use image data augmentation for the training dataset and that augmentation uses a type of pixel scaling that involves calculating statistics on the dataset (e.g. you call datagen.fit()), then those same statistics and pixel scaling techniques must also be used during test-time augmentation.
Further Reading
This section provides more resources on the topic if you are looking to go deeper.
API
Image Preprocessing Keras API.
Keras Sequential Model API.
numpy.argmax API
Articles
Image Segmentation With Test Time Augmentation With Keras
keras_tta, Simple test-time augmentation (TTA) for keras python library.
tta_wrapper, Test Time image Augmentation (TTA) wrapper for Keras model.
Summary
In this tutorial, you discovered test-time augmentation for improving the performance of models for image classification tasks.
Specifically, you learned:
Test-time augmentation is the application of data augmentation techniques normally used during training when making predictions.
How to implement test-time augmentation from scratch in Keras.
How to use test-time augmentation to improve the performance of a convolutional neural network model on a standard image classification task.
Do you have any questions? Ask your questions in the comments below and I will do my best to answer.
The post How to Use Test-Time Augmentation to Improve Model Performance for Image Classification appeared first on Machine Learning Mastery.
Machine Learning Mastery published first on Machine Learning Mastery
0 notes
Text
Kicking neural network automation into high gear
A new area in artificial intelligence involves using algorithms to automatically design machine-learning systems known as neural networks, which are more accurate and efficient than those developed by human engineers. But this so-called neural architecture search (NAS) technique is computationally expensive.
One of the state-of-the-art NAS algorithms recently developed by Google took 48,000 hours of work by a squad of graphical processing units (GPUs) to produce a single convolutional neural network, used for image classification and identification tasks. Google has the wherewithal to run hundreds of GPUs and other specialized circuits in parallel, but that’s out of reach for many others.
In a paper being presented at the International Conference on Learning Representations in May, MIT researchers describe an NAS algorithm that can directly learn specialized convolutional neural networks (CNNs) for target hardware platforms — when run on a massive image dataset — in only 200 GPU hours, which could enable far broader use of these types of algorithms.
Resource-strapped researchers and companies could benefit from the time- and cost-saving algorithm, the researchers say. The broad goal is “to democratize AI,” says co-author Song Han, an assistant professor of electrical engineering and computer science and a researcher in the Microsystems Technology Laboratories at MIT. “We want to enable both AI experts and nonexperts to efficiently design neural network architectures with a push-button solution that runs fast on a specific hardware.”
Han adds that such NAS algorithms will never replace human engineers. “The aim is to offload the repetitive and tedious work that comes with designing and refining neural network architectures,” says Han, who is joined on the paper by two researchers in his group, Han Cai and Ligeng Zhu.
“Path-level” binarization and pruning
In their work, the researchers developed ways to delete unnecessary neural network design components, to cut computing times and use only a fraction of hardware memory to run a NAS algorithm. An additional innovation ensures each outputted CNN runs more efficiently on specific hardware platforms — CPUs, GPUs, and mobile devices — than those designed by traditional approaches. In tests, the researchers’ CNNs were 1.8 times faster measured on a mobile phone than traditional gold-standard models with similar accuracy.
A CNN’s architecture consists of layers of computation with adjustable parameters, called “filters,” and the possible connections between those filters. Filters process image pixels in grids of squares — such as 3×3, 5×5, or 7×7 — with each filter covering one square. The filters essentially move across the image and combine all the colors of their covered grid of pixels into a single pixel. Different layers may have different-sized filters, and connect to share data in different ways. The output is a condensed image — from the combined information from all the filters — that can be more easily analyzed by a computer.
Because the number of possible architectures to choose from — called the “search space” — is so large, applying NAS to create a neural network on massive image datasets is computationally prohibitive. Engineers typically run NAS on smaller proxy datasets and transfer their learned CNN architectures to the target task. This generalization method reduces the model’s accuracy, however. Moreover, the same outputted architecture also is applied to all hardware platforms, which leads to efficiency issues.
The researchers trained and tested their new NAS algorithm on an image classification task in the ImageNet dataset, which contains millions of images in a thousand classes. They first created a search space that contains all possible candidate CNN “paths” — meaning how the layers and filters connect to process the data. This gives the NAS algorithm free reign to find an optimal architecture.
This would typically mean all possible paths must be stored in memory, which would exceed GPU memory limits. To address this, the researchers leverage a technique called “path-level binarization,” which stores only one sampled path at a time and saves an order of magnitude in memory consumption. They combine this binarization with “path-level pruning,” a technique that traditionally learns which “neurons” in a neural network can be deleted without affecting the output. Instead of discarding neurons, however, the researchers’ NAS algorithm prunes entire paths, which completely changes the neural network’s architecture.
In training, all paths are initially given the same probability for selection. The algorithm then traces the paths — storing only one at a time — to note the accuracy and loss (a numerical penalty assigned for incorrect predictions) of their outputs. It then adjusts the probabilities of the paths to optimize both accuracy and efficiency. In the end, the algorithm prunes away all the low-probability paths and keeps only the path with the highest probability — which is the final CNN architecture.
Hardware-aware
Another key innovation was making the NAS algorithm “hardware aware,” Han says, meaning it uses the latency on each hardware platform as a feedback signal to optimize the architecture. To measure this latency on mobile devices, for instance, big companies such as Google will employ a “farm” of mobile devices, which is very expensive. The researchers instead built a model that predicts the latency using only a single mobile phone.
For each chosen layer of the network, the algorithm samples the architecture on that latency-prediction model. It then uses that information to design an architecture that runs as quickly as possible, while achieving high accuracy. In experiments, the researchers’ CNN ran nearly twice as fast as a gold-standard model on mobile devices.
One interesting result, Han says, was that their NAS algorithm designed CNN architectures that were long dismissed as being too inefficient — but, in the researchers’ tests, they were actually optimized for certain hardware. For instance, engineers have essentially stopped using 7×7 filters, because they’re computationally more expensive than multiple, smaller filters. Yet, the researchers’ NAS algorithm found architectures with some layers of 7×7 filters ran optimally on GPUs. That’s because GPUs have high parallelization — meaning they compute many calculations simultaneously — so can process a single large filter at once more efficiently than processing multiple small filters one at a time.
“This goes against previous human thinking,” Han says. “The larger the search space, the more unknown things you can find. You don’t know if something will be better than the past human experience. Let the AI figure it out.”
The work was supported, in part, by the MIT Quest for Intelligence, the MIT-IBM Watson AI lab, and SenseTime.
Kicking neural network automation into high gear syndicated from https://osmowaterfilters.blogspot.com/
0 notes
Photo
"[D] Eat Your VGGtables, or, Why Does Neural Style Transfer Work Best With Old VGG CNNs' Features?"- Detail: Previous: Twitter discussion.An acquaintance a year or two ago was messing around with neural style transfer (Gatys et al 2016), experimenting with some different approaches, like a tile-based GPU implementation for making large poster-size transfers, or optimizing images to look different using a two-part loss: one to encourage being like the style of the style image, and a negative one to penalize having content like the source image; this is unstable and can diverge, but when it works, looks cool. (Example: "The Great Wave" + Golden Gate Bridge. I tried further Klimt-ising it but at that point too much has been lost.)VGG worked best for style transferOne thing they noticed was that using features from a pretrained ImageNet VGG-16/19 CNN from 2014 (4 years ago), like the original Gatys paper did, worked much better than anything else; indeed, almost any set of 4-5 layers in VGG would provide great features for the style transfer optimization to target (as long as they were spread out and weren't exclusively bottom or top layers), while using more modern resnets (resnet-50) or GoogLeNet Inception v1 didn't work - it was hard to find sets of layers that would work at all and when they did, the quality of the style transfer was not as good. Interestingly, this appeared to be true of VGG CNNs trained on the MIT Places scene recognition database too, suggesting there's something architectural going on which is not database specific or peculiar to those two trained models. And their attempt at an upscaling CNN modeled on Johnson et al 2016's VGG-16 for CIFAR-100 worked well too.Everyone uses VGGIndeed, VGG is used pervasively through style transfer implementations & research beyond what one would expect from cargo-culting or copy-paste, even in applications as exotic as inferring images from human fMRI scans (Shen et al 2017). This surprising because 4 years in DL is a long time, and the newer CNNs outperform VGG at everything else like image classification or object localization (Tapa Ghosh disagrees on object localization) rendering VGG obsolete due to its large model size (much of which comes from the 3 large fully-connected layers at the top) & slowness & poor accuracy, and style transfer itself has made major advances in, among other things, going from days on a desktop to generate a new image to being capable of realtime on smartphones. For example, SqueezeNet outperforms VGG in every way, but its style transfer results are distinctly worse (but extremely fast!). Although this VGG-specificity appears to be folklore among practitioners, this is not something I have seen noticed in neural style transfer papers; indeed, the review Jing et al 2017 explicitly says that other models work fine, but their reference is to Johnson's list of models where almost every single model is (still) VGG-based and the ones which are not come with warnings (NIN-Imagenet: "May need heavy tweaking to achieve reasonable results"; Illustration2vec: "Best used with anime content...Be warned that it can sometimes be difficult to avoid the burn marks that the model sometimes creates"; PASCAL VOC FCN-32s: "Uses more resources than VGG-19, but can produce better results depending on your style and/or content image." etc).HypothesesSome possible explanations:VGG is so big that it is incidentally capturing a lot of information that the other models discard and accidentally generalizing better despite worse task-specific performance. (Do resnets in general do transfer-learning worse, compared to earlier CNNs, than would be expected based on their superior task-specific performance?)but while VGG is giant compared to other ImageNet models, 500M vs <50MB (Keras table), most of this appears to be coming from the FC layers rather than the convolutions being sampled (leaving 58/80MB for the rest), so where is the supposed knowledge being stored? Nor does VGG appear to have tame internal dynamics lacking in other models - the layer average norms differ greatly, and rescaling appears to be unnecessary (neither they nor Johnson needed to do that like the Bethge lab did).on the gripping hand, could the FC layers in some way be forcing the lower convolutions to be different in terms of abstractions than equivalent convolutions in later less-FC-heavy models?resnets are unrolled iteration/shallow ensembles: the features do exist but they are too spread out to be pulled out easily and the levels of abstraction are all mixed up - instead of getting a nice balance of features from the bottom and top, they're spread out wildly between layer #3 and #33 and #333 etc. While VGGs, being relatively shallow and modular and having no residual connections or other special tricks to smuggle raw information up the layers, are forced to create more of a clearcut hierarchical pyramid of abstractions.Here there may be some straightforward way to better capture resnet knowledge; Pierre Richemond suggestionsProbably ResNets feature maps need to be summed depthwise before taking the Gram matrix. By that logic, one'd think DenseNets should work better than Resnets but worse than VGG (due to gradient flows from earlier layers).Residual connections themselves somehow mess up the optimization procedure by affecting properties like independence of features, with "blurring" from layers so easily passing around activations, suggests Kyle Kastner (this might be the same thing as "resnets have too many layers & split up features")VGG's better performance is due to not downsampling aggressively, doing so only after two convolutions and then max poolingIn this interpretation, GoogLeNet fails because it downsamples in the first layer.Testing hypothesesWhat tests could be done?train much bigger resnet/DenseNets to see if expanding model capacity helps; alternately, retrain much smaller VGGs to create models which are comparable in parameters to see if the gap goes away. If a small VGG can't do better style transfer than an equal-sized resnet, that suggests there is no special mystery.Add/remove FC layers from retrained VGG and resnet models. Does that lead to large gains/losses in quality?experiment with different ways of picking or summing layers to generate features; possibly brute force, trying out a large number of subsets until one works.Another approach would be to try to remove layers entirely: resnets are resistant to deleting random layers, or one could try model distillation to train a shallow but wide resnet from a SOTA deep resnet. With similar parameters, it should perform just as well, but the layer features should be more compressed and easier to find a good set.Model distillation again but for an equivalent resnet minus all residual connections? I don't know if that's trainable at all.train competing models but with VGG-style initial layers.Fixing this limitation to VGG, or showing that current resnets actually do work well and this folklore is false, could speed up style transfer training by replacing VGG with a smaller faster model, or a better one, and might give some interesting insights into what CNNs are learning & how that's affected by their architecture.. Caption by gwern. Posted By: www.eurekaking.com
0 notes