#Scrape Tweets Data Using snscrape
Explore tagged Tumblr posts
Text
Scrape Tweets Data by Location Using Python and snscrap

In this blog, we will take a comprehensive look into scraping Python wrapper and its functionality and specifically focus on using it to search for tweets based on location. We will also delve into why the wrapper may not always perform as expected. Let's dive in
snscrape is a remarkable Python library that enables users to scrape tweets from Twitter without the need for personal API keys. With its lightning-fast performance, it can retrieve thousands of tweets within seconds. Moreover, snscrape offers powerful search capabilities, allowing for highly customizable queries. While the documentation for scraping tweets by location is currently limited, this blog aims to comprehensively introduce this topic. Let's delve into the details:
Introduction to Snscrape: Snscrape is a feature-rich Python library that simplifies scraping tweets from Twitter. Unlike traditional methods that require API keys, snscrape bypasses this requirement, making it accessible to users without prior authorization. Its speed and efficiency make it an ideal choice for various applications, from research and analysis to data collection.
The Power of Location-Based Tweet Scraping: Location-based tweet scraping allows users to filter tweets based on geographical coordinates or place names. This functionality is handy for conducting location-specific analyses, monitoring regional trends, or extracting data relevant to specific areas. By leveraging Snscrape's capabilities, users can gain valuable insights from tweets originating in their desired locations.
Exploring Snscrape's Location-Based Search Tools: Snscrape provides several powerful tools for conducting location-based tweet searches. Users can effectively narrow their search results to tweets from a particular location by utilizing specific parameters and syntax. This includes defining the search query, specifying the geographical coordinates or place names, setting search limits, and configuring the desired output format. Understanding and correctly using these tools is crucial for successful location-based tweet scraping.
Overcoming Documentation Gaps: While snscrape is a powerful library, its documentation on scraping tweets by location is currently limited. This article will provide a comprehensive introduction to the topic to bridge this gap, covering the necessary syntax, parameters, and strategies for effective location-based searches. Following the step-by-step guidelines, users can overcome the lack of documentation and successfully utilize snscrape for their location-specific scraping needs.
Best Practices and Tips: Alongside exploring Snscrape's location-based scraping capabilities, this article will also offer best practices and tips for maximizing the efficiency and reliability of your scraping tasks. This includes handling rate limits, implementing error-handling mechanisms, ensuring data consistency, and staying updated with any changes or updates in Snscrape's functionality.
Introduction of snscrape Using Python
In this blog, we’ll use tahe development version of snscrape that can be installed withpip install git+https://github.com/JustAnotherArchivist/snscrape.git
Note: this needs Python 3.8 or latest
Some familiarity of the Pandas module is needed.
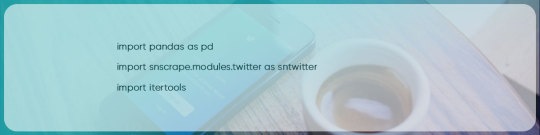




We encourage you to explore and experiment with the various features of snscrape to better understand its capabilities. Additionally, you can refer to the mentioned article for more in-depth information on the subject. Later in this blog, we will delve deeper into the user field and its significance in tweet scraping. By gaining a deeper understanding of these concepts, you can harness the full potential of snscrape for your scraping tasks.
Advanced Search Features
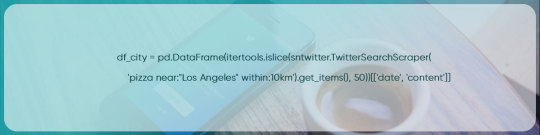
In this code snippet, we define the search query as "pizza near:Los Angeles within:10km", which specifies that we want to search for tweets containing the word "pizza" near Los Angeles within a radius of 10 km. The TwitterSearchScraper object is created with the search query, and then we iterate over the retrieved tweets and print their content.
Feel free to adjust the search query and radius per your specific requirements.
For comparing results, we can utilize an inner merging on two DataFrames:common_rows = df_coord.merge(df_city, how='inner')
That returns 50 , for example, they both have the same rows.
What precisely is this place or location?
When determining the location of tweets on Twitter, there are two primary sources: the geo-tag associated with a specific tweet and the user's location mentioned in their profile. However, it's important to note that only a small percentage of tweets (approximately 1-2%) are geo-tagged, making it an unreliable metric for location-based searches. On the other hand, many users include a location in their profile, but it's worth noting that these locations can be arbitrary and inaccurate. Some users provide helpful information like "London, England," while others might use humorous or irrelevant descriptions like "My Parents' Basement."
Despite the limited availability and potential inaccuracies of geo-tagged tweets and user profile locations, Twitter employs algorithms as part of its advanced search functionality to interpret a user's location based on their profile. This means that when you look for tweets through coordinates or city names, the search results will include tweets geotagged from the location and tweets posted by users who have that location (or a location nearby) mentioned in their profile.

To illustrate the usage of location-based searching on Twitter, let's consider an example. Suppose we perform a search for tweets near "London." Here are two examples of tweets that were found using different methods:
The first tweet is geo-tagged, which means it contains specific geographic coordinates indicating its location. In this case, the tweet was found because of its geo-tag, regardless of whether the user has a location mentioned in their profile or not.
The following tweet isn’t geo-tagged, which means that it doesn't have explicit geographic coordinates associated with it. However, it was still included in the search results because a user has given a location in the profile that matches or is closely associated with London.
When performing a location-based search on Twitter, you can come across tweets that are either geo-tagged or have users with matching or relevant locations mentioned in their profiles. This allows for a more comprehensive search, capturing tweets from specific geographic locations and users who have declared their association with those locations.
Get Location From Scraped Tweets
If you're using snscrape to scrape tweets and want to extract the user's location from the scraped data, you can do so by following these steps. In the example below, we scrape 50 tweets within a 10km radius of Los Angeles, store the data in a DataFrame, and then create a new column to capture the user's location.

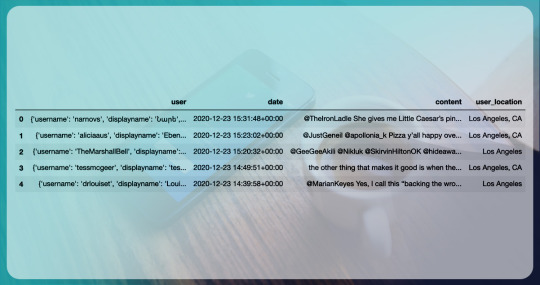
If It Doesn’t Work According to Your Expectations
The use of the near: and geocode: tags in Twitter's advanced search can sometimes yield inconsistent results, especially when searching for specific towns, villages, or countries. For instance, while searching for tweets nearby Lewisham, the results may show tweets from a completely different location, such as Hobart, Australia, which is over 17,000 km away.
To ensure more accurate results when scraping tweets by locations using snscrape, it is recommended to use the geocode tag having longitude & latitude coordinates, along with a specified radius, to narrow down the search area. This approach will provide more reliable and precise results based on the available data and features.
Conclusion
In conclusion, the snscrape Python module is a valuable tool for conducting specific and powerful searches on Twitter. Twitter has made significant efforts to convert user input locations into real places, enabling easy searching by name or coordinates. By leveraging its capabilities, users can extract relevant information from tweets based on various criteria.
For research, analysis, or other purposes, snscrape empowers users to extract valuable insights from Twitter data. Tweets serve as a valuable source of information. When combined with the capabilities of snscrape, even individuals with limited experience in Data Science or subject knowledge can undertake exciting projects.
Happy scrapping!
For more details, you can contact Actowiz Solutions anytime! Call us for all your mobile app scraping and web scraping services requirements.
know more https://www.actowizsolutions.com/scrape-tweets-data-by-location-python-snscrape.php
#ScrapeTweetsDataUsingPython#ScrapeTweetsDataUsingSnscrap#TweetsDataCollection#TweetsDataScraping#TweetsDataExtractor
0 notes
Text
How to Scrape Tweets Data by Location Using Python and snscrape?

In this blog, we will take a comprehensive look into scraping Python wrapper and its functionality and specifically focus on using it to search for tweets based on location. We will also delve into why the wrapper may not always perform as expected. Let's dive in
snscrape is a remarkable Python library that enables users to scrape tweets from Twitter without the need for personal API keys. With its lightning-fast performance, it can retrieve thousands of tweets within seconds. Moreover, snscrape offers powerful search capabilities, allowing for highly customizable queries. While the documentation for scraping tweets by location is currently limited, this blog aims to comprehensively introduce this topic. Let's delve into the details:
Introduction to Snscrape: Snscrape is a feature-rich Python library that simplifies scraping tweets from Twitter. Unlike traditional methods that require API keys, snscrape bypasses this requirement, making it accessible to users without prior authorization. Its speed and efficiency make it an ideal choice for various applications, from research and analysis to data collection.
The Power of Location-Based Tweet Scraping: Location-based tweet scraping allows users to filter tweets based on geographical coordinates or place names. This functionality is handy for conducting location-specific analyses, monitoring regional trends, or extracting data relevant to specific areas. By leveraging Snscrape's capabilities, users can gain valuable insights from tweets originating in their desired locations.
Exploring Snscrape's Location-Based Search Tools: Snscrape provides several powerful tools for conducting location-based tweet searches. Users can effectively narrow their search results to tweets from a particular location by utilizing specific parameters and syntax. This includes defining the search query, specifying the geographical coordinates or place names, setting search limits, and configuring the desired output format. Understanding and correctly using these tools is crucial for successful location-based tweet scraping.
Overcoming Documentation Gaps: While snscrape is a powerful library, its documentation on scraping tweets by location is currently limited. This article will provide a comprehensive introduction to the topic to bridge this gap, covering the necessary syntax, parameters, and strategies for effective location-based searches. Following the step-by-step guidelines, users can overcome the lack of documentation and successfully utilize snscrape for their location-specific scraping needs.
Best Practices and Tips: Alongside exploring Snscrape's location-based scraping capabilities, this article will also offer best practices and tips for maximizing the efficiency and reliability of your scraping tasks. This includes handling rate limits, implementing error-handling mechanisms, ensuring data consistency, and staying updated with any changes or updates in Snscrape's functionality.
Introduction of snscrape Using Python
In this blog, we’ll use tahe development version of snscrape that can be installed withpip install git+https://github.com/JustAnotherArchivist/snscrape.git
Note: this needs Python 3.8 or latest
Some familiarity of the Pandas module is needed.





We encourage you to explore and experiment with the various features of snscrape to better understand its capabilities. Additionally, you can refer to the mentioned article for more in-depth information on the subject. Later in this blog, we will delve deeper into the user field and its significance in tweet scraping. By gaining a deeper understanding of these concepts, you can harness the full potential of snscrape for your scraping tasks.
Advanced Search Features

In this code snippet, we define the search query as "pizza near:Los Angeles within:10km", which specifies that we want to search for tweets containing the word "pizza" near Los Angeles within a radius of 10 km. The TwitterSearchScraper object is created with the search query, and then we iterate over the retrieved tweets and print their content.
Feel free to adjust the search query and radius per your specific requirements.
For comparing results, we can utilize an inner merging on two DataFrames:common_rows = df_coord.merge(df_city, how='inner')
That returns 50 , for example, they both have the same rows.
What precisely is this place or location?
When determining the location of tweets on Twitter, there are two primary sources: the geo-tag associated with a specific tweet and the user's location mentioned in their profile. However, it's important to note that only a small percentage of tweets (approximately 1-2%) are geo-tagged, making it an unreliable metric for location-based searches. On the other hand, many users include a location in their profile, but it's worth noting that these locations can be arbitrary and inaccurate. Some users provide helpful information like "London, England," while others might use humorous or irrelevant descriptions like "My Parents' Basement."
Despite the limited availability and potential inaccuracies of geo-tagged tweets and user profile locations, Twitter employs algorithms as part of its advanced search functionality to interpret a user's location based on their profile. This means that when you look for tweets through coordinates or city names, the search results will include tweets geotagged from the location and tweets posted by users who have that location (or a location nearby) mentioned in their profile.

To illustrate the usage of location-based searching on Twitter, let's consider an example. Suppose we perform a search for tweets near "London." Here are two examples of tweets that were found using different methods:
The first tweet is geo-tagged, which means it contains specific geographic coordinates indicating its location. In this case, the tweet was found because of its geo-tag, regardless of whether the user has a location mentioned in their profile or not.
The following tweet isn’t geo-tagged, which means that it doesn't have explicit geographic coordinates associated with it. However, it was still included in the search results because a user has given a location in the profile that matches or is closely associated with London.
When performing a location-based search on Twitter, you can come across tweets that are either geo-tagged or have users with matching or relevant locations mentioned in their profiles. This allows for a more comprehensive search, capturing tweets from specific geographic locations and users who have declared their association with those locations.
Get Location From Scraped Tweets
If you're using snscrape to scrape tweets and want to extract the user's location from the scraped data, you can do so by following these steps. In the example below, we scrape 50 tweets within a 10km radius of Los Angeles, store the data in a DataFrame, and then create a new column to capture the user's location.


If It Doesn’t Work According to Your Expectations
The use of the near: and geocode: tags in Twitter's advanced search can sometimes yield inconsistent results, especially when searching for specific towns, villages, or countries. For instance, while searching for tweets nearby Lewisham, the results may show tweets from a completely different location, such as Hobart, Australia, which is over 17,000 km away.
To ensure more accurate results when scraping tweets by locations using snscrape, it is recommended to use the geocode tag having longitude & latitude coordinates, along with a specified radius, to narrow down the search area. This approach will provide more reliable and precise results based on the available data and features.
Conclusion
In conclusion, the snscrape Python module is a valuable tool for conducting specific and powerful searches on Twitter. Twitter has made significant efforts to convert user input locations into real places, enabling easy searching by name or coordinates. By leveraging its capabilities, users can extract relevant information from tweets based on various criteria.
For research, analysis, or other purposes, snscrape empowers users to extract valuable insights from Twitter data. Tweets serve as a valuable source of information. When combined with the capabilities of snscrape, even individuals with limited experience in Data Science or subject knowledge can undertake exciting projects.
Happy scrapping!
For more details, you can contact Actowiz Solutions anytime! Call us for all your mobile app scraping and web scraping services requirements.
sources :https://www.actowizsolutions.com/how-to-scrape-tweets-data-by-location-using-python-and-snscrape.php
#Tag :#Scrape Tweets Data Location#Scrape Tweets Data Using Python#Scrape Tweets Data Using snscrape#Twitter Scraper#Scrape Twitter Data#TwitterData Scraping Services
0 notes
Text
Eh all programming languages are good for certain use cases (aside from Java - Kotlin is better for android and Go is better for anything else).
Python is good at quick and dirty automation that just needs to get done. It’s very friendly to use and won’t pout at you when you ask it do something. Also once you learn to navigate pandas+numpy combined with Jupyter Notebooks it gets wayyyy faster and easier to use for data wrangling.
For example, I recently used Python to scrape hundreds of thousands of tweets via snscrape without having to use twitter’s API. Once I downloaded all the tweets it took me about 30 minutes to then do some basic analysis/labeling/sorting on said tweets.
Yes pip is terrible. Yes Python has only a hint of types (typescript style type hinting arrived in 3.something). Yes pickle creates so many vulnerabilities. Yes performant Python is basically C in a trench coat.
All that said, there’s a reason Python is many people’s first typed programming language and why I continue to use it whenever I have some data I have to fetch, transform, and analyze or whenever I’m just starting to explore a new field of computer science.
Writing Python is basically like writing pseudo code so I love it for anything that I just need to code up and run once or twice for either a proof of concept before moving to a more “serious” language or just discard the program is for my one-time personal use only.
No one should ever have to maintain more than 1k lines of Python but I will still occasionally write that much Python simply because it lets me explore high level techniques without worrying about being perfectly precise.
Python is not for production but instead for messing around. Python is that goofy ahh language that everyone likes because it doesn’t mind when you affectionately mess with it. Python is the adorable sidekick that makes programming fun again and for that I adore it
Java is a trash language that should burn in the parts of hell where hitler is
Rust on the other hand is a bratty lil language that should burn in the parts of hell where queers party
139 notes
·
View notes