#aialgorithms
Explore tagged Tumblr posts
Text
AI replacing "racist" with "Trump"
So Apple currently has this bug where when you type "racist" and it thinks your typing "Trump". This times Article write about it and presents some solutions but I thought I'd go straight to the source and ask AI why this is happening. Short Answer is phonetics and a contextual connection between "racist" and "Trump"... um not surprised. Full chat here.
Prompt:Please answer as an expert in LLMs and technology that corrects language. Can you give me five technical explanations that likely expalin the behavior descibed in phones in this article
Answer:
Here are five technical explanations that could explain the behavior described in the article:
Phonetic Overlap and Auto-Correction Algorithms: Apple's automatic dictation feature likely uses a phonetic algorithm to transcribe spoken words into text. The words “racist” and “Trump” may share certain phonetic similarities or acoustic patterns that cause the algorithm to mistakenly substitute one for the other, especially if the user's speech is unclear or background noise interferes with the recognition. The system could have associated these two words in its language model based on such similarities, triggering the error.
Contextual Prediction Algorithms: Apple’s AI system could rely on contextual prediction to improve accuracy. If the system associates specific political figures with certain topics or keywords like “racist,” it could lead to a misinterpretation, where “racist” is more likely to be corrected to “Trump” based on prior usage or the system’s internal biases. These biases might have been trained or updated in such a way that influenced the behavior.
Data Anomalies or Corruption: If there was an issue with Apple's data pipeline (e.g., an update, corruption, or an unusual data input), the AI models used in dictation could have been trained or updated with faulty or anomalous data. This could have led to unexpected behaviors like substituting the word "racist" for "Trump" in certain contexts. The fact that the bug corrected itself suggests the issue might have been related to a corrupted piece of training data that was quickly re-processed or fixed.
Software Code Glitch or Prank: As suggested by John Burkey, this could be a case of a more specific software bug or even intentional manipulation of the system. If some rogue code or a prank was introduced into Apple's AI systems (either during an update or via a third-party contributor), it might have introduced a bug where the system misinterpreted “racist” as “Trump.” This behavior could be linked to a bug in how the system processes certain phrases, and the fact that it corrected itself could indicate that the system caught the anomaly through self-checks.
Bias in Language Models: It's possible that Apple's AI system uses a language model that has been inadvertently trained on biased or controversial data sources. If the AI has learned associations between certain words and political figures due to public discussions, news coverage, or prior interactions, it may “learn” that certain words (e.g., “racist”) are more commonly associated with specific individuals (e.g., “Trump”). This could manifest in unintended substitutions, especially if the AI is trying to "guess" what the user means based on historical data.
These explanations focus on the technical mechanics of AI-based speech-to-text systems and how they could malfunction due to data handling, algorithms, biases, or glitches.
Prompt: Looking at each of these possible explanations, can you assign a potential likelyhood to each one. I'm trying to figure out what is most likely
Answer:
Certainly! Here’s an assessment of the likelihood for each explanation based on the details provided in the article:
Phonetic Overlap and Auto-Correction Algorithms Likelihood: Medium to High Phonetic overlap between words like “racist” and “Trump” is a plausible explanation for a speech-to-text error. Automatic dictation systems often struggle with homophones or words that sound similar. Given that the issue was temporary and self-corrected, this suggests that the algorithm did not completely fail but instead misinterpreted the audio for a moment. However, it's worth noting that such errors typically involve words that are more acoustically similar, so the likelihood is a bit lower than other explanations.
Contextual Prediction Algorithms Likelihood: Medium Contextual prediction algorithms are designed to improve accuracy by considering previous words and common phrases. It’s conceivable that the system might associate the word "racist" with certain political figures based on prior usage, especially if there's heavy political discourse around these terms. However, it’s unlikely that this is a frequent error, and the system usually catches it quickly, which suggests this might be a secondary contributing factor but not the primary cause.
Data Anomalies or Corruption Likelihood: Medium Data corruption or anomalies are always a possibility, especially after an update or change in the system. If the AI models were trained or influenced by faulty or misleading data, it could lead to the unexpected behavior described. Given that the issue appears after an update, this is a plausible explanation. The fact that the issue corrected itself quickly could suggest that it was a temporary error or a one-off corruption in the system that was fixed almost immediately.
Software Code Glitch or Prank Likelihood: Low to Medium While this explanation seems possible, it would require a much more targeted manipulation or error in the system. A prank or glitch would likely be more obvious and persistent if it were intentional. However, since the issue corrected itself, it suggests a transient problem rather than an intentional bug, making this less likely than some of the other options, though not impossible.
Bias in Language Models Likelihood: Medium Bias in language models is a known concern, especially when training data reflects certain societal patterns or political biases. Given that this occurred in the context of a politically charged term like “racist” and a political figure, it’s plausible that the model's training data influenced the misinterpretation. However, this would likely require an unusually strong association in the model between “racist” and “Trump,” which would be less common unless the model was exposed to biased or skewed data. Still, this remains a potential factor in the mix.
Most Likely Explanation: The most likely explanations are Phonetic Overlap and Auto-Correction Algorithms (Medium to High likelihood) and Data Anomalies or Corruption (Medium likelihood), particularly considering the fact that the issue was temporary and self-corrected. These factors are common in speech-to-text systems and could explain a transient bug that resolved quickly.
Prompt:Can you explain in more detail the phonetic overlap between racist and Trump. I don't hear it when I say the words
Answer:
Apple has acknowledged a bug in its iPhone dictation feature where the word "racist" was briefly transcribed as "Trump" before correcting itself. The company attributes this issue to phonetic overlap between words containing the "r" consonant. citeturn0news10
Phonetic overlap refers to the similarity in the sounds of different words. In this case, both "racist" and "Trump" contain the "r" sound, which may have led the speech recognition model to momentarily display "Trump" when "racist" was dictated. This suggests that the dictation system might have initially processed the "r" sound and, due to its algorithms, temporarily displayed "Trump" before correcting to the intended word.
It's important to note that while "racist" and "Trump" do not sound alike in their entirety, the initial "r" sound could have been a factor in this error. Apple is working on a fix to address this issue. citeturn0news10
navlistApple addresses dictation bug replacing 'racist' with 'Trump'turn0news10,turn0news11,turn0news12
Prompt:
So if I understand correctly - it hears the r word first and because maybe with the frequency with which people are using the name Trump - it's the first guess that comes up. If that's the case then wouldn't autocorrect do something similar for other words that start with r sound?
Answer
Yes, you're understanding it correctly. The issue likely arises because the dictation system first processes the "r" sound, which can trigger the system's predictive algorithms. Since “Trump” is a common word in certain contexts, especially politically, it may be one of the first suggestions the system provides as part of its predictive text process. Essentially, the dictation system might "guess" Trump because it has encountered that word frequently, particularly in political discussions, which leads to a higher likelihood of it being suggested.
Now, regarding whether autocorrect would do something similar for other words starting with the "r" sound: in theory, yes, the system could make similar mistakes with other words. However, several factors could prevent this from happening as frequently:
Frequency of Occurrence: "Trump" may be a unique case in that it's both a very recognizable name (with frequent appearances in political discourse) and tied to highly charged terms like "racist." Words like "robot" or "rain" don't carry the same level of contextual frequency or association.
Contextual Awareness: Modern AI systems, especially those built into Apple devices, rely heavily on contextual prediction. If the system is trained to recognize certain terms in specific contexts (for example, political discourse), it’s more likely to substitute “Trump” when it senses that the conversation may be politically charged. Words like "robot" or "racist" wouldn't trigger the same kind of predictive behavior because they aren't as commonly associated with one another.
Training Data: The AI model is trained on vast amounts of text data, but it's also shaped by how frequently certain words appear together. Words that are used together often (e.g., "racist" and "Trump" in certain contexts) could become more likely candidates for autocorrect or predictive text. Other words that start with "r" (e.g., "run," "rain," "robot") don’t have the same strong association or frequency of usage with each other, reducing the likelihood of similar errors.
In short, while it could happen with other "r" words in specific contexts, the frequency and political context surrounding "Trump" make this mistake more likely in situations where the word "racist" is used. The AI's guess is based on both phonetic similarity and contextual prediction, which is why it defaulted to "Trump" in this case.
Prompt
Wait a second, so it's about context. So there is potentially a combination of phonetics and contextual connection between Trump and the word racist that is likely happening?
Answer:
Yes, exactly! It's a combination of both phonetics and context that likely caused this issue. Here's how the two factors come into play:
Phonetics: When you say "racist," the system may first detect the "r" sound and look for possible matches in its database. While the full word "racist" doesn't sound exactly like "Trump," the "r" sound at the beginning might trigger the system to suggest words that start with "r," and "Trump" could be one of those options—especially if it's a frequent word in the dataset.
Context: This is the key part. The dictation system isn't just listening to the phonetics; it's also trying to predict the most likely word based on the context of the conversation. Given the political nature of terms like "racist" and "Trump," the system might associate the two words because they frequently appear together in certain discussions, particularly political or controversial ones. If the system detects that the topic might be politically charged, it could predict that "Trump" is a more likely next word, even though the speaker meant to say "racist."
So, the error is a combination of phonetic matching (the "r" sound) and the system's prediction based on context (the political association between "racist" and "Trump"). This dual influence is likely why the system made that substitution.
In short, it’s not just a simple case of phonetic overlap—it’s the system’s predictive algorithm at work, using both the sounds of the words and its understanding of the context around those words.
#AI#ArtificialIntelligence#SpeechRecognition#VoiceTech#MachineLearning#TechInnovation#Apple#Dictation#AIethics#BiasInAI#SpeechToText#NaturalLanguageProcessing#TechIssues#PhoneticOverlap#TechPredictiveModels#AIContextualPrediction#AIbehavior#AIAlgorithms#TechNews#DataBias
2 notes
·
View notes
Text
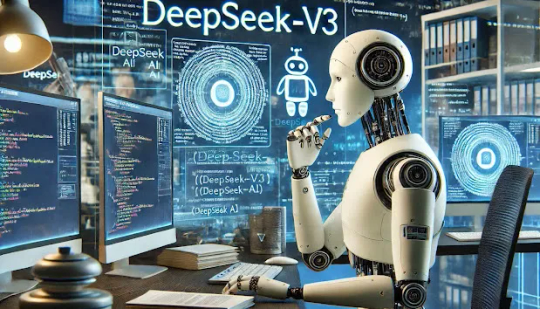
DeepSeek: The Chinese AI Company Challenging OpenAI, read more
#DeepSeek#AI#ArtificialIntelligence#DeepLearning#MachineLearning#TechInnovation#AIResearch#FutureOfAI#NeuralNetworks#BigData#AIAutomation#SmartTechnology#DataScience#AIAlgorithms#TechRevolution#FutureTechnology#AITools#AIExploration
3 notes
·
View notes
Text
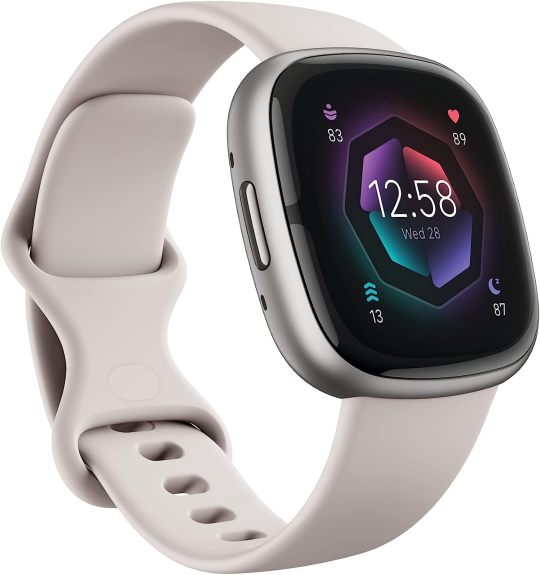
Unveiling Precision: Google Pixel Watch 2 and Fitbit Charge 6 Redefine Heart Rate Tracking
In the ever-evolving landscape of smartwatches and fitness trackers, the Google Pixel Watch 2 and Fitbit Charge 6 emerge as pioneers, showcasing the pinnacle of heart rate tracking technology. As we delve into the intricacies of these devices, it becomes evident that they are not just wearables; they are sophisticated health companions.
The Foundation: Advanced Sensors Contrary to the common misconception of heart rate tracking relying on pulse vibrations, the technology embedded in both devices surpasses expectations. The introduction of PPG sensors (photoplethysmography) marks a paradigm shift. By utilizing green LED light, these sensors measure blood circulation's volume and movement, providing a more nuanced understanding of heart rate.
The Google Pixel Watch 2 takes it a step further with its multi-path LED sensor, covering more skin surface area. This enhancement ensures more accurate recordings, especially during dynamic activities like High-Intensity Interval Training (HIIT). The struggle against the nemesis of heart-rate tracking—motion—is met head-on, resulting in a remarkable 40% improvement in accuracy during vigorous exercises. Illuminating the Path: Lights and Modes
The devices offer versatility in tracking modes. While passive all-day tracking optimizes battery life, the exercise mode illuminates all sensor lights, ensuring comprehensive data capture during intense workouts. It's a balancing act between efficiency and functionality, tailored to the user's needs.
Harnessing the Power of AI
A true game-changer lies in the integration of Artificial Intelligence (AI). The Pixel Watch 2 introduces an enhanced algorithm, a testament to the symbiosis of technology and health. These devices go beyond merely capturing heart rate data; they process it through advanced AI algorithms. The Charge 6, despite being a fitness tracker, inherits these algorithmic advancements, delivering a seven-day battery life while managing the added computational load.
Evolution Through Testing
The journey to perfection involves relentless testing. The development team's commitment is evident in their rigorous testing methods. From running on the beach to simulate unpredictable movements to accounting for diverse factors like skin tone, age, gender, and environmental conditions, the algorithms are refined to offer not just data but high-quality, reliable insights.
Beyond Numbers: Daily Readiness Score
It's not just about heart rate data; it's about actionable insights. The culmination of advanced HR sensors and AI-led algorithms births the Daily Readiness Score. Whether signaling readiness for a strenuous workout, indicating the need for rest, or suggesting a gentle push, these devices empower users to tailor their fitness journey.
A Healthier Tomorrow
In essence, the Google Pixel Watch 2 and Fitbit Charge 6 transcend the conventional boundaries of wearables. They are not just watches and trackers; they are health partners equipped with cutting-edge technology. The meticulous fusion of sensors, lights, AI, and continuous testing reaffirms their commitment to delivering accurate, meaningful data.
As we embrace these advancements, we not only monitor our heartbeats but embark on a journey toward a healthier and more informed lifestyle.
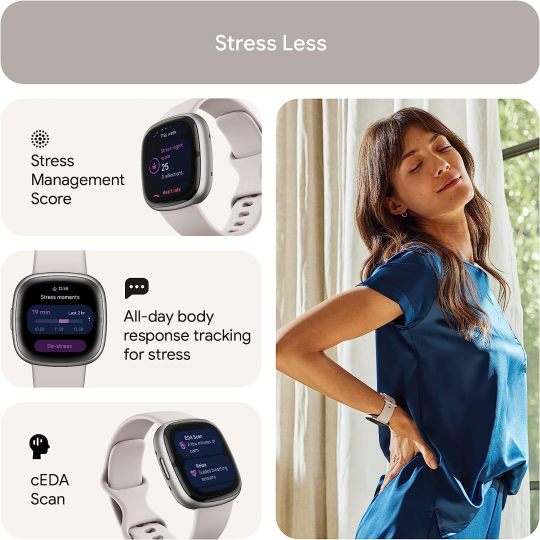
Learn More
#Smartwatch#WearableTech#PixelWatch2#HealthTech#WearableInnovation#Review#AIAlgorithms#FitTech#TechInnovations#Fitbit#GooglePixel#HealthTracking#HeartRateSensors#TechReview#Gadget
2 notes
·
View notes
Text
Artificial Intelligence (AI) is one of the most transformative technologies of the 21st century, revolutionizing industries, enhancing productivity, and shaping the future of humanity. "A Brief Introduction to Artificial Intelligence" provides a foundational understanding of AI, its concepts, applications, and implications. Below is a user-friendly, step-by-step breakdown of the key outcomes and takeaways from the book, designed to help readers grasp the essentials of AI in an accessible and structured manner.
#ArtificialIntelligence#AI#MachineLearning#TechTutorial#AIIntroduction#DeepLearning#AIForBeginners#TechEducation#DataScience#AIApplications#AIResources#AICommunity#ArtificialIntelligenceBasics#TechBooks#AIResearch#Automation#NeuralNetworks#AIProgramming#TechTrends#AIInIndustry#AIModels#AIDevelopment#FutureOfAI#Programming#TechLearning#AIAlgorithms
0 notes
Text
Why a 50 Basis Point Cut Won't Scare Investors: Fed Insights 📉💡
youtube
A 50 basis point cut might sound alarming, but is it really a cause for concern? Fed Chairman Powell’s comments after the announcement suggest they’re playing catch-up with interest rates. Despite the cut, expectations are leaning toward further rate reductions, which could keep the market steady. Let’s dive into the real reasons behind these decisions and what it means for your investments. 📊💭
#Bitcoin#CryptoExposure#AIInvesting#Smartfolio#iFlip#CryptoRevolution#BitcoinETFs#InvestmentStrategy#AIAlgorithms#TechDrivenInvesting#CryptoInvestments#FutureOfInvesting#Youtube
0 notes
Text
What Is Reinforcement Learning? And Its Applications

What is Reinforcement learning?
A machine learning (ML) method called Reinforcement Learning(RL) teaches software to make choices that will produce the best outcomes. It simulates the process of trial-and-error learning that people employ to accomplish their objectives. Actions in the software that advance your objective are rewarded, while those that hinder it are disregarded.
When processing data, RL algorithms employ a reward-and-punishment paradigm. They gain knowledge from each action’s input and figure out for themselves the most efficient processing routes to get desired results. Additionally, the algorithms can provide delayed satisfaction. The best course of action they find might involve some penalties or going back a step or two because the best overall plan might necessitate temporary sacrifices. RL is an effective technique for assisting artificial intelligence (AI) systems in achieving the best results in situations that cannot be observed.
What are the benefits of reinforcement learning?
Reinforcement learning (RL) has numerous advantages. These three, nevertheless, frequently stick out.
Excels in complex environments
In complicated systems with numerous rules and dependencies, RL algorithms can be applied. Even with superior environmental knowledge, a human might not be able to decide which course to pursue in the same situation. Rather, model-free RL algorithms discover innovative ways to maximize outcomes and quickly adjust to constantly shifting contexts.
Requires fewer interactions with people
In conventional machine learning methods, the algorithm is guided by human labeling of data pairings. Using an RL algorithm eliminates the need for this. It picks up knowledge on its own. In addition, it provides ways to include human input, enabling systems to adjust to human knowledge, preferences, and corrections.
Focuses on long-term objectives
Because RL is primarily concerned with maximizing long-term rewards, it is well-suited for situations in which decisions have long-term effects. Because it can learn from delayed incentives, it is especially well-suited for real-world scenarios where input isn’t always available at every stage.
For instance, choices regarding energy storage or consumption may have long-term effects. Long-term cost and energy efficiency can be maximized with RL. Additionally, RL agents can apply their learnt techniques to similar but distinct tasks with the right designs.
What are the use cases of reinforcement learning?
There are numerous real-world applications for reinforcement learning (RL). Next, AWS provide some examples.
Personalization in marketing
RL can tailor recommendations to specific users based on their interactions in applications such as recommendation systems. Experiences become more customized as a result. For instance, depending on certain demographic data, an application might show a user advertisements. In order to maximize product sales, the program learns which ads to show the user with each ad interaction.
Optimization problems
Conventional optimization techniques assess and contrast potential solutions according to predetermined standards in order to resolve issues. RL, on the other hand, uses interaction learning to gradually identify the best or nearly best answers.
For instance, RL is used by a cloud expenditure optimization system to select the best instance kinds, numbers, and configurations while adapting to changing resource requirements. It bases its choices on things like spending, use, and the state of the cloud infrastructure.
Forecasts for finances
Financial market dynamics are intricate, having changing statistical characteristics. By taking transaction costs into account and adjusting to changes in the market, RL algorithms can maximize long-term gains.
For example, before testing actions and recording related rewards, an algorithm could study the stock market’s laws and tendencies. It establishes a strategy to optimize earnings and dynamically generates a value function.
How does reinforcement learning work?
In behavioral psychology, the learning process of Reinforcement learning (RL) algorithms is comparable to that of human and animal reinforcement learning. A youngster might learn, for example, that when they clean or assist a sibling, they get praise from their parents, but when they yell or toss toys, they get unfavorable responses. The child quickly discovers which set of actions leads to the final reward.
A similar learning process is simulated by an RL algorithm. To get the final reward outcome, it attempts various tasks to learn the corresponding positive and negative values.
Important ideas
You should become familiar with the following important ideas in Reinforcement learning:
The ML algorithm, often known as the autonomous system, is the agent.
The environment, which has characteristics like variables, boundary values, rules, and legitimate activities, is the adaptive problem space.
The action is a move made by the RL agent to move through the surroundings.
The environment at a specific moment in time is the state.
The reward is the value that results from an activity; it can be positive, negative, or zero. The total of all incentives or the final amount is the cumulative reward.
Fundamentals of algorithms
The Markov decision process, a discrete time-step mathematical model of decision-making, is the foundation of reinforcement learning. The agent performs a new action at each stage, which changes the state of the environment. In a similar vein, the order of earlier activities is responsible for the current situation.Image credit to AWS
The agent develops a set of if-then rules or policies by navigating the environment and making mistakes. For the best cumulative reward, the policies assist it in determining the next course of action. Additionally, the agent has to decide whether to take known high-reward actions from a given state or continue exploring the environment to discover new state-action rewards. This is known as the trade-off between exploration and exploitation.
What are the types of reinforcement learning algorithms?
Reinforcement learning (RL) uses temporal difference learning, policy gradient approaches, Q-learning, and Monte Carlo methods. The use of deep neural networks for reinforcement learning is known as “deep RL.” TRPO, or Trust Region Policy Optimization, is an illustration of a deep reinforcement learning method.
Reinforcement Learning Example
Two major categories can be used to classify all of these algorithms.
Model based Reinforcement Learning
When testing in real-world situations is challenging and surroundings are well-defined and static, model-based reinforcement learning is usually employed.
First, the agent creates an internal model, or representation, of the surroundings. This procedure is used to create this model:
It acts in the surroundings and records the reward value and the new state.
It links the reward value to the action-state transition.
The agent simulates action sequences depending on the likelihood of optimal cumulative rewards after the model is finished. The action sequences themselves are then given additional values. In order to accomplish the intended end goal, the agent thus creates several tactics inside the environment.
Example
Imagine a robot that learns to find its way to a certain room in a new building. The robot first freely explores the building and creates an internal model, sometimes known as a map. For example, after advancing 10 meters from the main door, it may discover that it comes across an elevator. After creating the map, it might create a sequence of the shortest paths connecting the various places it commonly goes within the building.
Model-free RL
When the environment is big, complicated, and difficult to describe, model-free RL works best. There aren’t many serious drawbacks to environment-based testing, and it’s perfect in situations where the surroundings are unpredictable and changeable.
The environment and its dynamics are not internally modeled by the agent. Rather, it employs an environment-based trial-and-error method. In order to create a policy, it rates and records state-action pairings as well as sequences of state-action pairs.
Example
Think about a self-driving automobile that has to handle traffic in a city. The surroundings can be extremely dynamic and complex due to roads, traffic patterns, pedestrian behavior, and a myriad of other things. In the early phases, AI teams train the vehicle in a simulated environment. Depending on its current condition, the vehicle acts and is rewarded or penalized.
Without explicitly simulating all traffic dynamics, the car learns which behaviors are optimal for each state over time by traveling millions of miles in various virtual scenarios. The vehicle applies the learnt policy when it is first deployed in the real world, but it keeps improving it with fresh information.
What is the difference between reinforced, supervised, and unsupervised machine learning?
ML methods including supervised, unsupervised, and Reinforcement learning (RL) differ in AI.
Comparing supervised and reinforcement learning
Both the input and the anticipated corresponding result are defined in supervised learning. The algorithm is supposed to recognize a new animal image as either a dog or a cat, for example, if you give it a collection of pictures tagged “dogs” or “cats.”
Algorithms for supervised learning discover correlations and patterns between input and output pairs. Then, using fresh input data, they forecast results. In a training data set, each data record must be assigned an output by a supervisor, who is usually a human.
On the other hand, RL lacks a supervisor to pre-label related data, but it does have a clearly stated end objective in the form of a desired outcome. It maps inputs with potential outcomes during training rather than attempting to map inputs with known outputs. You give the greatest results more weight when you reward desired behaviors.
Reinforcement vs. unsupervised learning
During training, unsupervised learning algorithms are given inputs without any predetermined outputs. They use statistical methods to uncover hidden links and patterns in the data. For example, if you provide the algorithm a collection of documents, it might classify them into groups according to the terms it recognizes in the text. The results are inside a range and you don’t receive any particular results.
RL, on the other hand, has a preset ultimate goal. Even though it employs an exploratory methodology, the findings are regularly verified and enhanced to raise the likelihood of success. It has the ability to teach itself to achieve extremely particular results.
What are the challenges with reinforcement learning?
Although applications of Reinforcement learning(RL) have the potential to transform the world, implementing these algorithms may not be simple.
Realistic
It might not be feasible to test out reward and punishment schemes from the real world. For example, if a drone is tested in the real world without first being tested in a simulator, a large proportion of aircraft will break. Environments in the real world are subject to frequent, substantial, and little notice changes. In practice, it can make the algorithm less effective.
Interpretability
Data science examines conclusive research and findings to set standards and processes, just like any other scientific discipline. For provability and replication, data scientists would rather know how a particular result was arrived at.
It can be challenging to determine the motivations behind a specific step sequence in complicated RL algorithms. Which steps taken in a particular order produced the best outcome? Deducing this can be challenging, which makes implementation harder.
Read more on Govindhtech.com
#ReinforcementLearning#machinelearning#artificialintelligence#AIalgorithms#RLalgorithm#News#Technews#Technology#technologynews#technologytrends#govindhtech
1 note
·
View note
Text
AI Investing 2024: Why Top Investors Bet Big On New Algorithms & Learning Systems
youtube
Why Top Investors are Betting on AI & New Algorithms in 2024 #aiinvestment
With AI transforming every industry, investors seek new opportunities in this rapidly evolving field. Learn why leading experts diversify across various AI innovations, from learning systems to post-transformer algorithms.
This video explains the reasons behind massive AI investments, including market trends, emerging tech from places like Stanford and Paris, and the belief that intelligence invention could bring infinite returns. Join us to understand the real potential of AI and the key factors influencing today’s AI investments.
This video is about AI Investing 2024: Why Top Investors Bet Big On New Algorithms & Learning Systems. But It also covers the following topics:
Learning Systems AI
AI Revolution Explained
AI Funding Explained
🔗 Stay Connected With Us.
🔔 Stay on top of AI advancements and tech trends – subscribe for expert analysis, detailed reviews, industry insights, and practical tutorials! / @bytebreakthroughs
📩 For Business Inquiries:
=============================
#aiinvestment #techtrends #learningai #aialgorithms #futureofai #aiinnovation
© ByteBreakthroughs
1 note
·
View note
Text
The Rise of Artificial Intelligence in Healthcare: Transforming Patient Care and Medical Practices
Let’s focus on the topic: “The Rise of Artificial Intelligence in Healthcare: Transforming Patient Care and Medical Practices.” The Rise of Artificial Intelligence in Healthcare: Transforming Patient Care and Medical Practices Abstract Artificial Intelligence (AI) is revolutionizing healthcare, driving significant advancements in patient care, diagnostics, treatment planning, and operational…
#AIAlgorithms#AIinDiagnostics#AIinHealthcare#ArtificialIntelligence#DigitalHealth#HealthcareAI#HealthcareTrends#MedicalInnovation#PersonalizedMedicine#RoboticSurgery#Telemedicine#VirtualHealthAssistants
0 notes
Text

The Future of Talent Acquisition Through 𝐆𝐞𝐧𝐞𝐫𝐚𝐭𝐢𝐯𝐞 𝐀𝐈 . . 𝐆𝐞𝐧𝐞𝐫𝐚𝐭𝐢𝐯𝐞 𝐀𝐈 has revolutionized recruitment with its advanced mechanisms, ensuring no talent slips through the cracks. Utilizing various algorithms, it streamlines everything from sourcing to candidate engagement. Discover how generative 𝐀𝐈 is transforming recruitment and start hiring💼 top talent for free.
Continue👉 reading to learn more! https://www.placementindia.com/blog/the-future-of-talent-acquisition-through-generative-ai.htm
#GenerativeAI👨🦱#RecruitmentTech🫵#TalentAcquisition#AIRecruitment#HRTech#AIAlgorithms#RecruitmentInnovation#TalentRetention#RecruitmentProcess#HiringSolutions#TechInRecruitment#CandidateEngagement#AdvancedRecruitment#JobTech#FutureOfHiring#AITransformation#FreeHiring#ArtificialIntelligence#CareerNews#PlacementSolutions
0 notes
Text
Understanding Machine Learning and Big Data in Today's World
Machine learning is a branch of artificial intelligence wherein computers make predictions based on patterns that they have directly observed from data, without the need for explicit programming. This definition makes clear that machine learning is a branch of artificial intelligence. Since terminology like machine learning algorithms, artificial intelligence, deep learning, and even data science are frequently used interchangeably, let’s take a closer look at definitions.
#MLAlgorithms#MachineLearning#AIML#DataScience#AIAlgorithms#DeepLearning#MLTech#MLResearch#AlgorithmicAI#MLInnovation
0 notes
Text
Ready to revolutionize your approach to the stock market? Discover how AI Trading Algorithms are reshaping the investment landscape in our latest blog. From predictive analytics to automated trading strategies, unlock the secrets to success in today's dynamic markets.
Don't miss out on this insightful read! Click the link below to explore how AI Trading Algorithms can supercharge your portfolio and propel your financial goals to new heights.
0 notes
Text
How to Build An AI App: A Step-by-Step Guide (2024)

AI has changed the world by using robots for precise tasks and having cars that drive themselves. And it’s just getting started. Businesses have begun using AI a lot more, like, 270% more in the last four years! It’s not just for solving math problems anymore; it’s becoming a big deal that’s going to shape our future society and economy.
Big companies, even the huge ones, are using AI to make their work easier. And because more and more companies are using it, the AI market is growing super fast. It was worth $51.08 million in 2020, but by 2028, it’s predicted to be worth a massive $641.3 billion! Read More
0 notes
Text

Which AI application involves the use of algorithms to enable machines to improve their performance on a specific task over time?
a. Expert Systems b. Reinforcement Learning c. Genetic Algorithms d. Fuzzy Logic
#AI#AIquiz#AIPoll#followme#followforfollow#instadaily#follow4follow#like4like#letsconnect#scriptzol#AIInnovation#MachineLearning#ReinforcementLearning#AlgorithmsInAI#TechAdvancements#IntelligentMachines#FutureTechTrends#AIAlgorithms#SmartTech#InnovationInAI
0 notes
Text
This Device can Actually Record Your Dreams Like a Movie!

Being able to actually remember, record, dissect your own dreams could be an invaluable tool for everything from self-help to motivation, spirituality, and beyond. Read More. https://www.sify.com/ai-analytics/this-device-can-actually-record-your-dreams-like-a-movie/
#Dreams#AI#ArtificialIntelligence#DreamMachine#BigData#fMRImachine#FunctionalMagneticResonanceImaging#AIalgorithms#fMRIscan#sleep
0 notes
Text
Bitcoin & AI: Revolutionizing Crypto Exposure with Smartfolios 🚀💡
youtube
Many of our customers have been eager to gain exposure to Bitcoin, and we’ve listened! On the iFlip platform, we’ve developed a unique AI-driven approach to crypto investing. With the green lighting of Bitcoin ETFs in America, we’re able to offer smarter ways to manage Bitcoin investments using advanced algorithms and technology. 📈🔒 Ready to jump in? Let’s explore how AI is reshaping crypto
#Bitcoin#CryptoExposure#AIInvesting#Smartfolio#iFlip#CryptoRevolution#BitcoinETFs#InvestmentStrategy#AIAlgorithms#TechDrivenInvesting#CryptoInvestments#FutureOfInvesting#Youtube
0 notes
Text

#aialgorithms#artificialintelligence#automaticcontrol#bioinformatics#nlp#caddesign#computervision#datamining#machinelearning#fuzzylogic#heuristic#intelligentsystems#softwarearchitecture#mechatronics#neuralnetworks#patternrecognition#programminglanguages#reasoning#robotics#semanticweb#softcomputing
0 notes