#ok technically it is a set of lists to be used as datasets for a drawing idea generator
Explore tagged Tumblr posts
Text
the feeling of being autistic and making a list of cool things and then looking at your list :)
#ok technically it is a set of lists to be used as datasets for a drawing idea generator#with so far:#171 regular/modern professions#424 fantasy professions (although a lot of these are just. medieval jobs lol)#44 scifi professions#2356 real animals including extinct ones#614 fantasy animals#(not including anything from an extant major religion other than christianity.#something appearing on a random generator does not have the cultural context to be sensitive about that stuff)#(im ok appropriating christianity tho lol)#40 scifi animals/creatures#194 fungi#and 1151 plants SO FAR#im not done with plants yet#im going through wikipedia down the phylogenetic tree pretty much#and the rule is i can only write down a species if it's got a wikipedia article with a picture in it#to cut down on time#and then i write down all the cool looking ones :)#ill also write down species without a wiki article if i see something cool elsewhere but i dont go looking for them#i gotta finish this project sometime#oh yeah if anyone has suggestions for scifi stuff that isnt just space [thing] future [thing] robot [thing] etc#i would love to see them
4 notes
·
View notes
Text
Borland c++ builder onchange
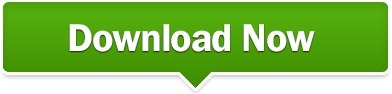
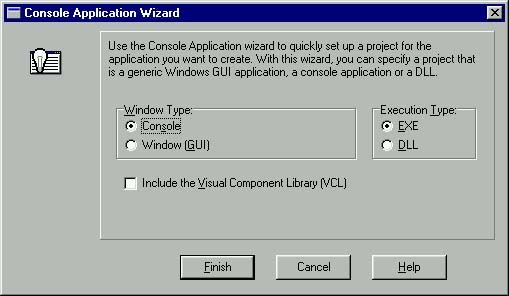
Int Alpha = ScrollBar1->Position // Alpha will store how much Alpha you want. Either place this code in the FormCreate method, or in a Buttwhenclickedon, a ScrollBar OnChange. Once you have done that, on to the code that matters. This method should work in all Builder versions however.įirst of all, you need to include the following defines If you are using 5, it is a bit easier than mentioned below, as your version includes newer API calls. Ok, im gonna presume that everyone is using borland C++ Builder 4. By using this code you agree to indemnify UtilMind Solutions from any liability that might arise from its use.
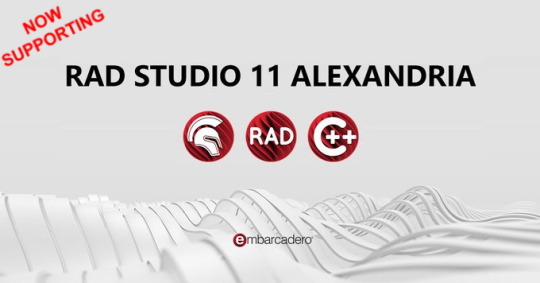
All this stuff is written in Borland Delphi. Its a really cool effect, below is how to do it. This page contains the set of freeware and shareware Delphi and C++ Builder components, which can help developers to write their own applications more effective and save their time. This is just one of the solutions for you to be successful. Bug - Fixed output monitor not saving regex match to variable. Bug - Persistent variables were only being saved after successful builds. Change - Added code completion to FBStandardGrid. Feature - Improvements to code completion in action studio. This also enables you to save space on the form.See the image below for examples of using the page control.XYZ軟體補給站 強力推薦!!!一定讓你值回票價,保證錯不了���-=-=-=-=-=-=-=-=-=-=-=-=-=-=-=-=-=-=-=-=-=-=-=-=-=-=-=-=-=-=-=-=-=-=-=-= DevExpress ExpressNavBar Suite v1.I just discovered how to make forms see through, like the start menu when u drag it. borland-c-builder-the-complete-reference 1/1 Downloaded from qa. on Novemby guest MOBI Borland C Builder The Complete Reference Yeah, reviewing a ebook borland c builder the complete reference could amass your near associates listings. Feature - Added OnChange event to CheckListBox control. Using this control allowsyou to break controls needed in your application intocategories. You will have to use a special descendant written by Dmitri Dimitrienko for Kylix support. It is however not designed to work directly with Kylix or Delphi for. Thus, you cancustomize the content of each page separately by droppingcontrols onto it.When switching between tabs, the controlautomatically displays the corresponding page.Use the page control if you need different set of controlsto reside on each separate page. Virtual Treeview is designed for Delphi 4 and higher and can also be used with Borland C++ Builder 4 and up. Borland Software Corporation may have patents and/or. Brian Long used to work at Borland UK, performing a number of duties including Technical Support on all the programming tools. It also contains a set of tabs and a client area.The only difference is that the page control provides aseparate page panel for each tab within it. that you can distribute in accordance with the C++Builder License Statement and Limited Warranty. See the image below for an example of using thetab control within other tab controls.The page control provides the same features as the tabcontrol. The image belowdisplays the tab control after completing the steps listedin that topic.You can also create more complex structures using the tabcontrol. Thecontent of controls can be dynamically changed by handlingthe OnChange event.Thus, one of the common applications ofthe tab control is to display dataset records.Tabs displayvalues of the dataset field that can identify a record.Controls within the page display values of other fieldsfrom the selected record.Please refer to the Using the TabControl to Display Dataset Records topic for an example ofusing the tab control in this manner. Thus, youcannot specify different page contents for different tabs(unless you change the page content dynamically in code).The tab control is most likely to be used if you want thesame set of controls to be displayed within its page. Note that unlike the page control, thetab control client area is a single panel.
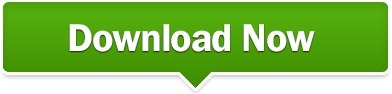
0 notes
Text
Page Level Query Analysis at Scale with Google Colab, Python, & the GSC API [Video Instructions Included]
The YouTube playlist referenced throughout this blog can be found here:6 Part YouTube Series [Setting Up & Using the Query Optimization Checker]
Anyone who does SEO as part of their job knows that there’s a lot of value in analyzing which queries are and are not sending traffic to specific pages on a site.
The most common uses for these datasets are to align on-page optimizations with existing rankings and traffic, and to identify gaps in ranking keywords.
However, working with this data is extremely tedious because it’s only available in the Google Search Console interface, and you have to look at only one page at a time.
On top of that, to get information on the text included in the ranking page, you either need to manually review it or extract it with a tool like Screaming Frog.
You need this kind of view:
…but even the above view would only be viable one page at a time, and as mentioned, the actual text extraction would have had to be separate as well.
Given these apparent issues with the readily available data at the SEO community’s disposal, the data engineering team at Inseev Interactive has been spending a lot of time thinking about how we can improve these processes at scale.
One specific example that we’ll be reviewing in this post is a simple script that allows you to get the above data in a flexible format for many great analytical views.
Better yet, this will all be available with only a few single input variables.
A quick rundown of tool functionality
The tool automatically compares the text on-page to the Google Search Console top queries at the page-level to let you know which queries are on-page as well as how many times they appear on the page. An optional XPath variable also allows you to specify the part of the page you want to analyze text on.
This means you’ll know exactly what queries are driving clicks/impressions that are not in your <title>, <h1>, or even something as specific as the first paragraph within the main content (MC). The sky's the limit.
For those of you not familiar, we’ve also provided some quick XPath expressions you can use, as well as how to create site-specific XPath expressions within the "Input Variables" section of the post.
Post setup usage & datasets
Once the process is set up, all that’s required is filling out a short list of variables and the rest is automated for you.
The output dataset includes multiple automated CSV datasets, as well as a structured file format to keep things organized. A simple pivot of the core analysis automated CSV can provide you with the below dataset and many other useful layouts.
… Even some "new metrics"?
Okay, not technically "new," but if you exclusively use the Google Search Console user interface, then you haven’t likely had access to metrics like these before: "Max Position," "Min Position," and "Count Position" for the specified date range – all of which are explained in the "Running your first analysis" section of the post.
To really demonstrate the impact and usefulness of this dataset, in the video below we use the Colab tool to:
[3 Minutes] — Find non-brand <title> optimization opportunities for https://www.inseev.com/ (around 30 pages in video, but you could do any number of pages)
[3 Minutes] — Convert the CSV to a more useable format
[1 Minute] – Optimize the first title with the resulting dataset
youtube
Okay, you’re all set for the initial rundown. Hopefully we were able to get you excited before moving into the somewhat dull setup process.
Keep in mind that at the end of the post, there is also a section including a few helpful use cases and an example template! To jump directly to each section of this post, please use the following links:
One-time setup of the script in Google Colab
Running your first analysis
Practical use cases and templates
[Quick Consideration #1] — The web scraper built into the tool DOES NOT support JavaScript rendering. If your website uses client-side rendering, the full functionality of the tool unfortunately will not work.
[Quick Consideration #2] — This tool has been heavily tested by the members of the Inseev team. Most bugs [specifically with the web scraper] have been found and fixed, but like any other program, it is possible that other issues may come up.
If you encounter any errors, feel free to reach out to us directly at [email protected] or [email protected], and either myself or one of the other members of the data engineering team at Inseev would be happy to help you out.
If new errors are encountered and fixed, we will always upload the updated script to the code repository linked in the sections below so the most up-to-date code can be utilized by all!
One-time setup of the script in Google Colab (in less than 20 minutes)
Things you’ll need:
Google Drive
Google Cloud Platform account
Google Search Console access
Video walkthrough: tool setup process
Below you’ll find step-by-step editorial instructions in order to set up the entire process. However, if following editorial instructions isn’t your preferred method, we recorded a video of the setup process as well.
As you’ll see, we start with a brand new Gmail and set up the entire process in approximately 12 minutes, and the output is completely worth the time.
youtube
Keep in mind that the setup is one-off, and once set up, the tool should work on command from there on!
Editorial walkthrough: tool setup process
Four-part process:
Download the files from Github and set up in Google Drive
Set up a Google Cloud Platform (GCP) Project (skip if you already have an account)
Create the OAuth 2.0 client ID for the Google Search Console (GSC) API (skip if you already have an OAuth client ID with the Search Console API enabled)
Add the OAuth 2.0 credentials to the Config.py file
Part one: Download the files from Github and set up in Google Drive
Download source files (no code required)
1. Navigate here.
2. Select "Code" > "Download Zip"
*You can also use 'git clone https://github.com/jmelm93/query-optmization-checker.git' if you’re more comfortable using the command prompt.
Initiate Google Colab in Google Drive
If you already have a Google Colaboratory setup in your Google Drive, feel free to skip this step.
1. Navigate here.
2. Click "New" > "More" > "Connect more apps".
3. Search "Colaboratory" > Click into the application page.
4. Click "Install" > "Continue" > Sign in with OAuth.
5. Click "OK" with the prompt checked so Google Drive automatically sets appropriate files to open with Google Colab (optional).
Import the downloaded folder to Google Drive & open in Colab
1. Navigate to Google Drive and create a folder called "Colab Notebooks".
IMPORTANT: The folder needs to be called "Colab Notebooks" as the script is configured to look for the "api" folder from within "Colab Notebooks".
Error resulting in improper folder naming.
2. Import the folder downloaded from Github into Google Drive.
At the end of this step, you should have a folder in your Google Drive that contains the below items:
Part two: Set up a Google Cloud Platform (GCP) project
If you already have a Google Cloud Platform (GCP) account, feel free to skip this part.
1. Navigate to the Google Cloud page.
2. Click on the "Get started for free" CTA (CTA text may change over time).
3. Sign in with the OAuth credentials of your choice. Any Gmail email will work.
4. Follow the prompts to sign up for your GCP account.
You’ll be asked to supply a credit card to sign up, but there is currently a $300 free trial and Google notes that they won’t charge you until you upgrade your account.
Part three: Create a 0Auth 2.0 client ID for the Google Search Console (GSC) API
1. Navigate here.
2. After you log in to your desired Google Cloud account, click "ENABLE".
3. Configure the consent screen.
In the consent screen creation process, select "External," then continue onto the "App Information."
Example below of minimum requirements:
Skip "Scopes"
Add the email(s) you’ll use for the Search Console API authentication into the "Test Users". There could be other emails versus just the one that owns the Google Drive. An example may be a client’s email where you access the Google Search Console UI to view their KPIs.
4. In the left-rail navigation, click into "Credentials" > "CREATE CREDENTIALS" > "OAuth Client ID" (Not in image).
5. Within the "Create OAuth client ID" form, fill in:
Application Type = Desktop app
Name = Google Colab
Click "CREATE"
6. Save the "Client ID" and "Client Secret" — as these will be added into the "api" folder config.py file from the Github files we downloaded.
These should have appeared in a popup after hitting "CREATE"
The "Client Secret" is functionally the password to your Google Cloud (DO NOT post this to the public/share it online)
Part four: Add the OAuth 2.0 credentials to the Config.py file
1. Return to Google Drive and navigate into the "api" folder.
2. Click into config.py.
3. Choose to open with "Text Editor" (or another app of your choice) to modify the config.py file.
4. Update the three areas highlighted below with your:
CLIENT_ID: From the OAuth 2.0 client ID setup process
CLIENT_SECRET: From the OAuth 2.0 client ID setup process
GOOGLE_CREDENTIALS: Email that corresponds with your CLIENT_ID & CLIENT_SECRET
5. Save the file once updated!
Congratulations, the boring stuff is over. You are now ready to start using the Google Colab file!
Running your first analysis
Running your first analysis may be a little intimidating, but stick with it and it will get easy fast.
Below, we’ve provided details regarding the input variables required, as well as notes on things to keep in mind when running the script and analyzing the resulting dataset.
After we walk through these items, there are also a few example projects and video walkthroughs showcasing ways to utilize these datasets for client deliverables.
Setting up the input variables
XPath extraction with the "xpath_selector" variable
Have you ever wanted to know every query driving clicks and impressions to a webpage that aren’t in your <title> or <h1> tag? Well, this parameter will allow you to do just that.
While optional, using this is highly encouraged and we feel it "supercharges" the analysis. Simply define site sections with Xpaths and the script will do the rest.
youtube
In the above video, you’ll find examples on how to create site specific extractions. In addition, below are some universal extractions that should work on almost any site on the web:
'//title' # Identifies a <title> tag
'//h1' # Identifies a <h1> tag
'//h2' # Identifies a <h2> tag
Site Specific: How to scrape only the main content (MC)?
Chaining Xpaths – Add a "|" Between Xpaths
'//title | //h1' # Gets you both the <title> and <h1> tag in 1 run
'//h1 | //h2 | //h3' # Gets you both the <h1>, <h2> and <h3> tags in 1 run
Other variables
Here’s a video overview of the other variables with a short description of each.
youtube
'colab_path' [Required] – The path in which the Colab file lives. This should be "/content/drive/My Drive/Colab Notebooks/".
'domain_lookup' [Required] – Homepage of the website utilized for analysis.
'startdate' & 'enddate' [Required] – Date range for the analysis period.
'gsc_sorting_field' [Required] – The tool pulls the top N pages as defined by the user. The "top" is defined by either "clicks_sum" or "impressions_sum." Please review the video for a more detailed description.
'gsc_limit_pages_number' [Required] – Numeric value that represents the number of resulting pages you’d like within the dataset.
'brand_exclusions' [Optional] – The string sequence(s) that commonly result in branded queries (e.g., anything containing "inseev" will be branded queries for "Inseev Interactive").
'impressions_exclusion' [Optional] – Numeric value used to exclude queries that are potentially irrelevant due to the lack of pre-existing impressions. This is primarily relevant for domains with strong pre-existing rankings on a large scale number of pages.
'page_inclusions' [Optional] – The string sequence(s) that are found within the desired analysis page type. If you’d like to analyze the entire domain, leave this section blank.
Running the script
Keep in mind that once the script finishes running, you’re generally going to use the "step3_query-optimizer_domain-YYYY-MM-DD.csv" file for analysis, but there are others with the raw datasets to browse as well.
Practical use cases for the "step3_query-optimizer_domain-YYYY-MM-DD.csv" file can be found in the "Practical use cases and templates" section.
That said, there are a few important things to note while testing things out:
1. No JavaScript Crawling: As mentioned at the start of the post, this script is NOT set up for JavaScript crawling, so if your target website uses a JS frontend with client-side rendering to populate the main content (MC), the scrape will not be useful. However, the basic functionality of quickly getting the top XX (user-defined) queries and pages can still be useful by itself.
2. Google Drive / GSC API Auth: The first time you run the script in each new session it will prompt you to authenticate both the Google Drive and the Google Search Console credentials.
Google Drive authentication: Authenticate to whatever email is associated with the Google Drive with the script.
GSC authentication: Authenticate whichever email has permission to use the desired Google Search Console account.
If you attempt to authenticate and you get an error that looks like the one below, please revisit the "Add the email(s) you’ll use the Colab app with into the 'Test Users'" from Part 3, step 3 in the process above: setting up the consent screen.
Quick tip: The Google Drive account and the GSC Authentication DO NOT have to be the same email, but they do require separate authentications with OAuth.
3. Running the script: Either navigate to "Runtime" > "Restart and Run All" or use the keyboard shortcut CTRL + fn9 to start running the script.
4. Populated datasets/folder structure: There are three CSVs populated by the script – all nested within a folder structure based on the "domain_lookup" input variable.
Automated Organization [Folders]: Each time you rerun the script on a new domain, it will create a new folder structure in order to keep things organized.
Automated Organization [File Naming]: The CSVs include the date of the export appended to the end, so you’ll always know when the process ran as well as the date range for the dataset.
5. Date range for dataset: Inside of the dataset there is a "gsc_datasetID" column generated, which includes the date range of the extraction.
6. Unfamiliar metrics: The resulting dataset has all the KPIs we know and love – e.g. clicks, impressions, average (mean) position — but there are also a few you cannot get directly from the GSC UI:
'count_instances_gsc' — the number of instances the query got at least 1 impression during the specified date range. Scenario example: GSC tells you that you were in an average position 6 for a large keyword like "flower delivery" and you only received 20 impressions in a 30-day date range. Doesn’t seem possible that you were really in position 6, right? Well, now you can see that was potentially because you only actually showed up on one day in that 30-day date range (e.g. count_instances_gsc = 1)
'max_position' & 'min_position' — the MAXIMUM and MINIMUM ranking position the identified page showed up for in Google Search within the specified date range.
Quick tip #1: Large variance in max/min may tell you that your keyword has been fluctuating heavily.
Quick tip #2: These KPIs, in conjunction with the "count_instances_gsc", can exponentially further your understanding of query performance and opportunity.
Practical use cases and templates
Access the recommended multi-use template.
Recommended use: Download file and use with Excel. Subjectively speaking, I believe Excel has a much more user friendly pivot table functionality in comparison to Google Sheets — which is critical for using this template.
Alternative use: If you do not have Microsoft Excel or you prefer a different tool, you can use most spreadsheet apps that contain pivot functionality.
For those who opt for an alternative spreadsheet software/app:
Below are the pivot fields to mimic upon setup.
You may have to adjust the Vlookup functions found on the "Step 3 _ Analysis Final Doc" tab, depending on whether your updated pivot columns align with the current pivot I’ve supplied.
Project example: Title & H1 re-optimizations (video walkthrough)
Project description: Locate keywords that are driving clicks and impressions to high value pages and that do not exist within the <title> and <h1> tags by reviewing GSC query KPIs vs. current page elements. Use the resulting findings to re-optimize both the <title> and <h1> tags for pre-existing pages.
Project assumptions: This process assumes that inserting keywords into both the <title> and <h1> tags is a strong SEO practice for relevancy optimization, and that it’s important to include related keyword variants into these areas (e.g. non-exact match keywords with matching SERP intent).
youtube
Project example: On-page text refresh/re-optimization
Project description: Locate keywords that are driving clicks and impressions to editorial pieces of content that DO NOT exist within the first paragraph within the body of the main content (MC). Perform an on-page refresh of introductory content within editorial pages to include high value keyword opportunities.
Project assumptions: This process assumes that inserting keywords into the first several sentences of a piece of content is a strong SEO practice for relevancy optimization, and that it’s important to include related keyword variants into these areas (e.g. non-exact match keywords with matching SERP intent).
youtube
Final thoughts
We hope this post has been helpful and opened you up to the idea of using Python and Google Colab to supercharge your relevancy optimization strategy.
As mentioned throughout the post, keep the following in mind:
Github repository will be updated with any changes we make in the future.
There is the possibility of undiscovered errors. If these occur, Inseev is happy to help! In fact, we would actually appreciate you reaching out to investigate and fix errors (if any do appear). This way others don’t run into the same problems.
Other than the above, if you have any ideas on ways to Colab (pun intended) on data analytics projects, feel free to reach out with ideas.
0 notes
Text
Page Level Query Analysis at Scale with Google Colab, Python, & the GSC API [Video Instructions Included]
The YouTube playlist referenced throughout this blog can be found here:6 Part YouTube Series [Setting Up & Using the Query Optimization Checker]
Anyone who does SEO as part of their job knows that there’s a lot of value in analyzing which queries are and are not sending traffic to specific pages on a site.
The most common uses for these datasets are to align on-page optimizations with existing rankings and traffic, and to identify gaps in ranking keywords.
However, working with this data is extremely tedious because it’s only available in the Google Search Console interface, and you have to look at only one page at a time.
On top of that, to get information on the text included in the ranking page, you either need to manually review it or extract it with a tool like Screaming Frog.
You need this kind of view:
…but even the above view would only be viable one page at a time, and as mentioned, the actual text extraction would have had to be separate as well.
Given these apparent issues with the readily available data at the SEO community’s disposal, the data engineering team at Inseev Interactive has been spending a lot of time thinking about how we can improve these processes at scale.
One specific example that we’ll be reviewing in this post is a simple script that allows you to get the above data in a flexible format for many great analytical views.
Better yet, this will all be available with only a few single input variables.
A quick rundown of tool functionality
The tool automatically compares the text on-page to the Google Search Console top queries at the page-level to let you know which queries are on-page as well as how many times they appear on the page. An optional XPath variable also allows you to specify the part of the page you want to analyze text on.
This means you’ll know exactly what queries are driving clicks/impressions that are not in your <title>, <h1>, or even something as specific as the first paragraph within the main content (MC). The sky's the limit.
For those of you not familiar, we’ve also provided some quick XPath expressions you can use, as well as how to create site-specific XPath expressions within the "Input Variables" section of the post.
Post setup usage & datasets
Once the process is set up, all that’s required is filling out a short list of variables and the rest is automated for you.
The output dataset includes multiple automated CSV datasets, as well as a structured file format to keep things organized. A simple pivot of the core analysis automated CSV can provide you with the below dataset and many other useful layouts.
… Even some "new metrics"?
Okay, not technically "new," but if you exclusively use the Google Search Console user interface, then you haven’t likely had access to metrics like these before: "Max Position," "Min Position," and "Count Position" for the specified date range – all of which are explained in the "Running your first analysis" section of the post.
To really demonstrate the impact and usefulness of this dataset, in the video below we use the Colab tool to:
[3 Minutes] — Find non-brand <title> optimization opportunities for https://www.inseev.com/ (around 30 pages in video, but you could do any number of pages)
[3 Minutes] — Convert the CSV to a more useable format
[1 Minute] – Optimize the first title with the resulting dataset
youtube
Okay, you’re all set for the initial rundown. Hopefully we were able to get you excited before moving into the somewhat dull setup process.
Keep in mind that at the end of the post, there is also a section including a few helpful use cases and an example template! To jump directly to each section of this post, please use the following links:
One-time setup of the script in Google Colab
Running your first analysis
Practical use cases and templates
[Quick Consideration #1] — The web scraper built into the tool DOES NOT support JavaScript rendering. If your website uses client-side rendering, the full functionality of the tool unfortunately will not work.
[Quick Consideration #2] — This tool has been heavily tested by the members of the Inseev team. Most bugs [specifically with the web scraper] have been found and fixed, but like any other program, it is possible that other issues may come up.
If you encounter any errors, feel free to reach out to us directly at [email protected] or [email protected], and either myself or one of the other members of the data engineering team at Inseev would be happy to help you out.
If new errors are encountered and fixed, we will always upload the updated script to the code repository linked in the sections below so the most up-to-date code can be utilized by all!
One-time setup of the script in Google Colab (in less than 20 minutes)
Things you’ll need:
Google Drive
Google Cloud Platform account
Google Search Console access
Video walkthrough: tool setup process
Below you’ll find step-by-step editorial instructions in order to set up the entire process. However, if following editorial instructions isn’t your preferred method, we recorded a video of the setup process as well.
As you’ll see, we start with a brand new Gmail and set up the entire process in approximately 12 minutes, and the output is completely worth the time.
youtube
Keep in mind that the setup is one-off, and once set up, the tool should work on command from there on!
Editorial walkthrough: tool setup process
Four-part process:
Download the files from Github and set up in Google Drive
Set up a Google Cloud Platform (GCP) Project (skip if you already have an account)
Create the OAuth 2.0 client ID for the Google Search Console (GSC) API (skip if you already have an OAuth client ID with the Search Console API enabled)
Add the OAuth 2.0 credentials to the Config.py file
Part one: Download the files from Github and set up in Google Drive
Download source files (no code required)
1. Navigate here.
2. Select "Code" > "Download Zip"
*You can also use 'git clone https://github.com/jmelm93/query-optmization-checker.git' if you’re more comfortable using the command prompt.
Initiate Google Colab in Google Drive
If you already have a Google Colaboratory setup in your Google Drive, feel free to skip this step.
1. Navigate here.
2. Click "New" > "More" > "Connect more apps".
3. Search "Colaboratory" > Click into the application page.
4. Click "Install" > "Continue" > Sign in with OAuth.
5. Click "OK" with the prompt checked so Google Drive automatically sets appropriate files to open with Google Colab (optional).
Import the downloaded folder to Google Drive & open in Colab
1. Navigate to Google Drive and create a folder called "Colab Notebooks".
IMPORTANT: The folder needs to be called "Colab Notebooks" as the script is configured to look for the "api" folder from within "Colab Notebooks".
Error resulting in improper folder naming.
2. Import the folder downloaded from Github into Google Drive.
At the end of this step, you should have a folder in your Google Drive that contains the below items:
Part two: Set up a Google Cloud Platform (GCP) project
If you already have a Google Cloud Platform (GCP) account, feel free to skip this part.
1. Navigate to the Google Cloud page.
2. Click on the "Get started for free" CTA (CTA text may change over time).
3. Sign in with the OAuth credentials of your choice. Any Gmail email will work.
4. Follow the prompts to sign up for your GCP account.
You’ll be asked to supply a credit card to sign up, but there is currently a $300 free trial and Google notes that they won’t charge you until you upgrade your account.
Part three: Create a 0Auth 2.0 client ID for the Google Search Console (GSC) API
1. Navigate here.
2. After you log in to your desired Google Cloud account, click "ENABLE".
3. Configure the consent screen.
In the consent screen creation process, select "External," then continue onto the "App Information."
Example below of minimum requirements:
Skip "Scopes"
Add the email(s) you’ll use for the Search Console API authentication into the "Test Users". There could be other emails versus just the one that owns the Google Drive. An example may be a client’s email where you access the Google Search Console UI to view their KPIs.
4. In the left-rail navigation, click into "Credentials" > "CREATE CREDENTIALS" > "OAuth Client ID" (Not in image).
5. Within the "Create OAuth client ID" form, fill in:
Application Type = Desktop app
Name = Google Colab
Click "CREATE"
6. Save the "Client ID" and "Client Secret" — as these will be added into the "api" folder config.py file from the Github files we downloaded.
These should have appeared in a popup after hitting "CREATE"
The "Client Secret" is functionally the password to your Google Cloud (DO NOT post this to the public/share it online)
Part four: Add the OAuth 2.0 credentials to the Config.py file
1. Return to Google Drive and navigate into the "api" folder.
2. Click into config.py.
3. Choose to open with "Text Editor" (or another app of your choice) to modify the config.py file.
4. Update the three areas highlighted below with your:
CLIENT_ID: From the OAuth 2.0 client ID setup process
CLIENT_SECRET: From the OAuth 2.0 client ID setup process
GOOGLE_CREDENTIALS: Email that corresponds with your CLIENT_ID & CLIENT_SECRET
5. Save the file once updated!
Congratulations, the boring stuff is over. You are now ready to start using the Google Colab file!
Running your first analysis
Running your first analysis may be a little intimidating, but stick with it and it will get easy fast.
Below, we’ve provided details regarding the input variables required, as well as notes on things to keep in mind when running the script and analyzing the resulting dataset.
After we walk through these items, there are also a few example projects and video walkthroughs showcasing ways to utilize these datasets for client deliverables.
Setting up the input variables
XPath extraction with the "xpath_selector" variable
Have you ever wanted to know every query driving clicks and impressions to a webpage that aren’t in your <title> or <h1> tag? Well, this parameter will allow you to do just that.
While optional, using this is highly encouraged and we feel it "supercharges" the analysis. Simply define site sections with Xpaths and the script will do the rest.
youtube
In the above video, you’ll find examples on how to create site specific extractions. In addition, below are some universal extractions that should work on almost any site on the web:
'//title' # Identifies a <title> tag
'//h1' # Identifies a <h1> tag
'//h2' # Identifies a <h2> tag
Site Specific: How to scrape only the main content (MC)?
Chaining Xpaths – Add a "|" Between Xpaths
'//title | //h1' # Gets you both the <title> and <h1> tag in 1 run
'//h1 | //h2 | //h3' # Gets you both the <h1>, <h2> and <h3> tags in 1 run
Other variables
Here’s a video overview of the other variables with a short description of each.
youtube
'colab_path' [Required] – The path in which the Colab file lives. This should be "/content/drive/My Drive/Colab Notebooks/".
'domain_lookup' [Required] – Homepage of the website utilized for analysis.
'startdate' & 'enddate' [Required] – Date range for the analysis period.
'gsc_sorting_field' [Required] – The tool pulls the top N pages as defined by the user. The "top" is defined by either "clicks_sum" or "impressions_sum." Please review the video for a more detailed description.
'gsc_limit_pages_number' [Required] – Numeric value that represents the number of resulting pages you’d like within the dataset.
'brand_exclusions' [Optional] – The string sequence(s) that commonly result in branded queries (e.g., anything containing "inseev" will be branded queries for "Inseev Interactive").
'impressions_exclusion' [Optional] – Numeric value used to exclude queries that are potentially irrelevant due to the lack of pre-existing impressions. This is primarily relevant for domains with strong pre-existing rankings on a large scale number of pages.
'page_inclusions' [Optional] – The string sequence(s) that are found within the desired analysis page type. If you’d like to analyze the entire domain, leave this section blank.
Running the script
Keep in mind that once the script finishes running, you’re generally going to use the "step3_query-optimizer_domain-YYYY-MM-DD.csv" file for analysis, but there are others with the raw datasets to browse as well.
Practical use cases for the "step3_query-optimizer_domain-YYYY-MM-DD.csv" file can be found in the "Practical use cases and templates" section.
That said, there are a few important things to note while testing things out:
1. No JavaScript Crawling: As mentioned at the start of the post, this script is NOT set up for JavaScript crawling, so if your target website uses a JS frontend with client-side rendering to populate the main content (MC), the scrape will not be useful. However, the basic functionality of quickly getting the top XX (user-defined) queries and pages can still be useful by itself.
2. Google Drive / GSC API Auth: The first time you run the script in each new session it will prompt you to authenticate both the Google Drive and the Google Search Console credentials.
Google Drive authentication: Authenticate to whatever email is associated with the Google Drive with the script.
GSC authentication: Authenticate whichever email has permission to use the desired Google Search Console account.
If you attempt to authenticate and you get an error that looks like the one below, please revisit the "Add the email(s) you’ll use the Colab app with into the 'Test Users'" from Part 3, step 3 in the process above: setting up the consent screen.
Quick tip: The Google Drive account and the GSC Authentication DO NOT have to be the same email, but they do require separate authentications with OAuth.
3. Running the script: Either navigate to "Runtime" > "Restart and Run All" or use the keyboard shortcut CTRL + fn9 to start running the script.
4. Populated datasets/folder structure: There are three CSVs populated by the script – all nested within a folder structure based on the "domain_lookup" input variable.
Automated Organization [Folders]: Each time you rerun the script on a new domain, it will create a new folder structure in order to keep things organized.
Automated Organization [File Naming]: The CSVs include the date of the export appended to the end, so you’ll always know when the process ran as well as the date range for the dataset.
5. Date range for dataset: Inside of the dataset there is a "gsc_datasetID" column generated, which includes the date range of the extraction.
6. Unfamiliar metrics: The resulting dataset has all the KPIs we know and love – e.g. clicks, impressions, average (mean) position — but there are also a few you cannot get directly from the GSC UI:
'count_instances_gsc' — the number of instances the query got at least 1 impression during the specified date range. Scenario example: GSC tells you that you were in an average position 6 for a large keyword like "flower delivery" and you only received 20 impressions in a 30-day date range. Doesn’t seem possible that you were really in position 6, right? Well, now you can see that was potentially because you only actually showed up on one day in that 30-day date range (e.g. count_instances_gsc = 1)
'max_position' & 'min_position' — the MAXIMUM and MINIMUM ranking position the identified page showed up for in Google Search within the specified date range.
Quick tip #1: Large variance in max/min may tell you that your keyword has been fluctuating heavily.
Quick tip #2: These KPIs, in conjunction with the "count_instances_gsc", can exponentially further your understanding of query performance and opportunity.
Practical use cases and templates
Access the recommended multi-use template.
Recommended use: Download file and use with Excel. Subjectively speaking, I believe Excel has a much more user friendly pivot table functionality in comparison to Google Sheets — which is critical for using this template.
Alternative use: If you do not have Microsoft Excel or you prefer a different tool, you can use most spreadsheet apps that contain pivot functionality.
For those who opt for an alternative spreadsheet software/app:
Below are the pivot fields to mimic upon setup.
You may have to adjust the Vlookup functions found on the "Step 3 _ Analysis Final Doc" tab, depending on whether your updated pivot columns align with the current pivot I’ve supplied.
Project example: Title & H1 re-optimizations (video walkthrough)
Project description: Locate keywords that are driving clicks and impressions to high value pages and that do not exist within the <title> and <h1> tags by reviewing GSC query KPIs vs. current page elements. Use the resulting findings to re-optimize both the <title> and <h1> tags for pre-existing pages.
Project assumptions: This process assumes that inserting keywords into both the <title> and <h1> tags is a strong SEO practice for relevancy optimization, and that it’s important to include related keyword variants into these areas (e.g. non-exact match keywords with matching SERP intent).
youtube
Project example: On-page text refresh/re-optimization
Project description: Locate keywords that are driving clicks and impressions to editorial pieces of content that DO NOT exist within the first paragraph within the body of the main content (MC). Perform an on-page refresh of introductory content within editorial pages to include high value keyword opportunities.
Project assumptions: This process assumes that inserting keywords into the first several sentences of a piece of content is a strong SEO practice for relevancy optimization, and that it’s important to include related keyword variants into these areas (e.g. non-exact match keywords with matching SERP intent).
youtube
Final thoughts
We hope this post has been helpful and opened you up to the idea of using Python and Google Colab to supercharge your relevancy optimization strategy.
As mentioned throughout the post, keep the following in mind:
Github repository will be updated with any changes we make in the future.
There is the possibility of undiscovered errors. If these occur, Inseev is happy to help! In fact, we would actually appreciate you reaching out to investigate and fix errors (if any do appear). This way others don’t run into the same problems.
Other than the above, if you have any ideas on ways to Colab (pun intended) on data analytics projects, feel free to reach out with ideas.
0 notes
Text
Page Level Query Analysis at Scale with Google Colab, Python, & the GSC API [Video Instructions Included]
The YouTube playlist referenced throughout this blog can be found here:6 Part YouTube Series [Setting Up & Using the Query Optimization Checker]
Anyone who does SEO as part of their job knows that there’s a lot of value in analyzing which queries are and are not sending traffic to specific pages on a site.
The most common uses for these datasets are to align on-page optimizations with existing rankings and traffic, and to identify gaps in ranking keywords.
However, working with this data is extremely tedious because it’s only available in the Google Search Console interface, and you have to look at only one page at a time.
On top of that, to get information on the text included in the ranking page, you either need to manually review it or extract it with a tool like Screaming Frog.
You need this kind of view:
…but even the above view would only be viable one page at a time, and as mentioned, the actual text extraction would have had to be separate as well.
Given these apparent issues with the readily available data at the SEO community’s disposal, the data engineering team at Inseev Interactive has been spending a lot of time thinking about how we can improve these processes at scale.
One specific example that we’ll be reviewing in this post is a simple script that allows you to get the above data in a flexible format for many great analytical views.
Better yet, this will all be available with only a few single input variables.
A quick rundown of tool functionality
The tool automatically compares the text on-page to the Google Search Console top queries at the page-level to let you know which queries are on-page as well as how many times they appear on the page. An optional XPath variable also allows you to specify the part of the page you want to analyze text on.
This means you’ll know exactly what queries are driving clicks/impressions that are not in your <title>, <h1>, or even something as specific as the first paragraph within the main content (MC). The sky's the limit.
For those of you not familiar, we’ve also provided some quick XPath expressions you can use, as well as how to create site-specific XPath expressions within the "Input Variables" section of the post.
Post setup usage & datasets
Once the process is set up, all that’s required is filling out a short list of variables and the rest is automated for you.
The output dataset includes multiple automated CSV datasets, as well as a structured file format to keep things organized. A simple pivot of the core analysis automated CSV can provide you with the below dataset and many other useful layouts.
… Even some "new metrics"?
Okay, not technically "new," but if you exclusively use the Google Search Console user interface, then you haven’t likely had access to metrics like these before: "Max Position," "Min Position," and "Count Position" for the specified date range – all of which are explained in the "Running your first analysis" section of the post.
To really demonstrate the impact and usefulness of this dataset, in the video below we use the Colab tool to:
[3 Minutes] — Find non-brand <title> optimization opportunities for https://www.inseev.com/ (around 30 pages in video, but you could do any number of pages)
[3 Minutes] — Convert the CSV to a more useable format
[1 Minute] – Optimize the first title with the resulting dataset
youtube
Okay, you’re all set for the initial rundown. Hopefully we were able to get you excited before moving into the somewhat dull setup process.
Keep in mind that at the end of the post, there is also a section including a few helpful use cases and an example template! To jump directly to each section of this post, please use the following links:
One-time setup of the script in Google Colab
Running your first analysis
Practical use cases and templates
[Quick Consideration #1] — The web scraper built into the tool DOES NOT support JavaScript rendering. If your website uses client-side rendering, the full functionality of the tool unfortunately will not work.
[Quick Consideration #2] — This tool has been heavily tested by the members of the Inseev team. Most bugs [specifically with the web scraper] have been found and fixed, but like any other program, it is possible that other issues may come up.
If you encounter any errors, feel free to reach out to us directly at [email protected] or [email protected], and either myself or one of the other members of the data engineering team at Inseev would be happy to help you out.
If new errors are encountered and fixed, we will always upload the updated script to the code repository linked in the sections below so the most up-to-date code can be utilized by all!
One-time setup of the script in Google Colab (in less than 20 minutes)
Things you’ll need:
Google Drive
Google Cloud Platform account
Google Search Console access
Video walkthrough: tool setup process
Below you’ll find step-by-step editorial instructions in order to set up the entire process. However, if following editorial instructions isn’t your preferred method, we recorded a video of the setup process as well.
As you’ll see, we start with a brand new Gmail and set up the entire process in approximately 12 minutes, and the output is completely worth the time.
youtube
Keep in mind that the setup is one-off, and once set up, the tool should work on command from there on!
Editorial walkthrough: tool setup process
Four-part process:
Download the files from Github and set up in Google Drive
Set up a Google Cloud Platform (GCP) Project (skip if you already have an account)
Create the OAuth 2.0 client ID for the Google Search Console (GSC) API (skip if you already have an OAuth client ID with the Search Console API enabled)
Add the OAuth 2.0 credentials to the Config.py file
Part one: Download the files from Github and set up in Google Drive
Download source files (no code required)
1. Navigate here.
2. Select "Code" > "Download Zip"
*You can also use 'git clone https://github.com/jmelm93/query-optmization-checker.git' if you’re more comfortable using the command prompt.
Initiate Google Colab in Google Drive
If you already have a Google Colaboratory setup in your Google Drive, feel free to skip this step.
1. Navigate here.
2. Click "New" > "More" > "Connect more apps".
3. Search "Colaboratory" > Click into the application page.
4. Click "Install" > "Continue" > Sign in with OAuth.
5. Click "OK" with the prompt checked so Google Drive automatically sets appropriate files to open with Google Colab (optional).
Import the downloaded folder to Google Drive & open in Colab
1. Navigate to Google Drive and create a folder called "Colab Notebooks".
IMPORTANT: The folder needs to be called "Colab Notebooks" as the script is configured to look for the "api" folder from within "Colab Notebooks".
Error resulting in improper folder naming.
2. Import the folder downloaded from Github into Google Drive.
At the end of this step, you should have a folder in your Google Drive that contains the below items:
Part two: Set up a Google Cloud Platform (GCP) project
If you already have a Google Cloud Platform (GCP) account, feel free to skip this part.
1. Navigate to the Google Cloud page.
2. Click on the "Get started for free" CTA (CTA text may change over time).
3. Sign in with the OAuth credentials of your choice. Any Gmail email will work.
4. Follow the prompts to sign up for your GCP account.
You’ll be asked to supply a credit card to sign up, but there is currently a $300 free trial and Google notes that they won’t charge you until you upgrade your account.
Part three: Create a 0Auth 2.0 client ID for the Google Search Console (GSC) API
1. Navigate here.
2. After you log in to your desired Google Cloud account, click "ENABLE".
3. Configure the consent screen.
In the consent screen creation process, select "External," then continue onto the "App Information."
Example below of minimum requirements:
Skip "Scopes"
Add the email(s) you’ll use for the Search Console API authentication into the "Test Users". There could be other emails versus just the one that owns the Google Drive. An example may be a client’s email where you access the Google Search Console UI to view their KPIs.
4. In the left-rail navigation, click into "Credentials" > "CREATE CREDENTIALS" > "OAuth Client ID" (Not in image).
5. Within the "Create OAuth client ID" form, fill in:
Application Type = Desktop app
Name = Google Colab
Click "CREATE"
6. Save the "Client ID" and "Client Secret" — as these will be added into the "api" folder config.py file from the Github files we downloaded.
These should have appeared in a popup after hitting "CREATE"
The "Client Secret" is functionally the password to your Google Cloud (DO NOT post this to the public/share it online)
Part four: Add the OAuth 2.0 credentials to the Config.py file
1. Return to Google Drive and navigate into the "api" folder.
2. Click into config.py.
3. Choose to open with "Text Editor" (or another app of your choice) to modify the config.py file.
4. Update the three areas highlighted below with your:
CLIENT_ID: From the OAuth 2.0 client ID setup process
CLIENT_SECRET: From the OAuth 2.0 client ID setup process
GOOGLE_CREDENTIALS: Email that corresponds with your CLIENT_ID & CLIENT_SECRET
5. Save the file once updated!
Congratulations, the boring stuff is over. You are now ready to start using the Google Colab file!
Running your first analysis
Running your first analysis may be a little intimidating, but stick with it and it will get easy fast.
Below, we’ve provided details regarding the input variables required, as well as notes on things to keep in mind when running the script and analyzing the resulting dataset.
After we walk through these items, there are also a few example projects and video walkthroughs showcasing ways to utilize these datasets for client deliverables.
Setting up the input variables
XPath extraction with the "xpath_selector" variable
Have you ever wanted to know every query driving clicks and impressions to a webpage that aren’t in your <title> or <h1> tag? Well, this parameter will allow you to do just that.
While optional, using this is highly encouraged and we feel it "supercharges" the analysis. Simply define site sections with Xpaths and the script will do the rest.
youtube
In the above video, you’ll find examples on how to create site specific extractions. In addition, below are some universal extractions that should work on almost any site on the web:
'//title' # Identifies a <title> tag
'//h1' # Identifies a <h1> tag
'//h2' # Identifies a <h2> tag
Site Specific: How to scrape only the main content (MC)?
Chaining Xpaths – Add a "|" Between Xpaths
'//title | //h1' # Gets you both the <title> and <h1> tag in 1 run
'//h1 | //h2 | //h3' # Gets you both the <h1>, <h2> and <h3> tags in 1 run
Other variables
Here’s a video overview of the other variables with a short description of each.
youtube
'colab_path' [Required] – The path in which the Colab file lives. This should be "/content/drive/My Drive/Colab Notebooks/".
'domain_lookup' [Required] – Homepage of the website utilized for analysis.
'startdate' & 'enddate' [Required] – Date range for the analysis period.
'gsc_sorting_field' [Required] – The tool pulls the top N pages as defined by the user. The "top" is defined by either "clicks_sum" or "impressions_sum." Please review the video for a more detailed description.
'gsc_limit_pages_number' [Required] – Numeric value that represents the number of resulting pages you’d like within the dataset.
'brand_exclusions' [Optional] – The string sequence(s) that commonly result in branded queries (e.g., anything containing "inseev" will be branded queries for "Inseev Interactive").
'impressions_exclusion' [Optional] – Numeric value used to exclude queries that are potentially irrelevant due to the lack of pre-existing impressions. This is primarily relevant for domains with strong pre-existing rankings on a large scale number of pages.
'page_inclusions' [Optional] – The string sequence(s) that are found within the desired analysis page type. If you’d like to analyze the entire domain, leave this section blank.
Running the script
Keep in mind that once the script finishes running, you’re generally going to use the "step3_query-optimizer_domain-YYYY-MM-DD.csv" file for analysis, but there are others with the raw datasets to browse as well.
Practical use cases for the "step3_query-optimizer_domain-YYYY-MM-DD.csv" file can be found in the "Practical use cases and templates" section.
That said, there are a few important things to note while testing things out:
1. No JavaScript Crawling: As mentioned at the start of the post, this script is NOT set up for JavaScript crawling, so if your target website uses a JS frontend with client-side rendering to populate the main content (MC), the scrape will not be useful. However, the basic functionality of quickly getting the top XX (user-defined) queries and pages can still be useful by itself.
2. Google Drive / GSC API Auth: The first time you run the script in each new session it will prompt you to authenticate both the Google Drive and the Google Search Console credentials.
Google Drive authentication: Authenticate to whatever email is associated with the Google Drive with the script.
GSC authentication: Authenticate whichever email has permission to use the desired Google Search Console account.
If you attempt to authenticate and you get an error that looks like the one below, please revisit the "Add the email(s) you’ll use the Colab app with into the 'Test Users'" from Part 3, step 3 in the process above: setting up the consent screen.
Quick tip: The Google Drive account and the GSC Authentication DO NOT have to be the same email, but they do require separate authentications with OAuth.
3. Running the script: Either navigate to "Runtime" > "Restart and Run All" or use the keyboard shortcut CTRL + fn9 to start running the script.
4. Populated datasets/folder structure: There are three CSVs populated by the script – all nested within a folder structure based on the "domain_lookup" input variable.
Automated Organization [Folders]: Each time you rerun the script on a new domain, it will create a new folder structure in order to keep things organized.
Automated Organization [File Naming]: The CSVs include the date of the export appended to the end, so you’ll always know when the process ran as well as the date range for the dataset.
5. Date range for dataset: Inside of the dataset there is a "gsc_datasetID" column generated, which includes the date range of the extraction.
6. Unfamiliar metrics: The resulting dataset has all the KPIs we know and love – e.g. clicks, impressions, average (mean) position — but there are also a few you cannot get directly from the GSC UI:
'count_instances_gsc' — the number of instances the query got at least 1 impression during the specified date range. Scenario example: GSC tells you that you were in an average position 6 for a large keyword like "flower delivery" and you only received 20 impressions in a 30-day date range. Doesn’t seem possible that you were really in position 6, right? Well, now you can see that was potentially because you only actually showed up on one day in that 30-day date range (e.g. count_instances_gsc = 1)
'max_position' & 'min_position' — the MAXIMUM and MINIMUM ranking position the identified page showed up for in Google Search within the specified date range.
Quick tip #1: Large variance in max/min may tell you that your keyword has been fluctuating heavily.
Quick tip #2: These KPIs, in conjunction with the "count_instances_gsc", can exponentially further your understanding of query performance and opportunity.
Practical use cases and templates
Access the recommended multi-use template.
Recommended use: Download file and use with Excel. Subjectively speaking, I believe Excel has a much more user friendly pivot table functionality in comparison to Google Sheets — which is critical for using this template.
Alternative use: If you do not have Microsoft Excel or you prefer a different tool, you can use most spreadsheet apps that contain pivot functionality.
For those who opt for an alternative spreadsheet software/app:
Below are the pivot fields to mimic upon setup.
You may have to adjust the Vlookup functions found on the "Step 3 _ Analysis Final Doc" tab, depending on whether your updated pivot columns align with the current pivot I’ve supplied.
Project example: Title & H1 re-optimizations (video walkthrough)
Project description: Locate keywords that are driving clicks and impressions to high value pages and that do not exist within the <title> and <h1> tags by reviewing GSC query KPIs vs. current page elements. Use the resulting findings to re-optimize both the <title> and <h1> tags for pre-existing pages.
Project assumptions: This process assumes that inserting keywords into both the <title> and <h1> tags is a strong SEO practice for relevancy optimization, and that it’s important to include related keyword variants into these areas (e.g. non-exact match keywords with matching SERP intent).
youtube
Project example: On-page text refresh/re-optimization
Project description: Locate keywords that are driving clicks and impressions to editorial pieces of content that DO NOT exist within the first paragraph within the body of the main content (MC). Perform an on-page refresh of introductory content within editorial pages to include high value keyword opportunities.
Project assumptions: This process assumes that inserting keywords into the first several sentences of a piece of content is a strong SEO practice for relevancy optimization, and that it’s important to include related keyword variants into these areas (e.g. non-exact match keywords with matching SERP intent).
youtube
Final thoughts
We hope this post has been helpful and opened you up to the idea of using Python and Google Colab to supercharge your relevancy optimization strategy.
As mentioned throughout the post, keep the following in mind:
Github repository will be updated with any changes we make in the future.
There is the possibility of undiscovered errors. If these occur, Inseev is happy to help! In fact, we would actually appreciate you reaching out to investigate and fix errors (if any do appear). This way others don’t run into the same problems.
Other than the above, if you have any ideas on ways to Colab (pun intended) on data analytics projects, feel free to reach out with ideas.
0 notes
Text
Page Level Query Analysis at Scale with Google Colab, Python, & the GSC API [Video Instructions Included]
The YouTube playlist referenced throughout this blog can be found here:6 Part YouTube Series [Setting Up & Using the Query Optimization Checker]
Anyone who does SEO as part of their job knows that there’s a lot of value in analyzing which queries are and are not sending traffic to specific pages on a site.
The most common uses for these datasets are to align on-page optimizations with existing rankings and traffic, and to identify gaps in ranking keywords.
However, working with this data is extremely tedious because it’s only available in the Google Search Console interface, and you have to look at only one page at a time.
On top of that, to get information on the text included in the ranking page, you either need to manually review it or extract it with a tool like Screaming Frog.
You need this kind of view:
…but even the above view would only be viable one page at a time, and as mentioned, the actual text extraction would have had to be separate as well.
Given these apparent issues with the readily available data at the SEO community’s disposal, the data engineering team at Inseev Interactive has been spending a lot of time thinking about how we can improve these processes at scale.
One specific example that we’ll be reviewing in this post is a simple script that allows you to get the above data in a flexible format for many great analytical views.
Better yet, this will all be available with only a few single input variables.
A quick rundown of tool functionality
The tool automatically compares the text on-page to the Google Search Console top queries at the page-level to let you know which queries are on-page as well as how many times they appear on the page. An optional XPath variable also allows you to specify the part of the page you want to analyze text on.
This means you’ll know exactly what queries are driving clicks/impressions that are not in your <title>, <h1>, or even something as specific as the first paragraph within the main content (MC). The sky's the limit.
For those of you not familiar, we’ve also provided some quick XPath expressions you can use, as well as how to create site-specific XPath expressions within the "Input Variables" section of the post.
Post setup usage & datasets
Once the process is set up, all that’s required is filling out a short list of variables and the rest is automated for you.
The output dataset includes multiple automated CSV datasets, as well as a structured file format to keep things organized. A simple pivot of the core analysis automated CSV can provide you with the below dataset and many other useful layouts.
… Even some "new metrics"?
Okay, not technically "new," but if you exclusively use the Google Search Console user interface, then you haven’t likely had access to metrics like these before: "Max Position," "Min Position," and "Count Position" for the specified date range – all of which are explained in the "Running your first analysis" section of the post.
To really demonstrate the impact and usefulness of this dataset, in the video below we use the Colab tool to:
[3 Minutes] — Find non-brand <title> optimization opportunities for https://www.inseev.com/ (around 30 pages in video, but you could do any number of pages)
[3 Minutes] — Convert the CSV to a more useable format
[1 Minute] – Optimize the first title with the resulting dataset
youtube
Okay, you’re all set for the initial rundown. Hopefully we were able to get you excited before moving into the somewhat dull setup process.
Keep in mind that at the end of the post, there is also a section including a few helpful use cases and an example template! To jump directly to each section of this post, please use the following links:
One-time setup of the script in Google Colab
Running your first analysis
Practical use cases and templates
[Quick Consideration #1] — The web scraper built into the tool DOES NOT support JavaScript rendering. If your website uses client-side rendering, the full functionality of the tool unfortunately will not work.
[Quick Consideration #2] — This tool has been heavily tested by the members of the Inseev team. Most bugs [specifically with the web scraper] have been found and fixed, but like any other program, it is possible that other issues may come up.
If you encounter any errors, feel free to reach out to us directly at [email protected] or [email protected], and either myself or one of the other members of the data engineering team at Inseev would be happy to help you out.
If new errors are encountered and fixed, we will always upload the updated script to the code repository linked in the sections below so the most up-to-date code can be utilized by all!
One-time setup of the script in Google Colab (in less than 20 minutes)
Things you’ll need:
Google Drive
Google Cloud Platform account
Google Search Console access
Video walkthrough: tool setup process
Below you’ll find step-by-step editorial instructions in order to set up the entire process. However, if following editorial instructions isn’t your preferred method, we recorded a video of the setup process as well.
As you’ll see, we start with a brand new Gmail and set up the entire process in approximately 12 minutes, and the output is completely worth the time.
youtube
Keep in mind that the setup is one-off, and once set up, the tool should work on command from there on!
Editorial walkthrough: tool setup process
Four-part process:
Download the files from Github and set up in Google Drive
Set up a Google Cloud Platform (GCP) Project (skip if you already have an account)
Create the OAuth 2.0 client ID for the Google Search Console (GSC) API (skip if you already have an OAuth client ID with the Search Console API enabled)
Add the OAuth 2.0 credentials to the Config.py file
Part one: Download the files from Github and set up in Google Drive
Download source files (no code required)
1. Navigate here.
2. Select "Code" > "Download Zip"
*You can also use 'git clone https://github.com/jmelm93/query-optmization-checker.git' if you’re more comfortable using the command prompt.
Initiate Google Colab in Google Drive
If you already have a Google Colaboratory setup in your Google Drive, feel free to skip this step.
1. Navigate here.
2. Click "New" > "More" > "Connect more apps".
3. Search "Colaboratory" > Click into the application page.
4. Click "Install" > "Continue" > Sign in with OAuth.
5. Click "OK" with the prompt checked so Google Drive automatically sets appropriate files to open with Google Colab (optional).
Import the downloaded folder to Google Drive & open in Colab
1. Navigate to Google Drive and create a folder called "Colab Notebooks".
IMPORTANT: The folder needs to be called "Colab Notebooks" as the script is configured to look for the "api" folder from within "Colab Notebooks".
Error resulting in improper folder naming.
2. Import the folder downloaded from Github into Google Drive.
At the end of this step, you should have a folder in your Google Drive that contains the below items:
Part two: Set up a Google Cloud Platform (GCP) project
If you already have a Google Cloud Platform (GCP) account, feel free to skip this part.
1. Navigate to the Google Cloud page.
2. Click on the "Get started for free" CTA (CTA text may change over time).
3. Sign in with the OAuth credentials of your choice. Any Gmail email will work.
4. Follow the prompts to sign up for your GCP account.
You’ll be asked to supply a credit card to sign up, but there is currently a $300 free trial and Google notes that they won’t charge you until you upgrade your account.
Part three: Create a 0Auth 2.0 client ID for the Google Search Console (GSC) API
1. Navigate here.
2. After you log in to your desired Google Cloud account, click "ENABLE".
3. Configure the consent screen.
In the consent screen creation process, select "External," then continue onto the "App Information."
Example below of minimum requirements:
Skip "Scopes"
Add the email(s) you’ll use for the Search Console API authentication into the "Test Users". There could be other emails versus just the one that owns the Google Drive. An example may be a client’s email where you access the Google Search Console UI to view their KPIs.
4. In the left-rail navigation, click into "Credentials" > "CREATE CREDENTIALS" > "OAuth Client ID" (Not in image).
5. Within the "Create OAuth client ID" form, fill in:
Application Type = Desktop app
Name = Google Colab
Click "CREATE"
6. Save the "Client ID" and "Client Secret" — as these will be added into the "api" folder config.py file from the Github files we downloaded.
These should have appeared in a popup after hitting "CREATE"
The "Client Secret" is functionally the password to your Google Cloud (DO NOT post this to the public/share it online)
Part four: Add the OAuth 2.0 credentials to the Config.py file
1. Return to Google Drive and navigate into the "api" folder.
2. Click into config.py.
3. Choose to open with "Text Editor" (or another app of your choice) to modify the config.py file.
4. Update the three areas highlighted below with your:
CLIENT_ID: From the OAuth 2.0 client ID setup process
CLIENT_SECRET: From the OAuth 2.0 client ID setup process
GOOGLE_CREDENTIALS: Email that corresponds with your CLIENT_ID & CLIENT_SECRET
5. Save the file once updated!
Congratulations, the boring stuff is over. You are now ready to start using the Google Colab file!
Running your first analysis
Running your first analysis may be a little intimidating, but stick with it and it will get easy fast.
Below, we’ve provided details regarding the input variables required, as well as notes on things to keep in mind when running the script and analyzing the resulting dataset.
After we walk through these items, there are also a few example projects and video walkthroughs showcasing ways to utilize these datasets for client deliverables.
Setting up the input variables
XPath extraction with the "xpath_selector" variable
Have you ever wanted to know every query driving clicks and impressions to a webpage that aren’t in your <title> or <h1> tag? Well, this parameter will allow you to do just that.
While optional, using this is highly encouraged and we feel it "supercharges" the analysis. Simply define site sections with Xpaths and the script will do the rest.
youtube
In the above video, you’ll find examples on how to create site specific extractions. In addition, below are some universal extractions that should work on almost any site on the web:
'//title' # Identifies a <title> tag
'//h1' # Identifies a <h1> tag
'//h2' # Identifies a <h2> tag
Site Specific: How to scrape only the main content (MC)?
Chaining Xpaths – Add a "|" Between Xpaths
'//title | //h1' # Gets you both the <title> and <h1> tag in 1 run
'//h1 | //h2 | //h3' # Gets you both the <h1>, <h2> and <h3> tags in 1 run
Other variables
Here’s a video overview of the other variables with a short description of each.
youtube
'colab_path' [Required] – The path in which the Colab file lives. This should be "/content/drive/My Drive/Colab Notebooks/".
'domain_lookup' [Required] – Homepage of the website utilized for analysis.
'startdate' & 'enddate' [Required] – Date range for the analysis period.
'gsc_sorting_field' [Required] – The tool pulls the top N pages as defined by the user. The "top" is defined by either "clicks_sum" or "impressions_sum." Please review the video for a more detailed description.
'gsc_limit_pages_number' [Required] – Numeric value that represents the number of resulting pages you’d like within the dataset.
'brand_exclusions' [Optional] – The string sequence(s) that commonly result in branded queries (e.g., anything containing "inseev" will be branded queries for "Inseev Interactive").
'impressions_exclusion' [Optional] – Numeric value used to exclude queries that are potentially irrelevant due to the lack of pre-existing impressions. This is primarily relevant for domains with strong pre-existing rankings on a large scale number of pages.
'page_inclusions' [Optional] – The string sequence(s) that are found within the desired analysis page type. If you’d like to analyze the entire domain, leave this section blank.
Running the script
Keep in mind that once the script finishes running, you’re generally going to use the "step3_query-optimizer_domain-YYYY-MM-DD.csv" file for analysis, but there are others with the raw datasets to browse as well.
Practical use cases for the "step3_query-optimizer_domain-YYYY-MM-DD.csv" file can be found in the "Practical use cases and templates" section.
That said, there are a few important things to note while testing things out:
1. No JavaScript Crawling: As mentioned at the start of the post, this script is NOT set up for JavaScript crawling, so if your target website uses a JS frontend with client-side rendering to populate the main content (MC), the scrape will not be useful. However, the basic functionality of quickly getting the top XX (user-defined) queries and pages can still be useful by itself.
2. Google Drive / GSC API Auth: The first time you run the script in each new session it will prompt you to authenticate both the Google Drive and the Google Search Console credentials.
Google Drive authentication: Authenticate to whatever email is associated with the Google Drive with the script.
GSC authentication: Authenticate whichever email has permission to use the desired Google Search Console account.
If you attempt to authenticate and you get an error that looks like the one below, please revisit the "Add the email(s) you’ll use the Colab app with into the 'Test Users'" from Part 3, step 3 in the process above: setting up the consent screen.
Quick tip: The Google Drive account and the GSC Authentication DO NOT have to be the same email, but they do require separate authentications with OAuth.
3. Running the script: Either navigate to "Runtime" > "Restart and Run All" or use the keyboard shortcut CTRL + fn9 to start running the script.
4. Populated datasets/folder structure: There are three CSVs populated by the script – all nested within a folder structure based on the "domain_lookup" input variable.
Automated Organization [Folders]: Each time you rerun the script on a new domain, it will create a new folder structure in order to keep things organized.
Automated Organization [File Naming]: The CSVs include the date of the export appended to the end, so you’ll always know when the process ran as well as the date range for the dataset.
5. Date range for dataset: Inside of the dataset there is a "gsc_datasetID" column generated, which includes the date range of the extraction.
6. Unfamiliar metrics: The resulting dataset has all the KPIs we know and love – e.g. clicks, impressions, average (mean) position — but there are also a few you cannot get directly from the GSC UI:
'count_instances_gsc' — the number of instances the query got at least 1 impression during the specified date range. Scenario example: GSC tells you that you were in an average position 6 for a large keyword like "flower delivery" and you only received 20 impressions in a 30-day date range. Doesn’t seem possible that you were really in position 6, right? Well, now you can see that was potentially because you only actually showed up on one day in that 30-day date range (e.g. count_instances_gsc = 1)
'max_position' & 'min_position' — the MAXIMUM and MINIMUM ranking position the identified page showed up for in Google Search within the specified date range.
Quick tip #1: Large variance in max/min may tell you that your keyword has been fluctuating heavily.
Quick tip #2: These KPIs, in conjunction with the "count_instances_gsc", can exponentially further your understanding of query performance and opportunity.
Practical use cases and templates
Access the recommended multi-use template.
Recommended use: Download file and use with Excel. Subjectively speaking, I believe Excel has a much more user friendly pivot table functionality in comparison to Google Sheets — which is critical for using this template.
Alternative use: If you do not have Microsoft Excel or you prefer a different tool, you can use most spreadsheet apps that contain pivot functionality.
For those who opt for an alternative spreadsheet software/app:
Below are the pivot fields to mimic upon setup.
You may have to adjust the Vlookup functions found on the "Step 3 _ Analysis Final Doc" tab, depending on whether your updated pivot columns align with the current pivot I’ve supplied.
Project example: Title & H1 re-optimizations (video walkthrough)
Project description: Locate keywords that are driving clicks and impressions to high value pages and that do not exist within the <title> and <h1> tags by reviewing GSC query KPIs vs. current page elements. Use the resulting findings to re-optimize both the <title> and <h1> tags for pre-existing pages.
Project assumptions: This process assumes that inserting keywords into both the <title> and <h1> tags is a strong SEO practice for relevancy optimization, and that it’s important to include related keyword variants into these areas (e.g. non-exact match keywords with matching SERP intent).
youtube
Project example: On-page text refresh/re-optimization
Project description: Locate keywords that are driving clicks and impressions to editorial pieces of content that DO NOT exist within the first paragraph within the body of the main content (MC). Perform an on-page refresh of introductory content within editorial pages to include high value keyword opportunities.
Project assumptions: This process assumes that inserting keywords into the first several sentences of a piece of content is a strong SEO practice for relevancy optimization, and that it’s important to include related keyword variants into these areas (e.g. non-exact match keywords with matching SERP intent).
youtube
Final thoughts
We hope this post has been helpful and opened you up to the idea of using Python and Google Colab to supercharge your relevancy optimization strategy.
As mentioned throughout the post, keep the following in mind:
Github repository will be updated with any changes we make in the future.
There is the possibility of undiscovered errors. If these occur, Inseev is happy to help! In fact, we would actually appreciate you reaching out to investigate and fix errors (if any do appear). This way others don’t run into the same problems.
Other than the above, if you have any ideas on ways to Colab (pun intended) on data analytics projects, feel free to reach out with ideas.
0 notes
Text
Page Level Query Analysis at Scale with Google Colab, Python, & the GSC API [Video Instructions Included]
The YouTube playlist referenced throughout this blog can be found here:6 Part YouTube Series [Setting Up & Using the Query Optimization Checker]
Anyone who does SEO as part of their job knows that there’s a lot of value in analyzing which queries are and are not sending traffic to specific pages on a site.
The most common uses for these datasets are to align on-page optimizations with existing rankings and traffic, and to identify gaps in ranking keywords.
However, working with this data is extremely tedious because it’s only available in the Google Search Console interface, and you have to look at only one page at a time.
On top of that, to get information on the text included in the ranking page, you either need to manually review it or extract it with a tool like Screaming Frog.
You need this kind of view:
…but even the above view would only be viable one page at a time, and as mentioned, the actual text extraction would have had to be separate as well.
Given these apparent issues with the readily available data at the SEO community’s disposal, the data engineering team at Inseev Interactive has been spending a lot of time thinking about how we can improve these processes at scale.
One specific example that we’ll be reviewing in this post is a simple script that allows you to get the above data in a flexible format for many great analytical views.
Better yet, this will all be available with only a few single input variables.
A quick rundown of tool functionality
The tool automatically compares the text on-page to the Google Search Console top queries at the page-level to let you know which queries are on-page as well as how many times they appear on the page. An optional XPath variable also allows you to specify the part of the page you want to analyze text on.
This means you’ll know exactly what queries are driving clicks/impressions that are not in your <title>, <h1>, or even something as specific as the first paragraph within the main content (MC). The sky's the limit.
For those of you not familiar, we’ve also provided some quick XPath expressions you can use, as well as how to create site-specific XPath expressions within the "Input Variables" section of the post.
Post setup usage & datasets
Once the process is set up, all that’s required is filling out a short list of variables and the rest is automated for you.
The output dataset includes multiple automated CSV datasets, as well as a structured file format to keep things organized. A simple pivot of the core analysis automated CSV can provide you with the below dataset and many other useful layouts.
… Even some "new metrics"?
Okay, not technically "new," but if you exclusively use the Google Search Console user interface, then you haven’t likely had access to metrics like these before: "Max Position," "Min Position," and "Count Position" for the specified date range – all of which are explained in the "Running your first analysis" section of the post.
To really demonstrate the impact and usefulness of this dataset, in the video below we use the Colab tool to:
[3 Minutes] — Find non-brand <title> optimization opportunities for https://www.inseev.com/ (around 30 pages in video, but you could do any number of pages)
[3 Minutes] — Convert the CSV to a more useable format
[1 Minute] – Optimize the first title with the resulting dataset
youtube
Okay, you’re all set for the initial rundown. Hopefully we were able to get you excited before moving into the somewhat dull setup process.
Keep in mind that at the end of the post, there is also a section including a few helpful use cases and an example template! To jump directly to each section of this post, please use the following links:
One-time setup of the script in Google Colab
Running your first analysis
Practical use cases and templates
[Quick Consideration #1] — The web scraper built into the tool DOES NOT support JavaScript rendering. If your website uses client-side rendering, the full functionality of the tool unfortunately will not work.
[Quick Consideration #2] — This tool has been heavily tested by the members of the Inseev team. Most bugs [specifically with the web scraper] have been found and fixed, but like any other program, it is possible that other issues may come up.
If you encounter any errors, feel free to reach out to us directly at [email protected] or [email protected], and either myself or one of the other members of the data engineering team at Inseev would be happy to help you out.
If new errors are encountered and fixed, we will always upload the updated script to the code repository linked in the sections below so the most up-to-date code can be utilized by all!
One-time setup of the script in Google Colab (in less than 20 minutes)
Things you’ll need:
Google Drive
Google Cloud Platform account
Google Search Console access
Video walkthrough: tool setup process
Below you’ll find step-by-step editorial instructions in order to set up the entire process. However, if following editorial instructions isn’t your preferred method, we recorded a video of the setup process as well.
As you’ll see, we start with a brand new Gmail and set up the entire process in approximately 12 minutes, and the output is completely worth the time.
youtube
Keep in mind that the setup is one-off, and once set up, the tool should work on command from there on!
Editorial walkthrough: tool setup process
Four-part process:
Download the files from Github and set up in Google Drive
Set up a Google Cloud Platform (GCP) Project (skip if you already have an account)
Create the OAuth 2.0 client ID for the Google Search Console (GSC) API (skip if you already have an OAuth client ID with the Search Console API enabled)
Add the OAuth 2.0 credentials to the Config.py file
Part one: Download the files from Github and set up in Google Drive
Download source files (no code required)
1. Navigate here.
2. Select "Code" > "Download Zip"
*You can also use 'git clone https://github.com/jmelm93/query-optmization-checker.git' if you’re more comfortable using the command prompt.
Initiate Google Colab in Google Drive
If you already have a Google Colaboratory setup in your Google Drive, feel free to skip this step.
1. Navigate here.
2. Click "New" > "More" > "Connect more apps".
3. Search "Colaboratory" > Click into the application page.
4. Click "Install" > "Continue" > Sign in with OAuth.
5. Click "OK" with the prompt checked so Google Drive automatically sets appropriate files to open with Google Colab (optional).
Import the downloaded folder to Google Drive & open in Colab
1. Navigate to Google Drive and create a folder called "Colab Notebooks".
IMPORTANT: The folder needs to be called "Colab Notebooks" as the script is configured to look for the "api" folder from within "Colab Notebooks".
Error resulting in improper folder naming.
2. Import the folder downloaded from Github into Google Drive.
At the end of this step, you should have a folder in your Google Drive that contains the below items:
Part two: Set up a Google Cloud Platform (GCP) project
If you already have a Google Cloud Platform (GCP) account, feel free to skip this part.
1. Navigate to the Google Cloud page.
2. Click on the "Get started for free" CTA (CTA text may change over time).
3. Sign in with the OAuth credentials of your choice. Any Gmail email will work.
4. Follow the prompts to sign up for your GCP account.
You’ll be asked to supply a credit card to sign up, but there is currently a $300 free trial and Google notes that they won’t charge you until you upgrade your account.
Part three: Create a 0Auth 2.0 client ID for the Google Search Console (GSC) API
1. Navigate here.
2. After you log in to your desired Google Cloud account, click "ENABLE".
3. Configure the consent screen.
In the consent screen creation process, select "External," then continue onto the "App Information."
Example below of minimum requirements:
Skip "Scopes"
Add the email(s) you’ll use for the Search Console API authentication into the "Test Users". There could be other emails versus just the one that owns the Google Drive. An example may be a client’s email where you access the Google Search Console UI to view their KPIs.
4. In the left-rail navigation, click into "Credentials" > "CREATE CREDENTIALS" > "OAuth Client ID" (Not in image).
5. Within the "Create OAuth client ID" form, fill in:
Application Type = Desktop app
Name = Google Colab
Click "CREATE"
6. Save the "Client ID" and "Client Secret" — as these will be added into the "api" folder config.py file from the Github files we downloaded.
These should have appeared in a popup after hitting "CREATE"
The "Client Secret" is functionally the password to your Google Cloud (DO NOT post this to the public/share it online)
Part four: Add the OAuth 2.0 credentials to the Config.py file
1. Return to Google Drive and navigate into the "api" folder.
2. Click into config.py.
3. Choose to open with "Text Editor" (or another app of your choice) to modify the config.py file.
4. Update the three areas highlighted below with your:
CLIENT_ID: From the OAuth 2.0 client ID setup process
CLIENT_SECRET: From the OAuth 2.0 client ID setup process
GOOGLE_CREDENTIALS: Email that corresponds with your CLIENT_ID & CLIENT_SECRET
5. Save the file once updated!
Congratulations, the boring stuff is over. You are now ready to start using the Google Colab file!
Running your first analysis
Running your first analysis may be a little intimidating, but stick with it and it will get easy fast.
Below, we’ve provided details regarding the input variables required, as well as notes on things to keep in mind when running the script and analyzing the resulting dataset.
After we walk through these items, there are also a few example projects and video walkthroughs showcasing ways to utilize these datasets for client deliverables.
Setting up the input variables
XPath extraction with the "xpath_selector" variable
Have you ever wanted to know every query driving clicks and impressions to a webpage that aren’t in your <title> or <h1> tag? Well, this parameter will allow you to do just that.
While optional, using this is highly encouraged and we feel it "supercharges" the analysis. Simply define site sections with Xpaths and the script will do the rest.
youtube
In the above video, you’ll find examples on how to create site specific extractions. In addition, below are some universal extractions that should work on almost any site on the web:
'//title' # Identifies a <title> tag
'//h1' # Identifies a <h1> tag
'//h2' # Identifies a <h2> tag
Site Specific: How to scrape only the main content (MC)?
Chaining Xpaths – Add a "|" Between Xpaths
'//title | //h1' # Gets you both the <title> and <h1> tag in 1 run
'//h1 | //h2 | //h3' # Gets you both the <h1>, <h2> and <h3> tags in 1 run
Other variables
Here’s a video overview of the other variables with a short description of each.
youtube
'colab_path' [Required] – The path in which the Colab file lives. This should be "/content/drive/My Drive/Colab Notebooks/".
'domain_lookup' [Required] – Homepage of the website utilized for analysis.
'startdate' & 'enddate' [Required] – Date range for the analysis period.
'gsc_sorting_field' [Required] – The tool pulls the top N pages as defined by the user. The "top" is defined by either "clicks_sum" or "impressions_sum." Please review the video for a more detailed description.
'gsc_limit_pages_number' [Required] – Numeric value that represents the number of resulting pages you’d like within the dataset.
'brand_exclusions' [Optional] – The string sequence(s) that commonly result in branded queries (e.g., anything containing "inseev" will be branded queries for "Inseev Interactive").
'impressions_exclusion' [Optional] – Numeric value used to exclude queries that are potentially irrelevant due to the lack of pre-existing impressions. This is primarily relevant for domains with strong pre-existing rankings on a large scale number of pages.
'page_inclusions' [Optional] – The string sequence(s) that are found within the desired analysis page type. If you’d like to analyze the entire domain, leave this section blank.
Running the script
Keep in mind that once the script finishes running, you’re generally going to use the "step3_query-optimizer_domain-YYYY-MM-DD.csv" file for analysis, but there are others with the raw datasets to browse as well.
Practical use cases for the "step3_query-optimizer_domain-YYYY-MM-DD.csv" file can be found in the "Practical use cases and templates" section.
That said, there are a few important things to note while testing things out:
1. No JavaScript Crawling: As mentioned at the start of the post, this script is NOT set up for JavaScript crawling, so if your target website uses a JS frontend with client-side rendering to populate the main content (MC), the scrape will not be useful. However, the basic functionality of quickly getting the top XX (user-defined) queries and pages can still be useful by itself.
2. Google Drive / GSC API Auth: The first time you run the script in each new session it will prompt you to authenticate both the Google Drive and the Google Search Console credentials.
Google Drive authentication: Authenticate to whatever email is associated with the Google Drive with the script.
GSC authentication: Authenticate whichever email has permission to use the desired Google Search Console account.
If you attempt to authenticate and you get an error that looks like the one below, please revisit the "Add the email(s) you’ll use the Colab app with into the 'Test Users'" from Part 3, step 3 in the process above: setting up the consent screen.
Quick tip: The Google Drive account and the GSC Authentication DO NOT have to be the same email, but they do require separate authentications with OAuth.
3. Running the script: Either navigate to "Runtime" > "Restart and Run All" or use the keyboard shortcut CTRL + fn9 to start running the script.
4. Populated datasets/folder structure: There are three CSVs populated by the script – all nested within a folder structure based on the "domain_lookup" input variable.
Automated Organization [Folders]: Each time you rerun the script on a new domain, it will create a new folder structure in order to keep things organized.
Automated Organization [File Naming]: The CSVs include the date of the export appended to the end, so you’ll always know when the process ran as well as the date range for the dataset.
5. Date range for dataset: Inside of the dataset there is a "gsc_datasetID" column generated, which includes the date range of the extraction.
6. Unfamiliar metrics: The resulting dataset has all the KPIs we know and love – e.g. clicks, impressions, average (mean) position — but there are also a few you cannot get directly from the GSC UI:
'count_instances_gsc' — the number of instances the query got at least 1 impression during the specified date range. Scenario example: GSC tells you that you were in an average position 6 for a large keyword like "flower delivery" and you only received 20 impressions in a 30-day date range. Doesn’t seem possible that you were really in position 6, right? Well, now you can see that was potentially because you only actually showed up on one day in that 30-day date range (e.g. count_instances_gsc = 1)
'max_position' & 'min_position' — the MAXIMUM and MINIMUM ranking position the identified page showed up for in Google Search within the specified date range.
Quick tip #1: Large variance in max/min may tell you that your keyword has been fluctuating heavily.
Quick tip #2: These KPIs, in conjunction with the "count_instances_gsc", can exponentially further your understanding of query performance and opportunity.
Practical use cases and templates
Access the recommended multi-use template.
Recommended use: Download file and use with Excel. Subjectively speaking, I believe Excel has a much more user friendly pivot table functionality in comparison to Google Sheets — which is critical for using this template.
Alternative use: If you do not have Microsoft Excel or you prefer a different tool, you can use most spreadsheet apps that contain pivot functionality.
For those who opt for an alternative spreadsheet software/app:
Below are the pivot fields to mimic upon setup.
You may have to adjust the Vlookup functions found on the "Step 3 _ Analysis Final Doc" tab, depending on whether your updated pivot columns align with the current pivot I’ve supplied.
Project example: Title & H1 re-optimizations (video walkthrough)
Project description: Locate keywords that are driving clicks and impressions to high value pages and that do not exist within the <title> and <h1> tags by reviewing GSC query KPIs vs. current page elements. Use the resulting findings to re-optimize both the <title> and <h1> tags for pre-existing pages.
Project assumptions: This process assumes that inserting keywords into both the <title> and <h1> tags is a strong SEO practice for relevancy optimization, and that it’s important to include related keyword variants into these areas (e.g. non-exact match keywords with matching SERP intent).
youtube
Project example: On-page text refresh/re-optimization
Project description: Locate keywords that are driving clicks and impressions to editorial pieces of content that DO NOT exist within the first paragraph within the body of the main content (MC). Perform an on-page refresh of introductory content within editorial pages to include high value keyword opportunities.
Project assumptions: This process assumes that inserting keywords into the first several sentences of a piece of content is a strong SEO practice for relevancy optimization, and that it’s important to include related keyword variants into these areas (e.g. non-exact match keywords with matching SERP intent).
youtube
Final thoughts
We hope this post has been helpful and opened you up to the idea of using Python and Google Colab to supercharge your relevancy optimization strategy.
As mentioned throughout the post, keep the following in mind:
Github repository will be updated with any changes we make in the future.
There is the possibility of undiscovered errors. If these occur, Inseev is happy to help! In fact, we would actually appreciate you reaching out to investigate and fix errors (if any do appear). This way others don’t run into the same problems.
Other than the above, if you have any ideas on ways to Colab (pun intended) on data analytics projects, feel free to reach out with ideas.
0 notes
Text
Page Level Query Analysis at Scale with Google Colab, Python, & the GSC API [Video Instructions Included]
The YouTube playlist referenced throughout this blog can be found here:6 Part YouTube Series [Setting Up & Using the Query Optimization Checker]
Anyone who does SEO as part of their job knows that there’s a lot of value in analyzing which queries are and are not sending traffic to specific pages on a site.
The most common uses for these datasets are to align on-page optimizations with existing rankings and traffic, and to identify gaps in ranking keywords.
However, working with this data is extremely tedious because it’s only available in the Google Search Console interface, and you have to look at only one page at a time.
On top of that, to get information on the text included in the ranking page, you either need to manually review it or extract it with a tool like Screaming Frog.
You need this kind of view:
…but even the above view would only be viable one page at a time, and as mentioned, the actual text extraction would have had to be separate as well.
Given these apparent issues with the readily available data at the SEO community’s disposal, the data engineering team at Inseev Interactive has been spending a lot of time thinking about how we can improve these processes at scale.
One specific example that we’ll be reviewing in this post is a simple script that allows you to get the above data in a flexible format for many great analytical views.
Better yet, this will all be available with only a few single input variables.
A quick rundown of tool functionality
The tool automatically compares the text on-page to the Google Search Console top queries at the page-level to let you know which queries are on-page as well as how many times they appear on the page. An optional XPath variable also allows you to specify the part of the page you want to analyze text on.
This means you’ll know exactly what queries are driving clicks/impressions that are not in your <title>, <h1>, or even something as specific as the first paragraph within the main content (MC). The sky's the limit.
For those of you not familiar, we’ve also provided some quick XPath expressions you can use, as well as how to create site-specific XPath expressions within the "Input Variables" section of the post.
Post setup usage & datasets
Once the process is set up, all that’s required is filling out a short list of variables and the rest is automated for you.
The output dataset includes multiple automated CSV datasets, as well as a structured file format to keep things organized. A simple pivot of the core analysis automated CSV can provide you with the below dataset and many other useful layouts.
… Even some "new metrics"?
Okay, not technically "new," but if you exclusively use the Google Search Console user interface, then you haven’t likely had access to metrics like these before: "Max Position," "Min Position," and "Count Position" for the specified date range – all of which are explained in the "Running your first analysis" section of the post.
To really demonstrate the impact and usefulness of this dataset, in the video below we use the Colab tool to:
[3 Minutes] — Find non-brand <title> optimization opportunities for https://www.inseev.com/ (around 30 pages in video, but you could do any number of pages)
[3 Minutes] — Convert the CSV to a more useable format
[1 Minute] – Optimize the first title with the resulting dataset
youtube
Okay, you’re all set for the initial rundown. Hopefully we were able to get you excited before moving into the somewhat dull setup process.
Keep in mind that at the end of the post, there is also a section including a few helpful use cases and an example template! To jump directly to each section of this post, please use the following links:
One-time setup of the script in Google Colab
Running your first analysis
Practical use cases and templates
[Quick Consideration #1] — The web scraper built into the tool DOES NOT support JavaScript rendering. If your website uses client-side rendering, the full functionality of the tool unfortunately will not work.
[Quick Consideration #2] — This tool has been heavily tested by the members of the Inseev team. Most bugs [specifically with the web scraper] have been found and fixed, but like any other program, it is possible that other issues may come up.
If you encounter any errors, feel free to reach out to us directly at [email protected] or [email protected], and either myself or one of the other members of the data engineering team at Inseev would be happy to help you out.
If new errors are encountered and fixed, we will always upload the updated script to the code repository linked in the sections below so the most up-to-date code can be utilized by all!
One-time setup of the script in Google Colab (in less than 20 minutes)
Things you’ll need:
Google Drive
Google Cloud Platform account
Google Search Console access
Video walkthrough: tool setup process
Below you’ll find step-by-step editorial instructions in order to set up the entire process. However, if following editorial instructions isn’t your preferred method, we recorded a video of the setup process as well.
As you’ll see, we start with a brand new Gmail and set up the entire process in approximately 12 minutes, and the output is completely worth the time.
youtube
Keep in mind that the setup is one-off, and once set up, the tool should work on command from there on!
Editorial walkthrough: tool setup process
Four-part process:
Download the files from Github and set up in Google Drive
Set up a Google Cloud Platform (GCP) Project (skip if you already have an account)
Create the OAuth 2.0 client ID for the Google Search Console (GSC) API (skip if you already have an OAuth client ID with the Search Console API enabled)
Add the OAuth 2.0 credentials to the Config.py file
Part one: Download the files from Github and set up in Google Drive
Download source files (no code required)
1. Navigate here.
2. Select "Code" > "Download Zip"
*You can also use 'git clone https://github.com/jmelm93/query-optmization-checker.git' if you’re more comfortable using the command prompt.
Initiate Google Colab in Google Drive
If you already have a Google Colaboratory setup in your Google Drive, feel free to skip this step.
1. Navigate here.
2. Click "New" > "More" > "Connect more apps".
3. Search "Colaboratory" > Click into the application page.
4. Click "Install" > "Continue" > Sign in with OAuth.
5. Click "OK" with the prompt checked so Google Drive automatically sets appropriate files to open with Google Colab (optional).
Import the downloaded folder to Google Drive & open in Colab
1. Navigate to Google Drive and create a folder called "Colab Notebooks".
IMPORTANT: The folder needs to be called "Colab Notebooks" as the script is configured to look for the "api" folder from within "Colab Notebooks".
Error resulting in improper folder naming.
2. Import the folder downloaded from Github into Google Drive.
At the end of this step, you should have a folder in your Google Drive that contains the below items:
Part two: Set up a Google Cloud Platform (GCP) project
If you already have a Google Cloud Platform (GCP) account, feel free to skip this part.
1. Navigate to the Google Cloud page.
2. Click on the "Get started for free" CTA (CTA text may change over time).
3. Sign in with the OAuth credentials of your choice. Any Gmail email will work.
4. Follow the prompts to sign up for your GCP account.
You’ll be asked to supply a credit card to sign up, but there is currently a $300 free trial and Google notes that they won’t charge you until you upgrade your account.
Part three: Create a 0Auth 2.0 client ID for the Google Search Console (GSC) API
1. Navigate here.
2. After you log in to your desired Google Cloud account, click "ENABLE".
3. Configure the consent screen.
In the consent screen creation process, select "External," then continue onto the "App Information."
Example below of minimum requirements:
Skip "Scopes"
Add the email(s) you’ll use for the Search Console API authentication into the "Test Users". There could be other emails versus just the one that owns the Google Drive. An example may be a client’s email where you access the Google Search Console UI to view their KPIs.
4. In the left-rail navigation, click into "Credentials" > "CREATE CREDENTIALS" > "OAuth Client ID" (Not in image).
5. Within the "Create OAuth client ID" form, fill in:
Application Type = Desktop app
Name = Google Colab
Click "CREATE"
6. Save the "Client ID" and "Client Secret" — as these will be added into the "api" folder config.py file from the Github files we downloaded.
These should have appeared in a popup after hitting "CREATE"
The "Client Secret" is functionally the password to your Google Cloud (DO NOT post this to the public/share it online)
Part four: Add the OAuth 2.0 credentials to the Config.py file
1. Return to Google Drive and navigate into the "api" folder.
2. Click into config.py.
3. Choose to open with "Text Editor" (or another app of your choice) to modify the config.py file.
4. Update the three areas highlighted below with your:
CLIENT_ID: From the OAuth 2.0 client ID setup process
CLIENT_SECRET: From the OAuth 2.0 client ID setup process
GOOGLE_CREDENTIALS: Email that corresponds with your CLIENT_ID & CLIENT_SECRET
5. Save the file once updated!
Congratulations, the boring stuff is over. You are now ready to start using the Google Colab file!
Running your first analysis
Running your first analysis may be a little intimidating, but stick with it and it will get easy fast.
Below, we’ve provided details regarding the input variables required, as well as notes on things to keep in mind when running the script and analyzing the resulting dataset.
After we walk through these items, there are also a few example projects and video walkthroughs showcasing ways to utilize these datasets for client deliverables.
Setting up the input variables
XPath extraction with the "xpath_selector" variable
Have you ever wanted to know every query driving clicks and impressions to a webpage that aren’t in your <title> or <h1> tag? Well, this parameter will allow you to do just that.
While optional, using this is highly encouraged and we feel it "supercharges" the analysis. Simply define site sections with Xpaths and the script will do the rest.
youtube
In the above video, you’ll find examples on how to create site specific extractions. In addition, below are some universal extractions that should work on almost any site on the web:
'//title' # Identifies a <title> tag
'//h1' # Identifies a <h1> tag
'//h2' # Identifies a <h2> tag
Site Specific: How to scrape only the main content (MC)?
Chaining Xpaths – Add a "|" Between Xpaths
'//title | //h1' # Gets you both the <title> and <h1> tag in 1 run
'//h1 | //h2 | //h3' # Gets you both the <h1>, <h2> and <h3> tags in 1 run
Other variables
Here’s a video overview of the other variables with a short description of each.
youtube
'colab_path' [Required] – The path in which the Colab file lives. This should be "/content/drive/My Drive/Colab Notebooks/".
'domain_lookup' [Required] – Homepage of the website utilized for analysis.
'startdate' & 'enddate' [Required] – Date range for the analysis period.
'gsc_sorting_field' [Required] – The tool pulls the top N pages as defined by the user. The "top" is defined by either "clicks_sum" or "impressions_sum." Please review the video for a more detailed description.
'gsc_limit_pages_number' [Required] – Numeric value that represents the number of resulting pages you’d like within the dataset.
'brand_exclusions' [Optional] – The string sequence(s) that commonly result in branded queries (e.g., anything containing "inseev" will be branded queries for "Inseev Interactive").
'impressions_exclusion' [Optional] – Numeric value used to exclude queries that are potentially irrelevant due to the lack of pre-existing impressions. This is primarily relevant for domains with strong pre-existing rankings on a large scale number of pages.
'page_inclusions' [Optional] – The string sequence(s) that are found within the desired analysis page type. If you’d like to analyze the entire domain, leave this section blank.
Running the script
Keep in mind that once the script finishes running, you���re generally going to use the "step3_query-optimizer_domain-YYYY-MM-DD.csv" file for analysis, but there are others with the raw datasets to browse as well.
Practical use cases for the "step3_query-optimizer_domain-YYYY-MM-DD.csv" file can be found in the "Practical use cases and templates" section.
That said, there are a few important things to note while testing things out:
1. No JavaScript Crawling: As mentioned at the start of the post, this script is NOT set up for JavaScript crawling, so if your target website uses a JS frontend with client-side rendering to populate the main content (MC), the scrape will not be useful. However, the basic functionality of quickly getting the top XX (user-defined) queries and pages can still be useful by itself.
2. Google Drive / GSC API Auth: The first time you run the script in each new session it will prompt you to authenticate both the Google Drive and the Google Search Console credentials.
Google Drive authentication: Authenticate to whatever email is associated with the Google Drive with the script.
GSC authentication: Authenticate whichever email has permission to use the desired Google Search Console account.
If you attempt to authenticate and you get an error that looks like the one below, please revisit the "Add the email(s) you’ll use the Colab app with into the 'Test Users'" from Part 3, step 3 in the process above: setting up the consent screen.
Quick tip: The Google Drive account and the GSC Authentication DO NOT have to be the same email, but they do require separate authentications with OAuth.
3. Running the script: Either navigate to "Runtime" > "Restart and Run All" or use the keyboard shortcut CTRL + fn9 to start running the script.
4. Populated datasets/folder structure: There are three CSVs populated by the script – all nested within a folder structure based on the "domain_lookup" input variable.
Automated Organization [Folders]: Each time you rerun the script on a new domain, it will create a new folder structure in order to keep things organized.
Automated Organization [File Naming]: The CSVs include the date of the export appended to the end, so you’ll always know when the process ran as well as the date range for the dataset.
5. Date range for dataset: Inside of the dataset there is a "gsc_datasetID" column generated, which includes the date range of the extraction.
6. Unfamiliar metrics: The resulting dataset has all the KPIs we know and love – e.g. clicks, impressions, average (mean) position — but there are also a few you cannot get directly from the GSC UI:
'count_instances_gsc' — the number of instances the query got at least 1 impression during the specified date range. Scenario example: GSC tells you that you were in an average position 6 for a large keyword like "flower delivery" and you only received 20 impressions in a 30-day date range. Doesn’t seem possible that you were really in position 6, right? Well, now you can see that was potentially because you only actually showed up on one day in that 30-day date range (e.g. count_instances_gsc = 1)
'max_position' & 'min_position' — the MAXIMUM and MINIMUM ranking position the identified page showed up for in Google Search within the specified date range.
Quick tip #1: Large variance in max/min may tell you that your keyword has been fluctuating heavily.
Quick tip #2: These KPIs, in conjunction with the "count_instances_gsc", can exponentially further your understanding of query performance and opportunity.
Practical use cases and templates
Access the recommended multi-use template.
Recommended use: Download file and use with Excel. Subjectively speaking, I believe Excel has a much more user friendly pivot table functionality in comparison to Google Sheets — which is critical for using this template.
Alternative use: If you do not have Microsoft Excel or you prefer a different tool, you can use most spreadsheet apps that contain pivot functionality.
For those who opt for an alternative spreadsheet software/app:
Below are the pivot fields to mimic upon setup.
You may have to adjust the Vlookup functions found on the "Step 3 _ Analysis Final Doc" tab, depending on whether your updated pivot columns align with the current pivot I’ve supplied.
Project example: Title & H1 re-optimizations (video walkthrough)
Project description: Locate keywords that are driving clicks and impressions to high value pages and that do not exist within the <title> and <h1> tags by reviewing GSC query KPIs vs. current page elements. Use the resulting findings to re-optimize both the <title> and <h1> tags for pre-existing pages.
Project assumptions: This process assumes that inserting keywords into both the <title> and <h1> tags is a strong SEO practice for relevancy optimization, and that it’s important to include related keyword variants into these areas (e.g. non-exact match keywords with matching SERP intent).
youtube
Project example: On-page text refresh/re-optimization
Project description: Locate keywords that are driving clicks and impressions to editorial pieces of content that DO NOT exist within the first paragraph within the body of the main content (MC). Perform an on-page refresh of introductory content within editorial pages to include high value keyword opportunities.
Project assumptions: This process assumes that inserting keywords into the first several sentences of a piece of content is a strong SEO practice for relevancy optimization, and that it’s important to include related keyword variants into these areas (e.g. non-exact match keywords with matching SERP intent).
youtube
Final thoughts
We hope this post has been helpful and opened you up to the idea of using Python and Google Colab to supercharge your relevancy optimization strategy.
As mentioned throughout the post, keep the following in mind:
Github repository will be updated with any changes we make in the future.
There is the possibility of undiscovered errors. If these occur, Inseev is happy to help! In fact, we would actually appreciate you reaching out to investigate and fix errors (if any do appear). This way others don’t run into the same problems.
Other than the above, if you have any ideas on ways to Colab (pun intended) on data analytics projects, feel free to reach out with ideas.
https://ift.tt/3uhS1Bo
0 notes
Text
Page Level Query Analysis at Scale with Google Colab, Python, & the GSC API [Video Instructions Included]
The YouTube playlist referenced throughout this blog can be found here:6 Part YouTube Series [Setting Up & Using the Query Optimization Checker]
Anyone who does SEO as part of their job knows that there’s a lot of value in analyzing which queries are and are not sending traffic to specific pages on a site.
The most common uses for these datasets are to align on-page optimizations with existing rankings and traffic, and to identify gaps in ranking keywords.
However, working with this data is extremely tedious because it’s only available in the Google Search Console interface, and you have to look at only one page at a time.
On top of that, to get information on the text included in the ranking page, you either need to manually review it or extract it with a tool like Screaming Frog.
You need this kind of view:
…but even the above view would only be viable one page at a time, and as mentioned, the actual text extraction would have had to be separate as well.
Given these apparent issues with the readily available data at the SEO community’s disposal, the data engineering team at Inseev Interactive has been spending a lot of time thinking about how we can improve these processes at scale.
One specific example that we’ll be reviewing in this post is a simple script that allows you to get the above data in a flexible format for many great analytical views.
Better yet, this will all be available with only a few single input variables.
A quick rundown of tool functionality
The tool automatically compares the text on-page to the Google Search Console top queries at the page-level to let you know which queries are on-page as well as how many times they appear on the page. An optional XPath variable also allows you to specify the part of the page you want to analyze text on.
This means you’ll know exactly what queries are driving clicks/impressions that are not in your <title>, <h1>, or even something as specific as the first paragraph within the main content (MC). The sky's the limit.
For those of you not familiar, we’ve also provided some quick XPath expressions you can use, as well as how to create site-specific XPath expressions within the "Input Variables" section of the post.
Post setup usage & datasets
Once the process is set up, all that’s required is filling out a short list of variables and the rest is automated for you.
The output dataset includes multiple automated CSV datasets, as well as a structured file format to keep things organized. A simple pivot of the core analysis automated CSV can provide you with the below dataset and many other useful layouts.
… Even some "new metrics"?
Okay, not technically "new," but if you exclusively use the Google Search Console user interface, then you haven’t likely had access to metrics like these before: "Max Position," "Min Position," and "Count Position" for the specified date range – all of which are explained in the "Running your first analysis" section of the post.
To really demonstrate the impact and usefulness of this dataset, in the video below we use the Colab tool to:
[3 Minutes] — Find non-brand <title> optimization opportunities for https://www.inseev.com/ (around 30 pages in video, but you could do any number of pages)
[3 Minutes] — Convert the CSV to a more useable format
[1 Minute] – Optimize the first title with the resulting dataset
youtube
Okay, you’re all set for the initial rundown. Hopefully we were able to get you excited before moving into the somewhat dull setup process.
Keep in mind that at the end of the post, there is also a section including a few helpful use cases and an example template! To jump directly to each section of this post, please use the following links:
One-time setup of the script in Google Colab
Running your first analysis
Practical use cases and templates
[Quick Consideration #1] — The web scraper built into the tool DOES NOT support JavaScript rendering. If your website uses client-side rendering, the full functionality of the tool unfortunately will not work.
[Quick Consideration #2] — This tool has been heavily tested by the members of the Inseev team. Most bugs [specifically with the web scraper] have been found and fixed, but like any other program, it is possible that other issues may come up.
If you encounter any errors, feel free to reach out to us directly at [email protected] or [email protected], and either myself or one of the other members of the data engineering team at Inseev would be happy to help you out.
If new errors are encountered and fixed, we will always upload the updated script to the code repository linked in the sections below so the most up-to-date code can be utilized by all!
One-time setup of the script in Google Colab (in less than 20 minutes)
Things you’ll need:
Google Drive
Google Cloud Platform account
Google Search Console access
Video walkthrough: tool setup process
Below you’ll find step-by-step editorial instructions in order to set up the entire process. However, if following editorial instructions isn’t your preferred method, we recorded a video of the setup process as well.
As you’ll see, we start with a brand new Gmail and set up the entire process in approximately 12 minutes, and the output is completely worth the time.
youtube
Keep in mind that the setup is one-off, and once set up, the tool should work on command from there on!
Editorial walkthrough: tool setup process
Four-part process:
Download the files from Github and set up in Google Drive
Set up a Google Cloud Platform (GCP) Project (skip if you already have an account)
Create the OAuth 2.0 client ID for the Google Search Console (GSC) API (skip if you already have an OAuth client ID with the Search Console API enabled)
Add the OAuth 2.0 credentials to the Config.py file
Part one: Download the files from Github and set up in Google Drive
Download source files (no code required)
1. Navigate here.
2. Select "Code" > "Download Zip"
*You can also use 'git clone https://github.com/jmelm93/query-optmization-checker.git' if you’re more comfortable using the command prompt.
Initiate Google Colab in Google Drive
If you already have a Google Colaboratory setup in your Google Drive, feel free to skip this step.
1. Navigate here.
2. Click "New" > "More" > "Connect more apps".
3. Search "Colaboratory" > Click into the application page.
4. Click "Install" > "Continue" > Sign in with OAuth.
5. Click "OK" with the prompt checked so Google Drive automatically sets appropriate files to open with Google Colab (optional).
Import the downloaded folder to Google Drive & open in Colab
1. Navigate to Google Drive and create a folder called "Colab Notebooks".
IMPORTANT: The folder needs to be called "Colab Notebooks" as the script is configured to look for the "api" folder from within "Colab Notebooks".
Error resulting in improper folder naming.
2. Import the folder downloaded from Github into Google Drive.
At the end of this step, you should have a folder in your Google Drive that contains the below items:
Part two: Set up a Google Cloud Platform (GCP) project
If you already have a Google Cloud Platform (GCP) account, feel free to skip this part.
1. Navigate to the Google Cloud page.
2. Click on the "Get started for free" CTA (CTA text may change over time).
3. Sign in with the OAuth credentials of your choice. Any Gmail email will work.
4. Follow the prompts to sign up for your GCP account.
You’ll be asked to supply a credit card to sign up, but there is currently a $300 free trial and Google notes that they won’t charge you until you upgrade your account.
Part three: Create a 0Auth 2.0 client ID for the Google Search Console (GSC) API
1. Navigate here.
2. After you log in to your desired Google Cloud account, click "ENABLE".
3. Configure the consent screen.
In the consent screen creation process, select "External," then continue onto the "App Information."
Example below of minimum requirements:
Skip "Scopes"
Add the email(s) you’ll use for the Search Console API authentication into the "Test Users". There could be other emails versus just the one that owns the Google Drive. An example may be a client’s email where you access the Google Search Console UI to view their KPIs.
4. In the left-rail navigation, click into "Credentials" > "CREATE CREDENTIALS" > "OAuth Client ID" (Not in image).
5. Within the "Create OAuth client ID" form, fill in:
Application Type = Desktop app
Name = Google Colab
Click "CREATE"
6. Save the "Client ID" and "Client Secret" — as these will be added into the "api" folder config.py file from the Github files we downloaded.
These should have appeared in a popup after hitting "CREATE"
The "Client Secret" is functionally the password to your Google Cloud (DO NOT post this to the public/share it online)
Part four: Add the OAuth 2.0 credentials to the Config.py file
1. Return to Google Drive and navigate into the "api" folder.
2. Click into config.py.
3. Choose to open with "Text Editor" (or another app of your choice) to modify the config.py file.
4. Update the three areas highlighted below with your:
CLIENT_ID: From the OAuth 2.0 client ID setup process
CLIENT_SECRET: From the OAuth 2.0 client ID setup process
GOOGLE_CREDENTIALS: Email that corresponds with your CLIENT_ID & CLIENT_SECRET
5. Save the file once updated!
Congratulations, the boring stuff is over. You are now ready to start using the Google Colab file!
Running your first analysis
Running your first analysis may be a little intimidating, but stick with it and it will get easy fast.
Below, we’ve provided details regarding the input variables required, as well as notes on things to keep in mind when running the script and analyzing the resulting dataset.
After we walk through these items, there are also a few example projects and video walkthroughs showcasing ways to utilize these datasets for client deliverables.
Setting up the input variables
XPath extraction with the "xpath_selector" variable
Have you ever wanted to know every query driving clicks and impressions to a webpage that aren’t in your <title> or <h1> tag? Well, this parameter will allow you to do just that.
While optional, using this is highly encouraged and we feel it "supercharges" the analysis. Simply define site sections with Xpaths and the script will do the rest.
youtube
In the above video, you’ll find examples on how to create site specific extractions. In addition, below are some universal extractions that should work on almost any site on the web:
'//title' # Identifies a <title> tag
'//h1' # Identifies a <h1> tag
'//h2' # Identifies a <h2> tag
Site Specific: How to scrape only the main content (MC)?
Chaining Xpaths – Add a "|" Between Xpaths
'//title | //h1' # Gets you both the <title> and <h1> tag in 1 run
'//h1 | //h2 | //h3' # Gets you both the <h1>, <h2> and <h3> tags in 1 run
Other variables
Here’s a video overview of the other variables with a short description of each.
youtube
'colab_path' [Required] – The path in which the Colab file lives. This should be "/content/drive/My Drive/Colab Notebooks/".
'domain_lookup' [Required] – Homepage of the website utilized for analysis.
'startdate' & 'enddate' [Required] – Date range for the analysis period.
'gsc_sorting_field' [Required] – The tool pulls the top N pages as defined by the user. The "top" is defined by either "clicks_sum" or "impressions_sum." Please review the video for a more detailed description.
'gsc_limit_pages_number' [Required] – Numeric value that represents the number of resulting pages you’d like within the dataset.
'brand_exclusions' [Optional] – The string sequence(s) that commonly result in branded queries (e.g., anything containing "inseev" will be branded queries for "Inseev Interactive").
'impressions_exclusion' [Optional] – Numeric value used to exclude queries that are potentially irrelevant due to the lack of pre-existing impressions. This is primarily relevant for domains with strong pre-existing rankings on a large scale number of pages.
'page_inclusions' [Optional] – The string sequence(s) that are found within the desired analysis page type. If you’d like to analyze the entire domain, leave this section blank.
Running the script
Keep in mind that once the script finishes running, you’re generally going to use the "step3_query-optimizer_domain-YYYY-MM-DD.csv" file for analysis, but there are others with the raw datasets to browse as well.
Practical use cases for the "step3_query-optimizer_domain-YYYY-MM-DD.csv" file can be found in the "Practical use cases and templates" section.
That said, there are a few important things to note while testing things out:
1. No JavaScript Crawling: As mentioned at the start of the post, this script is NOT set up for JavaScript crawling, so if your target website uses a JS frontend with client-side rendering to populate the main content (MC), the scrape will not be useful. However, the basic functionality of quickly getting the top XX (user-defined) queries and pages can still be useful by itself.
2. Google Drive / GSC API Auth: The first time you run the script in each new session it will prompt you to authenticate both the Google Drive and the Google Search Console credentials.
Google Drive authentication: Authenticate to whatever email is associated with the Google Drive with the script.
GSC authentication: Authenticate whichever email has permission to use the desired Google Search Console account.
If you attempt to authenticate and you get an error that looks like the one below, please revisit the "Add the email(s) you’ll use the Colab app with into the 'Test Users'" from Part 3, step 3 in the process above: setting up the consent screen.
Quick tip: The Google Drive account and the GSC Authentication DO NOT have to be the same email, but they do require separate authentications with OAuth.
3. Running the script: Either navigate to "Runtime" > "Restart and Run All" or use the keyboard shortcut CTRL + fn9 to start running the script.
4. Populated datasets/folder structure: There are three CSVs populated by the script – all nested within a folder structure based on the "domain_lookup" input variable.
Automated Organization [Folders]: Each time you rerun the script on a new domain, it will create a new folder structure in order to keep things organized.
Automated Organization [File Naming]: The CSVs include the date of the export appended to the end, so you’ll always know when the process ran as well as the date range for the dataset.
5. Date range for dataset: Inside of the dataset there is a "gsc_datasetID" column generated, which includes the date range of the extraction.
6. Unfamiliar metrics: The resulting dataset has all the KPIs we know and love – e.g. clicks, impressions, average (mean) position — but there are also a few you cannot get directly from the GSC UI:
'count_instances_gsc' — the number of instances the query got at least 1 impression during the specified date range. Scenario example: GSC tells you that you were in an average position 6 for a large keyword like "flower delivery" and you only received 20 impressions in a 30-day date range. Doesn’t seem possible that you were really in position 6, right? Well, now you can see that was potentially because you only actually showed up on one day in that 30-day date range (e.g. count_instances_gsc = 1)
'max_position' & 'min_position' — the MAXIMUM and MINIMUM ranking position the identified page showed up for in Google Search within the specified date range.
Quick tip #1: Large variance in max/min may tell you that your keyword has been fluctuating heavily.
Quick tip #2: These KPIs, in conjunction with the "count_instances_gsc", can exponentially further your understanding of query performance and opportunity.
Practical use cases and templates
Access the recommended multi-use template.
Recommended use: Download file and use with Excel. Subjectively speaking, I believe Excel has a much more user friendly pivot table functionality in comparison to Google Sheets — which is critical for using this template.
Alternative use: If you do not have Microsoft Excel or you prefer a different tool, you can use most spreadsheet apps that contain pivot functionality.
For those who opt for an alternative spreadsheet software/app:
Below are the pivot fields to mimic upon setup.
You may have to adjust the Vlookup functions found on the "Step 3 _ Analysis Final Doc" tab, depending on whether your updated pivot columns align with the current pivot I’ve supplied.
Project example: Title & H1 re-optimizations (video walkthrough)
Project description: Locate keywords that are driving clicks and impressions to high value pages and that do not exist within the <title> and <h1> tags by reviewing GSC query KPIs vs. current page elements. Use the resulting findings to re-optimize both the <title> and <h1> tags for pre-existing pages.
Project assumptions: This process assumes that inserting keywords into both the <title> and <h1> tags is a strong SEO practice for relevancy optimization, and that it’s important to include related keyword variants into these areas (e.g. non-exact match keywords with matching SERP intent).
youtube
Project example: On-page text refresh/re-optimization
Project description: Locate keywords that are driving clicks and impressions to editorial pieces of content that DO NOT exist within the first paragraph within the body of the main content (MC). Perform an on-page refresh of introductory content within editorial pages to include high value keyword opportunities.
Project assumptions: This process assumes that inserting keywords into the first several sentences of a piece of content is a strong SEO practice for relevancy optimization, and that it’s important to include related keyword variants into these areas (e.g. non-exact match keywords with matching SERP intent).
youtube
Final thoughts
We hope this post has been helpful and opened you up to the idea of using Python and Google Colab to supercharge your relevancy optimization strategy.
As mentioned throughout the post, keep the following in mind:
Github repository will be updated with any changes we make in the future.
There is the possibility of undiscovered errors. If these occur, Inseev is happy to help! In fact, we would actually appreciate you reaching out to investigate and fix errors (if any do appear). This way others don’t run into the same problems.
Other than the above, if you have any ideas on ways to Colab (pun intended) on data analytics projects, feel free to reach out with ideas.
0 notes
Text
Page Level Query Analysis at Scale with Google Colab, Python, & the GSC API [Video Instructions Included]
The YouTube playlist referenced throughout this blog can be found here:6 Part YouTube Series [Setting Up & Using the Query Optimization Checker]
Anyone who does SEO as part of their job knows that there’s a lot of value in analyzing which queries are and are not sending traffic to specific pages on a site.
The most common uses for these datasets are to align on-page optimizations with existing rankings and traffic, and to identify gaps in ranking keywords.
However, working with this data is extremely tedious because it’s only available in the Google Search Console interface, and you have to look at only one page at a time.
On top of that, to get information on the text included in the ranking page, you either need to manually review it or extract it with a tool like Screaming Frog.
You need this kind of view:
…but even the above view would only be viable one page at a time, and as mentioned, the actual text extraction would have had to be separate as well.
Given these apparent issues with the readily available data at the SEO community’s disposal, the data engineering team at Inseev Interactive has been spending a lot of time thinking about how we can improve these processes at scale.
One specific example that we’ll be reviewing in this post is a simple script that allows you to get the above data in a flexible format for many great analytical views.
Better yet, this will all be available with only a few single input variables.
A quick rundown of tool functionality
The tool automatically compares the text on-page to the Google Search Console top queries at the page-level to let you know which queries are on-page as well as how many times they appear on the page. An optional XPath variable also allows you to specify the part of the page you want to analyze text on.
This means you’ll know exactly what queries are driving clicks/impressions that are not in your <title>, <h1>, or even something as specific as the first paragraph within the main content (MC). The sky's the limit.
For those of you not familiar, we’ve also provided some quick XPath expressions you can use, as well as how to create site-specific XPath expressions within the "Input Variables" section of the post.
Post setup usage & datasets
Once the process is set up, all that’s required is filling out a short list of variables and the rest is automated for you.
The output dataset includes multiple automated CSV datasets, as well as a structured file format to keep things organized. A simple pivot of the core analysis automated CSV can provide you with the below dataset and many other useful layouts.
… Even some "new metrics"?
Okay, not technically "new," but if you exclusively use the Google Search Console user interface, then you haven’t likely had access to metrics like these before: "Max Position," "Min Position," and "Count Position" for the specified date range – all of which are explained in the "Running your first analysis" section of the post.
To really demonstrate the impact and usefulness of this dataset, in the video below we use the Colab tool to:
[3 Minutes] — Find non-brand <title> optimization opportunities for https://www.inseev.com/ (around 30 pages in video, but you could do any number of pages)
[3 Minutes] — Convert the CSV to a more useable format
[1 Minute] – Optimize the first title with the resulting dataset
youtube
Okay, you’re all set for the initial rundown. Hopefully we were able to get you excited before moving into the somewhat dull setup process.
Keep in mind that at the end of the post, there is also a section including a few helpful use cases and an example template! To jump directly to each section of this post, please use the following links:
One-time setup of the script in Google Colab
Running your first analysis
Practical use cases and templates
[Quick Consideration #1] — The web scraper built into the tool DOES NOT support JavaScript rendering. If your website uses client-side rendering, the full functionality of the tool unfortunately will not work.
[Quick Consideration #2] — This tool has been heavily tested by the members of the Inseev team. Most bugs [specifically with the web scraper] have been found and fixed, but like any other program, it is possible that other issues may come up.
If you encounter any errors, feel free to reach out to us directly at [email protected] or [email protected], and either myself or one of the other members of the data engineering team at Inseev would be happy to help you out.
If new errors are encountered and fixed, we will always upload the updated script to the code repository linked in the sections below so the most up-to-date code can be utilized by all!
One-time setup of the script in Google Colab (in less than 20 minutes)
Things you’ll need:
Google Drive
Google Cloud Platform account
Google Search Console access
Video walkthrough: tool setup process
Below you’ll find step-by-step editorial instructions in order to set up the entire process. However, if following editorial instructions isn’t your preferred method, we recorded a video of the setup process as well.
As you’ll see, we start with a brand new Gmail and set up the entire process in approximately 12 minutes, and the output is completely worth the time.
youtube
Keep in mind that the setup is one-off, and once set up, the tool should work on command from there on!
Editorial walkthrough: tool setup process
Four-part process:
Download the files from Github and set up in Google Drive
Set up a Google Cloud Platform (GCP) Project (skip if you already have an account)
Create the OAuth 2.0 client ID for the Google Search Console (GSC) API (skip if you already have an OAuth client ID with the Search Console API enabled)
Add the OAuth 2.0 credentials to the Config.py file
Part one: Download the files from Github and set up in Google Drive
Download source files (no code required)
1. Navigate here.
2. Select "Code" > "Download Zip"
*You can also use 'git clone https://github.com/jmelm93/query-optmization-checker.git' if you’re more comfortable using the command prompt.
Initiate Google Colab in Google Drive
If you already have a Google Colaboratory setup in your Google Drive, feel free to skip this step.
1. Navigate here.
2. Click "New" > "More" > "Connect more apps".
3. Search "Colaboratory" > Click into the application page.
4. Click "Install" > "Continue" > Sign in with OAuth.
5. Click "OK" with the prompt checked so Google Drive automatically sets appropriate files to open with Google Colab (optional).
Import the downloaded folder to Google Drive & open in Colab
1. Navigate to Google Drive and create a folder called "Colab Notebooks".
IMPORTANT: The folder needs to be called "Colab Notebooks" as the script is configured to look for the "api" folder from within "Colab Notebooks".
Error resulting in improper folder naming.
2. Import the folder downloaded from Github into Google Drive.
At the end of this step, you should have a folder in your Google Drive that contains the below items:
Part two: Set up a Google Cloud Platform (GCP) project
If you already have a Google Cloud Platform (GCP) account, feel free to skip this part.
1. Navigate to the Google Cloud page.
2. Click on the "Get started for free" CTA (CTA text may change over time).
3. Sign in with the OAuth credentials of your choice. Any Gmail email will work.
4. Follow the prompts to sign up for your GCP account.
You’ll be asked to supply a credit card to sign up, but there is currently a $300 free trial and Google notes that they won’t charge you until you upgrade your account.
Part three: Create a 0Auth 2.0 client ID for the Google Search Console (GSC) API
1. Navigate here.
2. After you log in to your desired Google Cloud account, click "ENABLE".
3. Configure the consent screen.
In the consent screen creation process, select "External," then continue onto the "App Information."
Example below of minimum requirements:
Skip "Scopes"
Add the email(s) you’ll use for the Search Console API authentication into the "Test Users". There could be other emails versus just the one that owns the Google Drive. An example may be a client’s email where you access the Google Search Console UI to view their KPIs.
4. In the left-rail navigation, click into "Credentials" > "CREATE CREDENTIALS" > "OAuth Client ID" (Not in image).
5. Within the "Create OAuth client ID" form, fill in:
Application Type = Desktop app
Name = Google Colab
Click "CREATE"
6. Save the "Client ID" and "Client Secret" — as these will be added into the "api" folder config.py file from the Github files we downloaded.
These should have appeared in a popup after hitting "CREATE"
The "Client Secret" is functionally the password to your Google Cloud (DO NOT post this to the public/share it online)
Part four: Add the OAuth 2.0 credentials to the Config.py file
1. Return to Google Drive and navigate into the "api" folder.
2. Click into config.py.
3. Choose to open with "Text Editor" (or another app of your choice) to modify the config.py file.
4. Update the three areas highlighted below with your:
CLIENT_ID: From the OAuth 2.0 client ID setup process
CLIENT_SECRET: From the OAuth 2.0 client ID setup process
GOOGLE_CREDENTIALS: Email that corresponds with your CLIENT_ID & CLIENT_SECRET
5. Save the file once updated!
Congratulations, the boring stuff is over. You are now ready to start using the Google Colab file!
Running your first analysis
Running your first analysis may be a little intimidating, but stick with it and it will get easy fast.
Below, we’ve provided details regarding the input variables required, as well as notes on things to keep in mind when running the script and analyzing the resulting dataset.
After we walk through these items, there are also a few example projects and video walkthroughs showcasing ways to utilize these datasets for client deliverables.
Setting up the input variables
XPath extraction with the "xpath_selector" variable
Have you ever wanted to know every query driving clicks and impressions to a webpage that aren’t in your <title> or <h1> tag? Well, this parameter will allow you to do just that.
While optional, using this is highly encouraged and we feel it "supercharges" the analysis. Simply define site sections with Xpaths and the script will do the rest.
youtube
In the above video, you’ll find examples on how to create site specific extractions. In addition, below are some universal extractions that should work on almost any site on the web:
'//title' # Identifies a <title> tag
'//h1' # Identifies a <h1> tag
'//h2' # Identifies a <h2> tag
Site Specific: How to scrape only the main content (MC)?
Chaining Xpaths – Add a "|" Between Xpaths
'//title | //h1' # Gets you both the <title> and <h1> tag in 1 run
'//h1 | //h2 | //h3' # Gets you both the <h1>, <h2> and <h3> tags in 1 run
Other variables
Here’s a video overview of the other variables with a short description of each.
youtube
'colab_path' [Required] – The path in which the Colab file lives. This should be "/content/drive/My Drive/Colab Notebooks/".
'domain_lookup' [Required] – Homepage of the website utilized for analysis.
'startdate' & 'enddate' [Required] – Date range for the analysis period.
'gsc_sorting_field' [Required] – The tool pulls the top N pages as defined by the user. The "top" is defined by either "clicks_sum" or "impressions_sum." Please review the video for a more detailed description.
'gsc_limit_pages_number' [Required] – Numeric value that represents the number of resulting pages you’d like within the dataset.
'brand_exclusions' [Optional] – The string sequence(s) that commonly result in branded queries (e.g., anything containing "inseev" will be branded queries for "Inseev Interactive").
'impressions_exclusion' [Optional] – Numeric value used to exclude queries that are potentially irrelevant due to the lack of pre-existing impressions. This is primarily relevant for domains with strong pre-existing rankings on a large scale number of pages.
'page_inclusions' [Optional] – The string sequence(s) that are found within the desired analysis page type. If you’d like to analyze the entire domain, leave this section blank.
Running the script
Keep in mind that once the script finishes running, you’re generally going to use the "step3_query-optimizer_domain-YYYY-MM-DD.csv" file for analysis, but there are others with the raw datasets to browse as well.
Practical use cases for the "step3_query-optimizer_domain-YYYY-MM-DD.csv" file can be found in the "Practical use cases and templates" section.
That said, there are a few important things to note while testing things out:
1. No JavaScript Crawling: As mentioned at the start of the post, this script is NOT set up for JavaScript crawling, so if your target website uses a JS frontend with client-side rendering to populate the main content (MC), the scrape will not be useful. However, the basic functionality of quickly getting the top XX (user-defined) queries and pages can still be useful by itself.
2. Google Drive / GSC API Auth: The first time you run the script in each new session it will prompt you to authenticate both the Google Drive and the Google Search Console credentials.
Google Drive authentication: Authenticate to whatever email is associated with the Google Drive with the script.
GSC authentication: Authenticate whichever email has permission to use the desired Google Search Console account.
If you attempt to authenticate and you get an error that looks like the one below, please revisit the "Add the email(s) you’ll use the Colab app with into the 'Test Users'" from Part 3, step 3 in the process above: setting up the consent screen.
Quick tip: The Google Drive account and the GSC Authentication DO NOT have to be the same email, but they do require separate authentications with OAuth.
3. Running the script: Either navigate to "Runtime" > "Restart and Run All" or use the keyboard shortcut CTRL + fn9 to start running the script.
4. Populated datasets/folder structure: There are three CSVs populated by the script – all nested within a folder structure based on the "domain_lookup" input variable.
Automated Organization [Folders]: Each time you rerun the script on a new domain, it will create a new folder structure in order to keep things organized.
Automated Organization [File Naming]: The CSVs include the date of the export appended to the end, so you’ll always know when the process ran as well as the date range for the dataset.
5. Date range for dataset: Inside of the dataset there is a "gsc_datasetID" column generated, which includes the date range of the extraction.
6. Unfamiliar metrics: The resulting dataset has all the KPIs we know and love – e.g. clicks, impressions, average (mean) position — but there are also a few you cannot get directly from the GSC UI:
'count_instances_gsc' — the number of instances the query got at least 1 impression during the specified date range. Scenario example: GSC tells you that you were in an average position 6 for a large keyword like "flower delivery" and you only received 20 impressions in a 30-day date range. Doesn’t seem possible that you were really in position 6, right? Well, now you can see that was potentially because you only actually showed up on one day in that 30-day date range (e.g. count_instances_gsc = 1)
'max_position' & 'min_position' — the MAXIMUM and MINIMUM ranking position the identified page showed up for in Google Search within the specified date range.
Quick tip #1: Large variance in max/min may tell you that your keyword has been fluctuating heavily.
Quick tip #2: These KPIs, in conjunction with the "count_instances_gsc", can exponentially further your understanding of query performance and opportunity.
Practical use cases and templates
Access the recommended multi-use template.
Recommended use: Download file and use with Excel. Subjectively speaking, I believe Excel has a much more user friendly pivot table functionality in comparison to Google Sheets — which is critical for using this template.
Alternative use: If you do not have Microsoft Excel or you prefer a different tool, you can use most spreadsheet apps that contain pivot functionality.
For those who opt for an alternative spreadsheet software/app:
Below are the pivot fields to mimic upon setup.
You may have to adjust the Vlookup functions found on the "Step 3 _ Analysis Final Doc" tab, depending on whether your updated pivot columns align with the current pivot I’ve supplied.
Project example: Title & H1 re-optimizations (video walkthrough)
Project description: Locate keywords that are driving clicks and impressions to high value pages and that do not exist within the <title> and <h1> tags by reviewing GSC query KPIs vs. current page elements. Use the resulting findings to re-optimize both the <title> and <h1> tags for pre-existing pages.
Project assumptions: This process assumes that inserting keywords into both the <title> and <h1> tags is a strong SEO practice for relevancy optimization, and that it’s important to include related keyword variants into these areas (e.g. non-exact match keywords with matching SERP intent).
youtube
Project example: On-page text refresh/re-optimization
Project description: Locate keywords that are driving clicks and impressions to editorial pieces of content that DO NOT exist within the first paragraph within the body of the main content (MC). Perform an on-page refresh of introductory content within editorial pages to include high value keyword opportunities.
Project assumptions: This process assumes that inserting keywords into the first several sentences of a piece of content is a strong SEO practice for relevancy optimization, and that it’s important to include related keyword variants into these areas (e.g. non-exact match keywords with matching SERP intent).
youtube
Final thoughts
We hope this post has been helpful and opened you up to the idea of using Python and Google Colab to supercharge your relevancy optimization strategy.
As mentioned throughout the post, keep the following in mind:
Github repository will be updated with any changes we make in the future.
There is the possibility of undiscovered errors. If these occur, Inseev is happy to help! In fact, we would actually appreciate you reaching out to investigate and fix errors (if any do appear). This way others don’t run into the same problems.
Other than the above, if you have any ideas on ways to Colab (pun intended) on data analytics projects, feel free to reach out with ideas.
0 notes
Text
Page Level Query Analysis at Scale with Google Colab, Python, & the GSC API [Video Instructions Included]
The YouTube playlist referenced throughout this blog can be found here:6 Part YouTube Series [Setting Up & Using the Query Optimization Checker]
Anyone who does SEO as part of their job knows that there’s a lot of value in analyzing which queries are and are not sending traffic to specific pages on a site.
The most common uses for these datasets are to align on-page optimizations with existing rankings and traffic, and to identify gaps in ranking keywords.
However, working with this data is extremely tedious because it’s only available in the Google Search Console interface, and you have to look at only one page at a time.
On top of that, to get information on the text included in the ranking page, you either need to manually review it or extract it with a tool like Screaming Frog.
You need this kind of view:
…but even the above view would only be viable one page at a time, and as mentioned, the actual text extraction would have had to be separate as well.
Given these apparent issues with the readily available data at the SEO community’s disposal, the data engineering team at Inseev Interactive has been spending a lot of time thinking about how we can improve these processes at scale.
One specific example that we’ll be reviewing in this post is a simple script that allows you to get the above data in a flexible format for many great analytical views.
Better yet, this will all be available with only a few single input variables.
A quick rundown of tool functionality
The tool automatically compares the text on-page to the Google Search Console top queries at the page-level to let you know which queries are on-page as well as how many times they appear on the page. An optional XPath variable also allows you to specify the part of the page you want to analyze text on.
This means you’ll know exactly what queries are driving clicks/impressions that are not in your <title>, <h1>, or even something as specific as the first paragraph within the main content (MC). The sky's the limit.
For those of you not familiar, we’ve also provided some quick XPath expressions you can use, as well as how to create site-specific XPath expressions within the "Input Variables" section of the post.
Post setup usage & datasets
Once the process is set up, all that’s required is filling out a short list of variables and the rest is automated for you.
The output dataset includes multiple automated CSV datasets, as well as a structured file format to keep things organized. A simple pivot of the core analysis automated CSV can provide you with the below dataset and many other useful layouts.
… Even some "new metrics"?
Okay, not technically "new," but if you exclusively use the Google Search Console user interface, then you haven’t likely had access to metrics like these before: "Max Position," "Min Position," and "Count Position" for the specified date range – all of which are explained in the "Running your first analysis" section of the post.
To really demonstrate the impact and usefulness of this dataset, in the video below we use the Colab tool to:
[3 Minutes] — Find non-brand <title> optimization opportunities for https://www.inseev.com/ (around 30 pages in video, but you could do any number of pages)
[3 Minutes] — Convert the CSV to a more useable format
[1 Minute] – Optimize the first title with the resulting dataset
youtube
Okay, you’re all set for the initial rundown. Hopefully we were able to get you excited before moving into the somewhat dull setup process.
Keep in mind that at the end of the post, there is also a section including a few helpful use cases and an example template! To jump directly to each section of this post, please use the following links:
One-time setup of the script in Google Colab
Running your first analysis
Practical use cases and templates
[Quick Consideration #1] — The web scraper built into the tool DOES NOT support JavaScript rendering. If your website uses client-side rendering, the full functionality of the tool unfortunately will not work.
[Quick Consideration #2] — This tool has been heavily tested by the members of the Inseev team. Most bugs [specifically with the web scraper] have been found and fixed, but like any other program, it is possible that other issues may come up.
If you encounter any errors, feel free to reach out to us directly at [email protected] or [email protected], and either myself or one of the other members of the data engineering team at Inseev would be happy to help you out.
If new errors are encountered and fixed, we will always upload the updated script to the code repository linked in the sections below so the most up-to-date code can be utilized by all!
One-time setup of the script in Google Colab (in less than 20 minutes)
Things you’ll need:
Google Drive
Google Cloud Platform account
Google Search Console access
Video walkthrough: tool setup process
Below you’ll find step-by-step editorial instructions in order to set up the entire process. However, if following editorial instructions isn’t your preferred method, we recorded a video of the setup process as well.
As you’ll see, we start with a brand new Gmail and set up the entire process in approximately 12 minutes, and the output is completely worth the time.
youtube
Keep in mind that the setup is one-off, and once set up, the tool should work on command from there on!
Editorial walkthrough: tool setup process
Four-part process:
Download the files from Github and set up in Google Drive
Set up a Google Cloud Platform (GCP) Project (skip if you already have an account)
Create the OAuth 2.0 client ID for the Google Search Console (GSC) API (skip if you already have an OAuth client ID with the Search Console API enabled)
Add the OAuth 2.0 credentials to the Config.py file
Part one: Download the files from Github and set up in Google Drive
Download source files (no code required)
1. Navigate here.
2. Select "Code" > "Download Zip"
*You can also use 'git clone https://github.com/jmelm93/query-optmization-checker.git' if you’re more comfortable using the command prompt.
Initiate Google Colab in Google Drive
If you already have a Google Colaboratory setup in your Google Drive, feel free to skip this step.
1. Navigate here.
2. Click "New" > "More" > "Connect more apps".
3. Search "Colaboratory" > Click into the application page.
4. Click "Install" > "Continue" > Sign in with OAuth.
5. Click "OK" with the prompt checked so Google Drive automatically sets appropriate files to open with Google Colab (optional).
Import the downloaded folder to Google Drive & open in Colab
1. Navigate to Google Drive and create a folder called "Colab Notebooks".
IMPORTANT: The folder needs to be called "Colab Notebooks" as the script is configured to look for the "api" folder from within "Colab Notebooks".
Error resulting in improper folder naming.
2. Import the folder downloaded from Github into Google Drive.
At the end of this step, you should have a folder in your Google Drive that contains the below items:
Part two: Set up a Google Cloud Platform (GCP) project
If you already have a Google Cloud Platform (GCP) account, feel free to skip this part.
1. Navigate to the Google Cloud page.
2. Click on the "Get started for free" CTA (CTA text may change over time).
3. Sign in with the OAuth credentials of your choice. Any Gmail email will work.
4. Follow the prompts to sign up for your GCP account.
You’ll be asked to supply a credit card to sign up, but there is currently a $300 free trial and Google notes that they won’t charge you until you upgrade your account.
Part three: Create a 0Auth 2.0 client ID for the Google Search Console (GSC) API
1. Navigate here.
2. After you log in to your desired Google Cloud account, click "ENABLE".
3. Configure the consent screen.
In the consent screen creation process, select "External," then continue onto the "App Information."
Example below of minimum requirements:
Skip "Scopes"
Add the email(s) you’ll use for the Search Console API authentication into the "Test Users". There could be other emails versus just the one that owns the Google Drive. An example may be a client’s email where you access the Google Search Console UI to view their KPIs.
4. In the left-rail navigation, click into "Credentials" > "CREATE CREDENTIALS" > "OAuth Client ID" (Not in image).
5. Within the "Create OAuth client ID" form, fill in:
Application Type = Desktop app
Name = Google Colab
Click "CREATE"
6. Save the "Client ID" and "Client Secret" — as these will be added into the "api" folder config.py file from the Github files we downloaded.
These should have appeared in a popup after hitting "CREATE"
The "Client Secret" is functionally the password to your Google Cloud (DO NOT post this to the public/share it online)
Part four: Add the OAuth 2.0 credentials to the Config.py file
1. Return to Google Drive and navigate into the "api" folder.
2. Click into config.py.
3. Choose to open with "Text Editor" (or another app of your choice) to modify the config.py file.
4. Update the three areas highlighted below with your:
CLIENT_ID: From the OAuth 2.0 client ID setup process
CLIENT_SECRET: From the OAuth 2.0 client ID setup process
GOOGLE_CREDENTIALS: Email that corresponds with your CLIENT_ID & CLIENT_SECRET
5. Save the file once updated!
Congratulations, the boring stuff is over. You are now ready to start using the Google Colab file!
Running your first analysis
Running your first analysis may be a little intimidating, but stick with it and it will get easy fast.
Below, we’ve provided details regarding the input variables required, as well as notes on things to keep in mind when running the script and analyzing the resulting dataset.
After we walk through these items, there are also a few example projects and video walkthroughs showcasing ways to utilize these datasets for client deliverables.
Setting up the input variables
XPath extraction with the "xpath_selector" variable
Have you ever wanted to know every query driving clicks and impressions to a webpage that aren’t in your <title> or <h1> tag? Well, this parameter will allow you to do just that.
While optional, using this is highly encouraged and we feel it "supercharges" the analysis. Simply define site sections with Xpaths and the script will do the rest.
youtube
In the above video, you’ll find examples on how to create site specific extractions. In addition, below are some universal extractions that should work on almost any site on the web:
'//title' # Identifies a <title> tag
'//h1' # Identifies a <h1> tag
'//h2' # Identifies a <h2> tag
Site Specific: How to scrape only the main content (MC)?
Chaining Xpaths – Add a "|" Between Xpaths
'//title | //h1' # Gets you both the <title> and <h1> tag in 1 run
'//h1 | //h2 | //h3' # Gets you both the <h1>, <h2> and <h3> tags in 1 run
Other variables
Here’s a video overview of the other variables with a short description of each.
youtube
'colab_path' [Required] – The path in which the Colab file lives. This should be "/content/drive/My Drive/Colab Notebooks/".
'domain_lookup' [Required] – Homepage of the website utilized for analysis.
'startdate' & 'enddate' [Required] – Date range for the analysis period.
'gsc_sorting_field' [Required] – The tool pulls the top N pages as defined by the user. The "top" is defined by either "clicks_sum" or "impressions_sum." Please review the video for a more detailed description.
'gsc_limit_pages_number' [Required] – Numeric value that represents the number of resulting pages you’d like within the dataset.
'brand_exclusions' [Optional] – The string sequence(s) that commonly result in branded queries (e.g., anything containing "inseev" will be branded queries for "Inseev Interactive").
'impressions_exclusion' [Optional] – Numeric value used to exclude queries that are potentially irrelevant due to the lack of pre-existing impressions. This is primarily relevant for domains with strong pre-existing rankings on a large scale number of pages.
'page_inclusions' [Optional] – The string sequence(s) that are found within the desired analysis page type. If you’d like to analyze the entire domain, leave this section blank.
Running the script
Keep in mind that once the script finishes running, you’re generally going to use the "step3_query-optimizer_domain-YYYY-MM-DD.csv" file for analysis, but there are others with the raw datasets to browse as well.
Practical use cases for the "step3_query-optimizer_domain-YYYY-MM-DD.csv" file can be found in the "Practical use cases and templates" section.
That said, there are a few important things to note while testing things out:
1. No JavaScript Crawling: As mentioned at the start of the post, this script is NOT set up for JavaScript crawling, so if your target website uses a JS frontend with client-side rendering to populate the main content (MC), the scrape will not be useful. However, the basic functionality of quickly getting the top XX (user-defined) queries and pages can still be useful by itself.
2. Google Drive / GSC API Auth: The first time you run the script in each new session it will prompt you to authenticate both the Google Drive and the Google Search Console credentials.
Google Drive authentication: Authenticate to whatever email is associated with the Google Drive with the script.
GSC authentication: Authenticate whichever email has permission to use the desired Google Search Console account.
If you attempt to authenticate and you get an error that looks like the one below, please revisit the "Add the email(s) you’ll use the Colab app with into the 'Test Users'" from Part 3, step 3 in the process above: setting up the consent screen.
Quick tip: The Google Drive account and the GSC Authentication DO NOT have to be the same email, but they do require separate authentications with OAuth.
3. Running the script: Either navigate to "Runtime" > "Restart and Run All" or use the keyboard shortcut CTRL + fn9 to start running the script.
4. Populated datasets/folder structure: There are three CSVs populated by the script – all nested within a folder structure based on the "domain_lookup" input variable.
Automated Organization [Folders]: Each time you rerun the script on a new domain, it will create a new folder structure in order to keep things organized.
Automated Organization [File Naming]: The CSVs include the date of the export appended to the end, so you’ll always know when the process ran as well as the date range for the dataset.
5. Date range for dataset: Inside of the dataset there is a "gsc_datasetID" column generated, which includes the date range of the extraction.
6. Unfamiliar metrics: The resulting dataset has all the KPIs we know and love – e.g. clicks, impressions, average (mean) position — but there are also a few you cannot get directly from the GSC UI:
'count_instances_gsc' — the number of instances the query got at least 1 impression during the specified date range. Scenario example: GSC tells you that you were in an average position 6 for a large keyword like "flower delivery" and you only received 20 impressions in a 30-day date range. Doesn’t seem possible that you were really in position 6, right? Well, now you can see that was potentially because you only actually showed up on one day in that 30-day date range (e.g. count_instances_gsc = 1)
'max_position' & 'min_position' — the MAXIMUM and MINIMUM ranking position the identified page showed up for in Google Search within the specified date range.
Quick tip #1: Large variance in max/min may tell you that your keyword has been fluctuating heavily.
Quick tip #2: These KPIs, in conjunction with the "count_instances_gsc", can exponentially further your understanding of query performance and opportunity.
Practical use cases and templates
Access the recommended multi-use template.
Recommended use: Download file and use with Excel. Subjectively speaking, I believe Excel has a much more user friendly pivot table functionality in comparison to Google Sheets — which is critical for using this template.
Alternative use: If you do not have Microsoft Excel or you prefer a different tool, you can use most spreadsheet apps that contain pivot functionality.
For those who opt for an alternative spreadsheet software/app:
Below are the pivot fields to mimic upon setup.
You may have to adjust the Vlookup functions found on the "Step 3 _ Analysis Final Doc" tab, depending on whether your updated pivot columns align with the current pivot I’ve supplied.
Project example: Title & H1 re-optimizations (video walkthrough)
Project description: Locate keywords that are driving clicks and impressions to high value pages and that do not exist within the <title> and <h1> tags by reviewing GSC query KPIs vs. current page elements. Use the resulting findings to re-optimize both the <title> and <h1> tags for pre-existing pages.
Project assumptions: This process assumes that inserting keywords into both the <title> and <h1> tags is a strong SEO practice for relevancy optimization, and that it’s important to include related keyword variants into these areas (e.g. non-exact match keywords with matching SERP intent).
youtube
Project example: On-page text refresh/re-optimization
Project description: Locate keywords that are driving clicks and impressions to editorial pieces of content that DO NOT exist within the first paragraph within the body of the main content (MC). Perform an on-page refresh of introductory content within editorial pages to include high value keyword opportunities.
Project assumptions: This process assumes that inserting keywords into the first several sentences of a piece of content is a strong SEO practice for relevancy optimization, and that it’s important to include related keyword variants into these areas (e.g. non-exact match keywords with matching SERP intent).
youtube
Final thoughts
We hope this post has been helpful and opened you up to the idea of using Python and Google Colab to supercharge your relevancy optimization strategy.
As mentioned throughout the post, keep the following in mind:
Github repository will be updated with any changes we make in the future.
There is the possibility of undiscovered errors. If these occur, Inseev is happy to help! In fact, we would actually appreciate you reaching out to investigate and fix errors (if any do appear). This way others don’t run into the same problems.
Other than the above, if you have any ideas on ways to Colab (pun intended) on data analytics projects, feel free to reach out with ideas.
0 notes
Text
Page Level Query Analysis at Scale with Google Colab, Python, & the GSC API [Video Instructions Included]
The YouTube playlist referenced throughout this blog can be found here:6 Part YouTube Series [Setting Up & Using the Query Optimization Checker]
Anyone who does SEO as part of their job knows that there’s a lot of value in analyzing which queries are and are not sending traffic to specific pages on a site.
The most common uses for these datasets are to align on-page optimizations with existing rankings and traffic, and to identify gaps in ranking keywords.
However, working with this data is extremely tedious because it’s only available in the Google Search Console interface, and you have to look at only one page at a time.
On top of that, to get information on the text included in the ranking page, you either need to manually review it or extract it with a tool like Screaming Frog.
You need this kind of view:
…but even the above view would only be viable one page at a time, and as mentioned, the actual text extraction would have had to be separate as well.
Given these apparent issues with the readily available data at the SEO community’s disposal, the data engineering team at Inseev Interactive has been spending a lot of time thinking about how we can improve these processes at scale.
One specific example that we’ll be reviewing in this post is a simple script that allows you to get the above data in a flexible format for many great analytical views.
Better yet, this will all be available with only a few single input variables.
A quick rundown of tool functionality
The tool automatically compares the text on-page to the Google Search Console top queries at the page-level to let you know which queries are on-page as well as how many times they appear on the page. An optional XPath variable also allows you to specify the part of the page you want to analyze text on.
This means you’ll know exactly what queries are driving clicks/impressions that are not in your <title>, <h1>, or even something as specific as the first paragraph within the main content (MC). The sky's the limit.
For those of you not familiar, we’ve also provided some quick XPath expressions you can use, as well as how to create site-specific XPath expressions within the "Input Variables" section of the post.
Post setup usage & datasets
Once the process is set up, all that’s required is filling out a short list of variables and the rest is automated for you.
The output dataset includes multiple automated CSV datasets, as well as a structured file format to keep things organized. A simple pivot of the core analysis automated CSV can provide you with the below dataset and many other useful layouts.
… Even some "new metrics"?
Okay, not technically "new," but if you exclusively use the Google Search Console user interface, then you haven’t likely had access to metrics like these before: "Max Position," "Min Position," and "Count Position" for the specified date range – all of which are explained in the "Running your first analysis" section of the post.
To really demonstrate the impact and usefulness of this dataset, in the video below we use the Colab tool to:
[3 Minutes] — Find non-brand <title> optimization opportunities for https://www.inseev.com/ (around 30 pages in video, but you could do any number of pages)
[3 Minutes] — Convert the CSV to a more useable format
[1 Minute] – Optimize the first title with the resulting dataset
youtube
Okay, you’re all set for the initial rundown. Hopefully we were able to get you excited before moving into the somewhat dull setup process.
Keep in mind that at the end of the post, there is also a section including a few helpful use cases and an example template! To jump directly to each section of this post, please use the following links:
One-time setup of the script in Google Colab
Running your first analysis
Practical use cases and templates
[Quick Consideration #1] — The web scraper built into the tool DOES NOT support JavaScript rendering. If your website uses client-side rendering, the full functionality of the tool unfortunately will not work.
[Quick Consideration #2] — This tool has been heavily tested by the members of the Inseev team. Most bugs [specifically with the web scraper] have been found and fixed, but like any other program, it is possible that other issues may come up.
If you encounter any errors, feel free to reach out to us directly at [email protected] or [email protected], and either myself or one of the other members of the data engineering team at Inseev would be happy to help you out.
If new errors are encountered and fixed, we will always upload the updated script to the code repository linked in the sections below so the most up-to-date code can be utilized by all!
One-time setup of the script in Google Colab (in less than 20 minutes)
Things you’ll need:
Google Drive
Google Cloud Platform account
Google Search Console access
Video walkthrough: tool setup process
Below you’ll find step-by-step editorial instructions in order to set up the entire process. However, if following editorial instructions isn’t your preferred method, we recorded a video of the setup process as well.
As you’ll see, we start with a brand new Gmail and set up the entire process in approximately 12 minutes, and the output is completely worth the time.
youtube
Keep in mind that the setup is one-off, and once set up, the tool should work on command from there on!
Editorial walkthrough: tool setup process
Four-part process:
Download the files from Github and set up in Google Drive
Set up a Google Cloud Platform (GCP) Project (skip if you already have an account)
Create the OAuth 2.0 client ID for the Google Search Console (GSC) API (skip if you already have an OAuth client ID with the Search Console API enabled)
Add the OAuth 2.0 credentials to the Config.py file
Part one: Download the files from Github and set up in Google Drive
Download source files (no code required)
1. Navigate here.
2. Select "Code" > "Download Zip"
*You can also use 'git clone https://github.com/jmelm93/query-optmization-checker.git' if you’re more comfortable using the command prompt.
Initiate Google Colab in Google Drive
If you already have a Google Colaboratory setup in your Google Drive, feel free to skip this step.
1. Navigate here.
2. Click "New" > "More" > "Connect more apps".
3. Search "Colaboratory" > Click into the application page.
4. Click "Install" > "Continue" > Sign in with OAuth.
5. Click "OK" with the prompt checked so Google Drive automatically sets appropriate files to open with Google Colab (optional).
Import the downloaded folder to Google Drive & open in Colab
1. Navigate to Google Drive and create a folder called "Colab Notebooks".
IMPORTANT: The folder needs to be called "Colab Notebooks" as the script is configured to look for the "api" folder from within "Colab Notebooks".
Error resulting in improper folder naming.
2. Import the folder downloaded from Github into Google Drive.
At the end of this step, you should have a folder in your Google Drive that contains the below items:
Part two: Set up a Google Cloud Platform (GCP) project
If you already have a Google Cloud Platform (GCP) account, feel free to skip this part.
1. Navigate to the Google Cloud page.
2. Click on the "Get started for free" CTA (CTA text may change over time).
3. Sign in with the OAuth credentials of your choice. Any Gmail email will work.
4. Follow the prompts to sign up for your GCP account.
You’ll be asked to supply a credit card to sign up, but there is currently a $300 free trial and Google notes that they won’t charge you until you upgrade your account.
Part three: Create a 0Auth 2.0 client ID for the Google Search Console (GSC) API
1. Navigate here.
2. After you log in to your desired Google Cloud account, click "ENABLE".
3. Configure the consent screen.
In the consent screen creation process, select "External," then continue onto the "App Information."
Example below of minimum requirements:
Skip "Scopes"
Add the email(s) you’ll use for the Search Console API authentication into the "Test Users". There could be other emails versus just the one that owns the Google Drive. An example may be a client’s email where you access the Google Search Console UI to view their KPIs.
4. In the left-rail navigation, click into "Credentials" > "CREATE CREDENTIALS" > "OAuth Client ID" (Not in image).
5. Within the "Create OAuth client ID" form, fill in:
Application Type = Desktop app
Name = Google Colab
Click "CREATE"
6. Save the "Client ID" and "Client Secret" — as these will be added into the "api" folder config.py file from the Github files we downloaded.
These should have appeared in a popup after hitting "CREATE"
The "Client Secret" is functionally the password to your Google Cloud (DO NOT post this to the public/share it online)
Part four: Add the OAuth 2.0 credentials to the Config.py file
1. Return to Google Drive and navigate into the "api" folder.
2. Click into config.py.
3. Choose to open with "Text Editor" (or another app of your choice) to modify the config.py file.
4. Update the three areas highlighted below with your:
CLIENT_ID: From the OAuth 2.0 client ID setup process
CLIENT_SECRET: From the OAuth 2.0 client ID setup process
GOOGLE_CREDENTIALS: Email that corresponds with your CLIENT_ID & CLIENT_SECRET
5. Save the file once updated!
Congratulations, the boring stuff is over. You are now ready to start using the Google Colab file!
Running your first analysis
Running your first analysis may be a little intimidating, but stick with it and it will get easy fast.
Below, we’ve provided details regarding the input variables required, as well as notes on things to keep in mind when running the script and analyzing the resulting dataset.
After we walk through these items, there are also a few example projects and video walkthroughs showcasing ways to utilize these datasets for client deliverables.
Setting up the input variables
XPath extraction with the "xpath_selector" variable
Have you ever wanted to know every query driving clicks and impressions to a webpage that aren’t in your <title> or <h1> tag? Well, this parameter will allow you to do just that.
While optional, using this is highly encouraged and we feel it "supercharges" the analysis. Simply define site sections with Xpaths and the script will do the rest.
youtube
In the above video, you’ll find examples on how to create site specific extractions. In addition, below are some universal extractions that should work on almost any site on the web:
'//title' # Identifies a <title> tag
'//h1' # Identifies a <h1> tag
'//h2' # Identifies a <h2> tag
Site Specific: How to scrape only the main content (MC)?
Chaining Xpaths – Add a "|" Between Xpaths
'//title | //h1' # Gets you both the <title> and <h1> tag in 1 run
'//h1 | //h2 | //h3' # Gets you both the <h1>, <h2> and <h3> tags in 1 run
Other variables
Here’s a video overview of the other variables with a short description of each.
youtube
'colab_path' [Required] – The path in which the Colab file lives. This should be "/content/drive/My Drive/Colab Notebooks/".
'domain_lookup' [Required] – Homepage of the website utilized for analysis.
'startdate' & 'enddate' [Required] – Date range for the analysis period.
'gsc_sorting_field' [Required] – The tool pulls the top N pages as defined by the user. The "top" is defined by either "clicks_sum" or "impressions_sum." Please review the video for a more detailed description.
'gsc_limit_pages_number' [Required] – Numeric value that represents the number of resulting pages you’d like within the dataset.
'brand_exclusions' [Optional] – The string sequence(s) that commonly result in branded queries (e.g., anything containing "inseev" will be branded queries for "Inseev Interactive").
'impressions_exclusion' [Optional] – Numeric value used to exclude queries that are potentially irrelevant due to the lack of pre-existing impressions. This is primarily relevant for domains with strong pre-existing rankings on a large scale number of pages.
'page_inclusions' [Optional] – The string sequence(s) that are found within the desired analysis page type. If you’d like to analyze the entire domain, leave this section blank.
Running the script
Keep in mind that once the script finishes running, you’re generally going to use the "step3_query-optimizer_domain-YYYY-MM-DD.csv" file for analysis, but there are others with the raw datasets to browse as well.
Practical use cases for the "step3_query-optimizer_domain-YYYY-MM-DD.csv" file can be found in the "Practical use cases and templates" section.
That said, there are a few important things to note while testing things out:
1. No JavaScript Crawling: As mentioned at the start of the post, this script is NOT set up for JavaScript crawling, so if your target website uses a JS frontend with client-side rendering to populate the main content (MC), the scrape will not be useful. However, the basic functionality of quickly getting the top XX (user-defined) queries and pages can still be useful by itself.
2. Google Drive / GSC API Auth: The first time you run the script in each new session it will prompt you to authenticate both the Google Drive and the Google Search Console credentials.
Google Drive authentication: Authenticate to whatever email is associated with the Google Drive with the script.
GSC authentication: Authenticate whichever email has permission to use the desired Google Search Console account.
If you attempt to authenticate and you get an error that looks like the one below, please revisit the "Add the email(s) you’ll use the Colab app with into the 'Test Users'" from Part 3, step 3 in the process above: setting up the consent screen.
Quick tip: The Google Drive account and the GSC Authentication DO NOT have to be the same email, but they do require separate authentications with OAuth.
3. Running the script: Either navigate to "Runtime" > "Restart and Run All" or use the keyboard shortcut CTRL + fn9 to start running the script.
4. Populated datasets/folder structure: There are three CSVs populated by the script – all nested within a folder structure based on the "domain_lookup" input variable.
Automated Organization [Folders]: Each time you rerun the script on a new domain, it will create a new folder structure in order to keep things organized.
Automated Organization [File Naming]: The CSVs include the date of the export appended to the end, so you’ll always know when the process ran as well as the date range for the dataset.
5. Date range for dataset: Inside of the dataset there is a "gsc_datasetID" column generated, which includes the date range of the extraction.
6. Unfamiliar metrics: The resulting dataset has all the KPIs we know and love – e.g. clicks, impressions, average (mean) position — but there are also a few you cannot get directly from the GSC UI:
'count_instances_gsc' — the number of instances the query got at least 1 impression during the specified date range. Scenario example: GSC tells you that you were in an average position 6 for a large keyword like "flower delivery" and you only received 20 impressions in a 30-day date range. Doesn’t seem possible that you were really in position 6, right? Well, now you can see that was potentially because you only actually showed up on one day in that 30-day date range (e.g. count_instances_gsc = 1)
'max_position' & 'min_position' — the MAXIMUM and MINIMUM ranking position the identified page showed up for in Google Search within the specified date range.
Quick tip #1: Large variance in max/min may tell you that your keyword has been fluctuating heavily.
Quick tip #2: These KPIs, in conjunction with the "count_instances_gsc", can exponentially further your understanding of query performance and opportunity.
Practical use cases and templates
Access the recommended multi-use template.
Recommended use: Download file and use with Excel. Subjectively speaking, I believe Excel has a much more user friendly pivot table functionality in comparison to Google Sheets — which is critical for using this template.
Alternative use: If you do not have Microsoft Excel or you prefer a different tool, you can use most spreadsheet apps that contain pivot functionality.
For those who opt for an alternative spreadsheet software/app:
Below are the pivot fields to mimic upon setup.
You may have to adjust the Vlookup functions found on the "Step 3 _ Analysis Final Doc" tab, depending on whether your updated pivot columns align with the current pivot I’ve supplied.
Project example: Title & H1 re-optimizations (video walkthrough)
Project description: Locate keywords that are driving clicks and impressions to high value pages and that do not exist within the <title> and <h1> tags by reviewing GSC query KPIs vs. current page elements. Use the resulting findings to re-optimize both the <title> and <h1> tags for pre-existing pages.
Project assumptions: This process assumes that inserting keywords into both the <title> and <h1> tags is a strong SEO practice for relevancy optimization, and that it’s important to include related keyword variants into these areas (e.g. non-exact match keywords with matching SERP intent).
youtube
Project example: On-page text refresh/re-optimization
Project description: Locate keywords that are driving clicks and impressions to editorial pieces of content that DO NOT exist within the first paragraph within the body of the main content (MC). Perform an on-page refresh of introductory content within editorial pages to include high value keyword opportunities.
Project assumptions: This process assumes that inserting keywords into the first several sentences of a piece of content is a strong SEO practice for relevancy optimization, and that it’s important to include related keyword variants into these areas (e.g. non-exact match keywords with matching SERP intent).
youtube
Final thoughts
We hope this post has been helpful and opened you up to the idea of using Python and Google Colab to supercharge your relevancy optimization strategy.
As mentioned throughout the post, keep the following in mind:
Github repository will be updated with any changes we make in the future.
There is the possibility of undiscovered errors. If these occur, Inseev is happy to help! In fact, we would actually appreciate you reaching out to investigate and fix errors (if any do appear). This way others don’t run into the same problems.
Other than the above, if you have any ideas on ways to Colab (pun intended) on data analytics projects, feel free to reach out with ideas.
0 notes
Text
Page Level Query Analysis at Scale with Google Colab, Python, & the GSC API [Video Instructions Included]
The YouTube playlist referenced throughout this blog can be found here:6 Part YouTube Series [Setting Up & Using the Query Optimization Checker]
Anyone who does SEO as part of their job knows that there’s a lot of value in analyzing which queries are and are not sending traffic to specific pages on a site.
The most common uses for these datasets are to align on-page optimizations with existing rankings and traffic, and to identify gaps in ranking keywords.
However, working with this data is extremely tedious because it’s only available in the Google Search Console interface, and you have to look at only one page at a time.
On top of that, to get information on the text included in the ranking page, you either need to manually review it or extract it with a tool like Screaming Frog.
You need this kind of view:
…but even the above view would only be viable one page at a time, and as mentioned, the actual text extraction would have had to be separate as well.
Given these apparent issues with the readily available data at the SEO community’s disposal, the data engineering team at Inseev Interactive has been spending a lot of time thinking about how we can improve these processes at scale.
One specific example that we’ll be reviewing in this post is a simple script that allows you to get the above data in a flexible format for many great analytical views.
Better yet, this will all be available with only a few single input variables.
A quick rundown of tool functionality
The tool automatically compares the text on-page to the Google Search Console top queries at the page-level to let you know which queries are on-page as well as how many times they appear on the page. An optional XPath variable also allows you to specify the part of the page you want to analyze text on.
This means you’ll know exactly what queries are driving clicks/impressions that are not in your <title>, <h1>, or even something as specific as the first paragraph within the main content (MC). The sky's the limit.
For those of you not familiar, we’ve also provided some quick XPath expressions you can use, as well as how to create site-specific XPath expressions within the "Input Variables" section of the post.
Post setup usage & datasets
Once the process is set up, all that’s required is filling out a short list of variables and the rest is automated for you.
The output dataset includes multiple automated CSV datasets, as well as a structured file format to keep things organized. A simple pivot of the core analysis automated CSV can provide you with the below dataset and many other useful layouts.
… Even some "new metrics"?
Okay, not technically "new," but if you exclusively use the Google Search Console user interface, then you haven’t likely had access to metrics like these before: "Max Position," "Min Position," and "Count Position" for the specified date range – all of which are explained in the "Running your first analysis" section of the post.
To really demonstrate the impact and usefulness of this dataset, in the video below we use the Colab tool to:
[3 Minutes] — Find non-brand <title> optimization opportunities for https://www.inseev.com/ (around 30 pages in video, but you could do any number of pages)
[3 Minutes] — Convert the CSV to a more useable format
[1 Minute] – Optimize the first title with the resulting dataset
youtube
Okay, you’re all set for the initial rundown. Hopefully we were able to get you excited before moving into the somewhat dull setup process.
Keep in mind that at the end of the post, there is also a section including a few helpful use cases and an example template! To jump directly to each section of this post, please use the following links:
One-time setup of the script in Google Colab
Running your first analysis
Practical use cases and templates
[Quick Consideration #1] — The web scraper built into the tool DOES NOT support JavaScript rendering. If your website uses client-side rendering, the full functionality of the tool unfortunately will not work.
[Quick Consideration #2] — This tool has been heavily tested by the members of the Inseev team. Most bugs [specifically with the web scraper] have been found and fixed, but like any other program, it is possible that other issues may come up.
If you encounter any errors, feel free to reach out to us directly at [email protected] or [email protected], and either myself or one of the other members of the data engineering team at Inseev would be happy to help you out.
If new errors are encountered and fixed, we will always upload the updated script to the code repository linked in the sections below so the most up-to-date code can be utilized by all!
One-time setup of the script in Google Colab (in less than 20 minutes)
Things you’ll need:
Google Drive
Google Cloud Platform account
Google Search Console access
Video walkthrough: tool setup process
Below you’ll find step-by-step editorial instructions in order to set up the entire process. However, if following editorial instructions isn’t your preferred method, we recorded a video of the setup process as well.
As you’ll see, we start with a brand new Gmail and set up the entire process in approximately 12 minutes, and the output is completely worth the time.
youtube
Keep in mind that the setup is one-off, and once set up, the tool should work on command from there on!
Editorial walkthrough: tool setup process
Four-part process:
Download the files from Github and set up in Google Drive
Set up a Google Cloud Platform (GCP) Project (skip if you already have an account)
Create the OAuth 2.0 client ID for the Google Search Console (GSC) API (skip if you already have an OAuth client ID with the Search Console API enabled)
Add the OAuth 2.0 credentials to the Config.py file
Part one: Download the files from Github and set up in Google Drive
Download source files (no code required)
1. Navigate here.
2. Select "Code" > "Download Zip"
*You can also use 'git clone https://github.com/jmelm93/query-optmization-checker.git' if you’re more comfortable using the command prompt.
Initiate Google Colab in Google Drive
If you already have a Google Colaboratory setup in your Google Drive, feel free to skip this step.
1. Navigate here.
2. Click "New" > "More" > "Connect more apps".
3. Search "Colaboratory" > Click into the application page.
4. Click "Install" > "Continue" > Sign in with OAuth.
5. Click "OK" with the prompt checked so Google Drive automatically sets appropriate files to open with Google Colab (optional).
Import the downloaded folder to Google Drive & open in Colab
1. Navigate to Google Drive and create a folder called "Colab Notebooks".
IMPORTANT: The folder needs to be called "Colab Notebooks" as the script is configured to look for the "api" folder from within "Colab Notebooks".
Error resulting in improper folder naming.
2. Import the folder downloaded from Github into Google Drive.
At the end of this step, you should have a folder in your Google Drive that contains the below items:
Part two: Set up a Google Cloud Platform (GCP) project
If you already have a Google Cloud Platform (GCP) account, feel free to skip this part.
1. Navigate to the Google Cloud page.
2. Click on the "Get started for free" CTA (CTA text may change over time).
3. Sign in with the OAuth credentials of your choice. Any Gmail email will work.
4. Follow the prompts to sign up for your GCP account.
You’ll be asked to supply a credit card to sign up, but there is currently a $300 free trial and Google notes that they won’t charge you until you upgrade your account.
Part three: Create a 0Auth 2.0 client ID for the Google Search Console (GSC) API
1. Navigate here.
2. After you log in to your desired Google Cloud account, click "ENABLE".
3. Configure the consent screen.
In the consent screen creation process, select "External," then continue onto the "App Information."
Example below of minimum requirements:
Skip "Scopes"
Add the email(s) you’ll use for the Search Console API authentication into the "Test Users". There could be other emails versus just the one that owns the Google Drive. An example may be a client’s email where you access the Google Search Console UI to view their KPIs.
4. In the left-rail navigation, click into "Credentials" > "CREATE CREDENTIALS" > "OAuth Client ID" (Not in image).
5. Within the "Create OAuth client ID" form, fill in:
Application Type = Desktop app
Name = Google Colab
Click "CREATE"
6. Save the "Client ID" and "Client Secret" — as these will be added into the "api" folder config.py file from the Github files we downloaded.
These should have appeared in a popup after hitting "CREATE"
The "Client Secret" is functionally the password to your Google Cloud (DO NOT post this to the public/share it online)
Part four: Add the OAuth 2.0 credentials to the Config.py file
1. Return to Google Drive and navigate into the "api" folder.
2. Click into config.py.
3. Choose to open with "Text Editor" (or another app of your choice) to modify the config.py file.
4. Update the three areas highlighted below with your:
CLIENT_ID: From the OAuth 2.0 client ID setup process
CLIENT_SECRET: From the OAuth 2.0 client ID setup process
GOOGLE_CREDENTIALS: Email that corresponds with your CLIENT_ID & CLIENT_SECRET
5. Save the file once updated!
Congratulations, the boring stuff is over. You are now ready to start using the Google Colab file!
Running your first analysis
Running your first analysis may be a little intimidating, but stick with it and it will get easy fast.
Below, we’ve provided details regarding the input variables required, as well as notes on things to keep in mind when running the script and analyzing the resulting dataset.
After we walk through these items, there are also a few example projects and video walkthroughs showcasing ways to utilize these datasets for client deliverables.
Setting up the input variables
XPath extraction with the "xpath_selector" variable
Have you ever wanted to know every query driving clicks and impressions to a webpage that aren’t in your <title> or <h1> tag? Well, this parameter will allow you to do just that.
While optional, using this is highly encouraged and we feel it "supercharges" the analysis. Simply define site sections with Xpaths and the script will do the rest.
youtube
In the above video, you’ll find examples on how to create site specific extractions. In addition, below are some universal extractions that should work on almost any site on the web:
'//title' # Identifies a <title> tag
'//h1' # Identifies a <h1> tag
'//h2' # Identifies a <h2> tag
Site Specific: How to scrape only the main content (MC)?
Chaining Xpaths – Add a "|" Between Xpaths
'//title | //h1' # Gets you both the <title> and <h1> tag in 1 run
'//h1 | //h2 | //h3' # Gets you both the <h1>, <h2> and <h3> tags in 1 run
Other variables
Here’s a video overview of the other variables with a short description of each.
youtube
'colab_path' [Required] – The path in which the Colab file lives. This should be "/content/drive/My Drive/Colab Notebooks/".
'domain_lookup' [Required] – Homepage of the website utilized for analysis.
'startdate' & 'enddate' [Required] – Date range for the analysis period.
'gsc_sorting_field' [Required] – The tool pulls the top N pages as defined by the user. The "top" is defined by either "clicks_sum" or "impressions_sum." Please review the video for a more detailed description.
'gsc_limit_pages_number' [Required] – Numeric value that represents the number of resulting pages you’d like within the dataset.
'brand_exclusions' [Optional] – The string sequence(s) that commonly result in branded queries (e.g., anything containing "inseev" will be branded queries for "Inseev Interactive").
'impressions_exclusion' [Optional] – Numeric value used to exclude queries that are potentially irrelevant due to the lack of pre-existing impressions. This is primarily relevant for domains with strong pre-existing rankings on a large scale number of pages.
'page_inclusions' [Optional] – The string sequence(s) that are found within the desired analysis page type. If you’d like to analyze the entire domain, leave this section blank.
Running the script
Keep in mind that once the script finishes running, you’re generally going to use the "step3_query-optimizer_domain-YYYY-MM-DD.csv" file for analysis, but there are others with the raw datasets to browse as well.
Practical use cases for the "step3_query-optimizer_domain-YYYY-MM-DD.csv" file can be found in the "Practical use cases and templates" section.
That said, there are a few important things to note while testing things out:
1. No JavaScript Crawling: As mentioned at the start of the post, this script is NOT set up for JavaScript crawling, so if your target website uses a JS frontend with client-side rendering to populate the main content (MC), the scrape will not be useful. However, the basic functionality of quickly getting the top XX (user-defined) queries and pages can still be useful by itself.
2. Google Drive / GSC API Auth: The first time you run the script in each new session it will prompt you to authenticate both the Google Drive and the Google Search Console credentials.
Google Drive authentication: Authenticate to whatever email is associated with the Google Drive with the script.
GSC authentication: Authenticate whichever email has permission to use the desired Google Search Console account.
If you attempt to authenticate and you get an error that looks like the one below, please revisit the "Add the email(s) you’ll use the Colab app with into the 'Test Users'" from Part 3, step 3 in the process above: setting up the consent screen.
Quick tip: The Google Drive account and the GSC Authentication DO NOT have to be the same email, but they do require separate authentications with OAuth.
3. Running the script: Either navigate to "Runtime" > "Restart and Run All" or use the keyboard shortcut CTRL + fn9 to start running the script.
4. Populated datasets/folder structure: There are three CSVs populated by the script – all nested within a folder structure based on the "domain_lookup" input variable.
Automated Organization [Folders]: Each time you rerun the script on a new domain, it will create a new folder structure in order to keep things organized.
Automated Organization [File Naming]: The CSVs include the date of the export appended to the end, so you’ll always know when the process ran as well as the date range for the dataset.
5. Date range for dataset: Inside of the dataset there is a "gsc_datasetID" column generated, which includes the date range of the extraction.
6. Unfamiliar metrics: The resulting dataset has all the KPIs we know and love – e.g. clicks, impressions, average (mean) position — but there are also a few you cannot get directly from the GSC UI:
'count_instances_gsc' — the number of instances the query got at least 1 impression during the specified date range. Scenario example: GSC tells you that you were in an average position 6 for a large keyword like "flower delivery" and you only received 20 impressions in a 30-day date range. Doesn’t seem possible that you were really in position 6, right? Well, now you can see that was potentially because you only actually showed up on one day in that 30-day date range (e.g. count_instances_gsc = 1)
'max_position' & 'min_position' — the MAXIMUM and MINIMUM ranking position the identified page showed up for in Google Search within the specified date range.
Quick tip #1: Large variance in max/min may tell you that your keyword has been fluctuating heavily.
Quick tip #2: These KPIs, in conjunction with the "count_instances_gsc", can exponentially further your understanding of query performance and opportunity.
Practical use cases and templates
Access the recommended multi-use template.
Recommended use: Download file and use with Excel. Subjectively speaking, I believe Excel has a much more user friendly pivot table functionality in comparison to Google Sheets — which is critical for using this template.
Alternative use: If you do not have Microsoft Excel or you prefer a different tool, you can use most spreadsheet apps that contain pivot functionality.
For those who opt for an alternative spreadsheet software/app:
Below are the pivot fields to mimic upon setup.
You may have to adjust the Vlookup functions found on the "Step 3 _ Analysis Final Doc" tab, depending on whether your updated pivot columns align with the current pivot I’ve supplied.
Project example: Title & H1 re-optimizations (video walkthrough)
Project description: Locate keywords that are driving clicks and impressions to high value pages and that do not exist within the <title> and <h1> tags by reviewing GSC query KPIs vs. current page elements. Use the resulting findings to re-optimize both the <title> and <h1> tags for pre-existing pages.
Project assumptions: This process assumes that inserting keywords into both the <title> and <h1> tags is a strong SEO practice for relevancy optimization, and that it’s important to include related keyword variants into these areas (e.g. non-exact match keywords with matching SERP intent).
youtube
Project example: On-page text refresh/re-optimization
Project description: Locate keywords that are driving clicks and impressions to editorial pieces of content that DO NOT exist within the first paragraph within the body of the main content (MC). Perform an on-page refresh of introductory content within editorial pages to include high value keyword opportunities.
Project assumptions: This process assumes that inserting keywords into the first several sentences of a piece of content is a strong SEO practice for relevancy optimization, and that it’s important to include related keyword variants into these areas (e.g. non-exact match keywords with matching SERP intent).
youtube
Final thoughts
We hope this post has been helpful and opened you up to the idea of using Python and Google Colab to supercharge your relevancy optimization strategy.
As mentioned throughout the post, keep the following in mind:
Github repository will be updated with any changes we make in the future.
There is the possibility of undiscovered errors. If these occur, Inseev is happy to help! In fact, we would actually appreciate you reaching out to investigate and fix errors (if any do appear). This way others don’t run into the same problems.
Other than the above, if you have any ideas on ways to Colab (pun intended) on data analytics projects, feel free to reach out with ideas.
0 notes
Text
Page Level Query Analysis at Scale with Google Colab, Python, & the GSC API [Video Instructions Included]
The YouTube playlist referenced throughout this blog can be found here:6 Part YouTube Series [Setting Up & Using the Query Optimization Checker]
Anyone who does SEO as part of their job knows that there’s a lot of value in analyzing which queries are and are not sending traffic to specific pages on a site.
The most common uses for these datasets are to align on-page optimizations with existing rankings and traffic, and to identify gaps in ranking keywords.
However, working with this data is extremely tedious because it’s only available in the Google Search Console interface, and you have to look at only one page at a time.
On top of that, to get information on the text included in the ranking page, you either need to manually review it or extract it with a tool like Screaming Frog.
You need this kind of view:
…but even the above view would only be viable one page at a time, and as mentioned, the actual text extraction would have had to be separate as well.
Given these apparent issues with the readily available data at the SEO community’s disposal, the data engineering team at Inseev Interactive has been spending a lot of time thinking about how we can improve these processes at scale.
One specific example that we’ll be reviewing in this post is a simple script that allows you to get the above data in a flexible format for many great analytical views.
Better yet, this will all be available with only a few single input variables.
A quick rundown of tool functionality
The tool automatically compares the text on-page to the Google Search Console top queries at the page-level to let you know which queries are on-page as well as how many times they appear on the page. An optional XPath variable also allows you to specify the part of the page you want to analyze text on.
This means you’ll know exactly what queries are driving clicks/impressions that are not in your <title>, <h1>, or even something as specific as the first paragraph within the main content (MC). The sky's the limit.
For those of you not familiar, we’ve also provided some quick XPath expressions you can use, as well as how to create site-specific XPath expressions within the "Input Variables" section of the post.
Post setup usage & datasets
Once the process is set up, all that’s required is filling out a short list of variables and the rest is automated for you.
The output dataset includes multiple automated CSV datasets, as well as a structured file format to keep things organized. A simple pivot of the core analysis automated CSV can provide you with the below dataset and many other useful layouts.
… Even some "new metrics"?
Okay, not technically "new," but if you exclusively use the Google Search Console user interface, then you haven’t likely had access to metrics like these before: "Max Position," "Min Position," and "Count Position" for the specified date range – all of which are explained in the "Running your first analysis" section of the post.
To really demonstrate the impact and usefulness of this dataset, in the video below we use the Colab tool to:
[3 Minutes] — Find non-brand <title> optimization opportunities for https://www.inseev.com/ (around 30 pages in video, but you could do any number of pages)
[3 Minutes] — Convert the CSV to a more useable format
[1 Minute] – Optimize the first title with the resulting dataset
youtube
Okay, you’re all set for the initial rundown. Hopefully we were able to get you excited before moving into the somewhat dull setup process.
Keep in mind that at the end of the post, there is also a section including a few helpful use cases and an example template! To jump directly to each section of this post, please use the following links:
One-time setup of the script in Google Colab
Running your first analysis
Practical use cases and templates
[Quick Consideration #1] — The web scraper built into the tool DOES NOT support JavaScript rendering. If your website uses client-side rendering, the full functionality of the tool unfortunately will not work.
[Quick Consideration #2] — This tool has been heavily tested by the members of the Inseev team. Most bugs [specifically with the web scraper] have been found and fixed, but like any other program, it is possible that other issues may come up.
If you encounter any errors, feel free to reach out to us directly at [email protected] or [email protected], and either myself or one of the other members of the data engineering team at Inseev would be happy to help you out.
If new errors are encountered and fixed, we will always upload the updated script to the code repository linked in the sections below so the most up-to-date code can be utilized by all!
One-time setup of the script in Google Colab (in less than 20 minutes)
Things you’ll need:
Google Drive
Google Cloud Platform account
Google Search Console access
Video walkthrough: tool setup process
Below you’ll find step-by-step editorial instructions in order to set up the entire process. However, if following editorial instructions isn’t your preferred method, we recorded a video of the setup process as well.
As you’ll see, we start with a brand new Gmail and set up the entire process in approximately 12 minutes, and the output is completely worth the time.
youtube
Keep in mind that the setup is one-off, and once set up, the tool should work on command from there on!
Editorial walkthrough: tool setup process
Four-part process:
Download the files from Github and set up in Google Drive
Set up a Google Cloud Platform (GCP) Project (skip if you already have an account)
Create the OAuth 2.0 client ID for the Google Search Console (GSC) API (skip if you already have an OAuth client ID with the Search Console API enabled)
Add the OAuth 2.0 credentials to the Config.py file
Part one: Download the files from Github and set up in Google Drive
Download source files (no code required)
1. Navigate here.
2. Select "Code" > "Download Zip"
*You can also use 'git clone https://github.com/jmelm93/query-optmization-checker.git' if you’re more comfortable using the command prompt.
Initiate Google Colab in Google Drive
If you already have a Google Colaboratory setup in your Google Drive, feel free to skip this step.
1. Navigate here.
2. Click "New" > "More" > "Connect more apps".
3. Search "Colaboratory" > Click into the application page.
4. Click "Install" > "Continue" > Sign in with OAuth.
5. Click "OK" with the prompt checked so Google Drive automatically sets appropriate files to open with Google Colab (optional).
Import the downloaded folder to Google Drive & open in Colab
1. Navigate to Google Drive and create a folder called "Colab Notebooks".
IMPORTANT: The folder needs to be called "Colab Notebooks" as the script is configured to look for the "api" folder from within "Colab Notebooks".
Error resulting in improper folder naming.
2. Import the folder downloaded from Github into Google Drive.
At the end of this step, you should have a folder in your Google Drive that contains the below items:
Part two: Set up a Google Cloud Platform (GCP) project
If you already have a Google Cloud Platform (GCP) account, feel free to skip this part.
1. Navigate to the Google Cloud page.
2. Click on the "Get started for free" CTA (CTA text may change over time).
3. Sign in with the OAuth credentials of your choice. Any Gmail email will work.
4. Follow the prompts to sign up for your GCP account.
You’ll be asked to supply a credit card to sign up, but there is currently a $300 free trial and Google notes that they won’t charge you until you upgrade your account.
Part three: Create a 0Auth 2.0 client ID for the Google Search Console (GSC) API
1. Navigate here.
2. After you log in to your desired Google Cloud account, click "ENABLE".
3. Configure the consent screen.
In the consent screen creation process, select "External," then continue onto the "App Information."
Example below of minimum requirements:
Skip "Scopes"
Add the email(s) you’ll use for the Search Console API authentication into the "Test Users". There could be other emails versus just the one that owns the Google Drive. An example may be a client’s email where you access the Google Search Console UI to view their KPIs.
4. In the left-rail navigation, click into "Credentials" > "CREATE CREDENTIALS" > "OAuth Client ID" (Not in image).
5. Within the "Create OAuth client ID" form, fill in:
Application Type = Desktop app
Name = Google Colab
Click "CREATE"
6. Save the "Client ID" and "Client Secret" — as these will be added into the "api" folder config.py file from the Github files we downloaded.
These should have appeared in a popup after hitting "CREATE"
The "Client Secret" is functionally the password to your Google Cloud (DO NOT post this to the public/share it online)
Part four: Add the OAuth 2.0 credentials to the Config.py file
1. Return to Google Drive and navigate into the "api" folder.
2. Click into config.py.
3. Choose to open with "Text Editor" (or another app of your choice) to modify the config.py file.
4. Update the three areas highlighted below with your:
CLIENT_ID: From the OAuth 2.0 client ID setup process
CLIENT_SECRET: From the OAuth 2.0 client ID setup process
GOOGLE_CREDENTIALS: Email that corresponds with your CLIENT_ID & CLIENT_SECRET
5. Save the file once updated!
Congratulations, the boring stuff is over. You are now ready to start using the Google Colab file!
Running your first analysis
Running your first analysis may be a little intimidating, but stick with it and it will get easy fast.
Below, we’ve provided details regarding the input variables required, as well as notes on things to keep in mind when running the script and analyzing the resulting dataset.
After we walk through these items, there are also a few example projects and video walkthroughs showcasing ways to utilize these datasets for client deliverables.
Setting up the input variables
XPath extraction with the "xpath_selector" variable
Have you ever wanted to know every query driving clicks and impressions to a webpage that aren’t in your <title> or <h1> tag? Well, this parameter will allow you to do just that.
While optional, using this is highly encouraged and we feel it "supercharges" the analysis. Simply define site sections with Xpaths and the script will do the rest.
youtube
In the above video, you’ll find examples on how to create site specific extractions. In addition, below are some universal extractions that should work on almost any site on the web:
'//title' # Identifies a <title> tag
'//h1' # Identifies a <h1> tag
'//h2' # Identifies a <h2> tag
Site Specific: How to scrape only the main content (MC)?
Chaining Xpaths – Add a "|" Between Xpaths
'//title | //h1' # Gets you both the <title> and <h1> tag in 1 run
'//h1 | //h2 | //h3' # Gets you both the <h1>, <h2> and <h3> tags in 1 run
Other variables
Here’s a video overview of the other variables with a short description of each.
youtube
'colab_path' [Required] – The path in which the Colab file lives. This should be "/content/drive/My Drive/Colab Notebooks/".
'domain_lookup' [Required] – Homepage of the website utilized for analysis.
'startdate' & 'enddate' [Required] – Date range for the analysis period.
'gsc_sorting_field' [Required] – The tool pulls the top N pages as defined by the user. The "top" is defined by either "clicks_sum" or "impressions_sum." Please review the video for a more detailed description.
'gsc_limit_pages_number' [Required] – Numeric value that represents the number of resulting pages you’d like within the dataset.
'brand_exclusions' [Optional] – The string sequence(s) that commonly result in branded queries (e.g., anything containing "inseev" will be branded queries for "Inseev Interactive").
'impressions_exclusion' [Optional] – Numeric value used to exclude queries that are potentially irrelevant due to the lack of pre-existing impressions. This is primarily relevant for domains with strong pre-existing rankings on a large scale number of pages.
'page_inclusions' [Optional] – The string sequence(s) that are found within the desired analysis page type. If you’d like to analyze the entire domain, leave this section blank.
Running the script
Keep in mind that once the script finishes running, you’re generally going to use the "step3_query-optimizer_domain-YYYY-MM-DD.csv" file for analysis, but there are others with the raw datasets to browse as well.
Practical use cases for the "step3_query-optimizer_domain-YYYY-MM-DD.csv" file can be found in the "Practical use cases and templates" section.
That said, there are a few important things to note while testing things out:
1. No JavaScript Crawling: As mentioned at the start of the post, this script is NOT set up for JavaScript crawling, so if your target website uses a JS frontend with client-side rendering to populate the main content (MC), the scrape will not be useful. However, the basic functionality of quickly getting the top XX (user-defined) queries and pages can still be useful by itself.
2. Google Drive / GSC API Auth: The first time you run the script in each new session it will prompt you to authenticate both the Google Drive and the Google Search Console credentials.
Google Drive authentication: Authenticate to whatever email is associated with the Google Drive with the script.
GSC authentication: Authenticate whichever email has permission to use the desired Google Search Console account.
If you attempt to authenticate and you get an error that looks like the one below, please revisit the "Add the email(s) you’ll use the Colab app with into the 'Test Users'" from Part 3, step 3 in the process above: setting up the consent screen.
Quick tip: The Google Drive account and the GSC Authentication DO NOT have to be the same email, but they do require separate authentications with OAuth.
3. Running the script: Either navigate to "Runtime" > "Restart and Run All" or use the keyboard shortcut CTRL + fn9 to start running the script.
4. Populated datasets/folder structure: There are three CSVs populated by the script – all nested within a folder structure based on the "domain_lookup" input variable.
Automated Organization [Folders]: Each time you rerun the script on a new domain, it will create a new folder structure in order to keep things organized.
Automated Organization [File Naming]: The CSVs include the date of the export appended to the end, so you’ll always know when the process ran as well as the date range for the dataset.
5. Date range for dataset: Inside of the dataset there is a "gsc_datasetID" column generated, which includes the date range of the extraction.
6. Unfamiliar metrics: The resulting dataset has all the KPIs we know and love – e.g. clicks, impressions, average (mean) position — but there are also a few you cannot get directly from the GSC UI:
'count_instances_gsc' — the number of instances the query got at least 1 impression during the specified date range. Scenario example: GSC tells you that you were in an average position 6 for a large keyword like "flower delivery" and you only received 20 impressions in a 30-day date range. Doesn’t seem possible that you were really in position 6, right? Well, now you can see that was potentially because you only actually showed up on one day in that 30-day date range (e.g. count_instances_gsc = 1)
'max_position' & 'min_position' — the MAXIMUM and MINIMUM ranking position the identified page showed up for in Google Search within the specified date range.
Quick tip #1: Large variance in max/min may tell you that your keyword has been fluctuating heavily.
Quick tip #2: These KPIs, in conjunction with the "count_instances_gsc", can exponentially further your understanding of query performance and opportunity.
Practical use cases and templates
Access the recommended multi-use template.
Recommended use: Download file and use with Excel. Subjectively speaking, I believe Excel has a much more user friendly pivot table functionality in comparison to Google Sheets — which is critical for using this template.
Alternative use: If you do not have Microsoft Excel or you prefer a different tool, you can use most spreadsheet apps that contain pivot functionality.
For those who opt for an alternative spreadsheet software/app:
Below are the pivot fields to mimic upon setup.
You may have to adjust the Vlookup functions found on the "Step 3 _ Analysis Final Doc" tab, depending on whether your updated pivot columns align with the current pivot I’ve supplied.
Project example: Title & H1 re-optimizations (video walkthrough)
Project description: Locate keywords that are driving clicks and impressions to high value pages and that do not exist within the <title> and <h1> tags by reviewing GSC query KPIs vs. current page elements. Use the resulting findings to re-optimize both the <title> and <h1> tags for pre-existing pages.
Project assumptions: This process assumes that inserting keywords into both the <title> and <h1> tags is a strong SEO practice for relevancy optimization, and that it’s important to include related keyword variants into these areas (e.g. non-exact match keywords with matching SERP intent).
youtube
Project example: On-page text refresh/re-optimization
Project description: Locate keywords that are driving clicks and impressions to editorial pieces of content that DO NOT exist within the first paragraph within the body of the main content (MC). Perform an on-page refresh of introductory content within editorial pages to include high value keyword opportunities.
Project assumptions: This process assumes that inserting keywords into the first several sentences of a piece of content is a strong SEO practice for relevancy optimization, and that it’s important to include related keyword variants into these areas (e.g. non-exact match keywords with matching SERP intent).
youtube
Final thoughts
We hope this post has been helpful and opened you up to the idea of using Python and Google Colab to supercharge your relevancy optimization strategy.
As mentioned throughout the post, keep the following in mind:
Github repository will be updated with any changes we make in the future.
There is the possibility of undiscovered errors. If these occur, Inseev is happy to help! In fact, we would actually appreciate you reaching out to investigate and fix errors (if any do appear). This way others don’t run into the same problems.
Other than the above, if you have any ideas on ways to Colab (pun intended) on data analytics projects, feel free to reach out with ideas.
0 notes
Text
Page Level Query Analysis at Scale with Google Colab, Python, & the GSC API [Video Instructions Included]
The YouTube playlist referenced throughout this blog can be found here:6 Part YouTube Series [Setting Up & Using the Query Optimization Checker]
Anyone who does SEO as part of their job knows that there’s a lot of value in analyzing which queries are and are not sending traffic to specific pages on a site.
The most common uses for these datasets are to align on-page optimizations with existing rankings and traffic, and to identify gaps in ranking keywords.
However, working with this data is extremely tedious because it’s only available in the Google Search Console interface, and you have to look at only one page at a time.
On top of that, to get information on the text included in the ranking page, you either need to manually review it or extract it with a tool like Screaming Frog.
You need this kind of view:
…but even the above view would only be viable one page at a time, and as mentioned, the actual text extraction would have had to be separate as well.
Given these apparent issues with the readily available data at the SEO community’s disposal, the data engineering team at Inseev Interactive has been spending a lot of time thinking about how we can improve these processes at scale.
One specific example that we’ll be reviewing in this post is a simple script that allows you to get the above data in a flexible format for many great analytical views.
Better yet, this will all be available with only a few single input variables.
A quick rundown of tool functionality
The tool automatically compares the text on-page to the Google Search Console top queries at the page-level to let you know which queries are on-page as well as how many times they appear on the page. An optional XPath variable also allows you to specify the part of the page you want to analyze text on.
This means you’ll know exactly what queries are driving clicks/impressions that are not in your <title>, <h1>, or even something as specific as the first paragraph within the main content (MC). The sky's the limit.
For those of you not familiar, we’ve also provided some quick XPath expressions you can use, as well as how to create site-specific XPath expressions within the "Input Variables" section of the post.
Post setup usage & datasets
Once the process is set up, all that’s required is filling out a short list of variables and the rest is automated for you.
The output dataset includes multiple automated CSV datasets, as well as a structured file format to keep things organized. A simple pivot of the core analysis automated CSV can provide you with the below dataset and many other useful layouts.
… Even some "new metrics"?
Okay, not technically "new," but if you exclusively use the Google Search Console user interface, then you haven’t likely had access to metrics like these before: "Max Position," "Min Position," and "Count Position" for the specified date range – all of which are explained in the "Running your first analysis" section of the post.
To really demonstrate the impact and usefulness of this dataset, in the video below we use the Colab tool to:
[3 Minutes] — Find non-brand <title> optimization opportunities for https://www.inseev.com/ (around 30 pages in video, but you could do any number of pages)
[3 Minutes] — Convert the CSV to a more useable format
[1 Minute] – Optimize the first title with the resulting dataset
youtube
Okay, you’re all set for the initial rundown. Hopefully we were able to get you excited before moving into the somewhat dull setup process.
Keep in mind that at the end of the post, there is also a section including a few helpful use cases and an example template! To jump directly to each section of this post, please use the following links:
One-time setup of the script in Google Colab
Running your first analysis
Practical use cases and templates
[Quick Consideration #1] — The web scraper built into the tool DOES NOT support JavaScript rendering. If your website uses client-side rendering, the full functionality of the tool unfortunately will not work.
[Quick Consideration #2] — This tool has been heavily tested by the members of the Inseev team. Most bugs [specifically with the web scraper] have been found and fixed, but like any other program, it is possible that other issues may come up.
If you encounter any errors, feel free to reach out to us directly at [email protected] or [email protected], and either myself or one of the other members of the data engineering team at Inseev would be happy to help you out.
If new errors are encountered and fixed, we will always upload the updated script to the code repository linked in the sections below so the most up-to-date code can be utilized by all!
One-time setup of the script in Google Colab (in less than 20 minutes)
Things you’ll need:
Google Drive
Google Cloud Platform account
Google Search Console access
Video walkthrough: tool setup process
Below you’ll find step-by-step editorial instructions in order to set up the entire process. However, if following editorial instructions isn’t your preferred method, we recorded a video of the setup process as well.
As you’ll see, we start with a brand new Gmail and set up the entire process in approximately 12 minutes, and the output is completely worth the time.
youtube
Keep in mind that the setup is one-off, and once set up, the tool should work on command from there on!
Editorial walkthrough: tool setup process
Four-part process:
Download the files from Github and set up in Google Drive
Set up a Google Cloud Platform (GCP) Project (skip if you already have an account)
Create the OAuth 2.0 client ID for the Google Search Console (GSC) API (skip if you already have an OAuth client ID with the Search Console API enabled)
Add the OAuth 2.0 credentials to the Config.py file
Part one: Download the files from Github and set up in Google Drive
Download source files (no code required)
1. Navigate here.
2. Select "Code" > "Download Zip"
*You can also use 'git clone https://github.com/jmelm93/query-optmization-checker.git' if you’re more comfortable using the command prompt.
Initiate Google Colab in Google Drive
If you already have a Google Colaboratory setup in your Google Drive, feel free to skip this step.
1. Navigate here.
2. Click "New" > "More" > "Connect more apps".
3. Search "Colaboratory" > Click into the application page.
4. Click "Install" > "Continue" > Sign in with OAuth.
5. Click "OK" with the prompt checked so Google Drive automatically sets appropriate files to open with Google Colab (optional).
Import the downloaded folder to Google Drive & open in Colab
1. Navigate to Google Drive and create a folder called "Colab Notebooks".
IMPORTANT: The folder needs to be called "Colab Notebooks" as the script is configured to look for the "api" folder from within "Colab Notebooks".
Error resulting in improper folder naming.
2. Import the folder downloaded from Github into Google Drive.
At the end of this step, you should have a folder in your Google Drive that contains the below items:
Part two: Set up a Google Cloud Platform (GCP) project
If you already have a Google Cloud Platform (GCP) account, feel free to skip this part.
1. Navigate to the Google Cloud page.
2. Click on the "Get started for free" CTA (CTA text may change over time).
3. Sign in with the OAuth credentials of your choice. Any Gmail email will work.
4. Follow the prompts to sign up for your GCP account.
You’ll be asked to supply a credit card to sign up, but there is currently a $300 free trial and Google notes that they won’t charge you until you upgrade your account.
Part three: Create a 0Auth 2.0 client ID for the Google Search Console (GSC) API
1. Navigate here.
2. After you log in to your desired Google Cloud account, click "ENABLE".
3. Configure the consent screen.
In the consent screen creation process, select "External," then continue onto the "App Information."
Example below of minimum requirements:
Skip "Scopes"
Add the email(s) you’ll use for the Search Console API authentication into the "Test Users". There could be other emails versus just the one that owns the Google Drive. An example may be a client’s email where you access the Google Search Console UI to view their KPIs.
4. In the left-rail navigation, click into "Credentials" > "CREATE CREDENTIALS" > "OAuth Client ID" (Not in image).
5. Within the "Create OAuth client ID" form, fill in:
Application Type = Desktop app
Name = Google Colab
Click "CREATE"
6. Save the "Client ID" and "Client Secret" — as these will be added into the "api" folder config.py file from the Github files we downloaded.
These should have appeared in a popup after hitting "CREATE"
The "Client Secret" is functionally the password to your Google Cloud (DO NOT post this to the public/share it online)
Part four: Add the OAuth 2.0 credentials to the Config.py file
1. Return to Google Drive and navigate into the "api" folder.
2. Click into config.py.
3. Choose to open with "Text Editor" (or another app of your choice) to modify the config.py file.
4. Update the three areas highlighted below with your:
CLIENT_ID: From the OAuth 2.0 client ID setup process
CLIENT_SECRET: From the OAuth 2.0 client ID setup process
GOOGLE_CREDENTIALS: Email that corresponds with your CLIENT_ID & CLIENT_SECRET
5. Save the file once updated!
Congratulations, the boring stuff is over. You are now ready to start using the Google Colab file!
Running your first analysis
Running your first analysis may be a little intimidating, but stick with it and it will get easy fast.
Below, we’ve provided details regarding the input variables required, as well as notes on things to keep in mind when running the script and analyzing the resulting dataset.
After we walk through these items, there are also a few example projects and video walkthroughs showcasing ways to utilize these datasets for client deliverables.
Setting up the input variables
XPath extraction with the "xpath_selector" variable
Have you ever wanted to know every query driving clicks and impressions to a webpage that aren’t in your <title> or <h1> tag? Well, this parameter will allow you to do just that.
While optional, using this is highly encouraged and we feel it "supercharges" the analysis. Simply define site sections with Xpaths and the script will do the rest.
youtube
In the above video, you’ll find examples on how to create site specific extractions. In addition, below are some universal extractions that should work on almost any site on the web:
'//title' # Identifies a <title> tag
'//h1' # Identifies a <h1> tag
'//h2' # Identifies a <h2> tag
Site Specific: How to scrape only the main content (MC)?
Chaining Xpaths – Add a "|" Between Xpaths
'//title | //h1' # Gets you both the <title> and <h1> tag in 1 run
'//h1 | //h2 | //h3' # Gets you both the <h1>, <h2> and <h3> tags in 1 run
Other variables
Here’s a video overview of the other variables with a short description of each.
youtube
'colab_path' [Required] – The path in which the Colab file lives. This should be "/content/drive/My Drive/Colab Notebooks/".
'domain_lookup' [Required] – Homepage of the website utilized for analysis.
'startdate' & 'enddate' [Required] – Date range for the analysis period.
'gsc_sorting_field' [Required] – The tool pulls the top N pages as defined by the user. The "top" is defined by either "clicks_sum" or "impressions_sum." Please review the video for a more detailed description.
'gsc_limit_pages_number' [Required] – Numeric value that represents the number of resulting pages you’d like within the dataset.
'brand_exclusions' [Optional] – The string sequence(s) that commonly result in branded queries (e.g., anything containing "inseev" will be branded queries for "Inseev Interactive").
'impressions_exclusion' [Optional] – Numeric value used to exclude queries that are potentially irrelevant due to the lack of pre-existing impressions. This is primarily relevant for domains with strong pre-existing rankings on a large scale number of pages.
'page_inclusions' [Optional] – The string sequence(s) that are found within the desired analysis page type. If you’d like to analyze the entire domain, leave this section blank.
Running the script
Keep in mind that once the script finishes running, you’re generally going to use the "step3_query-optimizer_domain-YYYY-MM-DD.csv" file for analysis, but there are others with the raw datasets to browse as well.
Practical use cases for the "step3_query-optimizer_domain-YYYY-MM-DD.csv" file can be found in the "Practical use cases and templates" section.
That said, there are a few important things to note while testing things out:
1. No JavaScript Crawling: As mentioned at the start of the post, this script is NOT set up for JavaScript crawling, so if your target website uses a JS frontend with client-side rendering to populate the main content (MC), the scrape will not be useful. However, the basic functionality of quickly getting the top XX (user-defined) queries and pages can still be useful by itself.
2. Google Drive / GSC API Auth: The first time you run the script in each new session it will prompt you to authenticate both the Google Drive and the Google Search Console credentials.
Google Drive authentication: Authenticate to whatever email is associated with the Google Drive with the script.
GSC authentication: Authenticate whichever email has permission to use the desired Google Search Console account.
If you attempt to authenticate and you get an error that looks like the one below, please revisit the "Add the email(s) you’ll use the Colab app with into the 'Test Users'" from Part 3, step 3 in the process above: setting up the consent screen.
Quick tip: The Google Drive account and the GSC Authentication DO NOT have to be the same email, but they do require separate authentications with OAuth.
3. Running the script: Either navigate to "Runtime" > "Restart and Run All" or use the keyboard shortcut CTRL + fn9 to start running the script.
4. Populated datasets/folder structure: There are three CSVs populated by the script – all nested within a folder structure based on the "domain_lookup" input variable.
Automated Organization [Folders]: Each time you rerun the script on a new domain, it will create a new folder structure in order to keep things organized.
Automated Organization [File Naming]: The CSVs include the date of the export appended to the end, so you’ll always know when the process ran as well as the date range for the dataset.
5. Date range for dataset: Inside of the dataset there is a "gsc_datasetID" column generated, which includes the date range of the extraction.
6. Unfamiliar metrics: The resulting dataset has all the KPIs we know and love – e.g. clicks, impressions, average (mean) position — but there are also a few you cannot get directly from the GSC UI:
'count_instances_gsc' — the number of instances the query got at least 1 impression during the specified date range. Scenario example: GSC tells you that you were in an average position 6 for a large keyword like "flower delivery" and you only received 20 impressions in a 30-day date range. Doesn’t seem possible that you were really in position 6, right? Well, now you can see that was potentially because you only actually showed up on one day in that 30-day date range (e.g. count_instances_gsc = 1)
'max_position' & 'min_position' — the MAXIMUM and MINIMUM ranking position the identified page showed up for in Google Search within the specified date range.
Quick tip #1: Large variance in max/min may tell you that your keyword has been fluctuating heavily.
Quick tip #2: These KPIs, in conjunction with the "count_instances_gsc", can exponentially further your understanding of query performance and opportunity.
Practical use cases and templates
Access the recommended multi-use template.
Recommended use: Download file and use with Excel. Subjectively speaking, I believe Excel has a much more user friendly pivot table functionality in comparison to Google Sheets — which is critical for using this template.
Alternative use: If you do not have Microsoft Excel or you prefer a different tool, you can use most spreadsheet apps that contain pivot functionality.
For those who opt for an alternative spreadsheet software/app:
Below are the pivot fields to mimic upon setup.
You may have to adjust the Vlookup functions found on the "Step 3 _ Analysis Final Doc" tab, depending on whether your updated pivot columns align with the current pivot I’ve supplied.
Project example: Title & H1 re-optimizations (video walkthrough)
Project description: Locate keywords that are driving clicks and impressions to high value pages and that do not exist within the <title> and <h1> tags by reviewing GSC query KPIs vs. current page elements. Use the resulting findings to re-optimize both the <title> and <h1> tags for pre-existing pages.
Project assumptions: This process assumes that inserting keywords into both the <title> and <h1> tags is a strong SEO practice for relevancy optimization, and that it’s important to include related keyword variants into these areas (e.g. non-exact match keywords with matching SERP intent).
youtube
Project example: On-page text refresh/re-optimization
Project description: Locate keywords that are driving clicks and impressions to editorial pieces of content that DO NOT exist within the first paragraph within the body of the main content (MC). Perform an on-page refresh of introductory content within editorial pages to include high value keyword opportunities.
Project assumptions: This process assumes that inserting keywords into the first several sentences of a piece of content is a strong SEO practice for relevancy optimization, and that it’s important to include related keyword variants into these areas (e.g. non-exact match keywords with matching SERP intent).
youtube
Final thoughts
We hope this post has been helpful and opened you up to the idea of using Python and Google Colab to supercharge your relevancy optimization strategy.
As mentioned throughout the post, keep the following in mind:
Github repository will be updated with any changes we make in the future.
There is the possibility of undiscovered errors. If these occur, Inseev is happy to help! In fact, we would actually appreciate you reaching out to investigate and fix errors (if any do appear). This way others don’t run into the same problems.
Other than the above, if you have any ideas on ways to Colab (pun intended) on data analytics projects, feel free to reach out with ideas.
#túi_giấy_epacking_việt_nam #túi_giấy_epacking #in_túi_giấy_giá_rẻ #in_túi_giấy #epackingvietnam #tuigiayepacking
0 notes
Text
SEO Trend To Get Top Ranking On Google Search In 2021
SEO has always been an essential part of online business. With continuously evolving search algorithms, it becomes crucial for businesses to analyze the system time-to-time, and optimize the websites SEO according to the new trends in the search market. Some of which are explained below –
BERT –
With the introduction of BERT, Google has leaped forward in providing users with an enriched search result experience and is focusing more on the search intent and not the search string. Earlier, it was easier to punch in high-quality keywords and optimize your website based on what people are searching for. But now, things are going to get a little tricky as the natural language will be processed, and results will be provided based on what seekers are looking for.
Smart SEOs have taken the place of the keywords-based optimization, and editing featured snippets has become difficult. Different keyword tools will no longer be relevant as primary datasets for content creation with be based on pertinent search queries. So, a lot of attention has to be given to user-focused optimization for doing well in 2021.
Keyword planning will go obsolete shortly as predicted by SEO experts of reputed organizations. So, it’s almost mandatory that new strategies are created to go in sync with intent-based algorithms to rank high in the search results.
User Experience And Technical Seo-
Emphasis has to be given on the technical SEO and user-experience on the website. Through technical SEO you optimize your crawling, indexing which helps amplify the overall user-experience of the viewers.
Important things that are to be checked for the best user-experience and growth hacking in 2020 are-
Making the website mobile friendly –
Google now prefers and ranks mobile-friendly and responsive websites over the non-responsive ones. This is not a new practice, but it is necessary for giving the users the best run-through experience, which again boosts your standing. Making responsive websites are also important in gaining edges in the smart-phone driven world.
Optimizing XML Sitemaps-
Sitemaps help to index the website URL on the search consoles. This also gives you the option to prioritize your high-quality pages and index them accordingly.
Optimizing Internal Linkings-
Technical SEOs play an essential part while rating your websites, and internal linking is one of the most critical technical SEO bullet points. It’s essential to check that your website is thoroughly interlinked.
Secured Website –
Securing websites don’t have to do anything with the SEO, but it’s essential that you secure your web pages for enhanced user experience. Your customers will walk away from your website if they see a “not secure” warning. They perhaps would decide not to proceed with your webpage. This would impact your bounce rates, which would eventually affect your overall position on the search result page.
Structuring the data –
2020 will see a lot of changes happening in and around the search algorithms, and high intent-driven content will play a massive role in this process. But, the tricks are not easy; search algorithms don’t fully understand the context of the content. Structuring the data becomes critical here.
Data structuring means organizing the information in such a way that gives out “hints” to the search algorithms about the content. It’s critical to understand and give relevant hints to the system, which specifies not just what is on the page but also tells the system about each component connected with the page and other pages as well. The ultimate goal is to be understood both by search mechanisms and customers. Taking advantage of these options always helps to get through those search results.
Entity Optimization-
Google finds ways to incorporate y7our entity details along with the information it has about your website. Optimizing local SEOs is always important, but it has gone to a different level all-together. Optimizing local SEO has been there for years, and one can always optimize your local SEO and rank itself in the search results even if they don’t own a website. Good entity-based SEO systems also help to grow and capture customers searching for services nearby.
Zero-click searches
Answering customer search queries in the SERP features (Featured Snippets, Knowledge Graphs, Google Local Packs, etc.) itself helps to tap the customers on your website and increases your CTR rate.
With more than 5000 search results popping out with every search query, you must try to add in meta descriptions that brief your customers about the page and their query.
A successful marketer is somebody who can actually bring in people to the platform through clicks and not just tell people about the services through impressions-zero click search strategies help you do just that.
Identifying which keywords bring customers involves tactics. Using tools like Rank Tracker along with your Google Search console account can boost your keyword analysis and drive qualified audiences. This helps you save a lot of time and effort when you are optimizing for queries with keywords like “when,” “how,” “In which year,” etc.
Brand Building –
Sting based keyword attachments and blind stuffing are not going to help anymore, brand building and social media exercises are taking all the show-lights. Although paid campaigns on social media platforms are doing well, marketers are also focusing on organic brand-building through regular updates and social media handles. SEO keyword optimization processes are going off with new settings on the search algorithms, so you must hit out through different means, and brand building is one among the best on the list.
When viewers know about your brand, they will automatically search about it and, in the end, bring more traffic.
Voice Search Website Optimization –
People traditionally used strings and search based attachments to optimize their websites according to the keyword data available. But with a lot of people using voice searches for their queries, Optimising for that also becomes necessary, Ok Google has now become an interactive way to search about a certain thing, and a marketer should know how to optimize for that as well to gauge with a lot of search queries.
Voice searches have a huge impact on SEO as it’s more conversational and targeted, which facilitates Google’s ultimate aim of making the search results more intent-based.
Video Optimization –
Video Marketing is the most interactive means of indirect conversations, and it holds the edge in search results as well. Optimizing SEO structures for videos is essential to get a good rank on Google’s search results. Making responsive videos for mobile and desktop is essential for better search ranks on platforms and search pages.
Apart from the dynamic platforms, it’s essential to make interactive videos that give out all the information it’s made to give, with a catchy title and a good thumbnail.
Adding captions and choosing a trendy topic assists an interaction with the viewers that eventually helps the overall growth of the company.
Creating good quality videos about the services will not be enough because of the heavy competition, it should be regulated with advertising campaigns as well, and choosing the right audience to advertise those videos is crucial with the new algorithms coming up.
Conclusion:
With keyword planners slowly going off the search markets, it becomes really important that emphasis is given to other key elements involved in search optimization. New algorithms should go in sync with development of new strategies which should inculcate the ways which can give users the best experience.
You have to keep an eye on SEO current trends and adjust your strategies to the new challenges, pretty much always. That’s the only way you can give valuable content to users that keep them coming back and also keep your ranking. If you need SEO experts to help you, then it is best to connect with the best SEO company in Clearwater, FL.
0 notes