#DataLakeStorage
Explore tagged Tumblr posts
Text
Azure Data Lake Benefits
Azure Data Lake Benefits
Azure Data Lake is a highly efficient and scalable storage system that helps organizations store and manage large amounts of data in a secure and organized way. It is especially beneficial for businesses looking to utilize big data and advanced analytics.
Here’s why Azure Data Lake is valuable:
Scalability: It can store massive amounts of data without performance issues, making it ideal for businesses with rapidly growing data needs.
Cost-Effective: It offers affordable storage options, allowing organizations to store data without high infrastructure costs.
Flexibility: Azure Data Lake supports different types of data, from structured to unstructured, meaning businesses can store a wide variety of data types without needing to organize or transform them beforehand.
Integration with Analytics Tools: It works seamlessly with other Azure tools, like machine learning and big data analytics platforms, which help companies process and analyze their data more effectively.
Security: Azure Data Lake comes with built-in security features, ensuring that the stored data is protected and only accessible to authorized users.
What is Data Lake?
A Data Lake is essentially a huge storage space where you can keep all types of data—whether it's organized, semi-organized, or completely unorganized—without worrying about how to structure it beforehand. This flexibility allows businesses to store a wide variety of data from various sources, such as IoT sensors, social media posts, website logs, and much more.
The key feature of a Data Lake is its ability to handle massive amounts of data, often referred to as "big data," in its raw form. This means that businesses don’t need to spend time and resources cleaning or organizing the data before storing it. Once the data is in the lake, it can be processed, analyzed, and turned into valuable insights whenever needed.
Data Lake in Azure
Azure Data Lake is a cloud storage solution offered by Microsoft Azure that enables businesses to store large amounts of data securely and efficiently. It’s designed to handle a variety of data types, from simple log files to complex analytics data, all within one platform.
With Azure Data Lake, organizations don’t have to worry about the limitations of traditional storage systems. It’s highly scalable, meaning businesses can store data as their needs grow without running into performance issues. It also offers high performance, so users can access and analyze their data quickly, even when dealing with large volumes.
Because it’s built on the cloud, Azure Data Lake is perfect for modern data needs, such as advanced analytics, machine learning, and business intelligence. Organizations can easily integrate it with other tools to derive valuable insights from their data, helping them make informed decisions and drive business success.
When to Use Data Lake?
Data Lakes are most useful when your business deals with large volumes of diverse data that don’t necessarily need to be organized before storing. If your data comes from multiple sources—like sensors, websites, social media, or internal systems—and is in raw or unstructured form, a Data Lake is the right tool to store it efficiently.
You should consider using a Data Lake if you plan to perform big data analytics, as it can handle vast amounts of information and allows for deeper analysis later. It's also ideal if you're looking to build real-time analytics dashboards or develop machine learning models based on large datasets, particularly those that are unstructured (such as text, images, or logs). By storing all this data in its raw form, you can process and analyze it when needed, without worrying about organizing it first.
Data Lake Can Be Utilized in Various Scenarios
Big Data Analytics: If your company handles large and complex datasets, Azure Data Lake is an ideal solution. It allows businesses to process and analyze these huge amounts of data effectively, supporting advanced analytics that would be difficult with traditional storage systems.
Data Exploration: Researchers and data scientists use Data Lakes to explore raw, unprocessed data. They can dig into this data to discover patterns, trends, or generate new insights that can help with building machine learning models or AI applications.
Data Warehousing: Data Lakes allow businesses to store both structured (like numbers in tables) and unstructured data (like social media posts or images). By combining all types of data, companies can create powerful data warehouses that provide deeper business insights, helping them make better decisions.
Data Archiving: Data Lakes also make it easy to store large amounts of historical data over long periods. Businesses can keep this data safe and easily accessible for future analysis, without worrying about running out of storage space or managing it in traditional databases.
Are Data Lakes Important?
Yes, Data Lakes are very important in today’s data-driven world. They provide businesses with a flexible and scalable way to store massive amounts of data without the constraints of traditional storage systems. As companies generate more data from various sources—such as websites, social media, sensors, and more—Data Lakes make it easier to store all that information in its raw form.
This flexibility is crucial because it allows organizations to store different types of data—structured, semi-structured, or unstructured—without having to organize or transform it first. Data Lakes are also cost-effective, offering a more affordable solution for handling big data and enabling organizations to analyze it using advanced tools like machine learning, AI, and big data analytics.
By tapping into the full potential of their data, businesses can gain deeper insights, make better decisions, and improve their overall performance. This is why Data Lakes are becoming a key component in modern data architecture.
Advantages of Data Lake
Scalability: Azure Data Lake makes it easy for businesses to scale their storage needs as their data grows. As companies collect more data over time, Data Lake can handle the increase in volume without impacting performance, allowing businesses to store as much data as they need.
Cost-effective: Storing data in a Data Lake is usually much more affordable than using traditional databases. This is because Data Lakes are designed to store massive amounts of data efficiently, often at a lower cost per unit compared to more structured storage solutions.
Flexibility: One of the key benefits of a Data Lake is its ability to store various types of data—structured (like numbers), semi-structured (like logs), and unstructured (like images or videos). This flexibility means organizations don't need to prepare or transform data before storing it, making it easier to collect and store diverse data from multiple sources.
Advanced Analytics: With all your data stored in one place, businesses can perform complex analytics across different types of data, all without needing separate systems for each data source. This centralized data storage makes it easier to analyze data, run reports, or build predictive models, helping organizations make data-driven decisions faster and more efficiently.
Limitations of Data Lake
Data Quality: Since Data Lakes store raw, unprocessed data, it can be difficult to ensure the quality and consistency of the data. Raw data may contain errors, duplicates, or irrelevant information that hasn't been cleaned up before being stored. This can make it harder to analyze and use the data effectively without additional processing or quality checks.
Complexity: Although Data Lakes are flexible, managing the large volumes of data they store can be complex. As the data grows, it can become challenging to organize, categorize, and secure it properly. This often requires advanced tools, sophisticated processes, and skilled personnel to ensure that the data remains accessible, well-organized, and usable.
Security: Data security can be another challenge when using a Data Lake, especially when handling sensitive or private data from multiple sources. Ensuring the right access controls, encryption, and compliance with regulations (such as GDPR) can be more complicated than with traditional storage systems. Without proper security measures, organizations may be at risk of data breaches or unauthorized access.
Working of Azure Data Lake
Azure Data Lake provides a unified storage platform that allows businesses to store vast amounts of data in its raw form. It integrates with other Azure services, like Azure Databricks (for data processing), Azure HDInsight (for big data analytics), and Azure Synapse Analytics (for combining data storage and analytics). This integration makes it easier to store, query, and analyze data without having to organize or transform it first.
The platform also provides tools to manage who can access the data, ensuring security protocols are in place to protect sensitive information. Additionally, it offers powerful analytics capabilities, enabling businesses to extract insights from their data and make data-driven decisions without the need for complex transformations.
Who Can Use Azure Data Lake?
Data Scientists and Engineers: These professionals often work with large, unprocessed datasets to develop machine learning models or perform complex data analysis. Azure Data Lake provides the flexibility and scalability they need to work with vast amounts of data.
Business Analysts: Analysts use Data Lakes to explore both structured (organized data) and unstructured (raw or unorganized data) sources to gather insights and make informed business decisions.
Developers: Developers can use Azure Data Lake to store and manage data within their applications, allowing for more efficient decision-making and better data integration in their products or services. This enables applications to leverage big data for improved performance or features.
Azure Data Lake Store Security
Azure Data Lake Storage offers several layers of security to protect data:
Encryption: All data is encrypted while being transferred and when it's stored, ensuring that it cannot be accessed by unauthorized individuals.
Access Control: The service integrates with Azure Active Directory (AAD) for authentication, and businesses can set up RBAC to ensure that only authorized users or systems can access certain data.
Audit Logs: Azure Data Lake generates audit logs that record every action taken on the data, allowing organizations to track who accessed or modified the data. This feature helps maintain security and ensures compliance with regulations.
Components of Azure Data Lake Storage Gen 2
Containers: These are like storage units where data is organized. Containers are used to store blobs (data files) within Azure Storage.
Blobs: These are the actual data files or objects stored within containers. Blobs can be anything from text files to images, videos, or log files.
Folders: Within containers, data can be organized into folders (or directories) and subfolders, making it easier to access and manage large volumes of data.
Need of Azure Data Lake Storage Gen 2
Azure Data Lake Storage Gen2 is needed because businesses and organizations are dealing with an increasing amount of data, both structured and unstructured. Storing and processing such large volumes of data requires a storage solution that is both scalable and flexible. Azure Data Lake Storage Gen2 enables this by offering a secure, scalable way to store data, while also providing powerful tools for advanced analytics and machine learning. The combination of Blob Storage's efficiency and Data Lake's enhanced features allows businesses to extract more value from their data.
#AzureDataLake#BigData#CloudStorage#DataAnalytics#MachineLearning#DataScience#AzureStorage#DataSecurity#BigDataAnalytics#CloudComputing#DataLakeStorage#DataManagement#DataArchiving#AdvancedAnalytics#BusinessIntelligence#AzureGen2#DataIntegration#DataExploration#DataPrivacy#ScalableStorage#DataProtection#DataLakeSecurity
0 notes
Text
What Is Azure Blob Storage? And Azure Blob Storage Cost
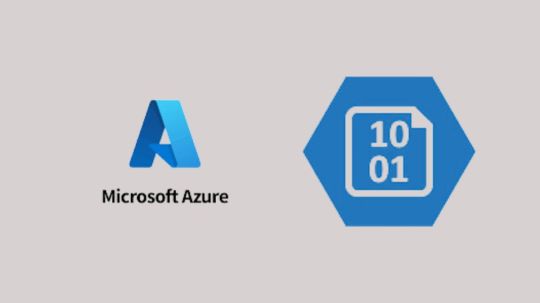
Microsoft Azure Blob Storage
Scalable, extremely safe, and reasonably priced cloud object storage
Incredibly safe and scalable object storage for high-performance computing, archiving, data lakes, cloud-native workloads, and machine learning.
What is Azure Blob Storage?
Microsoft’s cloud-based object storage solution is called Blob Storage. Massive volumes of unstructured data are best stored in blob storage. Text and binary data are examples of unstructured data, which deviates from a certain data model or specification.
Scalable storage and retrieval of unstructured data
Azure Blob Storage offers storage for developing robust cloud-native and mobile apps, as well as assistance in creating data lakes for your analytics requirements. For your long-term data, use tiered storage to minimize expenses, and scale up flexibly for tasks including high-performance computing and machine learning.
Construct robust cloud-native apps
Azure Blob Storage was designed from the ground up to meet the demands of cloud-native, online, and mobile application developers in terms of availability, security, and scale. For serverless systems like Azure Functions, use it as a foundation. Blob storage is the only cloud storage solution that provides a premium, SSD-based object storage layer for low-latency and interactive applications, and it supports the most widely used development frameworks, such as Java,.NET, Python, and Node.js.
Save petabytes of data in an economical manner
Store enormous volumes of rarely viewed or infrequently accessed data in an economical manner with automated lifecycle management and numerous storage layers. Azure Blob Storage can take the place of your tape archives, and you won’t have to worry about switching between hardware generations.
Construct robust data lakes
One of the most affordable and scalable data lake options for big data analytics is Azure Data Lake Storage. It helps you accelerate your time to insight by fusing the strength of a high-performance file system with enormous scalability and economy. Data Lake Storage is tailored for analytics workloads and expands the possibilities of Azure Blob Storage.
Scale out for billions of IoT devices or scale up for HPC
Azure Blob Storage offers the volume required to enable storage for billions of data points coming in from IoT endpoints while also satisfying the rigorous, high-throughput needs of HPC applications.
Features
Scalable, robust, and accessible
With geo-replication and the capacity to scale as needed, the durability is designed to be sixteen nines.
Safe and sound
Role-based access control (RBAC), Microsoft Entra ID (previously Azure Active Directory) authentication, sophisticated threat protection, and encryption at rest.
Data lake-optimized
Multi-protocol access and file namespace facilitate analytics workloads for data insights.
Complete data administration
Immutable (WORM) storage, policy-based access control, and end-to-end lifecycle management.
Integrated security and conformance
Complete security and conformance, integrated
Every year, Microsoft spends about $1 billion on cybersecurity research and development.
Over 3,500 security professionals who are committed to data security and privacy work for it.
Azure boasts one of the biggest portfolios of compliance certifications in the sector.
Azure Blob storage cost
Documents, films, images, backups, and other unstructured text or binary data can all be streamed and stored using block blob storage.
The most recent features are accessible through blob storage accounts, however they do not allow page blobs, files, queues, or tables. For the majority of users, general-purpose v2 storage accounts are advised.
Block blob storage’s overall cost is determined by:
Monthly amount of data kept.
Number and kinds of activities carried out, as well as any expenses related to data transfer.
The option for data redundancy was chosen.
Adaptable costs with reserved alternatives to satisfy your needs for cloud storage
Depending on how frequently you anticipate accessing the data, you can select from its storage tiers. Keep regularly accessed data in Hot, seldom accessed data in Cool and Cold, performance-sensitive data in Premium, and rarely accessed data in Archive. Save a lot of money by setting up storage space.
To continue building with the same free features after your credit, switch to pay as you go. Only make a payment if your monthly usage exceeds your free amounts.
You will continue to receive over fifty-five services at no cost after a year, and you will only be charged for the services you utilize above your monthly allotment.
Read more on Govindhtech.com
#AzureBlobStorage#BlobStorage#machinelearning#Cloudcomputing#cloudstorage#DataLakeStorage#datasecurity#News#Technews#Technology#Technologynews#Technologytrends#govindhtech
0 notes
Text
[PowerBi] Dos formas de obtener .parquet combinados
Hace no mucho tiempo Power bi ha incorporado soporte para la lectura de archivos Parquet evitando la creación de enormes funciones en power query para intentar llegar a dichos datos. La parte más atractiva de la extensión es leerla desde un Azure Data Lake puesto que sería más probable que los datos estuvieran almacenados allí.
Recientemente un compañero tuvo un inconveniente para conectarlo de forma tradicional y nativa. El “combinar” automático de Power Query no funcionaba. Fue entonces como llegue a una segunda forma de combinar los archivos parquet de una única tabla.
Éste artículo muestra como conectarnos a archivos parquet de un Azure Data Lake Gen2 en una carpeta que representa una sola tabla en dos formas distintas.
Antes de iniciar en los métodos específicos vamos a contextualizar brevemente lo necesario para conectarnos a un Data Lake. La conexión puede representarse de dos formas. Mediante una key generada por el administrador o mediante Azure Active Directory.
NOTA: es importante que si vamos por la segunda opción tengamos los permisos necesarios. Rol “Blob Data [contributor, reader, owner]” en el IAM de nuestro recurso en Azure y lectura en el Storage Explorer para el path que vamos a leer desde Power Bi. Más detalles: https://docs.microsoft.com/en-us/power-query/connectors/datalakestorage
Para conectarnos debemos obtener el path de la carpeta desde el Storage explorar correspondiente a DFS URL.
Es importante que sea el DFS porque el blob URL no será reconocido por Power Bi para conectarse.
Primer Método
Esta sería la forma convencional bajo la cual funciona la conexión a una carpeta en Power Bi. Obtenemos datos de Azure Data Lake Gen2 pegando la dirección y al momento de la vista previa de lectura podemos dar “Combinar” en la navegación para que nos genere el código automático de conexión.
De ese modo iremos directo al Editor de consultas con todas las funciones que genera automáticamente Power Bi para combinar los datos.
Si prestamos atención, el modo de combinar los datos consiste en llamar una función personalizada “Transform File” en cada fila mediante una nueva columna (Table.AddColumn)
De esa forma luego expande los datos y llega al resultado esperado.
Segundo Método
Por alguna razón he visto a ese primer método fracasar en un par de oportunidades. No sabría decir si sigue algo en preview por detrás o que sucede. La cuestión es que ese pantallazo de resolución que hace Power Query me invitó a darle otro ángulo más creativo.
Si intentamos conectar un solo archivo parquet con el conector de parquet nativo de Power Bi, el motor llamará a una transformación de archivo binary en “Parquet.Document”. Aprovechando ese aprendizaje vamos a manipular el contenido Power Query. En lugar de Combinar los archivos al leerlos, simplemente vamos a “Transform Data” para abrir el editor de consultas. Una vez allí vamos a dar click derecho en la columna que contiene los Binary y los transformamos en Json.
¿Por qué Json? no hay una razón particular, simplemente queremos que el motor genere el código que vamos a usar para nutrir nuestra transformación. Hoy en día Power Bi Desktop no tiene incorporada la transformación Parquet en la UI pero si en el motor.
Este nuevo paso de transformación a JSON va a generar el siguiente código:
= Table.TransformColumns(Source,{{"Content", Json.Document}})
Nuestra tarea será simplemente cambiar la palabra Json por Parquet para formar la misma transformación que vemos al leer un único archivo parquet que es “Parquet.Document”.
El código final se vería así generando que nuestros archivos binary se transformen en table.
A partir de ese momento podemos expandir el contenido de las tablas exactamente como lo haría el motor automáticamente en el Método anterior.
Esta forma termina dejando un código más limpio puesto que no genera varias funciones y samples que hace automáticamente el motor, sino que todo el código queda bajo una única query.
NOTA: es importante cambiar el nombre del paso que parsea a JSON por parse Parquet para que cualquier otra persona que revise el código entienda de que se trata.
De esta forma llegamos al final del post donde aprendimos dos modos de leer muchos archivos parquet como una sola tabla combinados dentro de una sola carpeta. Ojal�� les sirva si el método convencional falla o queremos nuestro código más limpio.
#Power bi#powerbi#Power bi desktop#power bi parquet#power bi training#power bi tips#power bi tutorial#power bi argentina#power bi cordoba#power bi jujuy
0 notes