#DataTransparency
Explore tagged Tumblr posts
Link
#AIcopyright#datatransparency#digitallabor#EU-AIAct#GenerativeAI#laborrights#OpenAIlitigation#SAG-AFTRA
0 notes
Text
The New Rules of B2B Lead Generation: Are You Keeping Up?
The game has changed. B2B buyers are no longer passive listeners sitting through endless sales pitches. They’re informed, independent, and tougher to impress. Businesses that fail to adapt are being left behind, while those embracing this shift are thriving.
So, what’s different? Let’s break it down.
B2B Buyers Are in Control
Gone are the days when buyers relied solely on sales teams for information. Thanks to the internet, they can research everything they need on their own. By the time they engage with your business, they’ve already made up their minds about what they want.
What Does This Mean for You?
Your lead generation strategy must do more than capture their attention—it needs to offer value. You must show that you’re more than just another checkbox in their research process.
It’s a Team Decision Now
B2B buying decisions rarely come down to one person. Committees are calling the shots, and each member has their own priorities.
The Finance Manager cares about ROI.
The Operations Lead worries about implementation.
The CEO thinks long-term strategy.
Your messaging must address all these perspectives to win them over as a team.
The Digital Experience Matters
Modern buyers have sky-high expectations for digital interactions. They want fast, seamless, and personalized experiences across all devices.
Is your website mobile-friendly?
Do your pages load quickly?
Are your forms easy to fill out?
If not, potential buyers will leave—and they won’t come back.
Trust is Everything
Buyers today are cautious. They won’t share their details unless they see real value in return. Want their email address? Offer something that’s worth it:
A free guide.
A helpful tool.
Insightful content that solves a problem.
Be transparent about how their data will be used. People value honesty, and building trust is key to generating quality leads.
Winning Lead Generation Strategies for 2025
Here are four must-dos to stay ahead:
Offer Value First Stop pushing sales messages. Instead, provide useful tips, tools, and insider knowledge that buyers genuinely appreciate.
Simplify Your Forms Don’t ask for too much upfront. Start with just a name and email. You can gather more details as the relationship builds.
Be Transparent About Data Clearly explain how you’ll use their information. Transparency builds trust, and trust builds leads.
Go Mobile-First Most buyers research on their phones. If your website isn’t mobile-friendly, you’re losing out.
Looking Ahead to 2025
As technology evolves, so do buyer expectations. With stricter privacy laws, smarter consumers, and AI-driven tools, businesses must strike a balance between automation and the human touch.
Lead generation is no longer just about collecting emails. It’s about understanding buyers’ needs, building relationships, and offering value at every step of their journey.
If you’re still relying on outdated tactics like “Sign up for our newsletter,” it’s time to rethink your strategy. The future belongs to businesses that give buyers what they want—on their terms.
Want to understand how different generations engage with content and how it influences buying decisions? Check out our in-depth guide here. For more information visit our Website or Contact us.
#B2BMarketing#LeadGeneration#DigitalTransformation#BuyerJourney#CustomerExperience#MarketingStrategy#B2BSales#ContentMarketing#TrustBuilding#ModernMarketing#MobileFirst#DataTransparency#ValueDrivenMarketing#B2BTrends#FutureOfMarketing#AIInMarketing#PersonalizedExperience
0 notes
Text
#MicrofinanceBankingSoftware#FinancialTransparency#BankingInnovation#MicrofinanceSolutions#BestMicrofinanceSoftware#BankingTechnology#FinTechSolutions#SoftwareForMicrofinance#GTechWebSolutions#BestSoftwareCompanyIndia#MicrofinanceExperts#FinancialReporting#DataTransparency#AuditTrails#RegulatoryCompliance#FinancialInclusion#SustainableFinance#BankingSolutionsForAll
0 notes
Text
𝐔𝐧𝐢𝐟𝐲𝐢𝐧𝐠 𝐃𝐚𝐭𝐚 𝐚𝐧𝐝 𝐈𝐧𝐬𝐢𝐠𝐡𝐭𝐬
Our reports leverage the full spectrum of Inrate’s ESG Ratings and Data, encompassing 𝐎𝐯𝐞𝐫𝐚𝐥𝐥 𝐈𝐦𝐩𝐚𝐜𝐭 𝐑𝐚𝐭𝐢𝐧𝐠 Gain a holistic view of your portfolio's sustainability strengths and weaknesses 𝐂𝐥𝐢𝐦𝐚𝐭𝐞 𝐄𝐱𝐩𝐨𝐬𝐮𝐫𝐞 Identify the climate impact of your portfolio using Inrate’s climate data and climate-specific indicators 𝐒𝐢𝐧𝐠𝐥𝐞 𝐈𝐧𝐝𝐢𝐜𝐚𝐭𝐨𝐫𝐬 Deep dive into specific ESG issues such as child labor or banned weapons to align your investments with your values 𝐓𝐡𝐞𝐦𝐚𝐭𝐢𝐜 𝐒𝐜𝐫𝐞𝐞𝐧𝐢𝐧𝐠 Screen your portfolio for alignment with specific sustainability themes or industry best practices 𝐅𝐞𝐚𝐭𝐮𝐫𝐞𝐬 𝐨𝐟 𝐏𝐨𝐫𝐭𝐟𝐨𝐥𝐢𝐨 𝐀𝐧𝐚𝐥𝐲𝐬𝐢𝐬 & 𝐑𝐞𝐩𝐨𝐫𝐭𝐢𝐧𝐠 𝐁𝐞𝐧𝐜𝐡𝐦𝐚𝐫𝐤𝐢𝐧𝐠 𝐟𝐨𝐫 𝐄𝐧𝐡𝐚𝐧𝐜𝐞𝐝 𝐔𝐧𝐝𝐞𝐫𝐬𝐭𝐚𝐧𝐝𝐢𝐧𝐠 We go beyond basic reporting by providing insightful comparisons to relevant benchmarks. Gauge your portfolio's sustainability contributions and performance relative to your peers, and track progress over time. 𝐂𝐨𝐧𝐬𝐢𝐬𝐭𝐞𝐧𝐭 𝐚𝐧𝐝 𝐕𝐞𝐫𝐬𝐚𝐭𝐢𝐥𝐞 Inrate's reporting framework ensures consistent analysis across all asset classes, from equities to real estate. This uniformity simplifies portfolio comparisons and facilitates holistic decision-making. 𝐂𝐥𝐢𝐞𝐧𝐭-𝐜𝐞𝐧𝐭𝐫𝐢𝐜 𝐃𝐞𝐬𝐢𝐠𝐧 We tailor reports to your specific needs and preferences. Whether you require detailed breakdowns or concise summaries, Inrate delivers the information you need in a clear and actionable format. 𝐀𝐥𝐢𝐠𝐧𝐞𝐝 𝐰𝐢𝐭𝐡 𝐋𝐞𝐚𝐝𝐢𝐧𝐠 𝐒𝐭𝐚𝐧𝐝𝐚𝐫𝐝𝐬 Our reports are designed to adhere to industry best practices, including the upcoming Swiss Climate Score and the ASIP Stewardship Reporting framework.
0 notes
Text

Unlock the true potential of data with integrity! Embrace data science ethics to ensure transparency, fairness, and trust in every algorithm. .
1 note
·
View note
Text
"बिहार में जाति आधारित गणना की रिपोर्ट: सरकार की नजर में सच्चाई"
कोर्ट कचहरी के चककरो से बाहर निकलकर बिहार में जाति आधारित गणना की रिपोर्ट सोमवार सार्वजनिक हो गयी। इस जनगणना के साथ राज्य की जनसंख्या का भी आंकलन (गणना) हो गया। इसमें सर्वाधिक जनसंख्या पिछड़े वर्ग की हैं। (36.1 %) 27.12 % की हिस्सेदारी के साथ अत्यंत पिछड़ा वर्ग दूसरे पायदान पर हैं।
#CasteCensusBihar#GovernmentData#BiharCasteReport#SocialStatistics#CasteDemographics#InclusiveIndia#BiharSociety#DataTransparency#NeetiShastriGovernment#CasteBasedAnalysis
0 notes
Text
Promoting Fairness in Analytics: A Path to Ethical Data Insights
In the age of data-driven decision-making, promoting fairness in analytics is more critical than ever. Organizations worldwide are leveraging analytics to gain insights, make predictions, and drive strategies. However, these powerful tools come with ethical responsibilities to ensure they do not perpetuate biases or discriminate against any group. This blog post explores why fairness in analytics matters and how we can foster a more equitable data landscape.
The Significance of Fairness in Analytics
Analytics, powered by machine learning algorithms, often rely on vast datasets to make predictions and decisions. These algorithms are only as good as the data they are trained on. If the data contains biases or inequalities, these issues can become amplified, resulting in unfair outcomes. Here's why fairness is essential:
Avoiding Discrimination: Unfair analytics can discriminate against certain groups based on race, gender, or socioeconomic factors, perpetuating social injustices.
Enhancing Trust: Fairness in analytics builds trust among users and stakeholders, ensuring that insights are reliable and unbiased.
Legal and Ethical Compliance: Regulatory bodies like GDPR and FCRA have stringent requirements regarding data fairness, making it essential for organizations to comply.
Challenges in Achieving Fairness
While the goal of fairness is clear, achieving it in practice presents challenges:
Data Bias: Historical biases in data can lead to biased predictions. Addressing data bias requires meticulous curation and preprocessing of datasets.
Algorithmic Bias: Algorithms can inadvertently introduce bias. Fair algorithms involve careful feature selection and model design.
Trade-offs: Balancing fairness with accuracy can be complex. Sometimes, optimizing for one may compromise the other.
Mitigating Bias in Analytics
Several strategies can help mitigate bias and promote fairness:
Data Preprocessing: Techniques like re-sampling, re-weighting, and data augmentation can balance class distributions and reduce bias.
Fair Feature Selection: Avoid using features that correlate with protected attributes. Seek alternative, unbiased features.
Algorithmic Fairness: Incorporate fairness constraints into the optimization objectives to ensure the model does not discriminate.
Post-processing and Adversarial Testing: Post-processing can further mitigate bias, while adversarial testing can uncover hidden biases.
Conclusion
Promoting fairness in analytics is not just a technological concern; it's an ethical imperative. As analytics continue to shape our world, it's crucial that we prioritize fairness to ensure that data-driven decisions benefit everyone equally. By understanding the significance of fairness, acknowledging the challenges, and implementing bias mitigation techniques, we can create a data landscape that is not only powerful but also just and equitable. Together, we can make data work for a fairer future.
#DataEthics#FairnessInAnalytics#BiasMitigation#EthicalData#AnalyticsForJustice#DataFairness#AlgorithmicFairness#DiversityAndInclusion#DataDrivenDecisions#TechEthics#DataPrivacy#EthicalAI#SocialJustice#ResponsibleDataUse#DigitalEthics#AnalyticsInsights#DataTransparency#LegalCompliance#EthicalTech#FairAnalytics
0 notes
Text
How do you ensure data consistency across various systems and databases within an organization?
Ensuring data consistency across various systems and databases within an organization is crucial for accurate reporting, effective decision-making, and smooth business operations. Data consistency can be achieved through various techniques and best practices, including:
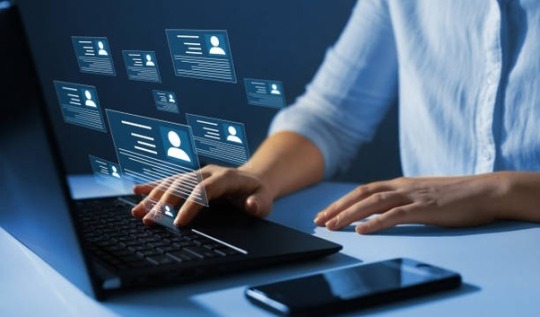
Data Integration and Centralization: Implement a robust data integration strategy to centralize data from different sources into a unified data repository or data warehouse. This ensures that all systems and applications within the organization access and update a single, authoritative source of data.
Master Data Management (MDM): Utilize Master Data Management solutions to create and maintain a master record of critical data entities (e.g., customers, products) that are shared across different systems. This helps in ensuring consistent and standardized data across the organization.
Data Standardization: Enforce data standardization practices to ensure uniformity in data format, naming conventions, and data definitions across various systems. This reduces the risk of data inconsistencies arising from variations in data representation.
Data Quality Management: Implement data quality management processes to identify and resolve data quality issues. Regular data quality checks and cleansing activities help maintain consistent and accurate data.
Data Governance: Establish a data governance framework that defines data ownership, data stewardship, and data management policies. This ensures that data consistency measures are implemented and monitored effectively.
Data Validation Rules: Define and enforce data validation rules and constraints for each data attribute in the databases and systems. These rules should check data integrity and enforce business rules to maintain consistency.
Transaction Management: Implement transaction management mechanisms to ensure that changes to data across multiple systems are carried out in a controlled manner. Transactions help maintain data consistency even in the event of system failures or errors.
Real-time Data Replication: Use real-time data replication technologies to synchronize data across databases and systems in real-time. This ensures that the most up-to-date information is available uniformly across the organization.
Change Management Processes: Implement change management processes to govern any modifications to data structures or data-related processes. These processes help maintain data consistency during system upgrades or changes.
Data Versioning and Timestamps: Implement version control and timestamps to track changes to data over time. This allows for easy identification of the most recent data and helps maintain data consistency in historical records.
Regular Data Audits: Conduct periodic data audits to identify any discrepancies or inconsistencies in data across systems. Address any identified issues promptly to maintain data consistency.
Training and Awareness: Train employees on data consistency best practices and the importance of data integrity. Awareness among staff helps minimize human errors and improves adherence to data consistency guidelines.
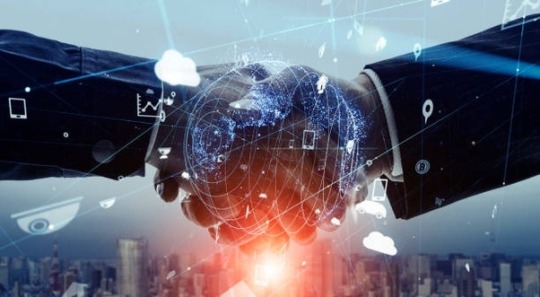
By combining these strategies, organizations can ensure data consistency across their various systems and databases, enabling reliable and accurate data for better decision-making and operational efficiency.
#DataIntegrity#DataQuality#DataAccuracy#DataConsistency#DataReliability#DataValidation#DataAudit#DataTrust#DataProtection#DataGovernance#DataSecurity#DataCompliance#DataManagement#DataPrivacy#DataTransparency#DataVerification#DataConfidentiality#DataAnalytics#DataInsights#DataDriven
0 notes
Text
Tracking the Future: Blockchain-Enabled Waste Tracking Market to Reach $12.3B by 2034
Blockchain-Enabled Waste Tracking Market is revolutionizing sustainable waste management by integrating blockchain technology to ensure transparency, accountability, and efficiency. This innovative approach facilitates real-time tracking, secure data sharing, and immutable record-keeping across waste generation, transportation, and disposal processes.
To Request Sample Report : https://www.globalinsightservices.com/request-sample/?id=GIS10895 &utm_source=SnehaPatil&utm_medium=Article
In 2023, the market processed an impressive 320 million metric tons of waste. The industrial waste segment dominated with a 45% market share, driven by strict regulations and the need for efficient management. Municipal waste captured 30%, while hazardous waste held 25%, reflecting growing awareness and adherence to environmental compliance standards.
North America leads the market due to stringent environmental regulations and a high adoption rate of advanced technologies. Europe ranks second, fueled by strong governmental policies and a focus on sustainability, with Germany setting the benchmark through proactive waste management strategies. The Asia-Pacific region is emerging as a key growth area, driven by rapid urbanization and rising environmental awareness.
Market segmentation highlights public, private, consortium, and hybrid blockchain types, with applications spanning municipal, industrial, hazardous, e-waste, and construction waste management. Software platforms dominate the market, offering advanced tracking and data analytics, while IoT-enabled hardware solutions are gaining traction for precise monitoring.
Key players like IBM, SAP, and Oracle are driving innovation with blockchain-powered waste tracking platforms, ensuring compliance, traceability, and optimized resource recovery. Regulatory frameworks, particularly in the EU and North America, play a crucial role in shaping the market’s growth trajectory.
With a projected CAGR of 15% through 2033 and blockchain investments set to increase by 20%, the market is poised to play a pivotal role in fostering sustainability and advancing circular economy initiatives.
#BlockchainForSustainability #WasteManagementInnovation #CircularEconomy #EnvironmentalTech #IoTInWasteTracking #DataTransparency #SmartContracts #EcoSolutions #SustainableFuture #WasteTrackingRevolution #GreenTech #ZeroWasteGoals #BlockchainApplications #DigitalTransformation
0 notes
Text
Ethical Considerations in AI-Powered Digital Marketing. Balancing Automation with Privacy
In the age of AI-driven digital marketing, automation has revolutionized how businesses interact with customers, analyze data, and deliver personalized experiences. However, with great power comes great responsibility. As AI tools become more advanced, ethical considerations surrounding privacy and transparency have emerged as critical concerns.
One major challenge is maintaining customer trust while leveraging vast amounts of personal data. AI algorithms rely on data to provide insights, optimize campaigns, and predict consumer behavior. But where do we draw the line between personalized experiences and invasive surveillance? Businesses must prioritize transparency, clearly communicating how customer data is collected, stored, and used.
Another ethical dilemma involves algorithmic biases. AI systems learn from historical data, which may inadvertently include biases. Left unchecked, these biases can lead to unfair targeting or exclusion of specific groups, raising ethical and legal concerns. Regular audits and inclusive data training sets are essential for mitigating such risks.
Balancing automation with human oversight is also key. While AI can streamline processes, relying solely on automation risks losing the human touch in marketing strategies. Ethical decision-making requires a hybrid approach where AI enhances human creativity, not replaces it.
As digital marketers, we must embrace AI responsibly, ensuring it aligns with ethical standards and respects consumer privacy. By addressing these concerns head-on, we can foster trust, create meaningful connections, and build a sustainable digital future.
#DigitalMarketing #EthicalAI #AIinMarketing #PrivacyMatters #AutomationEthics #ResponsibleTech #DataTransparency #MarketingEthics #AIandPrivacy
#digital marketing#i love them smmmm#media marketing#seo company#smm#smm panel#smm2#smmagency#smmarketing#social marketing
0 notes
Link
#AutomotiveEcosystem#automotivemanufacturing#AzureKnowledge#BabyBoomers#datatransparency#Futurride#GenXers#informationtransparency#Millennials#personalization#supplychain#Sustainability#sustainablemobility#ZebraTechnologies
0 notes
Text

Contact us now to try our weighbridge software for free!
Contact us :-
9979612342 | [email protected]
#sapintegration#datatransparency#emailnotifications#weighbridge#weighbridgesoftware#weighbridgesolutions#cameraintegration#weighmast#datamanagement
0 notes
Text
Journal Quality Barchart [+series overlay] using SGRENDER procedure in SAS/STAT 14.1, also SAS Studio
/**importing data set directly from sas studio enviornment**/ PROC SQL; CREATE TABLE WORK.query AS SELECT 'type'n , avail , afford , 'row'n FROM _TEMP5.coolviz1; RUN; QUIT;
PROC DATASETS NOLIST NODETAILS; CONTENTS DATA=WORK.query OUT=WORK.details; RUN;
PROC PRINT DATA=WORK.details; RUN;
/**creating dataset for vizualization**/ data data1; set work.query;
if type = "Karunya" then delete; run;
proc freq data =data1;table type;run;
data myattrmap; input id $ value $ markercolor $ markersymbol $; datalines; myid Cloudy grey cloud myid Sunny orange sun myid Showers blue umbrella ; run;
/**running the template procedure**/
proc template; define statgraph Bar_line_2 ; begingraph; entrytitle 'Availability vs. affordability of generic essential medicines in Kerala'; layout overlay / xaxisopts=(display=(ticks tickvalues)) yaxisopts=(griddisplay=on offsetmax=0.15 offsetmin=0 linearopts= (tickvaluesequence=(start=0 end=100 increment=5))) y2axisopts=(offsetmin=0 offsetmax=0.15 linearopts= (tickvaluepriority=true tickvaluesequence=(start=0 end=1.2 increment=.2))); barchart x=type y=avail / name='a' skin=modern legendlabel="Mean percentage availability" ; barchart x=type y=afford / yaxis=y2 datatransparency=1 ; seriesplot x=type y=afford / yaxis=y2 name='b' lineattrs=graphdata1(thickness=5) datatransparency=0.3 legendlabel="Affordability in mean no. of daily wages" ; scatterplot x=type y=afford / yaxis=y2 markerattrs=graphdata1(symbol=circlefilled size=15); scatterplot x=type y=afford / yaxis=y2 markerattrs=(symbol=circlefilled size=11 color=white);
discretelegend 'a' 'b' / location=outside valign=bottom halign=right across=1; endlayout; endgraph; end; run;
/**running proc sgrender procedure**/
ods graphics / reset width=4in height=4.0in imagename='Bar_Line_GTL_2' noborder ; proc sgrender data=data1 template=Bar_line_2; run;
Where to get help?
1. Graphically speaking is a forum for SAS graph users, check out this website, https://blogs.sas.com/content/graphicallyspeaking/. I am a massive fan of Sanjay Matange. Make sure to check his graph templates: https://blogs.sas.com/content/author/sanjaymatange/page/2/. I usually start with his set of codes and tweak and turn from there.
2. SAS community forum: check out what others have posted about your query
https://communities.sas.com/
0 notes
Text
Ginni Rometty zum Weltwirtschaftsforum: Unsere Verantwortung für die Daten
@GinniRometty zum #Weltwirtschaftsforum: Unsere Verantwortung für die Daten #DataTransparency #DataPrivacy
Derzeit findet das Weltwirtschaftsforum, das World Economic Forum, in Davos zwischen dem heutigen 23. und dem 26. Januar statt. Hiersind weitergehende Informationen und der Live Stream zu finden. Zu Forum sind 3000 Teilnehmer, darunter 70 Staats- und Regierungschefs und rund 1900 Konzernlenker angemeldet, darunter Bundeskanzlerin Merkel, Frankreichs Präsident Macron … und IBM CEO Ginni Rometty,…
View On WordPress
#Data Privacy#Datenschutz#Datentransparenz#featured;#Ginni Rometty#Künstliche Intelligenz#KI#World Economic Forum
0 notes
Text
Schema Management and Data Lineage Tracking in Metadata Tools
Metadata Management Tools are essential components of information management systems, enabling organizations to effectively organize, categorize, and manage metadata across diverse datasets and databases.
Metadata, which includes descriptive information about data elements, such as data types, formats, and relationships, plays a crucial role in facilitating data discovery, integration, and governance. Metadata Management Tools offer capabilities such as metadata extraction, schema management, and data lineage tracking to ensure data quality, consistency, and reliability throughout its lifecycle. These tools employ advanced algorithms and machine learning techniques to automate metadata extraction from various sources, including structured and unstructured data, enabling comprehensive data cataloging and indexing. Moreover, metadata enrichment functionalities enhance metadata with additional contextual information, such as business glossaries, data classifications, and regulatory compliance tags, ensuring that data assets are properly understood and utilized across the organization. Metadata Management Tools also support data governance initiatives by establishing policies, standards, and workflows for metadata creation, validation, and access control. Integration with data governance platforms and master data management (MDM) systems ensures alignment with organizational data policies and regulatory requirements. As organizations increasingly rely on data-driven insights for decision-making, Metadata Management Tools are instrumental in promoting data transparency, enhancing data lineage, and supporting effective data stewardship practices.#MetadataManagement #DataGovernance #DataQuality #DataCatalog #MachineLearning #AI #DataLineage #DataTransparency #DataInsights #DataStewardship #DataManagement #MDM #RegulatoryCompliance #BusinessGlossary #MetadataEnrichment #SchemaManagement #InformationManagement #DataIntegration #DataDiscovery #DataAssets #BigData #TechInnovation #DataAnalytics #DataDrivenDecisions #DigitalTransformation
0 notes
Text

Lab digitization is the easiest way to track accurate operations in your lab. Contact us now to know more and get a free demo!
Contact Us :- [email protected] +91 99796 12342 https://endel.digital/contact-us/
#datatransparency #DataManagement #laboratorymanagement #ResearchAndDevelopment #automationlaboratory #dataanalysis #LaboratorySolutions #labmast
0 notes