#textannotation
Explore tagged Tumblr posts
Text
Beginner's Guide to Text Annotation and its Role in NLP
In the rapidly evolving field of NLP, text annotation is key to enabling machines to comprehend language. EnFuse Solutions India offers expert services to help businesses achieve precise, efficient NLP applications and meet growing demands for intelligent systems with excellence.
#TextAnnotation#TextAnnotationGuide#NLPSolutions#TextLabeling#DataAnnotationServices#AnnotationForNLP#NaturalLanguageProcessing#MachineLearningAnnotation#LabeledTextData#NLPTrainingData#TextDataAnnotation#EnFuseSolutions#EnFuseSolutionsIndia
0 notes
Text
Who are the leading players in the data annotation tools market?
Data annotation market is highly competitive, with a mix of established companies and innovative newcomers offering a variety of tools and services. Depending on your specific needs—whether it's image, video, or text annotation—companies like EnFuse Solutions can provide the right solutions to support your AI initiatives.
#DataAnnotation#DataAnnotationTools#DataLabeling#AnnotationServices#AIDataLabeling#MachineLearningData#TrainingData#ImageAnnotation#TextAnnotation#VideoAnnotation#DataTagging#AIModelTraining#AnnotationMarketLeaders#EnFuseSolutions#EnFuseSolutionsIndia
0 notes
Text
What are the types of data annotation?
Choosing the right type of data annotation is essential for the success of any AI project. It not only improves the accuracy of machine learning models but also enhances the overall efficiency and reliability of the system. EnFuse Solutions stands out as the best data annotation service provider in India. Whether text, image, video, audio, or 3D data annotation, EnFuse Solutions delivers top-notch services to meet specific project needs.
#DataAnnotation#TypesOfDataAnnotation#DataLabeling#AIDataAnnotation#MLDataAnnotation#ImageAnnotation#TextAnnotation#VideoAnnotation#AudioAnnotation#SemanticAnnotation#BoundingBoxAnnotation#PolygonAnnotation#DataTagging#AnnotationForAI#AnnotationForML#TrainingDataAnnotation#DataAnnotationServices#AIAnnotationServices#DataAnnotationIndia#EnFuseAIAnnotation#EnFuseDataAnnotation#EnFuseSolutions
0 notes
Text
Understanding The Different Types Of Data Annotation: Text, Image, Audio, And Video
Data annotation plays a vital role in the advancement of AI and ML technologies. In this article, we will explore the various types of data annotation, including text, image, audio, and video, and their importance in AI and ML applications. Companies like EnFuse Solutions India provide high-quality data annotation services, contributing to the advancement of AI technologies across various industries.
#DataAnnotationTypes#TextAnnotation#ImageAnnotation#AudioAnnotation#VideoAnnotation#AIDataLabelingTools#EnFuseSolutionsIndia
0 notes
Text
Top 5 Quality Control Metrics in Text Annotation

High quality text annotation is important for preparing accurate training data for building AI models and to help them understand and process human language in a better way. However, ensuring the accuracy and reliability of annotated data requires stringent quality control.
0 notes
Text

Text Annotation Services for OCR
Are you struggling to make sense of unstructured text data? Elevate your OCR capabilities with our professional text annotation services!
Why Choose Us?
:: Precision & Accuracy: Our dedicated team ensures high-quality, error-free annotations that enhance the accuracy of your OCR models.
:: Scalability: Whether you have hundreds or thousands of documents, we can scale our services to meet your needs without compromising on quality.
:: Fast Turnaround: We understand the importance of time. Our streamlined processes deliver your annotated data quickly, so you can keep your projects moving.
:: Custom Solutions: Every project is unique. We offer tailored annotation solutions to fit your specific requirements and objectives.
:: Secure & Confidential: Your data security is our priority. We adhere to strict confidentiality standards to protect your sensitive information.
Contact us to learn more about how our text annotation services can help you unlock the full potential of your data. Visit our website or call us now for a free consultation!
https://www.wisepl.com | [email protected]
#textannotation#textlabeling#ocrdetection#annotationservices#datalabeling#imageannotation#machinelearning#computervision#dataannotation#ai#wisepl
0 notes
Text
Data Annotation for Fine-tuning Large Language Models(LLMs)
The beginning of ChatGPT and AI-generated text, about which everyone is now raving, occurred at the end of 2022. We always find new ways to push the limits of what we once thought was feasible as technology develops. One example of how we are using technology to make increasingly intelligent and sophisticated software is large language models. One of the most significant and often used tools in natural language processing nowadays is large language models (LLMs). LLMs allow machines to comprehend and produce text in a manner that is comparable to how people communicate. They are being used in a wide range of consumer and business applications, including chatbots, sentiment analysis, content development, and language translation.
What is a large language model (LLM)?
In simple terms, a language model is a system that understands and predicts human language. A large language model is an advanced artificial intelligence system that processes, understands, and generates human-like text based on massive amounts of data. These models are typically built using deep learning techniques, such as neural networks, and are trained on extensive datasets that include text from a broad range, such as books and websites, for natural language processing.
One of the critical aspects of a large language model is its ability to understand the context and generate coherent, relevant responses based on the input provided. The size of the model, in terms of the number of parameters and layers, allows it to capture intricate relationships and patterns within the text.
While analyzing large amounts of text data in order to fulfill this goal, language models acquire knowledge about the vocabulary, grammar, and semantic properties of a language. They capture the statistical patterns and dependencies present in a language. It makes AI-powered machines understand the user’s needs and personalize results according to those needs. Here’s how the large language model works:
1. LLMs need massive datasets to train AI models. These datasets are collected from different sources like blogs, research papers, and social media.
2. The collected data is cleaned and converted into computer language, making it easier for LLMs to train machines.
3. Training machines involves exposing them to the input data and fine-tuning its parameters using different deep-learning techniques.
4. LLMs sometimes use neural networks to train machines. A neural network comprises connected nodes that allow the model to understand complex relationships between words and the context of the text.
Need of Fine Tuning LLMs
Our capacity to process human language has improved as large language models (LLMs) have become more widely used. However, their generic training frequently yields below-average performance for particular tasks. LLMs are customized using fine-tuning techniques to meet the particular needs of various application domains, hence overcoming this constraint. Numerous top-notch open-source LLMs have been created thanks to the work of the AI community, including but not exclusive to Open LLaMA, Falcon, StableLM, and Pythia. These models can be fine-tuned using a unique instruction dataset to be customized for your particular goal, such as teaching a chatbot to respond to questions about finances.
Fine-tuning a large language model involves adjusting and adapting a pre-trained model to perform specific tasks or cater to a particular domain more effectively. The process usually entails training the model further on a targeted dataset that is relevant to the desired task or subject matter. The original large language model is pre-trained on vast amounts of diverse text data, which helps it to learn general language understanding, grammar, and context. Fine-tuning leverages this general knowledge and refines the model to achieve better performance and understanding in a specific domain.
Fine-tuning a large language model (LLM) is a meticulous process that goes beyond simple parameter adjustments. It involves careful planning, a clear understanding of the task at hand, and an informed approach to model training. Let's delve into the process step by step:
1. Identify the Task and Gather the Relevant Dataset -The first step is to identify the specific task or application for which you want to fine-tune the LLM. This could be sentiment analysis, named entity recognition, or text classification, among others. Once the task is defined, gather a relevant dataset that aligns with the task's objectives and covers a wide range of examples.
2. Preprocess and Annotate the Dataset -Before fine-tuning the LLM, preprocess the dataset by cleaning and formatting the text. This step may involve removing irrelevant information, standardizing the data, and handling any missing values. Additionally, annotate the dataset by labeling the text with the appropriate annotations for the task, such as sentiment labels or entity tags.
3. Initialize the LLM -Next, initialize the pre-trained LLM with the base model and its weights. This pre-trained model has been trained on vast amounts of general language data and has learned rich linguistic patterns and representations. Initializing the LLM ensures that the model has a strong foundation for further fine-tuning.
4. Fine-Tune the LLM -Fine-tuning involves training the LLM on the annotated dataset specific to the task. During this step, the LLM's parameters are updated through iterations of forward and backward propagation, optimizing the model to better understand and generate predictions for the specific task. The fine-tuning process involves carefully balancing the learning rate, batch size, and other hyperparameters to achieve optimal performance.
5. Evaluate and Iterate -After fine-tuning, it's crucial to evaluate the performance of the model using validation or test datasets. Measure key metrics such as accuracy, precision, recall, or F1 score to assess how well the model performs on the task. If necessary, iterate the process by refining the dataset, adjusting hyperparameters, or fine-tuning for additional epochs to improve the model's performance.
Data Annotation for Fine-tuning LLMs
The wonders that GPT and other large language models have come to reality due to a massive amount of labor done for annotation. To understand how large language models work, it's helpful to first look at how they are trained. Training a large language model involves feeding it large amounts of data, such as books, articles, or web pages so that it can learn the patterns and connections between words. The more data it is trained on, the better it will be at generating new content.
Data annotation is critical to tailoring large-language models for specific applications. For example, you can fine-tune the GPT model with in-depth knowledge of your business or industry. This way, you can create a ChatGPT-like chatbot to engage your customers with updated product knowledge. Data annotation plays a critical role in addressing the limitations of large language models (LLMs) and fine-tuning them for specific applications. Here's why data annotation is essential:
1. Specialized Tasks: LLMs by themselves cannot perform specialized or business-specific tasks. Data annotation allows the customization of LLMs to understand and generate accurate predictions in domains or industries with specific requirements. By annotating data relevant to the target application, LLMs can be trained to provide specialized responses or perform specific tasks effectively.
2. Bias Mitigation: LLMs are susceptible to biases present in the data they are trained on, which can impact the accuracy and fairness of their responses. Through data annotation, biases can be identified and mitigated. Annotators can carefully curate the training data, ensuring a balanced representation and minimizing biases that may lead to unfair predictions or discriminatory behavior.
3. Quality Control: Data annotation enables quality control by ensuring that LLMs generate appropriate and accurate responses. By carefully reviewing and annotating the data, annotators can identify and rectify any inappropriate or misleading information. This helps improve the reliability and trustworthiness of the LLMs in practical applications.
4. Compliance and Regulation: Data annotation allows for the inclusion of compliance measures and regulations specific to an industry or domain. By annotating data with legal, ethical, or regulatory considerations, LLMs can be trained to provide responses that adhere to industry standards and guidelines, ensuring compliance and avoiding potential legal or reputational risks.
Final thoughts
The process of fine-tuning large language models (LLMs) has proven to be essential for achieving optimal performance in specific applications. The ability to adapt pre-trained LLMs to perform specialized tasks with high accuracy has unlocked new possibilities in natural language processing. As we continue to explore the potential of fine-tuning LLMs, it is clear that this technique has the power to revolutionize the way we interact with language in various domains.
If you are seeking to fine-tune an LLM for your specific application, TagX is here to help. We have the expertise and resources to provide relevant datasets tailored to your task, enabling you to optimize the performance of your models. Contact us today to explore how our data solutions can assist you in achieving remarkable results in natural language processing and take your applications to new heights.
0 notes
Photo
Data Annotation Services and Its type.
For more follow Suntec.ai
0 notes
Photo
Industries that rely on data labeling find a new and different business approach. One such industry is real estate.
Data labeling offers valuable insights into real estate and helps predict property value, rental info, price, and other critical parameters.
Want to know more? Click here to read the blog, which explains the importance of data labeling in the real estate sector.
https://bit.ly/3M9COvX
#Springbord#RealEstate#DataLabeling#DataAnnotation#ImageAnnotation#TextAnnotation#Services#Outsourcing
0 notes
Text
Approaches to text annotation in PDF - Sumnotes
Reading is an essential part of college and university courses, but it can be difficult to remember all the information you read. Marking up your texts with notes and highlights will help make sure that key points stand out as well as provide helpful study aids for future readings. Filing away entire books in one sitting might feel like overkill at times - no matter how many pages there are.
There are many ways to engage with what you're reading, and one way is through annotations. By highlighting key phrases or making notes about particular ideas in your text as well as asking questions that lead toward more insight for the paper's topic then writing can become an active process rather than just background work on assignments.
A well-annotated text will help you better understand the author's argument by identifying key information, tracing their thought process, and introducing some of your own thoughts. Before making major annotations, it is recommended to read through a single time before making annotations. Doing so improves efficiency since readers become more familiar with what needs to be annotated.
There are several ways of text annotation in practice such as highlighting, paraphrasing, underlining, and commenting which are common ways of displaying text for emphasis. Let's dive into the methods and see how each of them works.
Highlighting: highlighting or underlining keywords and phrases is a common method of annotating written text. This method is an easy way to review materials, especially for exam preparation and research papers. Highlights are also useful when you want to emphasize specific parts within your text that can be cited in other places due to their relevance with what you are discussing or working on.
But what is the trouble with highlighting text? The trouble with highlighting is that it's easy to do a lot at once. You can highlight too much, and then when you come back later your notes may not have any point or context in them because they were just scattered around by chance while reading through everything else on the page. This would definitely mess up things and create an obstacle. We can easily say that worse than doing nothing is "over-highlighting".
The best way to keep track of what you read is by highlighting the important parts. Make your own notes on each section, or highlight phrases and words that come up again in other annotations for easy reference later.
Paraphrasing: in order to be able to capture the meaning of important ideas, one must become adept at paraphrasing them. This technique will help you solidify your understanding and prepare for any writing that may rely on reading comprehension in this manner by allowing for notes about key points within texts or extracts from books; acting as a quick summary that can then be easily adapted into other materials. Paraphrasing summarises what you think the author meant in his own words.
Description: using a descriptive outline is very helpful for organizing your thoughts when annotating text. It allows you not only to see where the main ideas are but also provides other types of support such as explanations and details which will make it easier to get everything out on paper without losing any important information in between sentences.
The process of making a descriptive outline not only helps you follow the construction and organization behind your text but also identifies which parts work together well. It can be used as an effective tool for understanding how writers argue their points or think about problems when they're writing expository texts.
Commenting: Annotations can go beyond understanding the text's meaning by noting the reactions of the reader. This is an excellent way to begin formulating your own ideas for writing assignments based on the topic. Comments respond with additional arguments or explanations when someone asks about something specific; it can also serve as note-taking throughout your readings if that helps convey information more efficiently during class time.
These are the main approaches you can use while reading a book, writing a research paper, preparing for an exam, or working on a legal case by going through thousands and thousands of reports.
SUMNOTES is one of the most popular tools that can help you with annotation management. Now is the best time to SUBSCRIBE as you can do it with 15% OFF. It is enough to use the SUMNOTES2022 promo code at the checkout.
0 notes
Link
Shaip is the best company to provide Text Annotation, Video Annotation, Image Annotation Services in all around the world. We offer the best-in-class text annotation services by leveraging world-class annotation services for Natural Language Processing (NLP) in machine learning.
#textannotation#TextAnnotationServices#TextAnnotationServicesOutsourcing#textannotationmachinelearning#AItextdatalabelingcompany#textannotationtools#documentannotation#textclassification
0 notes
Text
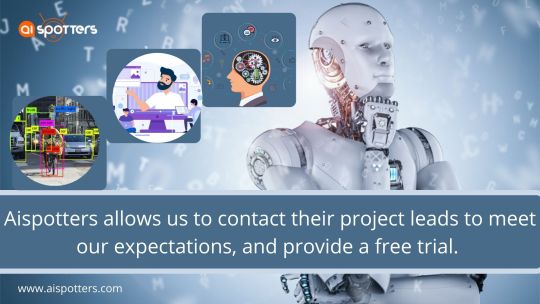
#machinelearning#deeplearning#ai#artificialintelligenceservices#humanintelligenceservices#imageannotation#audioannotation#nlp#textannotation#datalabeling#dataannotation#videoannotation#aispotters#AISPOTTERS#datasets
0 notes
Photo
"Aispotters provides high-quality annotated images with a futuristic solution to track all actions of humans"
#ai#aispotters#imageannotation#textannotation#video annotation services#audioannotation#machinelearning#deeplearning#natural language processing (nlp)#humanintelligenceservices#artificialinteligence#artificialintelligenceservices
0 notes
Photo

When you buy a used book to mark up and some has already marked it up. Bonus if it's the story that was the reason you bought the book in the first place. #whatwetalkaboitwhenwetalkaboutlove #raymondcarver #shortstory #usedbooks #bookstagram #textannotation
1 note
·
View note
Text
#machinelearning #deeplearning #ai #artificialintelligenceservices #humanintelligenceservices #imageannotation #audioannotation #nlp #textannotation #datalabeling #dataannotation #videoannotation #aispotters #AISPOTTERS #datasets
1 note
·
View note
Photo

License Plate Recognition Services
Wisepl annotating and labeling license plate numbers on vehicles captured in images or videos. This can be useful for tasks such as automated toll collection, parking management, or law enforcement.
#boundingbox#machinelearning#ai#computervision#imageannotation#dataannotation#datalabeling#numberplate annotation#textannotation#vehicles#cars#adas#labelingservices#wisepl
0 notes