Don't wanna be here? Send us removal request.
Text

Apache Iceberg and Delta Lake are powerful solutions with unique features and advantages for handling large-scale datasets. The following comparison highlights how to choose from these solutions based on specific business needs and data infrastructure.
Apache Iceberg vs. Delta Lake: The Better Solution for Your Data Architecture?
0 notes
Text
AI-Powered Agentic Workflows: The Paradigm Shift in Business OperationsFor businesses looking to be more agile and efficient, AI-driven agentic workflows stand out as truly game-changing, allowing organizations to automate routine activities and focus on more strategic matters. These workflows, powered by AI agents, adapt in real-time, improving efficiency, decision-making, and innovation across industries like finance, healthcare, and manufacturing. This shift is empowering businesses to stay ahead in a fast-changing environment.Read more on how AI is driving the next paradigm shift in business operations.https://bit.ly/497PRJM

0 notes
Text
Generative AI Integration to Enhance Efficiency and Revolutionize Patent Analysis
Discover how GenAI integration revolutionized patent analysis, boosting efficiency and accuracy. Read the full case study : https://www.contata.com/case-studies/revolutionized-patent-analysis-with-gpt-4-for-an-ip-firm/

0 notes
Text
Enhancing Knowledge Management with Generative AI Integration
Delve into the future of search with our latest blog! Discover how AI-powered search engines are transforming information retrieval and enhancing user experiences.
Read Complete Blog-
Knowledge Management with Generative AI

0 notes
Text
Overcoming Data Management Challenges with Apache NiFi: An Open-Source Platform
Over the years, Apache NiFi has emerged as a robust and scalable data platform, empowering businesses to minimize expenses and licensing complexities while still leveraging powerful data integration and processing capabilities. In this blog, we will learn about various challenges associated with proprietary data platforms and how Apache NiFi helps organizations overcome them.
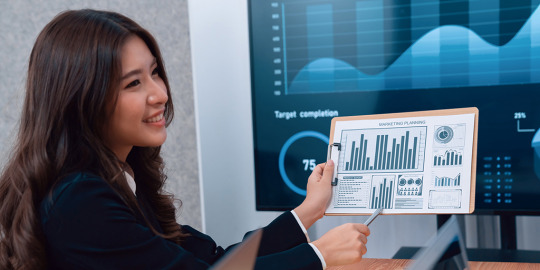
0 notes
Text
Digital Transformation of a Realty Company Looking to Optimize Data Collection
0 notes
Text
Leveraging Data Analytics and AI to Enhance Customer Experience & Boost Sales in the CPG Industry

The landscape of marketing has undergone a significant transformation over the years with data taking center stage in virtually all marketing initiatives. In contrast to conventional marketing, which was based on instincts, data-driven marketing or data-centric marketing strives to identify and reach the right customers.
Read Complete Blog- Leveraging Data Analytics and AI
0 notes
Text
Unleashing Synthetic Data: Fueling Innovation While Safeguarding Confidentiality
Modern organizations encounter data-related challenges such as privacy concerns and limited data diversity, which can significantly impede their ability to develop effective decision-making and growth strategies in two key areas.Read Complete Article

0 notes
Text
Data Science & Application Development- Contata Solutions

Contata Solutions, founded in 2000, is aData Science Serviceprovider & Software Application Development company headquartered in Minneapolis, US with 5 offices including offshore centers in India. We have a Software Engineering Group based in the Delhi-NCR region, India; a back-office support group based in Nagpur & Indore, India; and a sales office in Stockholm, Sweden.
Since 2000, Contata has maintained a legacy of a loyal client base distributed across the US and served customers in Norway, Sweden, Belgium, France, Netherlands & other EU countries across various business domains and verticals, primarily e-governance, Oil & Gas, Media, Pharma, IP/Legal, Retail, Automobile, and Manufacturing among others.
Our teams of Data Scientists (Ph.D. holders from top universities), Machine Learning experts, Algorithm writers, Solution Architects, and Development experts with enhanced and latest technology platforms make us a reliable, full-cycle Software Development and Data Intelligence company.
Most Demanded Services
Data Audit Services-
Get a clear picture of the quality and coverage of your data.
Have data but unsure if it can be trusted? Data auditing allows you to find out how good your data is and what can be leveraged for useful analytics. We can help you easily understand your data and track the lineage across systems via simple data profile visualizations. Also, auditing can help you comply with standards like GDPR, CCPA, and HIPPA to understand the origin, storage, and use of sensitive data you may have.
Data Science Services-
Make Your Business Data-Driven
We help aggregate your data from various sources, transforming and cleaning it in the process, so it offers an integrated 360-degree view of your organization. Reporting and machine learning tools can then be layered on that data to glean diagnostic and prescriptive insights to drive improvements for your business.
Data Engineering Consulting Services-
Design and build data repositories aggregating data across your systems to support 360-degree analysis.
We help organizations unlock the value of data received from disparate sources and gain critical business insights, translating a competitive advantage. Contata helps you understand and apply your data, ensuring security, quality, and regulatory compliance - fast and in real- time.
Business Intelligence Engineering-
Take your Business Intelligence to the next level.
There are a variety of approaches and tools out there for implementing analytics dashboards for your business. Depending on your data environment and business needs, the tools can be implemented with various levels of data-blending, filtering, and bucketing. Contata can help analyze your data and recommend a tool and implementation strategy to meet your needs.
Machine Learning Services-
Drive your business with strategies informed by data-mining and predictive modeling techniques, to optimize your business performance.
Artificial Intelligence (AI) and Machine Learning (ML) are at the core of digital transformation. By leveraging these technologies, businesses can improve business processes, simplify decision-making, generate actionable insights, and create a new business model. Contata’s digital-led transformation processes help companies run more efficient, agile, and profitable operations.
Customer Data Analytics Services-
Map the optimal path for your data analytics and AI projects.
Often the information you have on your prospects and customers is sparse – limited to basic contact information. We can help enrich the customer/ prospect information in your CRM by appending additional demographic and contact information of choice.
Text Document Analytics Services-
Document & Text Analytics process documents at scale, automatically categorizing them and enabling high-level text searches.
Contata provides you with advanced text analytics solutions to help you gain insights into your data and make data-driven decisions to meet the needs of businesses and individuals, including sentiment analysis, entity recognition, key phrase extraction, and more.
Oil And Gas Analytics Services-
Advanced analytics in Oil & Gas and explore use cases and perspectives.
Our Oil and Gas analytics solution uses cutting-edge algorithms and machine learning techniques to analyze large volumes of data, providing you with real-time insights into your operations. Whether you are an exploration and production company, a pipeline operator, or a service provider, our solutions can help you make informed decisions and improve your bottom line.
Software Application Development-
Build Re-Imagined Business and Consumer Apps
Our Application Development services bring innovation and business value throughout the digital value chain. Lean on us for all or any of the following services to help bring your vision to reality.
Software Quality Assurance-
Current market requirement of high-quality products and solutions.
In today’s hyper-connected world, quality makes a big difference between success and failure. In fact, challenges such as the inability to assess and adopt newer tools and technologies, longer development and test cycles, and limited expertise for specialized testing — affect system quality and reliability.
Contata Solutions understands this critical challenge and offers an entire gamut of quality testing services that help customers significantly improve their applications' quality, increase productivity, and reduce testing times.
Cloud Application Development-
Leverage cloud infrastructure for faster builds, reduced costs, and increased reliability. Contata provides experienced, savvy Dev-Ops engineers who build custom solutions leveraging AWS, Microsoft Azure, private cloud, and other cloud suppliers. By building in continuous integration, delivery, and performance testing, enterprises can realize always-on availability, workload mobility. and end-to-end visibility with their cloud infrastructures.
Digital Transformation Solutions-
Digitize documents and automate processes.
Review and analyze current processes in your organization to identify gaps and inefficiencies that result in reduced speed and higher costs. Formalize business rules guiding your process flows and explore opportunities for automating tasks performed manually.
Product Engineering Services -
Leverage our experience in all phases of the product lifecycle.
From product conceptualization to development and deployment, Contata comprehensively manages the entire product lifecycle. We can take the engineering aspects off your hands so you can focus on other critical business
operations. Our comprehensive product lifecycle management helps you:
Get to market faster
Deploy higher quality product
Lower compliance risk
Focus your management and marketing
Oil & Gas Industry Software Solution-
We have industry experts dedicated to the Oil & Gas industry.
Contata’s deep domain expertise of over 20 years in the Oil and Gas industry delivers the best practices and methodologies equipped with the latest tools and technologies. Our experts help you to navigate through your journey right from
the exploration, to the production, and the operation lifecycle.
Connect With Us:
To have a discussion with our consultant, reach out to us by sending an email to [email protected].
#data science consulting services#data science services company#data engineering consulting services#custom application development services
0 notes
Link
Data Science & Software Application Development Services-Contata Solutions.pdf
0 notes
Text
0 notes
Text
Security Challenges with the Bots in the Modern Digital Age
Bots are everywhere. You’ve likely encountered them so many times in your daily internet surfing or enquiring about any services like when you visit a website for a bank, credit card company, car sales website, or even a software business. Bots are all the rage these days. This is because they can answer your customers’ online inquiries 24 X 7 whether the customer representative is offline or online. It can also automate your routine task anytime with more accuracy and efficiency. So, what is a Bot? A Bot is an essential software application powered by Artificial Intelligence that runs automated tasks to meet the customer’s expectations and increasing demands, ease of operations, and cost-optimization across industries and businesses. Today’s digitally empowered ecosystem is looking for a solution that exceeds the end user’s expectations and reduces error. Gartner’s recent study predicts 69% of routine work currently done by managers will be fully automated by 2024. According to Global Market Insights, the market size for chatbots worldwide would be over $1.3 billion by 2024.
The application maker and programmers worldwide are concerned about how to make it more user-friendly, cost-effective, intelligent, and secure. In today’s data-filled environment, companies are running applications with multi-touchpoints and public and private data processing. These include the data collected from the customers as well as internal data of the companies. Data security is of topmost concern as users could very well be giving away private and personal information to an application that collects data. To avoid data breaches, a company must set up cumbersome security processes and respect them. In a nutshell, an essential question is: Can users trust this Bot? And it leads to another question about the data protection challenges.
Automated Attacks:
Sophisticated automated attacks from Skewing, Expediting, Scraping, Credential Stuffing, and Brute Force are all prevalent today. Automation is a ubiquitous talking point throughout boardrooms. Equally, attackers have embraced automation to create new types of attacks to reach far and wide with minimal effort!
Data Loss/Leakage:
Web scraping is a common attack whereby BOTS scrape relevant information from a website. e.g., prices from an Airline, eCommerce, or Hotel website
BOTS can launch automated attacks
Fingerprint on application or server
Check the existence of a vulnerability in an application or backend infrastructure
Check the existence of the user account/id
Mass password resets
Download/Archive publicly available information on a website
Distinguishing Good vs. Bad Bot:
There are good BOTS like search engines, partner APIs, etc
Biz needs to allow these good BOTS for obvious reasons
There are bad BOTS that collect information on site, try credential stuffing, scrape information, purposefully skew business forecasting, etc.
It is difficult to identify and differentiate good vs. bad BOTS and restrict access only to good BOTS
After the implementation of the GDPR, the Data Protection Officer must take consideration mapping the data of your company, determining the following:
What types of data are collected? Which is personal data? Are these data-sensitive?
Does the Bot take the affirmation before collecting the data from the users?
Where are data stored? What are the security measures?
Who can access this data?
Once the data mapping has been accomplished, the Data Protection Officer will have to implement procedures to ensure data security. Security professionals understand where it is vulnerable and how we can best protect against exploitation. There are some security measures and preventive steps to ensure the security of the Bot application.
Layered/Structured Solution for BOT Protection:
Proactive Bot Defence
Bot Signature
IP Geolocation
IP Reputation
TPS-Based DOS Protection
L7 Behavioural Detection& Mitigation
URL Flow
Programmability
Conclusion:
Bots are here to stay. The future application is accomplishing the automated task with minimal error across diverse business functions and consumer-centric applications. We at Contata, are helping enterprises understand their business needs and implement intelligent and user-friendly Bots with security at the top of the mind.
.
To learn how you can secure the Bot application for robust security, contact us at [email protected]
0 notes
Text
From Data Lake to Data Mesh – The Paradigm Twist

In the age of self-service business intelligence, nearly every company is at some stage of transitioning to becoming a data-first company. To make the transition a successful one, companies need to undertake this journey with a level of sophistication that involves strategic thinking and purposeful execution.
A Gartner report predicted that “By 2024, 75% of organizations will have established a centralized data and analytics (D&A) center of excellence to support federated D&A initiatives and prevent enterprise failure.”
Indeed, this is not surprising given that the current data architectures are not well equipped to handle data’s ubiquitous and increasingly complex, interconnected nature.
While attempting to address this issue, many companies’ questions remain: How can we best build our data architecture to maximize data efficiency for the growing complexity of data and its use cases?
In this article, we discuss the common data management challenges faced by organizations, DataLakes as an improvement over traditional management tools, and the shift towards Data-Mesh architecture.
Key Challenges in Data Management
Despite the rapid advancement in hardware technologies and architectural models over the past several years, data management still suffers in different measures from:
Ubiquity: With new domains being integrated into the enterprise and third-party data being piped in for analytics, the quantity, and types of data continue to expand (structured/unstructured, batch/streaming…). This requires an intelligent system that can efficiently transform the data from any one of many original formats to a normalized one either at the time of ingestion or when served up for consumption.
Ownership: Sourcing experts best understand the data semantics but are often removed from the downstream processes of ingesting, cleaning, and deploying it for analytics. This creates major bottlenecks as the data engineers mediate between consumers and producers of the data on issues relating to quality and semantics.
Redundancy: Data required for any analytics purpose must be brought together into a unified store. Different consumer systems creating their own analytics repositories result in duplication of data and mismatch due to a lack of synchronization across systems.
Infrastructure: The tools required to ingest, transform, and store data for analytics uses are complex and require experienced data engineers, creating a bottleneck in setting up and maintaining repositories with multiple sources.
Scope: Existing systems are designed with current needs and some expectation of new sources. As business users use the system and begin to apply it to different purposes, user needs evolve and expand. Furthermore, with analytics becoming the primary driving force, organizations often want to bring in more data from disparate sources to aid in the existing analytics and build additional capabilities.
Discoverability & Addressability: The lack of a centrally maintained discovery catalog in traditional systems is a major hindrance to locating and contextualizing the different types of data needed for complex analyses.
The Lake Approach
The Data-Lake architecture evolved as a potential solution to the issues companies faced in their efforts toward building enterprise analytics-ready Data Warehouses.
What is the Data-Lake architecture?
The Data Lake architecture promotes a monolithic, centralized, and domain-agnostic data management system. The premise of this model is to house data relating to all logical domains and use cases for a company in a single centralized store to which all concerned parties have access.
Since data is made available in its source form in a Data Lake repository, it gets registered in the Lake as soon as it becomes available from the source systems. Downstream consumers can then deploy processes to identify, explore, clean, merge or otherwise repurpose the relevant data to generate meaningful insights. Since such processes are context-specific, a separate pipeline is required to handle each consumer need.
This architecture is well equipped to handle real-time streaming data, and unification of batch and stream data, and can easily be deployed and maintained on the cloud, making it an easy choice instead of expensive Data Warehouse solutions.
Where the Data Lake falls short
Complexity: With the ubiquitous availability of data, the ability to bring it all into a centralized storage system is easy. But harmonizing data from different sources as part of the enrichment process to pull insights becomes a daunting task, and one that gets more complex as the volume and types of data expand.
Architectural Decomposition: Scaling the data platform is handled at the organization level by specialist teams, each responsible for a part of the enrichment (ETL or ELT) lifecycle. This creates a critical dependency bottleneck and lack of flexibility.
Operational Challenges: Ownership of the centralized data system is in the hands of a team of people having specialized skillset for handling data, but who are completely siloed from the operational units the data is sourced from. This isolation from business and domain knowledge puts engineers in a position to work with data without context, thus resulting in disconnected source teams, frustrated consumers, and an over-stretched data team.
Management: Even for skilled engineers, Data Lakes are hard to manage. Ensuring that the host infrastructure has the capacity to scale, dealing with redundant data, securing all the data, etc., are all complex tasks when the data is being stored and managed centrally.
Accountability & Trust: The distributed ownership of data makes it difficult for consumers to have faith in the quality and integrity of the data. Moreover, identifying the right point of contact for issue resolution is an added challenge.
All of the above-mentioned inherent limitations in the Data Lake’s architecture and organizational structure result in systems that do not scale well and do not always deliver on the promise of creating a data-driven organization.
The Mesh Approach
The Data Mesh approach, introduced by Zhamak Dehghani, evolved out of more holistic thinking about the needs and challenges in the data management process and where prior approaches have failed.
These principles together promote the idea of distributed data products oriented around domains and owned by independent cross-functional teams using self-serve shared infrastructure as a platform to operate on the data, all under centralized governance and standardization for interoperability.
Data Domains
Data as Product
Shared Infrastructure
Federated Governance
Implementing a Data-Mesh Architecture
As the world of data and operations is moving to a decentralized paradigm, deploying scalable infrastructures has become easier for organizations by leveraging cloud service providers.
All cloud service providers function on the premise of providing easily accessible infrastructure services to the end user while doing the heavy lifting of procurement, support, and orchestration.
Since the Data Mesh is more of an organizational principle, a holistic out-of-box tool to create one isn’t out there. Most cloud providers have services that only satisfy some of the requirements for building out a Data Mesh. This pushes us to get creative and implement the Data Mesh using a combination of native cloud tools along with other third-party tools in an organized and synchronous manner.
When thinking in terms of a Data Mesh, there are situations where a Data Lake or Data Warehouse still makes sense. Not as the overarching central entity, but as a node in the Mesh, for purposes such as combining data from two domains for intermediate or aggregate analysis. Similarly, if one has an existing Data Lake, it need not be completely discarded while migrating to a Data Mesh architecture. Rather, the Lake can continue to be used while reducing its complexity down to a single domain’s use case.
‘To Mesh or Not to Mesh’
Choosing a data strategy stems from the need to analyze data without having to move or transform it via complex extract, transform, and load (ETL or ELT) pipelines.
For many organizations, the decision to make is whether centralizing data management in a Data Lake is the right approach or is a distributed Data Mesh architecture the right fit for their organization.
Neither of the two options is wrong, but the choice of which one to adopt should account for multiple aspects of an organization’s needs such as:
How many data sources exist?
How many analysts, engineers, and product managers does the data team have?
How many functional teams rely on the data for decision-making?
How frequently does the data engineering team find itself in a bottleneck?
How important is data governance for the organization?
A Data Mesh would be helpful for organizations that store data across multiple databases, have a single team handling many data sources, and have a constant need to experiment with data at a rapid rate.
Whereas organizations working with the mindset to upskill employees across the enterprise to become citizen data scientists and looking for a solution that enables data-querying where it lives, in its original form, might benefit more from a Data Lake. Whereas organizations working with the mindset to upskill employees across the enterprise to become citizen data scientists and looking for a solution that enables data-querying where it lives, in its original form, might benefit more from a Data Lake. Know More- Data Engineering Services
0 notes