#AI and machine learning
Explore tagged Tumblr posts
Text
I hate the way people seem to view ai as some kind of terrible societal ill and desperately hope that people stop working on it forever, when in fact ai is very much JUST a tool, and the fact that it’s being used for terrible things is because the humans who use it make it do those things,,,,,
Like ai art isn’t made by some sentient robot, it’s made by people using the ai as a tool! ChatGPT isn’t writing things on it’s own, people are telling it to do things. Viewing ai as the problem and not the fact that we live in a society where ai is used for bad is ridiculous. It’s a tool, not a conscious thing. It doesn’t make any decisions.
#I hate people who say that they hate ai as if it is not some of the most insane human creation ever made#ai and machine learning#ai
51 notes
·
View notes
Text
(Source)
73K notes
·
View notes
Text
Geospatial Solutions Market Set to Hit $2401.1 Billion by 2035
The global market for Geospatial Solutions is expected to experience significant growth, with industry revenue projected to rise from $488.0 billion in 2023 to $2401.1 billion by 2035. This reflects a compound annual growth rate (CAGR) of 14.2% from 2023 to 2035.
Detailed Analysis - https://datastringconsulting.com/industry-analysis/geospatial-solutions-market-research-report
Geospatial solutions are essential across a wide range of applications, including precision agriculture, urban planning, logistics and transportation, as well as defense and security. The market's growth is primarily driven by the increasing adoption of geospatial technologies in key sectors such as agriculture, urban development, and defense.
Competitive Landscape and Market Leadership
The Geospatial Solutions market is highly competitive, with several key players driving innovation and market penetration. Leading companies in the market include:
Esri
Hexagon Geospatial
Trimble
Topcon
HERE Technologies
Fugro
TomTom
Geo-Insights
Blue Marble Geographics
SuperMap
Mapbox
Cyient
These companies are at the forefront of advancing geospatial technologies, such as artificial intelligence (AI), machine learning, and real-time data analytics, which are helping to meet the growing demand for smarter and more efficient solutions across industries.
Key Growth Drivers and Market Opportunities
The growth of the Geospatial Solutions market is fueled by several factors:
Precision Agriculture: The growing need for efficient land use and sustainable farming practices is driving the demand for geospatial solutions in agriculture, enabling better crop management and resource allocation.
Urbanization and Smart City Initiatives: The increasing push for smart city development and urban planning requires geospatial technologies to manage infrastructure, transportation, and urban environments more efficiently.
Defense and Security: Geospatial solutions are playing a crucial role in defense and security applications, including surveillance, reconnaissance, and situational awareness.
Integration of AI and Machine Learning: The application of AI and machine learning in geospatial data analysis is enhancing the capabilities of these solutions, enabling real-time insights and decision-making.
Demand for Real-time Location Data: The growing reliance on real-time data in various sectors, including logistics, transportation, and emergency management, is driving the adoption of geospatial technologies.
Regional Dynamics and Supply Chain Evolution
North America and Asia-Pacific are the dominant regions in the Geospatial Solutions market. Both regions benefit from strong technological infrastructure, high levels of investment, and significant demand from key industries. However, emerging markets in India, Brazil, and South Africa are becoming increasingly important for market growth, driven by rapid urbanization, industrialization, and the adoption of new technologies.
Despite challenges such as high initial investments, data privacy concerns, and integration complexities, the geospatial solutions market’s supply chain—from data providers and software developers to system integrators and service providers—is expected to evolve to meet these challenges. Companies are also focusing on strategic advancements in developing regions to diversify revenue streams and expand their total addressable market (TAM).
About DataString Consulting
DataString Consulting is a leading provider of market research and business intelligence solutions, offering a comprehensive range of services for both B2C and B2B markets. With over 30 years of combined industry experience, DataString specializes in delivering actionable insights that support strategic decision-making.
The company’s expertise spans multiple industries, providing tailored research services in strategy consulting, opportunity assessment, competitive intelligence, and market forecasting. DataString Consulting helps businesses navigate complex markets and capitalize on emerging trends to achieve long-term success.
#Geospatial Solutions#Geospatial Technologies#Market Growth#Precision Agriculture#Smart Cities#Urban Planning#Logistics and Transportation#Defense and Security#AI and Machine Learning#Real-time Location Data#Industry Trends#Market Leadership#Competitive Landscape#Emerging Markets#Data Privacy#Market Forecast#North America Geospatial Market#Asia-Pacific Geospatial Market#Market Expansion#Strategic Investments#DataString Consulting#Market Research
0 notes
Text
Leveraging SAS Tutorials for Mastering AI and Machine Learning Applications
The fields of Artificial Intelligence (AI) and Machine Learning (ML) are rapidly transforming industries by enabling data-driven decision-making and predictive analytics. For those with a foundation in SAS programming, delving into AI and ML through specialized SAS tutorials can open new avenues for professional growth and innovation.
The Intersection of SAS and AI/ML
SAS has evolved to incorporate AI and ML functionalities, particularly through platforms like SAS Viya. This integration allows users to build, train, and deploy sophisticated models within the familiar SAS environment. Understanding how to navigate these features is crucial for leveraging the full potential of SAS in AI and ML applications.
Educational Pathways
To master AI and ML using SAS, consider engaging with the following educational resources:
Machine Learning Using SAS Viya Course: Offered on Coursera, this course provides an in-depth exploration of machine learning techniques within SAS Viya. It covers topics such as data preprocessing, decision trees, neural networks, and model assessment, offering practical experience through hands-on assignments.
SAS AI and Machine Learning Courses: SAS offers a range of courses tailored to different proficiency levels, from AI literacy for beginners to advanced machine learning for professionals. These courses are designed to build a comprehensive understanding of AI and ML concepts within the SAS framework.
Practical Application of Tutorials
Engaging with these SAS tutorial enables users to:
Develop Predictive Models: Learn to create models that can forecast outcomes based on historical data, aiding in proactive decision-making.
Implement Neural Networks: Gain insights into building neural network architectures for complex pattern recognition tasks.
Assess and Deploy Models: Understand methodologies for evaluating model performance and strategies for deploying models into production environments.
Advantages of SAS-Based AI/ML Learning
Pursuing AI and ML education through SAS offers several benefits:
Integrated Environment: SAS provides a cohesive platform where data management, analysis, and model deployment can occur seamlessly.
Industry Recognition: Skills acquired through SAS are highly regarded in industries such as finance, healthcare, and government.
Comprehensive Support: SAS offers extensive documentation and a supportive community, facilitating continuous learning and problem-solving.
youtube
Conclusion
For individuals proficient in SAS programming, expanding expertise into AI and ML through targeted SAS tutorials is a strategic move. It not only enhances analytical capabilities but also positions professionals at the forefront of technological advancements, ready to tackle complex data challenges with confidence.
#sas tutorial#sas programming tutorial#sas programming#sas tutorial for beginners#AI and Machine Learning#Youtube
0 notes
Text
How Quantum Computing Will Redefine AI and Machine Learning

Introducing AI, machine learning, and quantum computing into the mix has already begun to transform the face of technology to greater heights. With time, the effects of quantum computing will revolutionize AI and other areas of machine learning beyond our current comprehension. But what is quantum computing, and how will it change the future of artificial intelligence and machine learning?
Let us take you straight into this wonderful crossroads.
Understanding Quantum Computing and AI
Quantum computation is a revolutionary computing system that utilizes the concept of quantum mechanics to solve problems faster than the best traditional methods available to computers. While classical computing uses binary bits, 0 or 1, Quantum computing uses quantum bits or qubits that exist in more than one state. The capacity of quantum computers to simultaneously consider numerous calculations or datasets is called superposition and it is pivotal for all modern AI and machine learning algorithms.
At Guruface, our mission is to make advanced learning accessible to all, including the latest breakthroughs in technology like quantum AI. By exploring courses on quantum computing and AI, you can gain insights into how these technologies are shaping the future.
How Quantum Computing Impacts Machine Learning
One of AI’s categories is machine learning, which involves training a program to identify patterns and algorithms to make accurate predictions using data input. In contrast, current common machine learning model paradigms require intense computation for processing big data, especially with higher dimensionality. That is where quantum machine learning comes into the picture.
Key Benefits of Quantum Computing in Machine Learning
Accelerated Data Processing
With quantum computing, one can solve problems at a speed that would be impossible for any classical computer. This acceleration is significant when it comes to applications that need real-time analysis, be it an autonomous car, or a medical decision-supporting system, where AI and machine learning models can adapt to new information.
Enhanced Pattern Recognition

Quantum algorithms are built to look for a pattern in a set of data, which is critical to AI and machine learning algorithms such as image or voice recognition and recommendation.
Optimization and Problem Solving
Business optimization problems are well-solved by quantum computers and any problem that AI models would solve better with optimized solution—logistics, finance, prediction and analysis—are good candidates for quantum computing.
Deep Learning Advancements
Taking the Deep learning to the next level, quantum computing might help machine learning immensely. Quantum networks when evolving will allow the training of highly sophisticated models which require considerably lower computational power than at present.
Applications of Quantum Computing in AI and Machine Learning
Drug Discovery and Healthcare
The adoption of quantum computing is seen as having the ability to transform drug discovery through simulation of quantum structures. The quantum simulations of these compounds can be analyzed further by AI and machine learning algorithms to reveal new drug compounds faster and faster, bringing new treatments to the market.
Financial Modeling and Risk Analysis

In finance, quantum AI may help to make a significantly better prediction of risks. It enhances the brevity of performing historical computations and market trends with high efficiency in optimizing machine learning with intelligent computing in stock movements and risks.
Climate Modeling and Environmental Science
In finance, quantum AI may help to make a significantly better prediction of risks. It enhances the brevity of performing historical computations and market trends with high efficiency in optimizing machine learning with intelligent computing in stock movements and risks.
The Future of AI and Quantum Technology
AI technology depends on quantum computing as its next major frontier. With prosaic quantum-inspired algorithms, AI models will also adopt quantum enhanced algorithms for faster and improved analysis of big data opening new frontiers. At Guruface, we know the significance of updating ourselves with the latest trends in technology, such as quantum AI and machine learning. Through studying with us you will be prepared for changes in this fast-growing area by developing the knowledge and skills for it.
Why Now is the Time to Learn Machine Learning and Quantum Computing

Enterprises are rapidly finding a need for skills in artificial intelligence and machine learning due to massive enhancements in quantum computing. As for narrowing down the machine learning algorithms, developing new applications in AI and quantum technologies, the opportunity is limitless.
It is useful to learn Machine Learning and quantum computing whether you want to become a data scientist or you are already working as an AI developer. We have a selection of online courses on Guruface to help you get a better understanding of the jobs within the quantum technology and AI market guidance as well as the necessary skills to succeed in this industry of the future.
Conclusion
The combination of quantum computing and of artificial Intelligence will revolutionize industries and generate new business models. The technology in the field of quantum is advancing and that is good news for AI and machine learning because their advancement will increase their value. Get yourself in Guruface to read more on how quantum computing is going to revolutionize the advances in artificial intelligence.
0 notes
Text
SAP Business AI: Revolutionizing the Future of Business Operations
In today’s fast-paced business environment, staying competitive requires adopting the latest technologies. SAP Business AI is one such innovation that’s transforming how organizations operate. By integrating artificial intelligence (AI) into SAP’s enterprise resource planning (ERP) systems, businesses are unlocking new levels of efficiency, productivity, and insight.
In this blog, we will explore what SAP Business AI is, how it works, and why it’s an essential tool for businesses seeking to improve their operations and decision-making.
What is SAP Business AI?
SAP Business AI is an advanced suite of artificial intelligence tools built into SAP's Business Technology Platform (BTP). It combines machine learning, natural language processing, and predictive analytics with existing SAP solutions to automate tasks, analyze data, and enhance decision-making. Essentially, it’s designed to help businesses make smarter decisions by using the power of AI to process large volumes of data and deliver actionable insights in real time.
Unlike traditional AI systems, SAP Business AI integrates seamlessly with existing SAP products like SAP S/4HANA or SAP SuccessFactors, making it easier for businesses to adopt and leverage AI technology without overhauling their entire infrastructure.
How Does SAP Business AI Work?
SAP Business AI works by analyzing vast amounts of data from various business processes and applying advanced machine learning algorithms to generate insights, predictions, and recommendations. It does so through these key steps:
Data Integration: SAP Business AI integrates with your existing SAP systems, pulling in data from different sources such as customer interactions, sales reports, or inventory data.
Predictive Analytics: Using machine learning, the system identifies patterns and trends within the data, helping businesses forecast future events like customer demand or market conditions.
Automation: SAP Business AI automates routine tasks like invoicing, customer support, and inventory management, improving overall operational efficiency.
Real-Time Insights: The platform provides actionable insights in real time, helping businesses make timely decisions based on the most up-to-date data.
Benefits of SAP Business AI
There’s a reason why SAP Business AI is rapidly gaining traction among businesses worldwide. Here are some of the most compelling benefits:
1. Improved Decision-Making
AI-driven insights enable better decision-making by analyzing historical and real-time data. Whether it's predicting market trends, evaluating customer preferences, or managing resources, SAP Business AI provides recommendations that lead to smarter business choices.
2. Enhanced Efficiency
By automating repetitive tasks like data entry, invoicing, or stock management, businesses can free up employees to focus on more strategic tasks. This leads to greater efficiency and cost savings.
3. Cost Reduction
By streamlining operations and minimizing human error, businesses can lower their operational costs. SAP Business AI helps identify areas of inefficiency, enabling companies to optimize processes and reduce unnecessary expenses.
4. Personalized Customer Experience
With its ability to analyze customer data, SAP Business AI helps businesses deliver personalized experiences. This can include tailored marketing messages, personalized product recommendations, and a more engaging customer service experience.
5. Faster Response Times
Real-time data processing allows businesses to make quicker decisions. Whether it’s responding to supply chain disruptions or addressing customer concerns, businesses can act faster and more effectively.
Applications of SAP Business AI in Business Operations
The versatility of SAP Business AI makes it applicable across various industries. Here are a few examples of how businesses can use it:
1. Supply Chain Optimization
Managing a supply chain involves dealing with multiple variables such as suppliers, logistics, and production schedules. SAP Business AI uses predictive analytics to forecast potential delays, optimize inventory, and ensure that products reach customers on time.
2. Sales and Marketing
Sales teams can leverage SAP Business AI to identify high-potential leads, track customer behaviors, and optimize marketing campaigns. With data-backed insights, sales and marketing teams can better target their efforts and increase revenue.
3. Human Resources
For HR departments, SAP Business AI simplifies tasks like recruitment, employee engagement, and performance analysis. The platform can also predict employee turnover, helping HR teams take proactive measures to retain talent.
4. Financial Management
In finance, SAP Business AI helps streamline tasks such as invoicing, budget forecasting, and expense management. It also provides real-time financial insights that support better decision-making and help businesses stay on top of cash flow.
Challenges of Adopting SAP Business AI
While SAP Business AI offers several benefits, there are a few challenges businesses might face:
1. Complexity of Implementation
Integrating AI with existing systems can be complex, especially for businesses without prior experience in AI or advanced data analytics. Companies may need to invest in training or hire experts to ensure a smooth implementation process.
2. Data Quality
The effectiveness of AI systems relies heavily on the quality of the data being fed into them. If the data is outdated, unstructured, or incomplete, it may result in inaccurate insights.
3. Cost
Though SAP Business AI provides significant long-term value, the initial investment required for implementation might be high, especially for smaller businesses. However, cloud-based options are available, making the technology more accessible.
Conclusion
SAP Business AI is a powerful tool that’s transforming how businesses operate. By integrating AI capabilities into SAP’s existing software, businesses can automate tasks, enhance decision-making, and gain a competitive edge. Whether you’re looking to improve efficiency, reduce costs, or offer a personalized customer experience, SAP Business AI is a game-changer that can help you achieve your goals.
As AI continues to evolve, its role in business operations will only grow. By embracing SAP Business AI, businesses can stay ahead of the curve and position themselves for future success in an increasingly digital world.
0 notes
Text
IIT Bhilai Artificial Intelligence Course | Executive MTech in AI
Boost your career with an Executive M.Tech in Artificial Intelligence. Learn about course structure, eligibility, fees, and exciting career opportunities in AI and machine learning.
#Executive M.Tech#M.Tech in Artificial Intelligence#Executive M.Tech in Artificial Intelligence#Future Career Opportunities#AI and machine learning
0 notes
Text
Which is the most vital element of a deep-learning workstation?

One of the most vital hardware parts of a deep-learning workstation is the GPU. Training neural networks is one of the important tasks in Deep learning are highly parallelizable. It means that the massive calculations in training neural networks can be divided into many smaller tasks that can be processed simultaneously. This is where GPUs work, as they are built for parallel computing, rendering them significantly faster than CPUs for deep learning tasks.
• Parallel Processing:
Deep learning, particularly training neural networks, includes immense amounts of calculations that can be segmented into smaller tasks processed simultaneously. GPUs are crafted for this kind of parallel processing, making them perfect for deep learning.
• CUDA Support:
NVIDIA GPUs with CUDA (Compute Unified Device Architecture) are favoured for deep learning due to their robust performance and compatibility with frameworks such as TensorFlow and PyTorch.
• VRAM:
The quantity of Video RAM (VRAM) on the GPU is also vital, as it dictates how large a model and dataset can be managed.
• Other Considerations:
While the GPU is essential, other components like the CPU (for standard processing), RAM (for managing large datasets), storage (for rapid data access), and a reliable power supply are also crucial. SO GPU is the most significant component for deep learning workstations.
When selecting a GPU, high-end models like the NVIDIA RTX series (e.g., RTX 3090 or the newer RTX
090) are perfect for deep-learning applications. These GPUs are equipped with thousands of CUDA cores, which allow them to carry out matrix operations that are crucial for training deep-learning models. Furthermore, newer GPUs in this series also include Tensor Cores, specifically optimized for AI workloads, further enhancing performance.
Key Considerations:
• Power Supply: High-end GPUs are incredibly power-hungry, often needing 350W or more. Make sure your workstation has a sufficiently powerful power supply unit (PSU), ideally 850W or above, to accommodate multiple GPUs.
• Cooling System: High-performance GPUs produce a lot of heat during demanding tasks. A workstation must possess an efficient cooling solution, which we will elaborate on later in this guide.
• Scalability: If your deep-learning projects grow, having a workstation that can accommodate multiple GPUs (2) is advantageous for accelerating training times.
The GPU is the core of any deep-learning system, as its performance directly correlates with the pace at which models can be trained, making it the most crucial component of your workstation.
#Deep learning workstation#ai and machine learning#AI and Machine learning workstation#animation workstation#CAD workstation#ai workstation
0 notes
Text
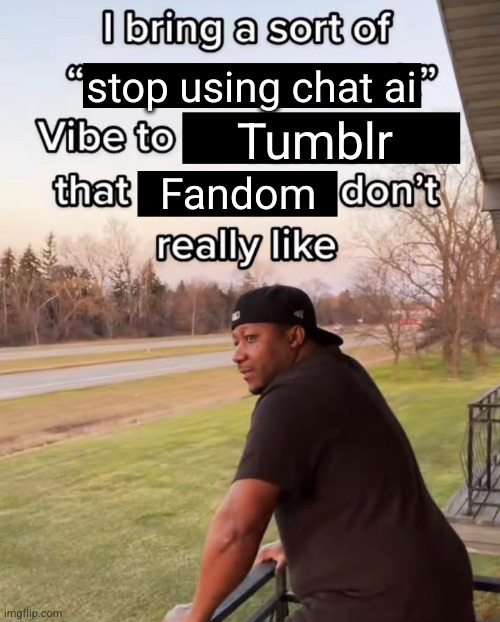
#I'm serious stop doing it#theyre scraping fanfics and other authors writing#'oh but i wanna rp with my favs' then learn to write#studios wanna use ai to put writers AND artists out of business stop feeding the fucking machine!!!!
166K notes
·
View notes
Text
Transforming Patient Care with Innovative IT Solutions for Healthcare
In an increasingly digital world, the healthcare sector is no exception when it comes to embracing technological advancements. From electronic health records (EHR) to remote patient monitoring and data-driven diagnostics, healthcare organizations rely on robust IT Solutions for Healthcare to elevate patient experiences, streamline operations, and ensure regulatory compliance. This synergy of healthcare and technology becomes even more powerful when combined with AI and Machine Learning, enabling data-driven decision-making that can profoundly transform patient care and organizational efficiency.
Mobiloitte offers comprehensive Healthcare IT Solutions that address the industry’s evolving needs. By leveraging advanced technologies and innovative strategies, healthcare facilities can improve patient outcomes, enhance operational processes, and remain competitive in an ever-shifting landscape.
1. The Evolving Role of Healthcare IT
Healthcare IT has come a long way from simple digital record-keeping to sophisticated platforms for patient engagement, workflow automation, and real-time data analytics. Today’s IT Solutions for Healthcare go far beyond maintaining patient records; they span a broad ecosystem that includes:
Telehealth and Remote Care
Virtual consultations reduce patient wait times and expand access to specialized care.
Healthcare providers can remotely monitor chronic conditions, enabling timely interventions.
Clinical Decision Support Systems
Real-time alerts and analytics inform medical staff about potential drug interactions, critical lab results, or anomalies in patient data.
This data-driven approach can significantly reduce errors and enhance patient safety.
Patient Engagement Platforms
Portals allow patients to schedule appointments, access records, and securely communicate with providers.
Enhanced engagement fosters trust and encourages proactive health management.
Connected Medical Devices
Wearables and IoT-enabled devices provide continuous insights into patient vitals.
Clinicians can intervene faster, preventing complications that might lead to expensive hospital admissions.
2. AI and Machine Learning in Healthcare
The confluence of AI and Machine Learning with healthcare IT introduces an entirely new dimension of predictive, personalized, and precision medicine.
Key Applications
Predictive Analytics: AI algorithms analyze vast sets of patient data (EHR, lab results, imaging) to forecast disease likelihood or complications, guiding preventive measures.
Medical Imaging and Diagnostics: Machine learning models assist radiologists in detecting anomalies such as tumors or lesions, often with remarkable accuracy.
Drug Discovery: Pharmaceutical researchers leverage AI-based simulations to fast-track the identification of promising compounds, reducing R&D timelines.
Natural Language Processing (NLP): This technology extracts valuable insights from unstructured clinical notes, broadening research capabilities and patient care strategies.
Benefits
Improved Diagnosis: Intelligent tools reduce diagnostic errors, facilitating earlier treatments and better outcomes.
Cost Savings: Targeted diagnoses and streamlined workflows often lead to diminished overheads.
Personalized Medicine: Data-driven insights allow clinicians to tailor therapies based on patient genetics, lifestyle, and medical history.
3. Ensuring Data Security and Compliance
Healthcare data is exceptionally sensitive, governed by stringent regulations like HIPAA (in the U.S.) or GDPR (in Europe). Healthcare IT Solutions must incorporate advanced security protocols—encryption, secure access controls, and rigorous audit trails—to keep patient records protected.
Network Segmentation
Dividing networks into isolated segments mitigates risks and confines breaches.
Encryption Standards
End-to-end encryption ensures that data transmitted between healthcare providers, patients, and cloud storage remains secure.
Continuous Monitoring
Automated intrusion detection systems (IDS) and intrusion prevention systems (IPS) can proactively identify suspicious activity.
By prioritizing robust cybersecurity measures, healthcare providers not only protect patient trust but also avoid financial and legal ramifications stemming from data breaches.
4. Interoperability and System Integration
A major challenge in healthcare is the effective exchange of information across multiple platforms (EHRs, billing, scheduling). IT Solutions for Healthcare that focus on seamless interoperability can achieve the following:
Improved Care Coordination
Physicians and specialists gain immediate access to complete patient histories, enabling collaborative treatment planning.
Reduced Redundancies
Eliminating duplicative tests or procedures minimizes patient inconvenience and reduces overall costs.
Enhanced Analytics
Unified data sets allow for more advanced analytics, revealing trends that can lead to evidence-based policy or operational changes.
Healthcare-focused IT providers often implement standardized data formats like HL7 or FHIR, ensuring faster and more secure data exchange among diverse systems.
5. Telehealth and Remote Patient Monitoring
Telehealth has rapidly risen to prominence, offering viable ways to overcome geographical or mobility barriers in healthcare:
Video Consultations
High-quality video conferencing allows real-time interaction between clinicians and patients from anywhere.
This flexibility reduces in-office wait times and frees resources for more critical cases.
Wearable Sensors
Devices monitor blood pressure, blood glucose, or cardiac metrics, automatically alerting medical teams of anomalies.
This leads to proactive care for chronic disease management, reducing costly hospital readmissions.
Patient Adherence
Tracking medication intake through digital solutions fosters adherence, significantly impacting patient outcomes.
By integrating telehealth into existing IT Solutions for Healthcare, providers can create a more versatile and resilient care model, especially in times of public health crises or natural disasters.
6. Custom Healthcare Software Development
Off-the-shelf solutions often fall short of the intricate demands of modern healthcare environments. Custom healthcare software development addresses unique organizational needs:
Tailored Workflows
Custom solutions align with each department’s specific processes, maximizing ease of use and staff productivity.
Scalable Architectures
As patient volumes or service portfolios expand, well-engineered software scales gracefully without performance bottlenecks.
Integration of AI and Machine Learning
Purpose-built modules can incorporate advanced algorithms for more nuanced analytics or automated decision support.
By partnering with experts like Mobiloitte, healthcare institutions can leverage custom solutions to effectively integrate emerging technologies like AI and Machine Learning while satisfying compliance mandates.
7. Driving Operational Efficiency with Analytics
Data analytics forms the backbone of strategic decision-making in healthcare:
Resource Allocation
Insights into peak visitation times and patient flows guide staffing, inventory management, and facility expansions.
Predictive Maintenance
Monitoring the performance of critical equipment can predict failures, reduce downtime and prevent disruption in patient care.
Financial Management
Analytical tools track billing cycles, claims processing, and insurance reimbursements, identifying areas to minimize costs.
By harnessing the power of analytics within Healthcare IT Solutions, organizations can evolve from reactive to proactive operations, ultimately improving patient satisfaction and organizational resilience.
8. The Future of Healthcare IT Solutions
Looking ahead, the healthcare landscape will continue to harness advanced technologies to improve patient outcomes and overall efficiency:
AI-Assisted Surgery
Robotic systems, powered by AI and Machine Learning, can enhance precision in surgical interventions.
Blockchain for Secure Data Sharing
Distributed ledger technology offers tamper-proof records, ensuring data integrity across different care providers.
Augmented Reality (AR) and Virtual Reality (VR)
Training programs for medical students and complex procedure simulations benefit from immersive VR environments.
Personalized Genomic Medicine
Genetic data integrated with AI-based analytics leads to personalized therapies tailored to individual patient profiles.
Each innovation builds upon core digital frameworks—robust data management, secure communication, and real-time analytics. Organizations that embrace these developments will remain at the cutting edge of healthcare.
Conclusion
The integration of Healthcare IT Solutions represents a pivotal shift in the healthcare ecosystem, focusing on more accurate diagnoses, efficient operations, and patient-centric care. With the inclusion of AI and Machine Learning, providers can unlock predictive insights, personalized treatment plans, and advanced decision support—all while maintaining top-notch security and regulatory compliance.
By collaborating with technology partners like Mobiloitte, healthcare institutions can implement holistic IT frameworks that scale and adapt to evolving medical landscapes. From telehealth services to AI-assisted analytics, the potential applications of robust healthcare IT are limitless—leading ultimately to better patient outcomes, reduced overheads, and more sustainable medical practices.
FAQs: People Also Ask
Q1: What are the key components of Healthcare IT Solutions?
A1: Key elements include EHR systems, telemedicine, healthcare analytics, AI-driven decision support, and secure data management. Interoperability ensures seamless data exchange between systems.
Q2: How can AI and Machine Learning benefit patient care?
A2: AI and Machine Learning enhance diagnostic accuracy, predict patient risks, streamline triage, and reduce manual processes. Machine learning models can analyze complex data sets (e.g., medical imaging, genomic data) to provide timely clinical insights.
Q3: Are healthcare providers required to adopt AI technologies?
A3: No, there’s no universal mandate to adopt AI. However, integrating AI-based solutions can offer a competitive advantage by improving accuracy, patient outcomes, and cost-efficiency.
Q4: How do IT Solutions for Healthcare handle sensitive data securely?
A4: Security measures typically include encryption (in transit and at rest), secure login protocols, role-based access controls, and regular security audits. Providers must also adhere to regulations like HIPAA in the U.S. or GDPR in the EU.
Q5: What is the difference between off-the-shelf and custom healthcare software?
A5: Off-the-shelf solutions are quick to deploy but often lack specialized features. Custom healthcare software is tailored to an organization’s unique workflows, data requirements, and integration needs—usually delivering better long-term value and flexibility.
Visit To Know More:- http://mobiloitte.com/contact-us
#Healthcare IT Solutions#AI and Machine Learning#IT Solutions for Healthcare#healthcare software development company#healthcare it service#healthcare solution
0 notes
Text
Boost Your Business with Cutting-Edge Artificial Intelligence Services
Unlock the full potential of artificial intelligence services to transform your business operations, streamline workflows, and enhance decision-making. Our advanced AI and machine learning services empower businesses with data-driven insights, intelligent automation, and predictive analytics. Whether you need AI-powered chatbots, recommendation systems, or process automation, we offer tailored solutions to meet your unique requirements. Stay ahead of the competition with cutting-edge AI technologies that drive innovation, improve efficiency, and boost productivity. Embrace the future of AI and unlock new growth opportunities for your business with our expert solutions. Let AI revolutionize the way you operate and achieve success.
#AI and machine learning#artificial intelligence services#ai and machine learning services#artificial intelligence and machine learning
0 notes
Text
The Power of Personalization in Email Marketing
In today's fast-paced digital world, email marketing remains one of the most effective tools for businesses to engage with their audience. However, with inboxes flooded daily, standing out requires more than just a generic message. This is where personalization in emailmarketing comes into play. By tailoring content to individual preferences and behaviors, brands can significantly boost open rates, click-through rates, and conversions.
What is Personalization in Email Marketing?
Personalization in email marketing is the practice of using customer data to create targeted and relevant email campaigns. It goes beyond just addressing the recipient by their first name; it involves leveraging behavioral insights, past purchases, browsing history, and preferences to craft messages that resonate.
Why Personalization Matters in Email Marketing
1. Increased Engagement
Studies show that personalized emails have higher open rates and engagement levels. When customers receive content that aligns with their interests, they are more likely to interact with the email, leading to better conversion rates.
2. Improved Customer Experience
Modern consumers expect brands to understand their needs. Personalized email marketing fosters a sense of connection, making customers feel valued and appreciated. This enhances brand loyalty and trust.
3. Higher ROI
According to research, businesses that implement email marketing personalization experience a substantial return on investment (ROI). By sending relevant content, brands reduce unsubscribes and improve email deliverability.
Effective Strategies for Email Personalization
1. Segmenting Your Audience
Segmentation is the foundation of email marketing personalization. By categorizing subscribers based on demographics, behavior, and preferences, businesses can send highly relevant content to each segment.
2. Dynamic Content
Using dynamic content allows marketers to customize email elements such as images, text, and offers based on user preferences. This technique enhances the relevance of the email, improving engagement and conversions.
3. Behavioral Triggers
Automating emails based on user behavior ensures timely and relevant interactions. Examples include:
● Welcome emails for new subscribers
● Cart abandonment emails
● Re-engagement emails for inactive users
● Post-purchase follow-ups
4. Personalized Subject Lines
An engaging subject line can make or break an email campaign. Studies indicate that personalized subject lines increase open rates significantly. Incorporating the recipient's name or referencing their recent activity can create a compelling first impression.
5. AI and Machine Learning
Artificial intelligence (AI) and machine learning help analyze customer data and predict preferences. These insights enable businesses to craft hyper-personalized email campaigns that drive better results.
The Role of MagixBowl in Personalized Email Marketing
At MagixBowl, we specialize in innovative email marketing strategies that incorporate advanced personalization techniques. Our team helps businesses leverage customer data to create impactful, results-driven campaigns that maximize engagement and ROI.
Case Study: How MagixBowl Transformed a Brand’s Email Strategy
A leading e-commerce brand partnered with MagixBowl to revamp its email marketing strategy. By implementing AI-driven personalization and dynamic content, the brand saw a 40% increase in open rates, a 25% boost in conversions, and a 30% reduction in unsubscribes within three months.
Measuring the Success of Personalized Email Campaigns
To ensure effectiveness, businesses must track key email marketing metrics such as:
● Open Rates – Percentage of recipients who open the email
● Click-Through Rates (CTR) – Number of recipients clicking on links
● Conversion Rates – Percentage of users taking the desired action
● Bounce Rates – Emails that fail to reach the recipient
● Unsubscribe Rates – Users opting out of future emails
Analyzing these metrics allows brands to refine their email marketing personalization strategy for optimal results.
Future Trends in Email Personalization
As technology evolves, the future of personalized email marketing will be driven by:
● AI-Powered Hyper-Personalization
● Interactive Emails with Dynamic Elements
● Predictive Analytics for Behavior-Based Campaigns
● Integration with Omnichannel Marketing Strategies
Conclusion
Email marketing personalization is no longer optional—it’s necessary for brands looking to stand out in crowded inboxes. Businesses can create impactful, highly engaging campaigns by leveraging customer insights, segmentation, dynamic content, and AI. Partnering with experts like MagixBowl ensures that your email marketing strategy is optimized for maximum success.
Are you ready to unlock the full potential of personalized email marketing? Contact MagixBowl today and elevate your brand’s engagement and conversion rates!
contact us : +91 7996316333
visit us: https://magixbowl.com/.
#Email marketing#Personalization in email marketing#Personalized emails#Email marketing personalization#Conversion rates#Brand loyalty#Email deliverability#Dynamic content#Personalized subject lines#Behavioral triggers#AI and machine learning#Email marketing strategies#Click-through rates (CTR)#Open rates#Bounce rates#Unsubscribe rates#Engagement levels#Customer experience
0 notes
Text
AI & ML Services by Aiinfox – Smarter Solutions for a Smarter Future!
AI is changing the game! From automation to predictive analytics, Aiinfox offers AI and Machine learning services that power innovation and efficiency. Let’s build the future together! ⚡
0 notes
Text
How Quantum Computing Will Redefine AI and Machine Learning

Introducing AI, machine learning, and quantum computing into the mix has already begun to transform the face of technology to greater heights. With time, the effects of quantum computing will revolutionize AI and other areas of machine learning beyond our current comprehension. But what is quantum computing, and how will it change the future of artificial intelligence and machine learning?
Let us take you straight into this wonderful crossroads.
Understanding Quantum Computing and AI
Quantum computation is a revolutionary computing system that utilizes the concept of quantum mechanics to solve problems faster than the best traditional methods available to computers. While classical computing uses binary bits, 0 or 1, Quantum computing uses quantum bits or qubits that exist in more than one state. The capacity of quantum computers to simultaneously consider numerous calculations or datasets is called superposition and it is pivotal for all modern AI and machine learning algorithms.
At Guruface, our mission is to make advanced learning accessible to all, including the latest breakthroughs in technology like quantum AI. By exploring courses on quantum computing and AI, you can gain insights into how these technologies are shaping the future.
How Quantum Computing Impacts Machine Learning
One of AI’s categories is machine learning, which involves training a program to identify patterns and algorithms to make accurate predictions using data input. In contrast, current common machine learning model paradigms require intense computation for processing big data, especially with higher dimensionality. That is where quantum machine learning comes into the picture.
Key Benefits of Quantum Computing in Machine Learning
Accelerated Data Processing
With quantum computing, one can solve problems at a speed that would be impossible for any classical computer. This acceleration is significant when it comes to applications that need real-time analysis, be it an autonomous car, or a medical decision-supporting system, where AI and machine learning models can adapt to new information.
Enhanced Pattern Recognition

Quantum algorithms are built to look for a pattern in a set of data, which is critical to AI and machine learning algorithms such as image or voice recognition and recommendation.
Optimization and Problem Solving
Business optimization problems are well-solved by quantum computers and any problem that AI models would solve better with optimized solution—logistics, finance, prediction and analysis—are good candidates for quantum computing.
Deep Learning Advancements
Taking the Deep learning to the next level, quantum computing might help machine learning immensely. Quantum networks when evolving will allow the training of highly sophisticated models which require considerably lower computational power than at present.
Applications of Quantum Computing in AI and Machine Learning
Drug Discovery and Healthcare
The adoption of quantum computing is seen as having the ability to transform drug discovery through simulation of quantum structures. The quantum simulations of these compounds can be analyzed further by AI and machine learning algorithms to reveal new drug compounds faster and faster, bringing new treatments to the market.
Financial Modeling and Risk Analysis

In finance, quantum AI may help to make a significantly better prediction of risks. It enhances the brevity of performing historical computations and market trends with high efficiency in optimizing machine learning with intelligent computing in stock movements and risks.
Climate Modeling and Environmental Science
In finance, quantum AI may help to make a significantly better prediction of risks. It enhances the brevity of performing historical computations and market trends with high efficiency in optimizing machine learning with intelligent computing in stock movements and risks.
The Future of AI and Quantum Technology
AI technology depends on quantum computing as its next major frontier. With prosaic quantum-inspired algorithms, AI models will also adopt quantum enhanced algorithms for faster and improved analysis of big data opening new frontiers. At Guruface, we know the significance of updating ourselves with the latest trends in technology, such as quantum AI and machine learning. Through studying with us you will be prepared for changes in this fast-growing area by developing the knowledge and skills for it.
Why Now is the Time to Learn Machine Learning and Quantum Computing

Enterprises are rapidly finding a need for skills in artificial intelligence and machine learning due to massive enhancements in quantum computing. As for narrowing down the machine learning algorithms, developing new applications in AI and quantum technologies, the opportunity is limitless.
It is useful to learn Machine Learning and quantum computing whether you want to become a data scientist or you are already working as an AI developer. We have a selection of online courses on Guruface to help you get a better understanding of the jobs within the quantum technology and AI market guidance as well as the necessary skills to succeed in this industry of the future.
Conclusion
The combination of quantum computing and of artificial Intelligence will revolutionize industries and generate new business models. The technology in the field of quantum is advancing and that is good news for AI and machine learning because their advancement will increase their value. Get yourself in Guruface to read more on how quantum computing is going to revolutionize the advances in artificial intelligence.
0 notes
Text
Digital Supply Chain: The Future of Logistics and Operations
The digital supply chain is not just a trend; it's the future of business logistics and operations. As companies continue to embrace the advancements in technology, the need for a more connected, data-driven approach to supply chain management has never been more apparent. In this article, we will dive deep into what a digital supply chain is, its components, and how businesses can leverage it to achieve significant improvements in efficiency, cost reduction, and customer satisfaction.
What is a Digital Supply Chain?
In simple terms, a digital supply chain refers to the integration of advanced technologies such as the Internet of Things (IoT), big data analytics, cloud computing, and artificial intelligence (AI) into traditional supply chain processes. These technologies work together to provide real-time insights, automate tasks, and create a more agile and responsive supply chain system.
The main objective of a digital supply chain is to optimize the flow of goods and information from suppliers to customers, reducing inefficiencies and improving decision-making processes.
Key Components of a Digital Supply Chain
A digital supply chain is made up of several critical components that work in harmony to ensure seamless operations:
1. Internet of Things (IoT)
The IoT refers to the network of connected devices that can communicate with each other and share data in real time. In the context of the supply chain, IoT devices like sensors and RFID tags can track the movement of goods, monitor inventory levels, and provide data on environmental conditions (such as temperature or humidity). This helps companies maintain control over their operations and mitigate risks such as theft or spoilage.
2. Big Data and Analytics
Big data plays a central role in the digital supply chain by enabling businesses to collect and analyze massive amounts of data. By using advanced analytics, companies can gain valuable insights into their operations, identify patterns, and make data-driven decisions. For instance, data from customer orders can be analyzed to predict demand, allowing companies to optimize their inventory and reduce excess stock.
3. Artificial Intelligence (AI) and Machine Learning
AI and machine learning are powerful tools that can automate various tasks within the supply chain. From predictive analytics that forecast demand to intelligent routing algorithms that optimize delivery routes, AI enables companies to make smarter, faster decisions. AI also plays a role in supply chain risk management, detecting potential disruptions before they become major problems.
4. Cloud Computing
Cloud computing allows for the centralized storage and sharing of data across the entire supply chain network. This ensures that all stakeholders, including suppliers, manufacturers, and distributors, have access to the same information in real time. Cloud-based platforms also enable easier collaboration and streamline communication between teams.
5. Blockchain Technology
Blockchain offers a secure, transparent way to track transactions across the supply chain. By creating an immutable record of each step in the supply chain process, blockchain reduces the risk of fraud, improves traceability, and increases trust among supply chain partners.
Benefits of a Digital Supply Chain
Adopting a digital supply chain strategy can bring a host of benefits to businesses, including:
1. Enhanced Efficiency
With real-time data and automation, companies can optimize their operations and reduce manual processes. Tasks such as inventory management, order fulfillment, and procurement can be streamlined, resulting in faster and more efficient workflows.
2. Cost Reduction
The digital supply chain helps companies reduce costs in several ways. By optimizing inventory management, businesses can avoid overstocking or stockouts, both of which can be costly. Automated processes also reduce the need for manual labor, and data-driven decision-making can help companies identify inefficiencies and eliminate waste.
3. Improved Customer Experience
A digital supply chain allows companies to deliver products faster and with greater accuracy. Real-time tracking and visibility into inventory levels enable businesses to provide customers with up-to-date information on their orders, leading to improved customer satisfaction and loyalty.
4. Increased Agility
The digital supply chain provides companies with the flexibility to quickly adapt to changing market conditions. By leveraging technologies like AI and big data, businesses can anticipate demand shifts, respond to disruptions, and make adjustments to their supply chain operations in real time.
5. Better Risk Management
With digital tools like predictive analytics and IoT sensors, businesses can identify and mitigate risks before they escalate. For example, predictive analytics can forecast potential supply chain disruptions caused by factors like weather, geopolitical events, or equipment failure. Early detection of these risks allows companies to take proactive measures to minimize their impact.
The Role of Data in a Digital Supply Chain
Data is at the heart of the digital supply chain. From demand forecasting to inventory management, supply chain analytics rely on large volumes of data to optimize operations and improve decision-making.
Real-Time Data and Visibility
One of the key advantages of a digital supply chain is the ability to access real-time data. This visibility allows businesses to track the movement of goods, monitor inventory levels, and receive updates on any delays or disruptions. With this information, companies can quickly make adjustments and communicate with stakeholders, ensuring a smoother and more efficient process.
Predictive Analytics
By analyzing historical data and using AI algorithms, businesses can predict future demand, identify trends, and prepare for potential disruptions. Predictive analytics can also help companies optimize their inventory, ensuring they have the right products in stock at the right time.
Data-Driven Decision Making
With the abundance of data available in a digital supply chain, companies can make decisions based on facts and insights rather than assumptions or guesswork. This leads to better outcomes in areas like procurement, logistics, and inventory management.
Challenges of Implementing a Digital Supply Chain
While the benefits of a digital supply chain are clear, implementing this type of system comes with its own set of challenges:
1. Integration with Legacy Systems
Many businesses are still using legacy systems that may not be compatible with newer technologies. Integrating these older systems with digital tools can be complex and costly, requiring a significant investment in both time and resources.
2. Data Security and Privacy
As supply chains become more digital, the risk of cyber threats and data breaches increases. Ensuring the security and privacy of sensitive data is a top priority for businesses adopting digital supply chain technologies. Companies must implement robust cybersecurity measures to protect their data from malicious attacks.
3. Skill Gaps and Training
The implementation of a digital supply chain requires skilled professionals who understand the technologies involved. There may be a gap in the existing workforce's knowledge and expertise, which can hinder the successful adoption of digital supply chain systems. Training and upskilling employees is essential for ensuring a smooth transition.
4. High Initial Costs
The initial investment in digital supply chain technologies can be significant. Businesses must budget for the costs of new software, hardware, and training. However, the long-term savings and efficiency gains often outweigh the upfront costs.
Future Trends in the Digital Supply Chain
The digital supply chain is continuously evolving, with new technologies and trends shaping its future. Some of the key trends to watch out for include:
1. 5G Connectivity
The rollout of 5G networks will revolutionize the digital supply chain by enabling faster and more reliable data transmission. This will improve the speed and accuracy of real-time tracking, enhance automation, and enable more connected devices across the supply chain.
2. Autonomous Vehicles and Drones
The use of autonomous vehicles and drones for transportation and delivery is becoming more widespread. These technologies have the potential to reduce delivery times, lower costs, and increase efficiency in the supply chain.
3. Sustainability and Green Supply Chains
As sustainability becomes a top priority for businesses and consumers alike, digital supply chains are being used to improve environmental performance. Technologies like blockchain can track the environmental impact of products throughout their lifecycle, while AI and data analytics can optimize routes and reduce emissions.
Conclusion
The digital supply chain is transforming the way businesses manage their logistics and operations. By leveraging technologies like IoT, AI, and big data analytics, companies can improve efficiency, reduce costs, and enhance customer satisfaction. However, the adoption of digital supply chain solutions comes with its own set of challenges, including integration with legacy systems and data security concerns. As technology continues to evolve, businesses that embrace the digital supply chain will be well-positioned to stay ahead of the competition and thrive in the future of logistics and operations.
Embrace the digital supply chain today, and unlock the potential for growth, efficiency, and innovation in your business.
#Supply Chain Management#digital supply chain#Artificial Intelligence#AI and machine learning#machine learning
0 notes