#BigQuerypipesyntax
Explore tagged Tumblr posts
Text
Boost AI Production With Data Agents And BigQuery Platform
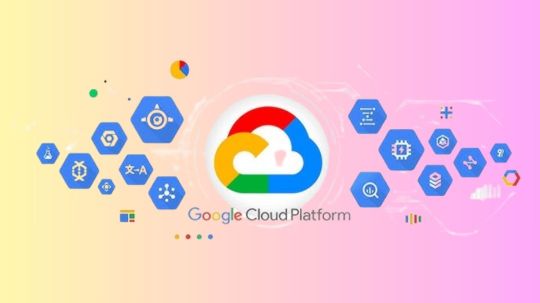
Data accessibility can hinder AI adoption since so much data is unstructured and unmanaged. Data should be accessible, actionable, and revolutionary for businesses. A data cloud based on open standards, that connects data to AI in real-time, and conversational data agents that stretch the limits of conventional AI are available today to help you do this.
An open real-time data ecosystem
Google Cloud announced intentions to combine BigQuery into a single data and AI use case platform earlier this year, including all data formats, numerous engines, governance, ML, and business intelligence. It also announces a managed Apache Iceberg experience for open-format customers. It adds document, audio, image, and video data processing to simplify multimodal data preparation.
Volkswagen bases AI models on car owner’s manuals, customer FAQs, help center articles, and official Volkswagen YouTube videos using BigQuery.
New managed services for Flink and Kafka enable customers to ingest, set up, tune, scale, monitor, and upgrade real-time applications. Data engineers can construct and execute data pipelines manually, via API, or on a schedule using BigQuery workflow previews.
Customers may now activate insights in real time using BigQuery continuous queries, another major addition. In the past, “real-time” meant examining minutes or hours old data. However, data ingestion and analysis are changing rapidly. Data, consumer engagement, decision-making, and AI-driven automation have substantially lowered the acceptable latency for decision-making. The demand for insights to activation must be smooth and take seconds, not minutes or hours. It has added real-time data sharing to the Analytics Hub data marketplace in preview.
Google Cloud launches BigQuery pipe syntax to enable customers manage, analyze, and gain value from log data. Data teams can simplify data conversions with SQL intended for semi-structured log data.
Connect all data to AI
BigQuery clients may produce and search embeddings at scale for semantic nearest-neighbor search, entity resolution, semantic search, similarity detection, RAG, and recommendations. Vertex AI integration makes integrating text, photos, video, multimodal data, and structured data easy. BigQuery integration with LangChain simplifies data pre-processing, embedding creation and storage, and vector search, now generally available.
It previews ScaNN searches for large queries to improve vector search. Google Search and YouTube use this technology. The ScaNN index supports over one billion vectors and provides top-notch query performance, enabling high-scale workloads for every enterprise.
It is also simplifying Python API data processing with BigQuery DataFrames. Synthetic data can replace ML model training and system testing. It teams with Gretel AI to generate synthetic data in BigQuery to expedite AI experiments. This data will closely resemble your actual data but won’t contain critical information.
Finer governance and data integration
Tens of thousands of companies fuel their data clouds with BigQuery and AI. However, in the data-driven AI era, enterprises must manage more data kinds and more tasks.
BigQuery’s serverless design helps Box process hundreds of thousands of events per second and manage petabyte-scale storage for billions of files and millions of users. Finer access control in BigQuery helps them locate, classify, and secure sensitive data fields.
Data management and governance become important with greater data-access and AI use cases. It unveils BigQuery’s unified catalog, which automatically harvests, ingests, and indexes information from data sources, AI models, and BI assets to help you discover your data and AI assets. BigQuery catalog semantic search in preview lets you find and query all those data assets, regardless of kind or location. Users may now ask natural language questions and BigQuery understands their purpose to retrieve the most relevant results and make it easier to locate what they need.
It enables more third-party data sources for your use cases and workflows. Equifax recently expanded its cooperation with Google Cloud to securely offer anonymized, differentiated loan, credit, and commercial marketing data using BigQuery.
Equifax believes more data leads to smarter decisions. By providing distinctive data on Google Cloud, it enables its clients to make predictive and informed decisions faster and more agilely by meeting them on their preferred channel.
Its new BigQuery metastore makes data available to many execution engines. Multiple engines can execute on a single copy of data across structured and unstructured object tables next month in preview, offering a unified view for policy, performance, and workload orchestration.
Looker lets you use BigQuery’s new governance capabilities for BI. You can leverage catalog metadata from Looker instances to collect Looker dashboards, exploration, and dimensions without setting up, maintaining, or operating your own connector.
Finally, BigQuery has catastrophe recovery for business continuity. This provides failover and redundant compute resources with a SLA for business-critical workloads. Besides your data, it enables BigQuery analytics workload failover.
Gemini conversational data agents
Global organizations demand LLM-powered data agents to conduct internal and customer-facing tasks, drive data access, deliver unique insights, and motivate action. It is developing new conversational APIs to enable developers to create data agents for self-service data access and monetize their data to differentiate their offerings.
Conversational analytics
It used these APIs to create Looker’s Gemini conversational analytics experience. Combine with Looker’s enterprise-scale semantic layer business logic models. You can root AI with a single source of truth and uniform metrics across the enterprise. You may then use natural language to explore your data like Google Search.
LookML semantic data models let you build regulated metrics and semantic relationships between data models for your data agents. LookML models don’t only describe your data; you can query them to obtain it.
Data agents run on a dynamic data knowledge graph. BigQuery powers the dynamic knowledge graph, which connects data, actions, and relationships using usage patterns, metadata, historical trends, and more.
Last but not least, Gemini in BigQuery is now broadly accessible, assisting data teams with data migration, preparation, code assist, and insights. Your business and analyst teams can now talk with your data and get insights in seconds, fostering a data-driven culture. Ready-to-run queries and AI-assisted data preparation in BigQuery Studio allow natural language pipeline building and decrease guesswork.
Connect all your data to AI by migrating it to BigQuery with the data migration application. This product roadmap webcast covers BigQuery platform updates.
Read more on Govindhtech.com
#DataAgents#BigQuery#BigQuerypipesyntax#vectorsearch#BigQueryDataFrames#BigQueryanalytics#LookMLmodels#news#technews#technology#technologynews#technologytrends#govindhtech
0 notes
Text
An Introduction Of Pipe Syntax In BigQuery And Cloud Logging

Organizations looking to improve user experiences, boost security, optimize performance, and comprehend application behavior now find that log data is a priceless resource. However, the sheer amount and intricacy of logs produced by contemporary applications can be debilitating.
Google Cloud is to give you the most effective and user-friendly solutions possible so you can fully utilize your log data. Google Cloud is excited to share with us a number of BigQuery and Cloud Logging advancements that will completely transform how you handle, examine, and use your log data.
Pipe syntax
An improvement to GoogleSQL called pipe syntax allows for a linear query structure that makes writing, reading, and maintaining your queries simpler.
Pipe syntax is supported everywhere in GoogleSQL writing. The operations supported by pipe syntax are the same as those supported by conventional GoogleSQL syntax, or standard syntax, such as joining, filtering, aggregating and grouping, and selection. However, the operations can be applied in any sequence and many times. Because of the linear form of pipe syntax, you may write queries so that the logical steps taken to construct the result table are reflected in the order in which the query syntax is written.
Pipe syntax queries are priced, run, and optimized in the same manner as their standard syntax equivalents. To minimize expenses and maximize query computation, adhere to the recommendations when composing queries using pipe syntax.
There are problems with standard syntax that can make it challenging to comprehend, write, and maintain. The way pipe syntax resolves these problems is illustrated in the following table:
SQL for log data reimagined with BigQuery pipe syntax
The days of understanding intricate, layered SQL queries are over. A new era of SQL is introduced by BigQuery pipe syntax, which was created with the semi-structured nature of log data in mind. The top-down, intuitive syntax of BigQuery’s pipe syntax is modeled around the way you typically handle data manipulations. According to Google’s latest research, this method significantly improves the readability and writability of queries. The pipe sign (|>) makes it very simple to visually distinguish between distinct phases of a query, which makes understanding the logical flow of data transformation much easier. Because each phase is distinct, self-contained, and unambiguous, your questions become easier to understand for both you and your team.
The pipe syntax in BigQuery allows you to work with your data in a more efficient and natural way, rather than merely writing cleaner SQL. Experience quicker insights, better teamwork, and more time spent extracting value rather than wrangling with code.
This simplified method is very effective in the field of log analysis.
The key to log analysis is investigation. Rarely is log analysis a simple question-answer process. Finding certain events or patterns in mountains of data is a common task when analyzing logs. Along the way, you delve deeper, learn new things, and hone your strategy. This iterative process is embraced by pipe syntax. To extract those golden insights, you can easily chain together filters (WHERE), aggregations (COUNT), and sorting (ORDER BY). Additionally, you can simply modify your analysis on the fly by adding or removing phases as you gain new insights from your data processing.
Let’s say you wish to determine how many users in January were impacted by the same faults more than 100 times in total. The data flows through each transformation as demonstrated by the pipe syntax’s linear structure, which starts with the table, filters by dates, counts by user ID and error type, filters for errors more than 100, and then counts the number of users impacted by the same faults.
— Pipe Syntax FROM log_table |> WHERE datetime BETWEEN DATETIME ‘2024-01-01’ AND ‘2024-01-31’ |> AGGREGATE COUNT(log_id) AS error_count GROUP BY user_id, error_type |> WHERE error_count>100 |> AGGREGATE COUNT(user_id) AS user_count GROUP BY
A subquery and non-linear structure are usually needed for the same example in standard syntax.
Currently, BigQuery pipe syntax is accessible in private preview. Please use this form to sign up for a private preview and watch this introductory video.
Beyond syntax: adaptability and performance
BigQuery can now handle JSON with more power and better performance, which will speed up your log analytics operations even more. Since most logs contain json data, it anticipate that most customers will find log analytics easier to understand as a result of these modifications.
Enhanced Point Lookups: Significantly speed up queries that filter on timestamps and unique IDs by use BigQuery’s numeric search indexes to swiftly identify important events in large datasets.
Robust JSON Analysis: With BigQuery’s JSON_KEYS function and JSONPath traversal capability, you can easily parse and analyze your JSON-formatted log data. Without breaking a sweat, extract particular fields, filter on nested data, and navigate intricate JSON structures.
JSON_KEYS facilitates schema exploration and discoverability by removing distinct JSON keys from JSON data.Query Results JSON_KEYS(JSON '{"a":{"b":1}}')["a", "a.b"]JSON_KEYS(JSON '{"a":[{"b":1}, {"c":2}]}', mode => "lax")["a", "a.b", "a.c"]JSON_KEYS(JSON '[[{"a":1},{"b":2}]]', mode => "lax recursive")["a", "b"]
You don’t need to use verbose UNNEST to download JSON arrays when using JSONPath with LAX modes. How to retrieve every phone number from the person field, both before and after, is demonstrated in the example below:
Log Analytics for Cloud Logging: Completing the Picture
Built on top of BigQuery, Log Analytics in Cloud Logging offers a user interface specifically designed for log analysis. By utilizing the JSON capabilities for charting, dashboarding, and an integrated date/time picker, Log Analytics is able to enable complex queries and expedite log analysis. It is also adding pipe syntax to Log Analytics (in Cloud Logging) to make it easier to include these potent features into your log management process. With the full potential of BigQuery pipe syntax, improved lookups, and JSON handling, you can now analyze your logs in Log Analytics on a single, unified platform.
The preview version of Log Analytics (Cloud Logging) now allows the use of pipe syntax.
Unlock log analytics’ future now
The combination of BigQuery and Cloud Logging offers an unparalleled method for organizing, examining, and deriving useful conclusions from your log data. Discover the power of these new skills by exploring them now.
Using pipe syntax for intuitive querying: an introductory video and documentation
Cloud logging’s Log Analytics provides unified log management and analysis.
Lightning-quick lookups using numeric search indexes – Support
JSON_KEYS and JSON_PATH allow for seamless JSON analysis
Read more on Govindhtech.com
#pipesyntax#BigQuery @GoogleSQL#BigQuery#BigQuerypipesyntax#news#technews#technology#technologynews#technologytrends#govindhtech
0 notes