#Data Extraction Service
Explore tagged Tumblr posts
Text
Explore why Intelligent Data Extraction matters. Enhance efficiency, decision-making, and insights. Stay ahead with advanced data extraction techniques.
#Intelligent Data Extraction#Data Extraction#data extraction service#data extraction solutions#web data extraction
0 notes
Text
0 notes
Text
Data Mining Services for Accurate Digital Marketing Strategies

Data mining is an essential approach for today’s digital marketing experts, to get hands on actionable insights and curate effective marketing strategies and make informed decisions. Here’s a detailed version of how data mining brings accuracy in digital marketing.
#data mining services#data mining services india#outsource data mining services#data mining service providers#data extraction services#data entry services#web data extraction services#outsource data extraction services
3 notes
·
View notes
Text
Market Research with Web Data Solutions – Dignexus
6 notes
·
View notes
Text

Lensnure Solution provides top-notch Food delivery and Restaurant data scraping services to avail benefits of extracted food data from various Restaurant listings and Food delivery platforms such as Zomato, Uber Eats, Deliveroo, Postmates, Swiggy, delivery.com, Grubhub, Seamless, DoorDash, and much more. We help you extract valuable and large amounts of food data from your target websites using our cutting-edge data scraping techniques.
Our Food delivery data scraping services deliver real-time and dynamic data including Menu items, restaurant names, Pricing, Delivery times, Contact information, Discounts, Offers, and Locations in required file formats like CSV, JSON, XLSX, etc.
Read More: Food Delivery Data Scraping
#data extraction#lensnure solutions#web scraping#web scraping services#food data scraping#food delivery data scraping#extract food ordering data#Extract Restaurant Listings Data
2 notes
·
View notes
Text
Best data extraction services in USA
In today's fiercely competitive business landscape, the strategic selection of a web data extraction services provider becomes crucial. Outsource Bigdata stands out by offering access to high-quality data through a meticulously crafted automated, AI-augmented process designed to extract valuable insights from websites. Our team ensures data precision and reliability, facilitating decision-making processes.
For more details, visit: https://outsourcebigdata.com/data-automation/web-scraping-services/web-data-extraction-services/.
About AIMLEAP
Outsource Bigdata is a division of Aimleap. AIMLEAP is an ISO 9001:2015 and ISO/IEC 27001:2013 certified global technology consulting and service provider offering AI-augmented Data Solutions, Data Engineering, Automation, IT Services, and Digital Marketing Services. AIMLEAP has been recognized as a ‘Great Place to Work®’.
With a special focus on AI and automation, we built quite a few AI & ML solutions, AI-driven web scraping solutions, AI-data Labeling, AI-Data-Hub, and Self-serving BI solutions. We started in 2012 and successfully delivered IT & digital transformation projects, automation-driven data solutions, on-demand data, and digital marketing for more than 750 fast-growing companies in the USA, Europe, New Zealand, Australia, Canada; and more.
-An ISO 9001:2015 and ISO/IEC 27001:2013 certified -Served 750+ customers -11+ Years of industry experience -98% client retention -Great Place to Work® certified -Global delivery centers in the USA, Canada, India & Australia
Our Data Solutions
APISCRAPY: AI driven web scraping & workflow automation platform APISCRAPY is an AI driven web scraping and automation platform that converts any web data into ready-to-use data. The platform is capable to extract data from websites, process data, automate workflows, classify data and integrate ready to consume data into database or deliver data in any desired format.
AI-Labeler: AI augmented annotation & labeling solution AI-Labeler is an AI augmented data annotation platform that combines the power of artificial intelligence with in-person involvement to label, annotate and classify data, and allowing faster development of robust and accurate models.
AI-Data-Hub: On-demand data for building AI products & services On-demand AI data hub for curated data, pre-annotated data, pre-classified data, and allowing enterprises to obtain easily and efficiently, and exploit high-quality data for training and developing AI models.
PRICESCRAPY: AI enabled real-time pricing solution An AI and automation driven price solution that provides real time price monitoring, pricing analytics, and dynamic pricing for companies across the world.
APIKART: AI driven data API solution hub APIKART is a data API hub that allows businesses and developers to access and integrate large volume of data from various sources through APIs. It is a data solution hub for accessing data through APIs, allowing companies to leverage data, and integrate APIs into their systems and applications.
Locations: USA: 1-30235 14656 Canada: +1 4378 370 063 India: +91 810 527 1615 Australia: +61 402 576 615 Email: [email protected]
2 notes
·
View notes
Text
How to Extract Amazon Product Prices Data with Python 3
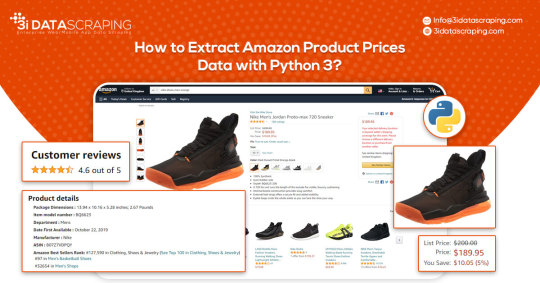
Web data scraping assists in automating web scraping from websites. In this blog, we will create an Amazon product data scraper for scraping product prices and details. We will create this easy web extractor using SelectorLib and Python and run that in the console.
#webscraping#data extraction#web scraping api#Amazon Data Scraping#Amazon Product Pricing#ecommerce data scraping#Data EXtraction Services
3 notes
·
View notes
Text
Kroger Grocery Data Scraping | Kroger Grocery Data Extraction
Shopping Kroger grocery online has become very common these days. At Foodspark, we scrape Kroger grocery apps data online with our Kroger grocery data scraping API as well as also convert data to appropriate informational patterns and statistics.
#food data scraping services#restaurantdataextraction#restaurant data scraping#web scraping services#grocerydatascraping#zomato api#fooddatascrapingservices#Scrape Kroger Grocery Data#Kroger Grocery Websites Apps#Kroger Grocery#Kroger Grocery data scraping company#Kroger Grocery Data#Extract Kroger Grocery Menu Data#Kroger grocery order data scraping services#Kroger Grocery Data Platforms#Kroger Grocery Apps#Mobile App Extraction of Kroger Grocery Delivery Platforms#Kroger Grocery delivery#Kroger grocery data delivery
2 notes
·
View notes
Text
#Web Scraping ASDA Liquor Products and Prices#Scrape Liquor Prices from ASDA#ASDA Alcohol Price Scraping Services#Extract Liquor Pricing Data from ASDA#Web Scraping ASDA Alcohol Prices#Extract Asda Alcohol and Liquor Price Data
0 notes
Text
The pharmaceutical industry is a highly competitive and dynamic sector where accurate and real-time data is essential. Monitoring drug prices and tracking pharmaceutical market trends enable businesses to make informed decisions, optimize pricing strategies, and remain competitive. Actowiz Solutions specializes in web scraping services that help pharmaceutical companies, healthcare providers, and regulatory bodies collect and analyze critical market data.
#Monitoring drug prices and tracking#web scraping and data extraction services#Scrapes drug pricing data#pharma web scraping
0 notes
Text
A Guide To Modern Data Extraction Services
As data surges with rapid technological breakthroughs and expanding industry capabilities, access to higher volume, laser-accurate, highly relevant and mission critical information becomes imperative to thrive in the market. In this guide, you’ll discover how modern data extraction services can transform your business and catapult you ahead of the competition. We cover everything from choosing the right strategy to implementing best practices and exploring how finding an ideal partner for your business can be game-changing.
What is Modern Data Extraction?
Modern data extraction harnesses cutting-edge technologies to efficiently collect, process, and analyze vast amounts of data from diverse sources. It employs AI-driven algorithms, machine learning, and cloud computing to deliver insights with unprecedented speed and accuracy. The goal is to empower businesses with timely, comprehensive, and actionable insights for strategic decision-making.
Businesses extract target data from various sources. The most common data sources are:
Websites: Critical information is available directly from various online sources.
Documents: Data from a wide range of document types, including emails, spreadsheets, PDFs, and images.
Databases: Structured and semi-structured data available in relational and non-relational databases.
Multimedia: Insights from visual and audio media content.
Custom: Tailored data is accessed from APIs, local drives, social media, and other unique sources.
Customer Data: Leverage your own treasure trove of customer interactions and behaviours.
Data Vendors: Augment your insights with specialized data from trusted providers.
Manual Data Collection: Complement automated processes with human-gathered intelligence.
Evolution of Data Extraction: Traditional to Modern
Technological advancements have driven the evolution of data extraction over the past decade. The market size is expected to grow from USD 2.33 billion in 2023 to USD 5.13 billion by 2030, with a compound annual growth rate (CAGR) of 11.9% (MMR).
Initially, data extraction relied heavily on manual processes, with large teams dedicating countless hours to painstaking data entry and basic extraction tasks. With the wave of globalization, these operations shifted offshore, taking advantage of cost efficiencies while maintaining the human-centric approach to data handling.
Alongside these manual efforts, early automation solutions emerged. However, their capabilities were limited, often requiring significant human oversight and intervention. This hybrid approach, combining manual with nascent automated tools, has characterized the data extraction landscape for years, and it has struggled to keep pace with the growing needs of the industry.
As digital transformation came into full swing, the volume and complexity of data skyrocketed. This growth catalyzed innovations in programming, giving rise to sophisticated computer algorithms for retrieving, modifying, and storing data. Enter the era of ETL (Extract, Transform, Load) processing and advanced data automation:
Extract: Extracting data from a variety of sources
Transform: Transforming the data per business rules
Load: Loading and storing data in the desired format
The flexibility of these automated workflows has created variations like ELT (Extract, Load, Transform) and ELTL (Extract, Load, Transform, Load), each tailored to specific industry needs and use cases.
Despite these advancements, new challenges have emerged in data management and scalability.
As businesses have expanded, the volume, variety, and velocity of extracted data have increased, overwhelming traditional systems. This has demanded more trailblazing approaches to data storage and processing.
To address these challenges, a trifecta of modern data storage solutions has emerged: data lakes, data warehouses, and data lakehouses. Each plays a crucial role in revolutionizing data management, offering unique advantages for different data needs.
Data lakes: Store vast amounts of raw, unprocessed data in its native format.
Data warehouses: Offer a structured approach to handling large volumes of data from multiple sources.
Data lakehouses: Combine the flexibility of data lakes with the performance features of data warehouses.
Complementing these storage solutions, cloud computing further redefined the data management landscape. By offering scalable infrastructure and on-demand resources, cloud platforms empower organizations to handle massive datasets and complex extraction tasks without significant upfront investments or commitments. Cloud-native data solutions leverage distributed computing to deliver unparalleled performance, reliability, and cost-efficiency.
This technological shift enabled organizations to process massive datasets and execute complex extraction tasks without substantial initial capital expenditure. The cloud’s elasticity and pay-as-you-go model democratized access to advanced data processing capabilities, facilitating the development and deployment of sophisticated data extraction technologies across various industries and organization sizes.
Understanding Modern Data Extraction Technologies
Modern data extraction technologies now leverage unprecedented data storage capacities and computing power to implement transformative strategies:
Automation: Identify repetitive tasks, streamline processes, reduce costs and process vast datasets with minimal manual intervention
Artificial Intelligence (AI) / Machine Learning (ML): Enhance decision-making, learn from patterns, and uncover hidden insights and continuous performance improvement through exposure to new data. AI/ML goes beyond rules-based logic to handle more complex situations, such as recognizing and maintaining relationships between interconnected data points across multiple data sources, building robust datasets from unstructured data or enabling advanced master data management without the need for explicit pre-defined rules
Natural Language Processing (NLP): Transform unstructured text data into actionable intelligence, mimicking human language understanding
Generative AI: Create human-like content, generate innovative solutions that can enhance big data quality, build intuition from currently available sources and checkpoints, provide deeper insights into performance and resolve inconsistencies with precision without human intervention and understand the context to produce relevant outputs across various domains
Artificial General Intelligence (AGI): While still largely theoretical, AI systems aim to match or exceed human-level intelligence. Development of AGI could revolutionize data extraction by enabling systems to understand and adapt to complex, novel situations without specific programming.
How Modern Data Extraction Changed Business Intelligence
AI and Natural Language Processing (NLP): NLP techniques extract valuable insights from unstructured text data at scale, enabling sophisticated sentiment analysis, topic modeling, and entity recognition. This capability transforms raw textual data into structured, actionable intelligence. Read more on: Introduction to News Crawlers: Powering Data Insights
Real-time Web Data Harvesting: Advanced web scraping techniques now enable the extraction of live data from dynamic websites. This provides crucial, up-to-the-minute insights for time-sensitive industries such as finance and e-commerce, facilitating rapid decision-making based on current market conditions. Read more on: Web Data Extraction: Techniques, Tools, and Applications
Intelligent Document Processing (IDP): AI-driven IDP systems automate the capture, classification, and extraction of data from diverse document types. Unlike traditional logic-based algorithms, these intelligent systems understand the context and build relationships between various data points, significantly enhancing the accuracy and depth of extracted information.
Generative AI in Data Augmentation: Emerging applications leverage generative models to create synthetic datasets for training models, eliminating the need for extensive labeling operations, augment existing data, provide summarization from vast resources of raw data, and assist in query formulation with human-like prompting, enabling users to “talk” to their data through visualizations, charts, or conversational interfaces. This technology expands the scope and quality of available data, enabling more robust analysis and model training.
Big Data and Cloud Computing Integration: The synergy between big data technologies and cloud computing enables real-time processing of vast datasets. This integration facilitates advanced analytics and drives the development of increasingly sophisticated extraction algorithms, all while optimizing infrastructure management, costs, processing speed, and data growth.
Custom Large Language Models (LLMs): Large Language Models, a subset of the AI/ML field, have fueled the evolution of Generative AI by exhibiting cognitive abilities to understand, process, and augment data with near-human intelligence. Building a custom LLM is equivalent to designing your own encyclopedia. Focused on your business needs, these models can help precisely identify areas of improvement, craft data-driven strategies, build resources to empower data use cases and enhance decision-making processes through intelligent automation and predictive analytics.
Retrieval-Augmented Generation (RAGs): Another breakthrough in enhancing capabilities for LLMs is the RAGs architecture. It blends the abilities of Information RAG Systems and Natural Language Generation to provide relevant and up-to-date insights. Imagine your custom LLMs (or encyclopedia for your business) always serving current data. An advanced responsibility is served by integrating RAGs with your LLMs.
Current Industry Challenges in Data Extraction
The transformative impact of modern data extraction technologies on business is undeniable. Yet, the accelerated evolution of these advanced solutions presents a paradox: as capabilities expand, so too does the complexity of implementation and integration. This complexity creates challenges in three key areas:
Business Challenges
Cost Management: Balancing investment in advanced extraction tools against potential ROI in a data-driven market.
Resource Allocation: Addressing the shortage of skilled data engineers and specialists while managing growing extraction needs.
Infrastructure Readiness: Upgrading systems to handle high-volume, real-time data extraction without disrupting operations.
Knowledge Gaps: Keeping teams updated on evolving extraction techniques, from web scraping to API integrations to Generative AI.
Decision-Making Complexity: Choosing between in-house solutions and third-party data extraction services in a crowded market.
Content Challenges
Unstructured Data: Extracting valuable insights from diverse sources like social media, emails, PDFs, etc. given the complex structure of embedded data that remains often inaccessible.
Data Freshness: Ensuring extracted data remains relevant in industries that require real-time data to serve their customer needs.
Ethical and Legal Considerations: Navigating data privacy regulations (GDPR, CCPA) while maintaining robust extraction practices.
Data Variety and Velocity: Handling the increasing diversity of data formats and the speed of data generation.
Technical Challenges
Data Quality: Maintaining accuracy and consistency when extracting from multiple and disparate sources.
Data Volume: Scaling extraction processes to handle terabytes of data without compromising performance or storage.
Scalability: Developing extraction systems that can grow with business needs and adapt to new data sources.
Flexibility: Fine-tuning data pipelines to accommodate changing requirements to meet business needs.
Integration with Existing Systems: Seamlessly incorporating extracted data into legacy systems and business intelligence tools.
Adopting Data Extraction Services in 2024
In 2024, an age of urgency, enterprises need efficient, plug-and-play data extraction solutions. As companies navigate the data-driven force, choosing the right extraction strategy is crucial.
Key pillars of a robust strategy include:
Identifying Your Business Needs
Assessing What Data is Essential to Your Business Goals: Determine which data directly supports your objectives. This could be business data enrichment, social media data stream, online news aggregation, or automated processing of millions of documents. Knowing what matters most helps focus your extraction efforts on the valuable sources.
Determining the Frequency, Volume, and Type of Data Required: Consider how often you need data updates, how much data you’re dealing with, and in what format it’s available. This could range from real-time streams to periodic updates or large historical datasets.
Choosing the Right Solution
Evaluating Vendors and Technologies Based on Your Specific Requirements: Carefully assess potential solutions. The key function to target is their strategic capabilities and partnership strength — this helps in aligning objectives from the outset and setting you up for streamlined operations. Additional areas are technology stack, integration ease, end-to-end data management support, and the ability to handle your critical data types. This ensures the chosen solution fits your business needs and technical capabilities.
Comparing In-house vs. Outsourced Data Extraction Solutions: Decide whether to manage extraction internally or outsource. In-house offers more control but requires significant resources. Outsourcing provides expert knowledge with less upfront investment. Weigh these options to find the best fit for your needs.
Working with Best Practices
Compatibility with Existing Workflows: The solution should ensure smooth integration with your current systems. This minimizes disruption and allows teams to use extracted data effectively without major process changes.
Data Quality and Accuracy: The solution should implement strong validation processes to support data integrity. This ensures your extracted data is accurate, complete, and consistent, enhancing decision-making and building trust in the data across your organization.
Scalability and Flexibility: The solution should provide scalability to meet your future needs. It should handle increasing data volumes without performance issues and adapt to changing business requirements and new technologies.
Data Security and Compliance: The solution should prioritize safeguarding your data. It should employ encryption, strict access controls, and regular audits to comply with regulations like GDPR and CCPA. This reduces risk and enhances your reputation as a trusted partner.
Continuous Improvement: The solution should have room for learning and improvements. It should support regular review and optimization of your processes. This includes monitoring performance, gathering user feedback, and staying informed about new trends to ensure your strategy remains effective and aligned with your goals.
Forage AI: Your One-Stop Data Automation Partner
We understand that managing the complexities of data extraction can seem overwhelming. At Forage AI, we specialize in providing robust solutions to these complex challenges. Our comprehensive suite of modern data extraction solutions address all the aspects discussed above and more. We design our full spectrum of services to be relevant to your data needs.
Multi-Modal Data Extraction: Our robust solutions use advanced techniques for data extraction from the web and documents. Coupled with battle-tested, multi-layered QA, you can unlock a treasure trove of insights.
Change Detection: Our bespoke solutions monitor, extract and report real-time changes, ensuring your data stays fresh and accurate.
Data Governance: We are GDPR and CCPA compliant, ensuring your data is secure and meets all regulatory standards.
Automation and NLP: We know exactly when and how to integrate these technologies to enhance your business processes. Our advanced techniques help preprocess and clean data going from noisy raw data to preparing high-value datasets.
Generative AI Integration: We stay at the forefront of innovation by wisely integrating Generative AI into our solutions, bringing new levels of automation and efficiency. Our approach is measured and responsible — carefully addressing common pitfalls like data bias and ensuring compliance with industry standards. By embracing this technology strategically, we deliver cutting-edge features while maintaining the accuracy, security, and reliability your business depends on.
Data Delivery Assurance: We provide full coverage with no missing data, and resilient data pipelines with SLAs in place.
Tailored Approach: We create custom plans relevant to your processes. This allows for tight data management, and flexibility to integrate with existing data systems.
True Partnership: We launch quickly, work closely with you, and focus on your success.
Final Thoughts
As we ride the waves of relentless innovation in 2024, where yesterday’s cutting-edge is today’s status quo, the critical role of modern data extraction services in driving business success becomes increasingly apparent. The evolution from manual processes to sophisticated AI-driven techniques represents a paradigm shift in how organizations acquire, process, and leverage information. This transformation offers unprecedented opportunities for gaining deeper insights, facilitating data-driven decision-making, and maintaining a competitive edge in an increasingly complex market environment.
The efficacy of these advanced data extraction methodologies hinges on access to high-quality, relevant data sources. Organizations must recognize that the value derived from data extraction technologies is directly proportional to the quality and relevance of the input data. As such, investing in premium data sources and maintaining robust data governance practices are essential components of a successful data strategy.
The future trajectory of data extraction technologies is promising, with emergent fields such as Generative AI and advanced Natural Language Processing techniques poised to further expand the capabilities of data extraction systems. However, it is crucial to recognize that the key to unlocking the full potential of these technologies lies not merely in their adoption, but in their strategic implementation and integration within existing business processes.
Those who successfully harness the power of advanced data extraction technologies will be well-positioned to thrive in an increasingly data-driven global economy, gaining actionable insights that drive innovation, enhance decision-making, and create sustainable competitive advantages.
Take the Next Step
Transform your business intelligence capabilities with Forage AI’s tailored data automation solutions. Our expert team stands ready to work with you through the complexities of modern data acquisition and analysis. Schedule a consultation today to explore how Forage AI’s advanced extraction techniques can unlock the full potential of your data assets and position your organization at the forefront of your industry.
#artificial intelligence#Web data extraction#data extraction#Data extraction services#machine learning#startup
0 notes
Text
Web Scraping 101: Everything You Need to Know in 2025
🕸️ What Is Web Scraping? An Introduction
Web scraping—also referred to as web data extraction—is the process of collecting structured information from websites using automated scripts or tools. Initially driven by simple scripts, it has now evolved into a core component of modern data strategies for competitive research, price monitoring, SEO, market intelligence, and more.
If you’re wondering “What is the introduction of web scraping?” — it’s this: the ability to turn unstructured web content into organized datasets businesses can use to make smarter, faster decisions.
💡 What Is Web Scraping Used For?
Businesses and developers alike use web scraping to:
Monitor competitors’ pricing and SEO rankings
Extract leads from directories or online marketplaces
Track product listings, reviews, and inventory
Aggregate news, blogs, and social content for trend analysis
Fuel AI models with large datasets from the open web
Whether it’s web scraping using Python, browser-based tools, or cloud APIs, the use cases are growing fast across marketing, research, and automation.
🔍 Examples of Web Scraping in Action
What is an example of web scraping?
A real estate firm scrapes listing data (price, location, features) from property websites to build a market dashboard.
An eCommerce brand scrapes competitor prices daily to adjust its own pricing in real time.
A SaaS company uses BeautifulSoup in Python to extract product reviews and social proof for sentiment analysis.
For many, web scraping is the first step in automating decision-making and building data pipelines for BI platforms.
⚖️ Is Web Scraping Legal?
Yes—if done ethically and responsibly. While scraping public data is legal in many jurisdictions, scraping private, gated, or copyrighted content can lead to violations.
To stay compliant:
Respect robots.txt rules
Avoid scraping personal or sensitive data
Prefer API access where possible
Follow website terms of service
If you’re wondering “Is web scraping legal?”—the answer lies in how you scrape and what you scrape.
🧠 Web Scraping with Python: Tools & Libraries
What is web scraping in Python? Python is the most popular language for scraping because of its ease of use and strong ecosystem.
Popular Python libraries for web scraping include:
BeautifulSoup – simple and effective for HTML parsing
Requests – handles HTTP requests
Selenium – ideal for dynamic JavaScript-heavy pages
Scrapy – robust framework for large-scale scraping projects
Puppeteer (via Node.js) – for advanced browser emulation
These tools are often used in tutorials like “Web scraping using Python BeautifulSoup” or “Python web scraping library for beginners.”
⚙️ DIY vs. Managed Web Scraping
You can choose between:
DIY scraping: Full control, requires dev resources
Managed scraping: Outsourced to experts, ideal for scale or non-technical teams
Use managed scraping services for large-scale needs, or build Python-based scrapers for targeted projects using frameworks and libraries mentioned above.
🚧 Challenges in Web Scraping (and How to Overcome Them)
Modern websites often include:
JavaScript rendering
CAPTCHA protection
Rate limiting and dynamic loading
To solve this:
Use rotating proxies
Implement headless browsers like Selenium
Leverage AI-powered scraping for content variation and structure detection
Deploy scrapers on cloud platforms using containers (e.g., Docker + AWS)
🔐 Ethical and Legal Best Practices
Scraping must balance business innovation with user privacy and legal integrity. Ethical scraping includes:
Minimal server load
Clear attribution
Honoring opt-out mechanisms
This ensures long-term scalability and compliance for enterprise-grade web scraping systems.
🔮 The Future of Web Scraping
As demand for real-time analytics and AI training data grows, scraping is becoming:
Smarter (AI-enhanced)
Faster (real-time extraction)
Scalable (cloud-native deployments)
From developers using BeautifulSoup or Scrapy, to businesses leveraging API-fed dashboards, web scraping is central to turning online information into strategic insights.
📘 Summary: Web Scraping 101 in 2025
Web scraping in 2025 is the automated collection of website data, widely used for SEO monitoring, price tracking, lead generation, and competitive research. It relies on powerful tools like BeautifulSoup, Selenium, and Scrapy, especially within Python environments. While scraping publicly available data is generally legal, it's crucial to follow website terms of service and ethical guidelines to avoid compliance issues. Despite challenges like dynamic content and anti-scraping defenses, the use of AI and cloud-based infrastructure is making web scraping smarter, faster, and more scalable than ever—transforming it into a cornerstone of modern data strategies.
🔗 Want to Build or Scale Your AI-Powered Scraping Strategy?
Whether you're exploring AI-driven tools, training models on web data, or integrating smart automation into your data workflows—AI is transforming how web scraping works at scale.
👉 Find AI Agencies specialized in intelligent web scraping on Catch Experts,
📲 Stay connected for the latest in AI, data automation, and scraping innovation:
💼 LinkedIn
🐦 Twitter
📸 Instagram
👍 Facebook
▶️ YouTube
#web scraping#what is web scraping#web scraping examples#AI-powered scraping#Python web scraping#web scraping tools#BeautifulSoup Python#web scraping using Python#ethical web scraping#web scraping 101#is web scraping legal#web scraping in 2025#web scraping libraries#data scraping for business#automated data extraction#AI and web scraping#cloud scraping solutions#scalable web scraping#managed scraping services#web scraping with AI
0 notes
Text
LinkedIn Job Scrapper
LinkedIn Job Scraper: A Comprehensive Guide to Extracting Job Listings and Data
LinkedIn has become an indispensable platform for professionals seeking new career opportunities and for recruiters looking to find the best talent. It's a treasure trove of job listings and valuable data. However, manually searching for jobs and extracting data can be time-consuming. That's where LinkedIn Job Scraper comes into play. In this comprehensive guide, we will explore what LinkedIn Job Scraper is, why you might want to use it, its legality and ethics, and how to effectively build and use a scraper.
What is a LinkedIn Job Scraper?
LinkedIn Job Scraper refers to a software tool or program that is designed to extract job listings and related data from LinkedIn, a popular professional networking platform. This tool automates the process of collecting information about job opportunities, such as job titles, company details, job descriptions, and other relevant data, from LinkedIn's job postings.
LinkedIn Job Scrapers are typically used by job seekers, recruiters, and data analysts
Why Use LinkedIn Job Scraper?
LinkedIn Job Scraper offers several advantages for job seekers, recruiters, and businesses:
Efficient Job Searching: For job seekers, it automates the process of searching for job listings that match their criteria, saving time compared to manual searching. It allows users to access a large number of job postings quickly and efficiently, increasing their chances of finding the right opportunity .
Streamlined Recruitment: Recruiters can use LinkedIn scrapers to gather relevant candidate profiles, helping them streamline the recruitment process. This tool automates the collection of candidate data, making it easier to identify potential hires.
Data-Driven Insights: Businesses can benefit from scraping job data to gain insights into job market trends, analyze companies' hiring practices, and gather data for various studies. This data-driven approach can inform strategic decisions related to hiring and workforce planning .
Competitive Analysis: Businesses can use job scraping to monitor job postings by competitors, helping them understand their hiring strategies and stay competitive in the talent market. This insight can be invaluable for staying ahead in the industry .
Consolidation of Job Posts: LinkedIn Job Scrapers can consolidate job posts from various sources into a single database. This feature is particularly useful for aggregating job listings from multiple job sites, making it easier for users to access a wide range of opportunities without the need to visit multiple platforms .
Cost-Effective Solutions: Some LinkedIn scraping tools offer cost-effective solutions for scraping and extracting data. This can be an affordable way to gather information from LinkedIn without the need for extensive manual labor.
Utilizing Pre-built Tools
Utilizing pre-built tools for web scraping, such as those designed for LinkedIn scraping, offers several advantages, including ease of use and time savings. Here are some pre-built tools that can help you in your web scraping endeavors:
Phantombuster: Phantombuster is a comprehensive web scraping tool that supports various platforms, including LinkedIn. It allows users to automate data extraction from LinkedIn profiles, posts, and more.
Captain Data: Captain Data is another versatile data scraping tool that can be used for LinkedIn scraping. It offers a user-friendly interface and supports data extraction from various sources.
La Growth Machine: This tool specializes in LinkedIn automation and data scraping. It can be useful for extracting data related to job postings, candidate profiles, and more.
Waalaxy: Waalaxy is a LinkedIn automation platform that includes scraping features. It can help automate tasks like connection requests, messaging, and profile scraping.
Dux-Soup: Dux-Soup is a LinkedIn automation tool that also supports scraping. It offers features for extracting data from profiles and automating various LinkedIn activities.
lemlist: lemlist provides automation and scraping capabilities for LinkedIn. It focuses on personalized outreach but can also be used for data extraction.
Evaboot: Evaboot is a LinkedIn automation and scraping tool designed to streamline outreach and data collection on the platform.
TexAu: TexAu is an all-in-one automation platform that includes LinkedIn scraping capabilities. It supports various LinkedIn-related tasks.
Linked Helper: Linked Helper is a LinkedIn automation tool with scraping features. It can automate connections, messaging, and data extraction.
Surfe (ex-Leadjet): Surfe is a LinkedIn scraping tool that enables users to collect data from LinkedIn profiles, including job-related information.
Real-World Applications
LinkedIn Job Scraper, or tools designed for scraping job postings and related data from LinkedIn, has real-world applications across various sectors:
Job Seekers:
Efficient Job Search: Job seekers can use LinkedIn scrapers to efficiently search for job listings that match their qualifications and preferences. These tools can help in quickly identifying relevant job opportunities.
Data Analysis: Scrapped job data can be analyzed to identify trends in job markets, helping job seekers make informed decisions about their career paths.
Recruiters:
Candidate Sourcing: Recruiters can use LinkedIn scrapers to gather candidate profiles and resumes that match specific job requirements. This speeds up the candidate sourcing process.
Talent Pool Management: LinkedIn scrapers enable recruiters to build and manage talent pools for future job openings. They can store candidate information for future reference.
Businesses:
Competitor Analysis: Businesses can monitor job postings by competitors on LinkedIn to gain insights into their hiring strategies and the skills they are seeking.
Market Research: Scraped job data can be used for market research to understand hiring trends, skill demand, and the overall job market health.
HR Analytics: HR departments can use LinkedIn scraping to analyze their own job postings and applicant data to make data-driven HR decisions.
Academic and Research: Researchers and academics can use LinkedIn scraping tools to collect data for studies related to employment trends, skills demand, and labor market analysis.
Career Counselors and Coaches: Professionals in career guidance can utilize scraped job data to provide informed advice to clients about job prospects and industry trends.
Government and Workforce Development: Government agencies and organizations responsible for workforce development can use scraped data to understand regional job markets and create strategies for employment growth.
Freelancers and Gig Workers: Freelancers can use scrapers to find short-term gigs and contract work on LinkedIn, making it easier to identify relevant projects.
Data Analysis and AI: Data scientists and AI developers can use scraped job data to develop algorithms and models for predicting job trends, skill demand, and salary expectations.
These real-world applications demonstrate the versatility of LinkedIn scrapers in supporting job-related activities, whether it's job searching, recruitment, business strategy, research, or career development. However, it's crucial to use these tools responsibly and in compliance with legal and ethical considerations.
Conclusion
LinkedIn scraping has become a crucial aspect of data acquisition in various fields, including business, research, and recruitment. The key takeaways regarding LinkedIn scraping and its future are as follows:
Growing Importance: Data scraping, especially from LinkedIn, is gaining popularity due to its significance in understanding market trends, making informed business decisions, and sourcing talent efficiently. It has become an essential tool for many professionals and organizations.
Automation: Automation is a significant trend in LinkedIn scraping. Businesses are increasingly using automated scraping tools to streamline data collection processes and free up time for other critical tasks. Automation is expected to continue evolving and becoming more accessible.
Business Impact: LinkedIn scraping has a significant impact on investment decisions and business strategies. By extracting data on startups' growth signals and market trends, it enables businesses to make informed choices, which, in turn, affect their bottom line positively.
Regulation and Compliance: As data scraping continues to grow, it is likely that there will be increased attention on data privacy and regulation. Scrappers need to stay updated with legal requirements and best practices to maintain the ethical and legal use of scraped data.
User-Friendly Tools: LinkedIn scraping tools are becoming more user-friendly and accessible, allowing individuals and organizations to harness the power of web scraping without extensive technical expertise. The future of LinkedIn scraping appears promising, with a focus on simplicity, efficiency, and responsible use. As technology evolves, scraping tools are expected to become even more sophisticated, offering greater insights into LinkedIn data and its applications. However, ethical considerations and compliance with data privacy regulations will remain essential to ensure the responsible use of scraped information.
0 notes
Text
Best Tools & Techniques for Data Extraction from Multiple Sources

Data extraction is common and rapidly grown in the business landscape. As the technology advances, it is vital to update tools and techniques for best extracted outcomes. Read further in detail about tools and techniques for data extraction services.
#data extraction services#data scraping services#data extraction company#data digitization#web data extraction services#data extraction services india#data extraction companies#outsource data extraction#outsource data extraction services
3 notes
·
View notes
Text
Automating Data Collection for Market Research with Web Scraping
0 notes
Text
Flight Price Monitoring Services | Scrape Airline Data
We Provide Flight Price Monitoring Services in USA, UK, Singapore, Italy, Canada, Spain and Australia and Extract or Scrape Airline Data from Online Airline / flight website and Mobile App like Booking, kayak, agoda.com, makemytrip, tripadvisor and Others.

#flight Price Monitoring#Scrape Airline Data#Airfare Data Extraction Service#flight prices scraping services#Flight Price Monitoring API#web scraping services
2 notes
·
View notes