#Large Language Model Powered Tools Market Scope
Explore tagged Tumblr posts
Text
Large Language Model Powered Tools Market Size, Share, Analysis, Forecast, and Growth Trends to 2032: Enterprise Adoption and Use Case Expansion
The Large Language Model Powered Tools Market was valued at USD 1.8 Billion in 2023 and is expected to reach USD 66.2 Billion by 2032, growing at a CAGR of 49.29% from 2024-2032.
The Large Language Model (LLM) Powered Tools Market is witnessing a transformative shift across industries, driven by rapid advancements in artificial intelligence and natural language processing. Organizations are adopting LLM-powered solutions to streamline operations, automate workflows, enhance customer service, and unlock new efficiencies. With capabilities like contextual understanding, semantic search, and generative content creation, these tools are reshaping how businesses interact with data and customers alike.
Large Language Model Powered Tools Market is evolving from niche applications to becoming essential components in enterprise tech stacks. From finance to healthcare, education to e-commerce, LLM-powered platforms are integrating seamlessly with existing systems, enabling smarter decision-making and reducing human dependency on repetitive cognitive tasks. This progression indicates a new era of intelligent automation that extends beyond traditional software functionalities.
Get Sample Copy of This Report: https://www.snsinsider.com/sample-request/5943
Market Keyplayers:
Google LLC – Gemini
Microsoft Corporation – Azure OpenAI Service
OpenAI – ChatGPT
Amazon Web Services (AWS) – Amazon Bedrock
IBM Corporation – Watsonx
Meta Platforms, Inc. – LLaMA
Anthropic – Claude AI
Cohere – Cohere Command R+
Hugging Face – Transformers Library
Salesforce, Inc. – Einstein GPT
Mistral AI – Mistral 7B
AI21 Labs – Jurassic-2
Stability AI – Stable LM
Baidu, Inc. – Ernie Bot
Alibaba Cloud – Tongyi Qianwen
Market Analysis The growth of the LLM-powered tools market is underpinned by increasing investments in AI infrastructure, rising demand for personalized digital experiences, and the scalability of cloud-based solutions. Businesses are recognizing the competitive advantage that comes with harnessing large-scale language understanding and generation capabilities. Moreover, the surge in multilingual support and cross-platform compatibility is making LLM solutions accessible across diverse markets and user segments.
Market Trends
Integration of LLMs with enterprise resource planning (ERP) and customer relationship management (CRM) systems
Surge in AI-driven content creation for marketing, documentation, and training purposes
Emergence of domain-specific LLMs offering tailored language models for niche sectors
Advancements in real-time language translation and transcription services
Rising focus on ethical AI, transparency, and model explainability
Incorporation of LLMs in low-code/no-code development platforms
Increased adoption of conversational AI in customer support and virtual assistants
Market Scope The scope of the LLM-powered tools market spans a wide range of industries and application areas. Enterprises are leveraging these tools for document summarization, sentiment analysis, smart search, language-based coding assistance, and more. Startups and established tech firms alike are building platforms that utilize LLMs for productivity enhancement, data extraction, knowledge management, and decision intelligence. As API-based and embedded LLM solutions gain popularity, the ecosystem is expanding to include developers, system integrators, and end-user organizations in both B2B and B2C sectors.
Market Forecast The market is projected to experience robust growth in the coming years, driven by innovation, increasing deployment across verticals, and rising digital transformation efforts globally. New entrants are introducing agile and customizable LLM tools that challenge traditional software paradigms. The convergence of LLMs with other emerging technologies such as edge computing, robotics, and the Internet of Things (IoT) is expected to unlock even more disruptive use cases. Strategic partnerships, mergers, and platform expansions will continue to shape the competitive landscape and accelerate the market’s trajectory.
Access Complete Report: https://www.snsinsider.com/reports/large-language-model-powered-tools-market-5943
Conclusion As businesses worldwide pursue smarter, faster, and more intuitive digital solutions, the Large Language Model Powered Tools Market stands at the forefront of this AI revolution. The convergence of language intelligence and machine learning is opening new horizons for productivity, engagement, and innovation. Forward-thinking companies that embrace these technologies now will not only gain operational advantages but also set the pace for the next generation of intelligent enterprise solutions.
About Us:
SNS Insider is one of the leading market research and consulting agencies that dominates the market research industry globally. Our company's aim is to give clients the knowledge they require in order to function in changing circumstances. In order to give you current, accurate market data, consumer insights, and opinions so that you can make decisions with confidence, we employ a variety of techniques, including surveys, video talks, and focus groups around the world.
Contact Us:
Jagney Dave - Vice President of Client Engagement
Phone: +1-315 636 4242 (US) | +44- 20 3290 5010 (UK)
#Large Language Model Powered Tools Market#Large Language Model Powered Tools Market Scope#Large Language Model Powered Tools Market Trends
0 notes
Text
What is Video Poet? Unleash Your Creative Potential with Free Text to Video AI !
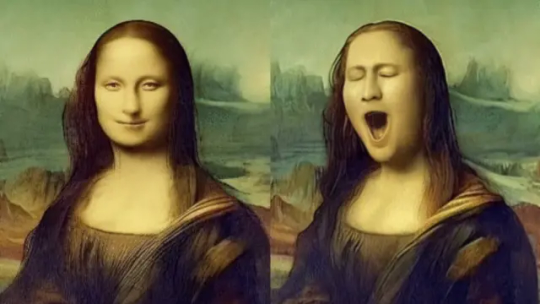
In the ever-evolving world of technology, Google's VideoPoet emerges as a game-changer in the realm of video generation. As a sophisticated Large Language Model (LLM), VideoPoet is not just a tool; it's a harbinger of a new era in visual storytelling. The Innovation of VideoPoet VideoPoet harnesses the power of LLMs to transform various inputs, such as text, images, and video clips, into high-quality videos. What sets it apart is its zero-shot learning capability, allowing it to produce dynamic, high-motion videos without extensive specialized training. Understanding VideoPoet’s Mechanism At its core, VideoPoet relies on multiple tokenizers to process different modalities - video, image, audio, and text. Each tokenizer, such as MAGVIT V2 for video and SoundStream for audio, plays a crucial role in converting these signals into a language the model understands. This intricate process enables VideoPoet to blend various content forms seamlessly.
VideoPoet’s Versatile Applications From animating still images to applying unique styles to videos, VideoPoet’s applications are vast. It can create videos that fill in missing elements or extend beyond their original scope, offering innovative solutions for content creation. The Future of Visual Storytelling VideoPoet is not just a technological marvel; it's a canvas for creativity. It opens up new avenues in fields like advertising, filmmaking, and digital content creation, where the boundaries of imagination are constantly being pushed. The Technical Breakthrough of VideoPoet Understanding VideoPoet's advanced mechanics offers a glimpse into its extraordinary capabilities. The platform utilizes state-of-the-art tokenizers for each modality it processes. For instance, the MAGVIT V2 tokenizer intricately handles video and images, capturing both spatial and temporal information. This precision is crucial in creating fluid, lifelike videos from static inputs. Similarly, the SoundStream tokenizer revolutionizes audio processing with its nuanced understanding of sound patterns, making the audio-video synchronization in VideoPoet remarkably realistic. Expanding Creative Horizons VideoPoet is not just a tool for creating content; it's a catalyst for creative exploration. Its ability to animate images, style videos, and even repair or expand existing videos opens up a world of possibilities for content creators. Imagine transforming a simple sketch into a full-fledged animated story or restyling a classic film scene into a modern art piece. VideoPoet makes these imaginative scenarios possible. Empowering Content Creators and Marketers In the realm of marketing and content creation, VideoPoet is a game-changer. It offers brands and creators a powerful way to convey their messages more engagingly and memorably. Whether it's for creating compelling advertisements, enhancing social media content, or producing educational materials, VideoPoet provides a platform that amplifies creativity and effectiveness.
Examples that would blow your mind
Text to video Text prompt: Two pandas playing cards
Image to video with text prompts Text prompt accompanying the images (from left): 1. A ship navigating the rough seas, thunderstorm and lightning, animated oil on canvas 2. Flying through a nebula with many twinkling stars 3. A wanderer on a cliff with a cane looking down at the swirling sea fog below on a windy day Image (left) and video generated (immediate right)
Credit: Google Zero-shot video stylization VideoPoet can also alter an existing video, using text prompts. In the examples below, the left video is the original and the one right next to it is the stylized video. From left: Wombat wearing sunglasses holding a beach ball on a sunny beach; teddy bears ice skating on a crystal clear frozen lake; a metal lion roaring in the light of a forge.
Credit: Google Video to audio The researchers first generated 2-second video clips and VideoPoet predicts the audio without any help from text prompts. VideoPoet also can create a short film by compiling several short clips. First, the researchers asked Bard, Google’s ChatGPT rival, to write a short screenplay with prompts. They then generated video from the prompts and then put everything together to produce the short film. Longer videos, editing and camera motion Google said VideoPoet can overcome the problem of generating longer videos by conditioning the last second of videos to predict the next second. “By chaining this repeatedly, we show that the model can not only extend the video well but also faithfully preserve the appearance of all objects even over several iterations,” they wrote. VideoPoet can also take existing videos and change how the objects in it move. For example, a video of the Mona Lisa is prompted to yawn.
Credit: Google Text prompts can also be used to change camera angles in existing images. For example, this prompt created the first image: Adventure game concept art of a sunrise over a snowy mountain by a crystal clear river. Then the following prompts were added, from left to right: Zoom out, Dolly zoom, Pan left, Arc shot, Crane shot, and FPV drone shot.
Ethical and Societal Implications As with any advanced technology, VideoPoet comes with its set of ethical considerations. The ease of creating realistic videos raises questions about authenticity and the potential for misuse. It's crucial for users and developers alike to navigate these challenges responsibly, ensuring that this powerful tool is used for positive and ethical purposes. Looking to the Future VideoPoet is not just a current marvel; it's a stepping stone to the future of digital storytelling. As AI continues to evolve, we can expect even more sophisticated and intuitive tools that further blur the lines between reality and digital creation. VideoPoet is leading the way, showing us a glimpse of the potential that AI holds in transforming how we see, interpret, and create our narratives. In conclusion, Google's VideoPoet stands as a testament to the incredible advancements in AI and machine learning. It's a tool that not only enhances the way we produce and consume video content but also challenges us to rethink the boundaries of creativity and technology. As we move forward, VideoPoet will undoubtedly continue to inspire and revolutionize the landscape of visual storytelling. Read the full article
2 notes
·
View notes
Text
AI is Driving Investment — But Entrepreneurs Need to be Careful With What They Claim
New Post has been published on https://thedigitalinsider.com/ai-is-driving-investment-but-entrepreneurs-need-to-be-careful-with-what-they-claim/
AI is Driving Investment — But Entrepreneurs Need to be Careful With What They Claim
Artificial intelligence (AI) remains one of the strongest drivers of venture capital investment, proving that the hype cycle isn’t even close to finished. According to a recent EY report, 37% of fundraising in the third quarter of 2024 was for AI-related companies, similar to second-quarter volume. Startups using AI are getting noticed for their ability to tackle big problems in robotics, automation, healthcare, logistics, and more. But the reality is that investors hear, “We’re using AI” all day. The degree to which entrepreneurs actually use it varies substantially. There is even backlash from investors, including a 31-page report by Goldman Sachs that questions how worthy AI is of investment.
The Federal Trade Commission (FTC) recently announced a crackdown on companies making deceptive AI claims. This “AI washing” — lobbing AI into marketing without backing it up — might grab attention, but it’s a fast track to losing credibility. Founders need to communicate clearly and honestly about how AI fits into their business. The focus has to be on actual innovation, not just chasing buzzwords.
It is critical to avoid situations like Theranos, where bold claims were made without substance, leading to severe consequences. The stakes are even higher with AI, as the technical complexity makes it harder to verify claims of how it’s used and easier for misuse to slip through. According to insurer Allianz, 38 AI-related securities class action lawsuits were filed between March 2020 and October 2024 — 13 of them came in 2024 alone.
AI’s appeal to investors isn’t just about technical sophistication. It’s about solving problems that matter and creating a real business. Founders who take shortcuts or exaggerate their AI capabilities risk alienating the very backers they’re trying to attract. With regulators sharpening their scrutiny and the market growing more discerning, delivering substance is essential.
AI’s broad reach
Artificial intelligence encompasses far more than the conversational AI tools that dominate headlines. Patrick Winston, the late computer scientist and professor at MIT, outlined the foundational elements of AI more than 30 years ago in his seminal textbook, “Artificial Intelligence.” Long before large language models captured the public’s imagination, AI was driving advancements in problem solving, quantitative reasoning, and algorithmic control. These roots highlight the diverse applications of AI beyond chatbots and natural language processing.
Consider the role of AI in robotics and computer vision. Simultaneous localization and mapping (SLAM), for example, is a groundbreaking technique enabling machines to navigate and interpret environments. It underpins critical autonomous systems and exemplifies AI’s capability to address complex technical challenges. While not as widely recognized as large language models, these advancements are just as transformative.
Fields such as speech recognition and computer vision, once considered AI innovations, have since matured into distinct disciplines, transforming industries in the process and, in many cases, losing the ‘AI’ label. Speech recognition has revolutionized accessibility and voice-driven interfaces, while computer vision powers advancements in areas like autonomous vehicles, medical imaging, face recognition, and retail analytics. For founders, this underscores the importance of articulating how their innovations fit within AI’s broader landscape. Demonstrating a nuanced understanding of AI’s scope enables startups to stand out in an increasingly competitive funding ecosystem for early-stage companies.
For instance, machine learning models can optimize supply chain logistics, predict equipment failures, or enable dynamic pricing strategies. These applications may not command the same attention as chatbots, but they offer immense value to industries focused on efficiency and innovation.
Speaking investors’ language
When communicating to founders how they use AI, founders should focus on measurable impacts, such as improved efficiency, better user outcomes, or unique technical advantages. Many investors are not deeply technical, so it’s essential to present AI capabilities in simple, accessible language. Explaining what the AI does, how it works, and why it matters builds trust and credibility.
Investors are growing weary of hearing the term “AI,” concerned that entrepreneurs are over-branding their ventures with the technology instead of how it helps them solve problems. AI has become table stakes in many industries, and its role should not be overstated in a company’s strategy.
Equally important is transparency. With the FTC cracking down on exaggerated AI claims, being truthful about what your technology can and cannot do is a necessity. Overstating capabilities might generate initial interest but can quickly backfire, leading to reputational damage or regulatory scrutiny.
Founders should also highlight how their use of AI aligns with broader market opportunities. For example, leveraging AI for predictive analytics, optimization, or decision-making systems can demonstrate foresight and innovation. These applications may not dominate headlines like chatbots, but they address real-world needs that resonate with investors.
Ultimately, it’s about presenting AI as a tool that drives value and solves pressing problems. By focusing on clear communication, honesty, and alignment with investor priorities, founders can position themselves as credible and forward-thinking leaders in the AI space.
#2024#Accessibility#ai#AI Investment#ai tools#ai washing#Analytics#applications#artificial#Artificial Intelligence#attention#automation#autonomous#autonomous systems#autonomous vehicles#Branding#Business#chatbots#command#communication#Companies#complexity#computer#Computer vision#conversational ai#Deceptive AI#driving#efficiency#Entrepreneur#equipment
0 notes
Text
ASX AI Stocks: Shaping the Future of Technology
Highlights:
ASX AI stocks are part of the growing artificial intelligence sector on the Australian Securities Exchange (ASX).
Companies in this field are innovating AI technologies to address challenges in industries like healthcare, finance, and manufacturing.
The AI market is rapidly expanding, with ASX-listed companies contributing to technological advancements.
The Australian Securities Exchange (ASX) hosts a range of companies engaged in the development and application of artificial intelligence (AI) solutions. AI is playing a pivotal role in transforming industries across the globe, and Australian businesses are increasingly at the forefront of this technological shift. These ASX AI stocks are advancing solutions that have the potential to change how industries operate and provide new capabilities in areas like healthcare, finance, and manufacturing.
AI’s Impact Across Various Industries
Artificial intelligence is now a vital component of numerous industries, and ASX AI stocks are contributing to this digital transformation. The ability of AI to analyze large datasets quickly and accurately is revolutionizing decision-making processes in sectors ranging from healthcare to finance and manufacturing.
In healthcare, AI-powered systems are used for medical imaging analysis, patient monitoring, and even the development of personalized treatment regimens. AI in finance is helping companies streamline operations, detect fraud, and automate processes that were once manual. In manufacturing, AI systems are boosting efficiency, ensuring product quality, and optimizing supply chains, demonstrating its wide-ranging applications.
Advancements in AI Technologies
ASX-listed companies are pushing the boundaries of AI, utilizing cutting-edge technologies such as machine learning, neural networks, and natural language processing. These innovations are enhancing automation capabilities and enabling more intelligent data-driven decision-making across different sectors.
Machine learning algorithms are being applied to improve predictive models, while natural language processing tools are being used to enhance customer service and business intelligence. As these technologies evolve, the scope of AI applications is expanding rapidly, creating opportunities for businesses that can leverage AI effectively.
Market Growth and Demand for AI Solutions
The demand for AI technologies is growing globally, and ASX AI stocks are positioned to play a significant role in this expanding market. As businesses increasingly recognize the potential of AI to improve productivity and reduce operational costs, there is a greater need for companies that can provide these advanced solutions.
This increasing demand is not limited to large corporations. Small and medium-sized enterprises (SMEs) are also exploring AI adoption, seeking ways to enhance their operations and compete in the digital age. This trend is expected to continue, with AI technologies becoming even more ingrained in everyday business practices.
Challenges and Risks for AI Companies
While the growth of AI presents substantial benefits, there are challenges for ASX AI stocks to navigate. Ethical issues, such as data privacy concerns and the potential for AI bias, remain a critical consideration. The rapid pace of technological advancement also means companies must remain agile to maintain a competitive edge.
Regulatory frameworks are evolving to keep up with AI advancements, and companies must ensure compliance with new laws and standards. Additionally, as the AI industry becomes more crowded, the competition will intensify, making it essential for companies to continuously innovate and differentiate their products and services.
Looking Ahead: The Future of ASX AI Stocks
The future of ASX AI stocks appears to be very promising, with AI technologies continuing to evolve and find applications across a wide range of industries. As businesses look for more ways to enhance their operations and address complex challenges, the demand for AI solutions is expected to rise.
The role of ASX-listed companies in this field will become increasingly important, as their innovative solutions help shape the future of industries and technologies. As AI capabilities advance, ASX AI stocks are well-positioned to benefit from the continued growth of this transformative sector.
0 notes
Text
Generative AI Market Size, Share, Scope, Analysis, Forecast, Regional Outlook and Industry Report 2032
TheGenerative AI Market Size was valued at USD 20.21 Billion in 2023 and is expected to reach USD 440 Billion by 2032 and grow at a CAGR of 41.31% over the forecast period 2024-2032.
Generative AI is rapidly transforming industries with its ability to create text, images, audio, and video content. Fueled by breakthroughs in machine learning and deep learning, this technology is reshaping productivity and creativity. From startups to tech giants, investments in generative AI are accelerating at an unprecedented pace.
Generative AI Market has become a central focus across sectors such as media, healthcare, marketing, and finance. Businesses are leveraging this technology to streamline operations, enhance customer experience, and unlock new revenue streams. With user-friendly tools and APIs now widely available, generative AI is no longer limited to tech-savvy developers—it's accessible to creators, marketers, and businesses of all sizes.
Get Sample Copy of This Report: https://www.snsinsider.com/sample-request/4490
Market Keyplayers:
Synthesia
IBM
Microsoft
Rephrase.ai
Genei AI Ltd
Google LLC
Adobe
Runway
Capgemini
Accenture
Mistral AI
Open AI
Trends Driving the Generative AI Market
AI-as-a-Service Models: Cloud-based platforms like OpenAI, Google Cloud, and AWS offer generative AI tools as plug-and-play services, making integration simpler for businesses.
Creative Automation: Generative AI is transforming content creation—from writing articles to generating code, music, and design prototypes—boosting productivity in creative industries.
Personalized Experiences: Brands are using generative AI to craft personalized emails, ads, and product recommendations at scale, increasing customer engagement.
Ethical AI and Regulation: As usage grows, concerns about misinformation, deepfakes, and data privacy are prompting governments and organizations to push for ethical AI practices and regulatory frameworks.
Enquiry of This Report: https://www.snsinsider.com/enquiry/4490
Market Segmentation:
BY PRODUCT TYPE
Software
Service
BY TECHNOLOGY
Variational Auto-encoders
Diffusion Networks
GANs
Transformers
BY APPLICATION
Computer Vision
Predictive Analysis
Content Generation
NLP
Robotics & Automation
Chatbots & Intelligent Virtual Assistants
Others
BY MODEL
Image & Video Generative Models
Chatbots & Intelligent Virtual Assistants
Large Language Models
Others
BY END USER
Media & Entertainment
BFSI
Automotive & Transportation
Gaming
IT & Telecommunication
Market Analysis: Key Insights
Sector Adoption: High adoption rates are seen in marketing & advertising, software development, healthcare diagnostics, and finance, where generative AI is improving efficiency and customer service.
Investment Surge: Venture capital and corporate investments are pouring into generative AI startups, with several billion-dollar valuations recorded in 2024 alone.
Talent Demand: The need for AI engineers, data scientists, and prompt designers has skyrocketed, reflecting a broader shift in required workforce skills.
Future Prospects
The future of the Generative AI Market is bright, with ongoing innovations paving the way for new use cases and business models. We can expect deeper integration of generative AI in enterprise workflows—from automated legal drafting and medical imaging analysis to real-time customer service and product prototyping.
As open-source models become more powerful and accessible, smaller companies will also compete on innovation. Multi-modal generative AI—where text, image, and video generation converge—will enable more seamless, immersive applications across industries. Additionally, developments in edge computing will allow AI models to run locally on devices, enhancing privacy and reducing latency.
In education, generative AI will support personalized learning, adaptive assessments, and content generation for diverse student needs. In entertainment, AI will play a key role in co-creating scripts, visuals, and even virtual actors. Cross-disciplinary applications like biotech and engineering design will benefit from simulation and testing powered by generative models.
Access Complete Report: https://www.snsinsider.com/reports/generative-ai-market-4490
Conclusion
The Generative AI Market is evolving at an extraordinary pace, offering transformative potential across industries. While challenges around ethical use and regulation remain, the technology’s ability to unlock creativity, speed up innovation, and reduce operational costs makes it a driving force in the digital economy.
As businesses continue to explore its full potential, generative AI is set to become an essential tool—not just for automation, but for imagination. Those who adapt early will gain a strong competitive edge in the age of intelligent creation.
About Us:
SNS Insider is one of the leading market research and consulting agencies that dominates the market research industry globally. Our company's aim is to give clients the knowledge they require in order to function in changing circumstances. In order to give you current, accurate market data, consumer insights, and opinions so that you can make decisions with confidence, we employ a variety of techniques, including surveys, video talks, and focus groups around the world.
Contact Us:
Jagney Dave - Vice President of Client Engagement
Phone: +1-315 636 4242 (US) | +44- 20 3290 5010 (UK)
0 notes
Text
Data Science with Generative AI Online Training: Generative AI Tools Every Data Scientist Should Know
The rise of data science with generative AI training has transformed how professionals solve problems, generate insights, and create innovative solutions. By leveraging cutting-edge tools, data scientists can automate processes, enhance creativity, and drive data-driven strategies. For those aspiring to excel in this field, enrolling in a data science with generative AI course is a game-changer, equipping learners with the skills to navigate complex AI landscapes. This article explores essential generative AI tools, their benefits, and actionable tips for mastering them through comprehensive data science with generative AI online training.

What is Data Science with Generative AI?
Data Science with Generative AI combines the principles of data science—data analysis, predictive modeling, and statistical computations—with the capabilities of generative AI. Generative AI enables machines to produce new content, such as images, text, and music, by learning from large datasets. Through data science with generative AI courses, learners gain expertise in integrating these powerful technologies to create innovative applications.
Importance of Generative AI in Data Science
Automation of Repetitive Tasks: Generative AI accelerates data cleaning, feature engineering, and visualization tasks.
Enhanced Creativity: From generating synthetic datasets to creating AI-driven designs, the scope is limitless.
Improved Decision-Making: Tools powered by generative AI enhance the accuracy of predictive analytics.
Cost Efficiency: Automating data workflows with generative AI reduces operational costs.
By enrolling in a data science with generative AI training program, professionals can harness these advantages while staying ahead in a competitive job market.
Key Generative AI Tools Every Data Scientist Should Know
GPT Models (Generative Pre-trained Transformers)
GPT models, like OpenAI’s GPT-4, are foundational for text generation, summarization, and translation. These models are widely used in chatbots, content creation, and natural language processing (NLP). Tips:
Use GPT tools for creating automated reports and summarizing datasets.
Experiment with fine-tuning GPT models for industry-specific applications.
DALL·E and Similar Image Generators
These tools create realistic images based on textual descriptions. They are invaluable in marketing, design, and data visualization. Tips:
Integrate these tools into projects requiring AI-driven infographics or visualizations.
Experiment with synthetic image generation for data augmentation in machine learning.
Deep Dream and Neural Style Transfer Tools
These tools enhance images or create artistic transformations. They are useful in fields like media, advertising, and creative analytics. Tips:
Apply these tools to improve visual storytelling in presentations.
Use them for prototyping designs in creative data projects.
AutoML (Automated Machine Learning) Platforms
AutoML platforms like Google AutoML simplify complex machine learning tasks, from model training to deployment. Tips:
Start with pre-built models to save time.
Leverage AutoML to democratize AI by enabling non-technical users to build effective models.
GANs (Generative Adversarial Networks)
GANs are pivotal in generating synthetic data for training machine learning models. Tips:
Use GANs for solving data scarcity problems by generating synthetic samples.
Explore GAN-based models for advanced projects, such as anomaly detection.
Benefits of Learning Generative AI Tools in a Course Setting
Structured Learning Path: A data science with generative AI course provides a well-organized curriculum to master generative AI tools.
Hands-on Practice: Courses often include practical projects to apply concepts.
Expert Guidance: Access to instructors ensures doubts are resolved efficiently.
Collaboration Opportunities: Working with peers enhances learning and broadens perspectives.
Tips for Excelling in Data Science with Generative AI Online Training
Stay Updated: Follow AI research papers, updates, and tools to stay ahead.
Practice Regularly: Implement concepts from the data science with generative AI training into real-world projects.
Focus on Applications: Understand the practical applications of each tool to bridge the gap between theory and practice.
Engage in Communities: Join forums and groups to learn from peers and industry experts.
Build a Portfolio: Showcase your skills through projects demonstrating your proficiency in generative AI.
Applications of Generative AI in Data Science
Text Analytics: Automated report generation, sentiment analysis, and customer feedback summaries.
Predictive Modeling: Accurate forecasting and risk analysis through synthetic data.
Creative Projects: AI-driven marketing campaigns and innovative visual designs.
Healthcare Analytics: Simulating patient outcomes or generating synthetic medical data for analysis.
Finance: Fraud detection using GANs and automated report generation with GPT models.
Why Choose a Data Science with Generative AI Course?
A data science with generative AI course bridges the gap between theory and industry application. From understanding AI fundamentals to mastering advanced tools, these courses empower learners to navigate complex challenges efficiently. Through structured data science with generative AI training, professionals gain access to real-world scenarios, making them industry-ready.
Conclusion
Mastering data science with generative AI tools is no longer optional—it’s a necessity for professionals aiming to excel in data-driven industries. Enrolling in a data science with generative AI course equips learners with essential skills, ensuring they remain competitive in an ever-evolving field. By leveraging tools like GPT, DALL·E, and GANs, data scientists can drive innovation, enhance decision-making, and create impactful solutions. With a commitment to continuous learning and practice, achieving success in this transformative domain is well within reach.
Visualpath Advance your career with Data Science with Generative Ai Course Hyderabad. Gain hands-on training, real-world skills, and certification. Enroll today for the best Data Science Course. We provide to individuals globally in the USA, UK, etc.
Call on: +91 9989971070
Course Covered:
Data Science, Programming Skills, Statistics and Mathematics, Data Analysis, Data Visualization, Machine Learning, Big Data Handling, SQL, Deep Learning and AI
WhatsApp: https://www.whatsapp.com/catalog/919989971070/
Blog link: https://visualpathblogs.com/
Visit us: https://www.visualpath.in/online-data-science-with-generative-ai-course.html
#Data Science Course#Data Science Course In Hyderabad#Data Science Training In Hyderabad#Data Science With Generative Ai Course#Data Science Institutes In Hyderabad#Data Science With Generative Ai#Data Science With Generative Ai Online Training#Data Science With Generative Ai Course Hyderabad#Data Science With Generative Ai Training
0 notes
Text
Small Language Model Market Size, Share, Analysis, Forecast, Growth 2032: SaaS Licensing Models Redefine Profit Margins for Developers
The Small Language Model Market was valued at USD 7.9 billion in 2023 and is expected to reach USD 29.64 billion by 2032, growing at a CAGR of 15.86% from 2024-2032.
The Small Language Model (SLM) market is witnessing a notable surge in adoption as businesses and developers increasingly seek efficient, cost-effective, and scalable AI solutions. With rising demand for on-device processing, reduced latency, and improved data privacy, SLMs are becoming a compelling alternative to large-scale models. These models are particularly well-suited for edge applications, customer service automation, IoT integration, and low-resource environments. The evolving digital landscape, coupled with rapid innovation in AI frameworks, is driving stakeholders across industries to explore the potential of these compact yet powerful models.
Small Language Model Market toward decentralization and embedded intelligence has fueled growth in the Small Language Model market. Enterprises are leveraging SLMs to deliver real-time insights, personalized interactions, and seamless user experiences without relying on cloud-based infrastructures. Startups and SMEs are also playing a critical role in accelerating the development and deployment of lightweight language models by focusing on niche solutions across verticals like healthcare, finance, education, and mobile applications. This evolution in AI strategy underscores the growing belief in the versatility and utility of SLMs beyond conventional natural language processing.
Get Sample Copy of This Report: https://www.snsinsider.com/sample-request/5947
Market Keyplayers:
Meta AI (LLaMA, BlenderBot)
Microsoft (Azure Cognitive Services, Turing NLG)
Salesforce AI (Einstein Language, Salesforce NLP)
Alibaba (AliMe, PAI NLP)
Mosaic ML (MosaicML Platform, MosaicML Optimizer)
Technology Innovation Institute (TII) (Falcon, GPT-3)
Hugging Face (Transformers, Datasets)
OpenAI (GPT-4, Codex)
Google DeepMind (BERT, Gemini)
Amazon Web Services (AWS) (Amazon Comprehend, Amazon SageMaker)
IBM Watson (Watson NLP, Watson Assistant)
Baidu (Ernie, Baidu Apollo)
Anthropic (Claude, Anthropic AI Safety)
Cohere (Cohere Command, Cohere Language Models)
xAI (founded by Elon Musk) (XAI GPT, XAI Chatbot)
Grammarly (Grammarly Writing Assistant, Grammarly Business)
Jasper AI (Jasper Chat, Jasper Art)
Replit (Replit AI, Ghostwriter)
Neudesic (Neudesic AI, Neudesic LLMs)
EleutherAI (GPT-Neo, GPT-J)
Market Analysis The competitive landscape is becoming increasingly dynamic with the entry of specialized AI startups and advancements from established tech giants. Investment in R&D for model compression, transfer learning, and energy-efficient deployment strategies is intensifying. Regional markets in Asia-Pacific, North America, and Europe are showcasing differentiated adoption patterns influenced by infrastructure readiness, regulatory frameworks, and digital maturity. The convergence of AI ethics, open-source innovation, and strategic collaborations is also shaping the market’s trajectory.
Market Trends
Increasing use of SLMs in embedded systems and mobile applications
Rise in open-source SLM platforms for academic and commercial use
Growing emphasis on responsible AI and explainability in smaller models
Integration of SLMs in real-time decision-making systems
Shift from cloud-based to on-device NLP processing
Surge in lightweight NLP tools for underserved languages
Expansion of low-code/no-code platforms powered by SLMs
Market Scope The Small Language Model market encompasses a wide range of industries, including healthcare, finance, e-commerce, education, and telecommunications. Its applicability spans chatbots, voice assistants, sentiment analysis tools, document summarization engines, and language translation applications. The compact nature of SLMs makes them ideal for deployment in smart devices, autonomous systems, and remote operations where bandwidth and computing power are limited. The scope further extends to government and defense sectors seeking secure, private AI operations without internet dependency.
Market Forecast The Small Language Model market is poised for accelerated growth over the coming years, driven by technological innovations and increasing business adoption of edge AI. Key players are anticipated to focus on refining model architectures, enhancing multilingual support, and optimizing inference speeds. With education and accessibility becoming primary themes, future models will likely emphasize low-resource adaptability, real-time learning, and seamless integration into diverse ecosystems. As demand for AI democratization grows, the market is expected to see a rise in community-driven frameworks and user-friendly development environments that will broaden participation and innovation.
Access Complete Report: https://www.snsinsider.com/reports/small-language-model-market-5947
Conclusion The future of artificial intelligence isn't only large and centralized—it’s also small, strategic, and scalable. The Small Language Model market is fast becoming a catalyst for inclusive, efficient, and adaptive AI solutions that meet the real-world needs of businesses and users alike. As this market matures, it promises to empower a new wave of intelligent applications that redefine the limits of what compact models can achieve.
About Us:
SNS Insider is one of the leading market research and consulting agencies that dominates the market research industry globally. Our company's aim is to give clients the knowledge they require in order to function in changing circumstances. In order to give you current, accurate market data, consumer insights, and opinions so that you can make decisions with confidence, we employ a variety of techniques, including surveys, video talks, and focus groups around the world.
Contact Us:
Jagney Dave - Vice President of Client Engagement
Phone: +1-315 636 4242 (US) | +44- 20 3290 5010 (UK)
#Small Language Model Market#Small Language Model Market Scope#Small Language Model Market Growth#Small Language Model Market Trends
0 notes
Text
Best Institute For Learning Data Science in Mumbai
Data science is more than just a buzzword; it's a transformative field that’s reshaping industries and redefining careers. Whether you're an aspiring professional or someone looking to make a career shift, understanding data science can open doors you never knew existed. With its growing importance in today's data-driven world, the demand for skilled data scientists continues to rise. Mumbai, often dubbed the financial capital of India, is teeming with opportunities for those equipped with data science skills. However, diving into this dynamic field requires the right guidance and resources. This is where choosing the best institute for learning Data Science becomes crucial. Join us as we explore what data science entails, why it's an attractive career path in 2023, and how Digi Sequel stands out as the premier choice for education in Mumbai's bustling landscape of technology and innovation.
What is Data Science?
Data science is the art and science of extracting meaningful insights from raw data. It combines statistics, mathematics, programming, and domain expertise to analyze complex information sets. At its core, data science involves collecting large volumes of data from various sources. This can include everything from social media interactions to sales records or even sensor readings in smart devices. Once gathered, this data goes through a rigorous process of cleaning and transformation. Analysts use advanced algorithms and statistical techniques to uncover patterns that might not be immediately apparent. The ultimate goal is to inform decision-making processes across different sectors. Whether it’s predicting customer behavior or optimizing business operations, data science empowers organizations with actionable intelligence in real time.
Data Science Tools
Data science tools are crucial for transforming raw data into meaningful insights. They facilitate the entire data analysis process, from collection to visualization. One of the most popular programming languages is Python. Its libraries, like Pandas and NumPy, make data manipulation straightforward. R is another powerful language favored by statisticians for its statistical computing capabilities. On the analytics side, Tableau and Power BI stand out. These tools allow users to create dynamic dashboards that present complex data visually. Moreover, machine learning libraries such as TensorFlow and Scikit-learn enable predictive modeling and deep learning applications. For big data processing, Apache Hadoop and Spark provide robust frameworks to handle vast datasets efficiently. Understanding these tools can significantly enhance your problem-solving abilities in real-world situations.
Why To Choose Data Science As A Career In 2023?
Data science is at the forefront of technological innovation. Companies across industries are increasingly relying on data-driven decisions. This growing demand makes it an attractive career choice for 2023. The potential for high earnings is significant in this field. With skills in machine learning, analytics, and programming, professionals can command competitive salaries. The financial rewards can be a strong motivator. Moreover, data science offers versatility. You can work in finance, healthcare, marketing, or even sports analytics. This diversity allows you to find a niche that excites you. Continuous learning is another appealing aspect of this career path. The landscape evolves rapidly with new tools and techniques emerging constantly. For those who thrive on challenges and want to stay ahead of the curve, data science presents endless opportunities for growth. Making impactful decisions based on real insights feels rewarding. Your work could drive fundamental changes within organizations and influence entire industries.
What Is The Future Scope of Data Science in 2024
The future scope of data science in 2024 appears promising. As businesses increasingly rely on data-driven decisions, the demand for skilled professionals continues to grow. Emerging technologies like artificial intelligence and machine learning will further enhance data analytics capabilities. This integration opens up new avenues for innovation across various sectors, including healthcare, finance, and retail. Moreover, as organizations prioritize ethical AI practices, there's a rising need for experts who can navigate these challenges. Data scientists will be essential in ensuring transparency and fairness within algorithms. Additionally, advancements in big data tools will enable more efficient processing of vast datasets. This evolution requires continuous skill upgrades among practitioners to stay relevant in the field. With remote work becoming standard practice post-pandemic, opportunities are expanding globally. Data scientists can collaborate with teams worldwide without geographical constraints.
How to get a job after learning data science skills
Once you’ve honed your data science skills, the next step is landing that dream job. Begin by building a strong portfolio showcasing your projects. Include diverse work—data analysis, machine learning models, and visualizations. Networking plays a crucial role too. Attend industry meetups or online forums to connect with professionals in the field. LinkedIn can help you expand your reach significantly. Consider internships as well. They offer invaluable real-world experience and often lead to full-time roles. Tailor your resume for each position you apply for, highlighting relevant skills and experiences directly related to data science. Prepare for interviews thoroughly. Practice common questions while also being ready to discuss specific projects from your portfolio in depth. Showing enthusiasm about the field will set you apart from other candidates.
Digi Sequel - Best Institute for Data Science in Mumbai
Digi Sequel stands out as the best institute for data science course in Mumbai, offering a comprehensive curriculum tailored for aspiring data scientists. Their program covers essential concepts and practical applications, ensuring students are well-prepared for real-world challenges. What sets Digi Sequel apart is its industry-experienced faculty. Instructors provide insights that go beyond textbooks, sharing their firsthand knowledge of current trends and technologies in data science. The hands-on approach at Digi Sequel fosters an engaging learning environment. Students work on live projects using cutting-edge tools. This not only enhances skills but also builds confidence to tackle complex problems. Moreover, the institute's strong placement support system connects graduates with top companies seeking skilled professionals. With a focus on career growth and development, Digi Sequel is dedicated to helping students achieve their aspirations in the dynamic field of data science.
Why Choose Digi Sequel?
Choosing the right institute is crucial for mastering data science. Digi Sequel stands out as the best institute for data science in Mumbai due to its comprehensive curriculum and hands-on approach. The faculty comprises industry experts who bring real-world experience into the classroom, enhancing your learning journey. Digi Sequel offers a blend of theoretical knowledge and practical skills using the latest tools in data science, such as Python, R, SQL, and machine learning frameworks. What sets this institute apart is its commitment to personalized mentorship. Each student receives guidance tailored to their individual career aspirations. Moreover, Digi Sequel provides access to an extensive network of industry connections. This can be invaluable when seeking internships or job placements after course completion. Regular workshops with guest speakers from top tech firms further enrich the learning environment. The flexible scheduling options make it convenient for working professionals interested in upskilling without disrupting their careers. With a focus on project-based learning, students not only understand concepts but also apply them effectively through real-life scenarios. Choosing Digi Sequel equips you with the necessary skills and confidence to thrive in today’s competitive job market while aligning your training with future industry demands.
0 notes
Text
AI Agent Development: A Complete Guide to Building Smart, Autonomous Systems in 2025
Artificial Intelligence (AI) has undergone an extraordinary transformation in recent years, and 2025 is shaping up to be a defining year for AI agent development. The rise of smart, autonomous systems is no longer confined to research labs or science fiction — it's happening in real-world businesses, homes, and even your smartphone.
In this guide, we’ll walk you through everything you need to know about AI Agent Development in 2025 — what AI agents are, how they’re built, their capabilities, the tools you need, and why your business should consider adopting them today.
What Are AI Agents?
AI agents are software entities that perceive their environment, reason over data, and take autonomous actions to achieve specific goals. These agents can range from simple chatbots to advanced multi-agent systems coordinating supply chains, running simulations, or managing financial portfolios.
In 2025, AI agents are powered by large language models (LLMs), multi-modal inputs, agentic memory, and real-time decision-making, making them far more intelligent and adaptive than their predecessors.
Key Components of a Smart AI Agent
To build a robust AI agent, the following components are essential:
1. Perception Layer
This layer enables the agent to gather data from various sources — text, voice, images, sensors, or APIs.
NLP for understanding commands
Computer vision for visual data
Voice recognition for spoken inputs
2. Cognitive Core (Reasoning Engine)
The brain of the agent where LLMs like GPT-4, Claude, or custom-trained models are used to:
Interpret data
Plan tasks
Generate responses
Make decisions
3. Memory and Context
Modern AI agents need to remember past actions, preferences, and interactions to offer continuity.
Vector databases
Long-term memory graphs
Episodic and semantic memory layers
4. Action Layer
Once decisions are made, the agent must act. This could be sending an email, triggering workflows, updating databases, or even controlling hardware.
5. Autonomy Layer
This defines the level of independence. Agents can be:
Reactive: Respond to stimuli
Proactive: Take initiative based on context
Collaborative: Work with other agents or humans
Use Cases of AI Agents in 2025
From automating tasks to delivering personalized user experiences, here’s where AI agents are creating impact:
1. Customer Support
AI agents act as 24/7 intelligent service reps that resolve queries, escalate issues, and learn from every interaction.
2. Sales & Marketing
Agents autonomously nurture leads, run A/B tests, and generate tailored outreach campaigns.
3. Healthcare
Smart agents monitor patient vitals, provide virtual consultations, and ensure timely medication reminders.
4. Finance & Trading
Autonomous agents perform real-time trading, risk analysis, and fraud detection without human intervention.
5. Enterprise Operations
Internal copilots assist employees in booking meetings, generating reports, and automating workflows.
Step-by-Step Process to Build an AI Agent in 2025
Step 1: Define Purpose and Scope
Identify the goals your agent must accomplish. This defines the data it needs, actions it should take, and performance metrics.
Step 2: Choose the Right Model
Leverage:
GPT-4 Turbo or Claude for text-based agents
Gemini or multimodal models for agents requiring image, video, or audio processing
Step 3: Design the Agent Architecture
Include layers for:
Input (API, voice, etc.)
LLM reasoning
External tool integration
Feedback loop and memory
Step 4: Train with Domain-Specific Knowledge
Integrate private datasets, knowledge bases, and policies relevant to your industry.
Step 5: Integrate with APIs and Tools
Use plugins or tools like LangChain, AutoGen, CrewAI, and RAG pipelines to connect agents with real-world applications and knowledge.
Step 6: Test and Simulate
Simulate environments where your agent will operate. Test how it handles corner cases, errors, and long-term memory retention.
Step 7: Deploy and Monitor
Run your agent in production, track KPIs, gather user feedback, and fine-tune the agent continuously.
Top Tools and Frameworks for AI Agent Development in 2025
LangChain – Chain multiple LLM calls and actions
AutoGen by Microsoft – For multi-agent collaboration
CrewAI – Team-based autonomous agent frameworks
OpenAgents – Prebuilt agents for productivity
Vector Databases – Pinecone, Weaviate, Chroma for long-term memory
LLMs – OpenAI, Anthropic, Mistral, Google Gemini
RAG Pipelines – Retrieval-Augmented Generation for knowledge integration
Challenges in Building AI Agents
Even with all this progress, there are hurdles to be aware of:
Hallucination: Agents may generate inaccurate information.
Context loss: Long conversations may lose relevancy without strong memory.
Security: Agents with action privileges must be protected from misuse.
Ethical boundaries: Agents must be aligned with company values and legal standards.
The Future of AI Agents: What’s Coming Next?
2025 marks a turning point where AI agents move from experimental to mission-critical systems. Expect to see:
Personalized AI Assistants for every employee
Decentralized Agent Networks (Autonomous DAOs)
AI Agents with Emotional Intelligence
Cross-agent Collaboration in real-time enterprise ecosystems
Final Thoughts
AI agent development in 2025 isn’t just about automating tasks — it’s about designing intelligent entities that can think, act, and grow autonomously in dynamic environments. As tools mature and real-time data becomes more accessible, your organization can harness AI agents to unlock unprecedented productivity and innovation.
Whether you’re building an internal operations copilot, a trading agent, or a personalized shopping assistant, the key lies in choosing the right architecture, grounding the agent in reliable data, and ensuring it evolves with your needs.
1 note
·
View note
Text
Data Science VS Data Analytics: Which is right for you?

Data Science and Data Analytics are interconnected yet distinct fields that play crucial roles in the modern data-driven landscape. Understanding their differences, similarities, and career implications can help individuals choose the right path based on their skills and interests.
Overview of Data Science and Data Analytics
Data Science
Data Science is a multidisciplinary field that employs scientific methods, processes, algorithms, and systems to extract insights from structured and unstructured data. It encompasses a broad range of activities, including data collection, cleaning, analysis, and modeling. Data Science relies heavily on advanced statistical techniques, machine learning, and artificial intelligence to uncover patterns, make predictions, and drive decision-making processes. The primary goal is to derive actionable insights that can inform strategic business decisions and foster innovation.
Key Characteristics of Data Science:
Scope: Encompasses various tasks from data preparation to building complex predictive models.
Techniques: Utilizes advanced statistical modeling, machine learning algorithms, and data mining.
Data Volume: Often deals with large, complex datasets, including unstructured data types like text and images.
Objective: Aim to discover hidden patterns and develop predictive models to solve complex problems.
Skills Required: Strong foundation in mathematics, statistics, programming (e.g., Python, R), and domain expertise.
Tools Used: Programming languages (Python, R), big data technologies (Hadoop, Spark), and machine learning libraries (TensorFlow, sci-kit-learn) are commonly employed.
Data Analytics
Data Analytics, on the other hand, focuses on examining datasets to draw conclusions and insights. It is more concerned with analyzing historical data to inform business decisions and optimize operations. Data Analysts typically use statistical tools and visualization techniques to interpret data, identify trends and present findings in a comprehensible manner. Their work is often more straightforward compared to that of Data Scientists, as they primarily deal with well-defined datasets and specific business questions.
Key Characteristics of Data Analytics:
Scope: Primarily focuses on analyzing existing data to answer specific business questions.
Techniques: Employ statistical analysis, descriptive statistics, and data visualization methods.
Data Volume: Generally works with structured data and smaller datasets compared to Data Science.
Objective: Aim to provide actionable insights for business improvements based on historical data.
Skills Required: Strong analytical skills, proficiency in data visualization tools (e.g., Tableau, Power BI), and knowledge of statistical software (e.g., SQL, Excel).
Tools Used: Commonly utilizes Excel, SQL, Tableau, and Power BI for data manipulation and visualization.
Career Paths and Job Roles
Data Scientist
Data Scientists are expected to have a more advanced skill set, often requiring a master's degree or higher in a related field. Their roles involve designing and constructing new processes for data modeling and production, employing machine learning techniques, and developing algorithms to predict future trends. They typically work on projects that involve building recommendation systems, fraud detection models, and natural language processing applications.
Data Analyst
Data Analysts usually require a bachelor's degree and focus on interpreting existing data to help organizations make informed decisions. They create visual representations of data, prepare reports, and communicate findings to stakeholders. Their roles can vary widely across industries, and they may hold titles such as business analyst, market research analyst, or financial analyst.
Choosing the Right Path
When deciding between a career in Data Science and Data Analytics, consider the following factors:
Interest in Technical Skills: If you enjoy programming, machine learning, and complex problem-solving, Data Science may be the right fit. Conversely, if you prefer working with data to derive insights and communicate findings, Data Analytics could be more suitable.
Educational Background: Data Science typically requires a stronger technical and mathematical background, often necessitating advanced degrees. Data Analytics roles may be more accessible with a bachelor's degree and relevant experience.
Career Goals: Consider your long-term career aspirations. Data Scientists often have higher earning potential due to the complexity of their work and the skills required. However, Data Analysts also play a vital role in organizations and can advance to senior positions with experience and expertise
Data Science VS Data Analytics Salary
Salary Overview
Data Analyst Salaries:
In the United States, the average salary for a Data Analyst is approximately $70,000 annually. Entry-level positions may start around $45,000, while senior roles can reach up to $120,000 per year.
In India, a Data Analyst typically earns around 6 lakhs per annum, with senior analysts making about 10 lakhs per annum.
Data Scientist Salaries:
Data Scientists command higher salaries, averaging around $120,000 annually in the U.S. Senior Data Scientists can earn upwards of $145,000. According to some sources, the average can also be cited as $100,000 to $114,141 depending on experience and specific job roles.
In India, the average salary for a Data Scientist is about 10.5 lakhs per annum, with senior positions earning around 20.5 lakhs per annum.
Comparison of Salaries
Data Analysts earn significantly less than Data Scientists due to the latter's advanced skill requirements, including expertise in machine learning and statistical modeling.
Salary Growth Potential: Both fields offer promising career paths, but Data Scientists generally have higher earning potential due to the complexity of their work and the demand for advanced analytical skills in the job market.
Difference Between Data Science and Data Analytics with Examples
Examples:
1. Data Science in Healthcare:
Analyzing medical records, imaging data, and genomic sequences to develop personalized treatment plans.
Using machine learning algorithms to predict disease outbreaks and optimize resource allocation.
2. Data Analytics in Retail:
Analyzing customer purchasing patterns to identify the most profitable product combinations.
Optimizing inventory levels based on historical sales data and forecasting demand.
Segmenting customers based on their behavior and preferences to personalize marketing campaigns.
3. Data Science in Finance:
Developing predictive models for stock price movements and portfolio optimization.
Detecting financial fraud using machine learning techniques.
Assessing credit risk and making lending decisions based on customer data.
4. Data Analytics in Supply Chain Management:
Analyzing supplier performance data to identify potential risks and optimize sourcing strategies.
Visualizing supply chain data to identify bottlenecks and inefficiencies.
Forecasting demand and optimizing inventory levels to minimize stockouts and excess inventory.
In summary, many colleges teach both Data Science and Data Analytics but Arya College of Engineering & I.T. is the best Engineering College in Jaipur that involves working with data, Data Science has a broader scope, focusing on solving complex problems using advanced techniques like machine learning and artificial intelligence, while Data Analytics is more focused on answering specific business questions using structured data and providing actionable insights to drive decision-making. both Data Science and Data Analytics offer valuable career opportunities, each with its unique focus, skill requirements, and job roles. Assessing your interests, educational background, and career goals will help guide your decision on your path.
Source: Click Here
#best btech college in jaipur#top engineering college in jaipur#best btech college in rajasthan#best engineering college in jaipur#best engineering college in rajasthan#best private engineering college in jaipur
0 notes
Text
LiDAR Market: Current Trends, Business Opportunities, Challenges & Industry Analysis by 2030
LiDAR Industry Overview
The global LiDAR market size was valued at USD 1.81 billion in 2021 and is expected to expand at a compound annual growth rate (CAGR) of 9.8% from 2022 to 2030.
The technological advancements in spatial resolution of LiDAR-based digital terrain models are providing better accuracy in applications such as change detection on hillsides, water runoff for agriculture or mining sites, and inland waterways. The growth of the market is attributed to the rising automation in LiDAR to reduce human efforts and increase efficiency.
Gather more insights about the market drivers, restrains and growth of the LiDAR Market
The rising demand for 3D imagery in application areas such as military & defense, topographical surveys, civil engineering, and corridor mapping is expected to drive the market growth over the forecast period. Textured 3D imagery is applied in applications such as 3D mapping, city planning, and corridor mapping. The installation of advanced safety features is increasing exponentially. Moreover, in countries such as the U.S., self-driving cars are approved by the government on the road; these vehicles are integrated with LiDAR for GPS and navigation applications.
LiDAR is also used in monitoring weather conditions and environmental monitoring. For instance, the Canadian environment monitoring stations utilize LiDAR technology to monitor tropospheric pollution. LiDAR stations are crucial in monitoring the ash from volcanic eruptions that can interfere with air travel. The technology also finds its application in mapping the oil sand monitoring system. These multiple applications of LiDAR technology owing to its enhanced accuracy are expected to propel the growth of the market over the forecast period.
The lack of educated customers is considered a major restraint for the growth of the market. Ascertaining the scope of customer education is essential to reduce the costs of implementing and designing such customer awareness and education programs. Customer education and awareness are crucial for improving the capacity of the people to address development and environmental issues and for stimulating sustainable development.
Browse through Grand View Research's Next Generation Technologies Industry Research Reports.
• The global drone sensor market size was estimated at USD 1.42 billion in 2023 and is expected to grow at a CAGR of 10.0% from 2024 to 2030.
• The global large language model powered tools market size was estimated at USD 1.43 billion in 2023 and is projected to grow at a CAGR of 48.8% from 2024 to 2030.
Key Companies & Market Share Insights
Various competitors in the market offer innovative solutions, which help consumers address changing technologies, business practices, and security needs. Europe comprises notable players who offer advanced solutions to meet varied application requirements. The key players in the market face intense competition to dominate the market, enabling market consolidation through strategic initiatives such as mergers & acquisitions. Industry participants have entered into a strategic partnership with suppliers and distributors to strengthen their product portfolio and geographical presence. Some prominent players in the global LiDAR market include:
Faro Technologies, Inc.
Leica Geosystem Holdings AG
Teledyne Optech Incorporated (A part of Teledyne Technologies)
Trimble Navigation Limited
RIEGL USA, Inc.
Quantum Spatial, Inc.
Velodyne LiDAR, Inc.
Sick AG
YellowScan
GeoDigital
Segments Covered in the Report
This report forecasts revenue growth at global, regional, and country levels and provides an analysis of the latest industry trends in each of the sub-segments from 2017 to 2030. For this study, Grand View Research has segmented the global LiDAR market report based on product type, application, component, and region:
Product Type Outlook (Revenue, USD Million, 2017 - 2030)
Airborne
Terrestrial
Mobile & UAV
Application Outlook (Revenue, USD Million, 2017 - 2030)
Corridor Mapping
Engineering
Environment
Exploration
Advanced Driver Assistance Systems (ADAS)
Others
Component Outlook (Revenue, USD Million, 2017 - 2030)
GPS
Navigation (IMU)
Laser Scanners
Others
Regional Outlook (Revenue, USD Million, 2017 - 2030)
North America
US
Canada
Mexico
Europe
Germany
UK
France
Asia Pacific
China
India
Japan
South America
Brazil
Middle East & Africa
Order a free sample PDF of the LiDAR Market Intelligence Study, published by Grand View Research.
0 notes
Text
Transforming Business with AI: Spritle Software’s Approach to Innovation
In today’s rapidly advancing digital era, artificial intelligence (AI) has become a crucial driver of technological progress. AI is revolutionizing industries by optimizing processes, enhancing customer experiences, and enabling smarter decision-making. For businesses aiming to remain competitive, collaborating with an experienced AI development company is vital. Spritle Software, a frontrunner in AI development, offers a diverse range of AI-driven solutions tailored to meet the specific needs of various industries.
Understanding AI Development
AI development refers to the process of creating intelligent systems that can be integrated into existing business operations and software applications. This involves designing algorithms that simulate human thought processes, allowing machines to perform tasks like decision-making, problem-solving, language comprehension, and visual recognition. The scope of AI development includes technologies such as machine learning, natural language processing (NLP), deep learning, and computer vision, all of which contribute to automating tasks and driving business growth.
The Growing Necessity of AI Solutions
In the competitive landscape of today, AI solutions are becoming essential for businesses that want to stay ahead. Here’s why organizations are increasingly adopting AI:
Boosted Productivity: AI systems can execute tasks more quickly and accurately than humans, reducing mistakes and saving valuable time.
Cost Reduction: By automating repetitive tasks, AI helps cut operational costs, enabling employees to focus on more strategic responsibilities.
Enhanced Customer Interaction: AI chatbots, virtual assistants, and personalized recommendation engines improve customer interactions, leading to greater satisfaction and loyalty.
Informed Decision-Making: AI can process large volumes of data to identify patterns and provide actionable insights, empowering businesses to make well-informed decisions.
Flexible and Scalable Solutions: AI technologies are adaptable and scalable, allowing businesses to grow and evolve with changing market demands.
Incorporating AI into business strategies is no longer a choice but a strategic necessity for maintaining a competitive edge.
Spritle Software: Leading the Charge in AI Development
As an innovative tech company, Spritle Software offers a wide array of AI development services designed to help businesses fully exploit the potential of AI. With profound expertise in software development and a commitment to AI innovation, Spritle Software is well-equipped to deliver tailored AI solutions that align with specific business objectives.
Why Spritle Software stands out among AI development providers:
1. Comprehensive AI Expertise
Spritle Software offers a wide range of AI services, from developing machine learning models to creating advanced chatbots and data analytics tools. Whether your goal is to optimize internal processes or enhance customer engagement, Spritle’s AI experts can develop solutions that align with your business objectives.
2. End-to-End AI Development
Spritle Software manages the entire AI development process, from initial concept to final deployment. Their team works closely with clients to understand their challenges, identify opportunities, and build AI-powered applications that deliver concrete results. With seamless integration and ongoing support, Spritle ensures that AI solutions remain effective and relevant.
3. Custom-Tailored AI Solutions
Understanding that each business has its own unique challenges, Spritle Software provides customized AI solutions tailored to meet the specific needs of different industries. Whether it’s healthcare, retail, finance, or logistics, Spritle’s AI systems are designed to address the distinct demands of each sector.
4. State-of-the-Art AI Chatbots
AI chatbots are transforming customer service, and Spritle Software is at the forefront of this evolution. With deep expertise in natural language processing (NLP), Spritle creates intelligent chatbots that interact with users in a conversational and intuitive manner. These chatbots handle inquiries, process transactions, and offer personalized recommendations, significantly reducing the burden on human customer support teams.
5. Advanced Data Analytics
Spritle Software’s AI services include sophisticated data analytics, enabling businesses to extract valuable insights from large datasets. Their AI systems can detect trends and patterns, providing companies with the data they need to make strategic, data-driven decisions. From predictive modeling to trend analysis, Spritle helps organizations leverage AI to gain a competitive edge.
6. Commitment to Innovation
Spritle Software’s success as an AI development company is driven by its commitment to continuous innovation. The team stays on top of the latest advancements in AI, ensuring that clients benefit from the most cutting-edge technologies. By combining advanced tools with deep industry knowledge, Spritle delivers AI solutions that not only meet current needs but also anticipate future challenges.
Real-World Applications: Spritle Software’s AI Impact Across Industries
AI is having a transformative impact across various industries, with businesses increasingly adopting AI-driven solutions to enhance their operations. Here’s a glimpse into how Spritle Software’s AI development services are making a difference:
1. Healthcare
AI is revolutionizing healthcare by improving diagnostic accuracy, streamlining patient care, and enabling personalized treatment plans. Spritle Software supports healthcare providers in developing AI systems that analyze medical data, detect anomalies, and enhance patient outcomes. From AI models for medical imaging to predictive analytics for patient management, Spritle is driving healthcare innovation.
2. E-commerce
In the e-commerce sector, AI is key to creating personalized shopping experiences, optimizing inventory management, and improving customer service. Spritle Software’s AI chatbots empower e-commerce platforms to engage customers with real-time assistance, product recommendations, and faster issue resolution, leading to higher conversion rates and increased customer loyalty.
3. Finance
The finance industry is harnessing AI to improve fraud detection, automate trading, and offer personalized financial advice. Spritle Software assists financial institutions in deploying AI models for risk management, fraud prevention, and customer engagement, enabling them to operate more efficiently and securely.
4. Retail
AI is transforming retail by helping businesses predict demand, optimize supply chains, and provide personalized customer experiences. Spritle Software supports retail companies in implementing AI solutions that enhance customer satisfaction and streamline operations.
Why Spritle Software is the Ideal AI Development Partner
Selecting the right AI development partner is essential for the success of your AI projects. Here’s why Spritle Software is the perfect choice:
Expertise and Experience: Spritle Software’s team of AI professionals brings deep expertise across various AI technologies, ensuring precise and effective project delivery.
Client-Focused Approach: Spritle works closely with clients to ensure that each AI solution is tailored to their specific needs and business goals.
Proven Success: With a strong track record of successful AI projects across multiple industries, Spritle Software has earned a reputation for delivering high-quality AI solutions that achieve measurable results.
Innovation-Driven: As a company focused on AI, Spritle Software is committed to continuous innovation, ensuring that clients stay ahead of technological trends and market demands.
Conclusion: Propel Your Business into the Future with AI
Artificial intelligence is more than just a technological advancement—it’s the future of business. By partnering with a leading AI development firm like Spritle Software, businesses can unlock new opportunities, increase efficiency, and deliver superior customer experiences. Whether your goal is to automate processes, develop intelligent applications, or gain valuable insights from data, Spritle’s AI development services are designed to help you succeed.
In a fast-paced technological world, staying ahead means embracing AI. With Spritle Software as your trusted AI partner, you can confidently navigate the complexities of AI and lead your industry into the future.
0 notes
Text
AI in Market Research: How It Is Disrupting Our Industry
Artificial Intelligence (AI) is rapidly transforming industries, and market research is no exception. From automating tedious processes to uncovering deep consumer insights, AI is revolutionizing the way researchers gather, analyze, and interpret data. In the digital age, market research has become a vital tool for businesses seeking to understand their audience, competitors, and market trends. AI’s integration into market research is not just enhancing efficiency but also enabling companies to make more accurate, data-driven decisions.
In this comprehensive blog, we’ll explore the impact of AI in market research, how it is disrupting traditional methodologies, and the future of this evolving landscape.
What Is AI in Market Research?
Before delving into how AI is transforming market research, it’s essential to define what we mean by AI in this context. AI refers to the simulation of human intelligence in machines that are programmed to think, learn, and adapt. In market research, AI takes the form of tools and technologies designed to streamline processes like data collection, analysis, interpretation, and reporting.
AI in market research involves a variety of techniques such as natural language processing (NLP), machine learning (ML), and predictive analytics. These technologies help researchers gain deeper insights, identify trends, and predict future behaviors with greater accuracy than traditional methods.
The Traditional Market Research Model
For decades, market research has relied on manual processes such as surveys, focus groups, and in-depth interviews. These methods are effective but can be time-consuming, costly, and prone to human error. Traditional research methods often involve months of gathering data, analyzing it, and presenting the findings—by which time, the market dynamics may have already shifted.
Let’s break down some of the limitations of traditional market research methods:
Time-consuming: Data collection and analysis take time, delaying actionable insights.
Expensive: Large-scale surveys, focus groups, and interviews require significant financial resources.
Human error: Manual data entry, coding, and analysis can lead to errors and bias.
Limited scope: Traditional methods may struggle to handle the large datasets that modern businesses demand.
How AI Is Disrupting the Market Research Industry
AI is addressing the challenges posed by traditional market research by automating various tasks and introducing smarter, faster, and more reliable ways to gather and analyze data. Below are the key ways AI is disrupting the industry:
1. Automation of Data Collection
One of the biggest advantages AI offers is automating the data collection process. Traditional methods of gathering data—such as sending out surveys or conducting interviews—are slow and resource-intensive. AI-powered chatbots and virtual assistants are now being used to collect real-time feedback from customers in a seamless manner.
For example, AI chatbots can conduct customer satisfaction surveys or gather consumer insights by engaging in natural language conversations. They can handle multiple conversations simultaneously, making them far more efficient than traditional methods. Additionally, AI systems can pull data from various digital platforms, social media, and online forums, which would have been nearly impossible for manual researchers to comb through.
2. Advanced Data Analysis Using Machine Learning
AI-powered data analysis is revolutionizing how market research companies handle large datasets. Machine learning algorithms can process and analyze vast amounts of data in minutes, identifying patterns and trends that would have taken human analysts weeks or even months to discover.
Machine learning models can identify hidden correlations in consumer behavior, detect market shifts, and forecast future trends. This kind of predictive analysis enables businesses to stay ahead of the curve by making informed decisions based on AI’s advanced calculations.
Case Example: A company may use machine learning to predict customer churn based on historical data. Instead of relying on assumptions, AI tools can identify key factors that contribute to customer attrition and recommend personalized strategies to retain them.
3. Improved Accuracy and Insights with Natural Language Processing (NLP)
Natural Language Processing (NLP) is another key area where AI is making a massive impact. Traditional survey responses, open-ended questions, and qualitative data were once difficult and time-consuming to analyze. NLP changes this by allowing machines to understand, interpret, and analyze human language more effectively than ever.
NLP-powered tools can sift through customer reviews, social media comments, and online feedback, extracting valuable insights into consumer sentiment, preferences, and emerging trends. By processing unstructured data (such as text, speech, and images), NLP helps market researchers gain a more comprehensive understanding of their audience.
For example, an AI tool can scan thousands of social media posts and identify overall sentiment around a brand, whether positive, negative, or neutral. This insight is crucial for businesses looking to adjust their strategies based on real-time consumer feedback.
4. Personalized and Targeted Research
AI is enabling more personalized market research, allowing businesses to gather highly targeted insights based on specific demographics, behaviors, or preferences. Traditional market research methods often produce broad, generalized data that may not accurately reflect the needs of niche markets. However, AI allows for hyper-targeted research, delivering insights that are specific to individual customer segments.
Through machine learning, AI can create personalized survey questions or focus group discussions tailored to each respondent’s past behavior, preferences, and feedback. This level of personalization provides more accurate insights and improves customer engagement.
5. Predictive Analytics for Future Trends
One of the most exciting ways AI is disrupting market research is through predictive analytics. By analyzing historical data and identifying trends, AI systems can forecast future market behaviors with a high degree of accuracy. This is invaluable for businesses looking to stay competitive in rapidly changing markets.
Predictive analytics can help companies anticipate consumer needs, spot potential market shifts, and proactively develop new products or services that align with emerging trends. This is a significant improvement over traditional research methods, which are often retrospective and reactive.
6. AI-Enhanced Data Visualization
Data visualization is a critical aspect of market research. AI tools now offer sophisticated data visualization techniques that make it easier for decision-makers to understand complex data patterns. AI-powered visualization tools can transform raw data into intuitive charts, graphs, and dashboards, offering actionable insights at a glance.
Tools like Tableau or Power BI, enhanced with AI algorithms, can provide dynamic, real-time visualizations, allowing businesses to monitor trends and performance metrics continuously. This enables faster decision-making and ensures that stakeholders have a clear understanding of the data at all times.
Benefits of AI in Market Research
AI’s integration into market research provides numerous benefits to businesses and researchers alike. These benefits include:
Faster insights: AI-powered tools can process and analyze data in real time, providing insights almost instantly.
Cost-efficiency: Automation reduces the need for large research teams, lowering overall costs.
Improved accuracy: Machine learning algorithms reduce the risk of human error, delivering more accurate insights.
Enhanced scalability: AI can handle vast amounts of data, making it ideal for large-scale market research projects.
Data-driven decision-making: AI’s predictive capabilities enable businesses to make informed, data-driven decisions.
Challenges and Considerations
While AI offers numerous benefits, it’s essential to recognize the challenges that come with its implementation. These challenges include:
Data Privacy Concerns: With increased data collection, the risk of breaches and privacy violations rises. Businesses need to ensure compliance with data protection regulations like GDPR.
Bias in AI Models: AI models are only as good as the data they are trained on. If the data is biased, the AI’s findings will be skewed, leading to inaccurate conclusions.
Job Displacement: As AI automates more tasks, some jobs in market research may become obsolete. This calls for a shift in skillsets, with more emphasis on AI and data science expertise.
Ethical Considerations: AI can be used to manipulate consumer behavior, raising ethical concerns about its use in market research.
The Future of AI in Market Research
The future of AI in market research is incredibly promising. As AI continues to evolve, we can expect even more advanced technologies that provide deeper insights, faster results, and more personalized data. Some key trends to watch for include:
AI-powered focus groups: Virtual focus groups driven by AI could provide real-time feedback, eliminating the need for in-person sessions.
Voice and image recognition: AI will further enhance its capabilities to analyze voice and image data, providing a more holistic view of consumer sentiment.
AI-generated insights: Rather than simply collecting and analyzing data, AI tools could autonomously generate insights and recommend actions based on those insights.
Conclusion
AI is undoubtedly disrupting the market research industry, offering unprecedented speed, accuracy, and depth in data collection and analysis. As businesses continue to adopt AI technologies, they will gain a significant competitive advantage, allowing them to understand consumer behavior more deeply and make data-driven decisions with confidence.
While there are challenges to consider, the benefits of AI in market research far outweigh the drawbacks. Companies that embrace AI will not only enhance their research processes but also unlock new opportunities for growth in a rapidly changing market landscape.
For more insights on how AI is reshaping the market research landscape and to explore how your business can leverage these technologies, contact us at Philomath Research—a leader in AI-driven market research solutions.
FAQs
1. What are market research solutions, and how do they help build better brands?
Market research solutions involve tools and methodologies for gathering and analyzing data on consumer preferences, market trends, and competitors. By understanding these insights, businesses can make data-driven decisions that enhance brand identity, improve customer satisfaction, and boost overall market performance.
2. What is primary market research, and why is it essential for brand growth?
Primary market research involves collecting data directly from your target audience through methods like surveys, interviews, and focus groups. It provides original, specific insights into customer behaviors and preferences, helping brands create tailored strategies for effective brand growth and customer engagement.
3. How does primary market research differ from secondary research?
Primary market research involves gathering firsthand data directly from consumers, while secondary research uses existing data from studies or reports created by others. Primary research offers more relevant and targeted insights tailored to your specific business needs.
4. How can primary market research improve a company’s branding strategy?
Primary market research provides direct feedback from consumers about their perceptions of a brand, helping companies understand what resonates with their audience. This data enables brands to refine their messaging, product offerings, and marketing campaigns to better align with customer needs and preferences, strengthening brand loyalty and recognition.
5. What are the key methods used in primary market research?
Common primary market research methods include:
Surveys: To collect quantitative data on customer opinions and behaviors.
Focus Groups: To gather qualitative insights by engaging small groups of participants in discussions.
In-depth Interviews: To explore detailed feedback and perspectives from individuals.
Observational Research: To understand how consumers interact with products in real-life scenarios.
6. How often should businesses conduct primary market research?
Businesses should conduct primary market research regularly to keep up with evolving consumer preferences and market trends. For established brands, this can mean conducting research annually or before major product launches or marketing campaigns. Emerging brands may benefit from more frequent research to ensure they are accurately targeting their audience.
7. Can primary market research help with product development?
Yes, primary market research is essential for product development. By collecting direct feedback from your target audience, you can better understand their needs, preferences, and pain points. This helps businesses design products that meet customer expectations, test product concepts, and reduce the risk of product failure in the market.
8. What are the benefits of using surveys for primary market research?
Surveys are a cost-effective method of collecting large volumes of quantitative data quickly. They allow businesses to gather insights on customer preferences, opinions, and behaviors, which can be used to refine branding, improve products, and tailor marketing strategies for better engagement.
9. How can primary market research help businesses differentiate themselves from competitors?
Primary market research helps businesses identify gaps in the market and understand what competitors are offering. By gaining a deeper understanding of customer needs and preferences, companies can create unique value propositions, tailor their branding strategies, and offer solutions that stand out from competitors.
10. How can Philomath Research assist with primary market research for building better brands?
Philomath Research specializes in customized primary market research solutions that deliver actionable insights. We design and execute surveys, focus groups, interviews, and other research methods to help businesses better understand their customers and market dynamics. Our solutions are tailored to improve brand strategies, product offerings, and customer engagement. Contact us today to discover how we can help your brand grow through primary research.
0 notes
Text
Compare Data Analyst vs. Data Scientist Careers
Data Science and Data Analytics are interconnected yet distinct fields that play crucial roles in the modern data-driven landscape. Understanding their differences, similarities, and career implications can help individuals choose the right path based on their skills and interests.
Overview of Data Science and Data Analytics
Data Science
Data Science is a multidisciplinary field that employs scientific methods, processes, algorithms, and systems to extract insights from structured and unstructured data. It encompasses a broad range of activities, including data collection, cleaning, analysis, and modeling. Data Science relies heavily on advanced statistical techniques, machine learning, and artificial intelligence to uncover patterns, make predictions, and drive decision-making processes. The primary goal is to derive actionable insights that can inform strategic business decisions and foster innovation.
Key Characteristics of Data Science:
Scope: Encompasses various tasks from data preparation to building complex predictive models.
Techniques: Utilizes advanced statistical modeling, machine learning algorithms, and data mining.
Data Volume: Often deals with large, complex datasets, including unstructured data types like text and images.
Objective: Aim to discover hidden patterns and develop predictive models to solve complex problems.
Skills Required: Strong foundation in mathematics, statistics, programming (e.g., Python, R), and domain expertise.
Tools Used: Programming languages (Python, R), big data technologies (Hadoop, Spark), and machine learning libraries (TensorFlow, sci-kit-learn) are commonly employed.
Data Analytics
Data Analytics, on the other hand, focuses on examining datasets to draw conclusions and insights. It is more concerned with analyzing historical data to inform business decisions and optimize operations. Data Analysts typically use statistical tools and visualization techniques to interpret data, identify trends and present findings in a comprehensible manner. Their work is often more straightforward compared to that of Data Scientists, as they primarily deal with well-defined datasets and specific business questions.
Key Characteristics of Data Analytics:
Scope: Primarily focuses on analyzing existing data to answer specific business questions.
Techniques: Employ statistical analysis, descriptive statistics, and data visualization methods.
Data Volume: Generally works with structured data and smaller datasets compared to Data Science.
Objective: Aim to provide actionable insights for business improvements based on historical data.
Skills Required: Strong analytical skills, proficiency in data visualization tools (e.g., Tableau, Power BI), and knowledge of statistical software (e.g., SQL, Excel).
Tools Used: Commonly utilizes Excel, SQL, Tableau, and Power BI for data manipulation and visualization.
Career Paths and Job Roles
Data Scientist
Data Scientists are expected to have a more advanced skill set, often requiring a master's degree or higher in a related field. Their roles involve designing and constructing new processes for data modeling and production, employing machine learning techniques, and developing algorithms to predict future trends. They typically work on projects that involve building recommendation systems, fraud detection models, and natural language processing applications.
Data Analyst
Data Analysts usually require a bachelor's degree and focus on interpreting existing data to help organizations make informed decisions. They create visual representations of data, prepare reports, and communicate findings to stakeholders. Their roles can vary widely across industries, and they may hold titles such as business analyst, market research analyst, or financial analyst.
Choosing the Right Path
When deciding between a career in Data Science and Data Analytics, consider the following factors:
Interest in Technical Skills: If you enjoy programming, machine learning, and complex problem-solving, Data Science may be the right fit. Conversely, if you prefer working with data to derive insights and communicate findings, Data Analytics could be more suitable.
Educational Background: Data Science typically requires a stronger technical and mathematical background, often necessitating advanced degrees. Data Analytics roles may be more accessible with a bachelor's degree and relevant experience.
Career Goals: Consider your long-term career aspirations. Data Scientists often have higher earning potential due to the complexity of their work and the skills required. However, Data Analysts also play a vital role in organizations and can advance to senior positions with experience and expertise.
In summary, many colleges teach both Data Science and Data Analytics but Arya College of Engineering & I.T. is the best Engineering College that involves working with data, Data Science has a broader scope, focusing on solving complex problems using advanced techniques like machine learning and artificial intelligence, while Data Analytics is more focused on answering specific business questions using structured data and providing actionable insights to drive decision-making. both Data Science and Data Analytics offer valuable career opportunities, each with its unique focus, skill requirements, and job roles. Assessing your interests, educational background, and career goals will help guide your decision on your path.
0 notes
Text
What is AI automation, its implementaton and Ethical Considerations? - Bionic

This Blog was Originally Published at:
What is AI automation, its implementaton and Ethical Considerations? — Bionic
The idea of business automation has always been based on the concept of efficiency and the realization of productivity. However, in 2024, AI has provided a new perspective to existing possibilities.
It is not about replacing a man’s job, it is about making that man a superhero, enhancing decision-making, discovering opportunities, and innovating new processes.
But, why is AI the game-changer? Traditional business automation focuses on automating routine, discrete processes that involve rule-based execution. AI, in contrast, works best in highly complex environments.

Let’s find out in this blog what AI automation is and how it can be implemented in your business.
What is AI Automation?
AI automation is a step beyond the other forms of automation in its capability and applicability.
While traditional automation can be defined as the execution of a certain set of instructions following predefined standards, AI automation adds a level of adaptability.
It equips machines with learning, reasoning, and decision-making capabilities which were earlier attributed to human beings only.
At its core, AI automation involves the use of intelligent systems that can:
1. Learn from Data: While AI models are designed with complex algorithms that work on large datasets, these models learn on their own, and that makes them effective predictors of what an analyst cannot see. The learning ability of these AI models means that their capabilities evolve.
2. Adapt to New Information: Another advantage of AI automation as opposed to rule-based systems, is that it can adapt to new input and conditions. Its versatility is particularly significant when applied to complicated and unpredictable situations that may arise.
3. Make Complex Decisions: AI automation is well suited for handling decision-making processes that involve balancing several considerations and possible consequences.
Is AI Automation the Same as Automation?
AI automation and traditional automation, while both aimed at task execution, are distinct in their approach and capabilities.
Traditional automation, often using Robotic Process Automation (RPA), follows pre-programmed rules and thrives in handling repetitive, high-volume tasks with clear instructions. However, it lacks the flexibility to adapt to new information or make complex decisions, limiting its scope to simpler processes.
AI automation harnesses the power of machine learning and other AI techniques, mimicking human cognitive abilities like pattern recognition, decision-making, and language understanding.
The AI Automation Advantage
Firms all around the world need to automate their enterprises. Enterprise automation can include automating IT processes, business workflows, or customer service interactions, often to improve efficiency, reduce costs, and enhance productivity.
AI-powered Enterprise automation is delivering impressive results across industries:
1. Reduced Costs: Making things easier and faster to do, reducing human intervention, and improving the utility of resources.
2. Increased Revenue: Entering new markets, customizing customer engagements, and speeding up the product delivery cycle.
3. Improved Customer Satisfaction: Offering quicker and more accurate replies and being proactive with customers.
4. Enhanced Employee Engagement: Easing them from routine work responsibilities that would consume a lot of their time and restrict them from doing more productive tasks.
5. Make better decisions: Novel AI-based artificial intelligence tools for business help to reveal clear visions of the current business situation.
6. Innovate faster: AI can produce new concepts and solutions in a much faster manner than humans thus shortening the time that is taken in the innovation process.
7. Build resilience: AI systems can be sensitive to interruptions and able to recognize if there are threats that can emerge later.
The State of AI Automation in 2024: A Data-Driven Reality Check
McKinsey estimates that AI could potentially automate activities that absorb 60 to 70 percent of employees’ time today.
Gartner predicts that by 2025, customer service organizations that embed AI in their multichannel customer engagement platforms will elevate operational efficiency by 25%.
World Economic Forum reports that AI is expected to create 97 million new jobs by 2025.

Implementation of AI Automation in Businesses
Integrating AI automation into your organization is a multi-faceted journey that demands careful planning and execution. A strategic approach is essential to ensure successful adoption and maximize the return on your investment.
1. Identify Opportunities:
Begin by conducting a thorough assessment of your business processes. Look for tasks that are:
Repetitive: Manual tasks that consume significant time and resources.
Rule-based: Processes that follow a clear set of instructions and logic.
Data-heavy: Tasks that involve analyzing large volumes of data to extract insights or make decisions.
Prioritize opportunities based on their potential impact on efficiency, cost savings, and customer experience.
2. Choose the Right Tools:
Artificial Intelligence Tools for Business leverage AI to improve business operations. These tools can be used for data analysis, customer service, marketing automation, or other specific business needs. Research and evaluate different AI platforms and solutions based on:
Your Specific Needs: Consider the types of tasks you want to automate, the scale of your operations, and your industry-specific requirements.
Budget: AI solutions can range from open-source libraries to enterprise-grade platforms. Choose options that align with your financial resources.
Ease of Use: Look for tools with intuitive interfaces and minimal technical barriers to entry.
Integration: Ensure the chosen solution can seamlessly integrate with your existing systems and workflows.
3. Data is Key
AI models thrive on data. Ensure you have:
Clean Data: Accurate, well-structured data is essential for training effective AI models. Invest in data cleaning and preprocessing to eliminate errors and inconsistencies.
Relevant Data: The data used for training should be representative of the real-world scenarios the AI will encounter.
Sufficient Data: The amount of data required depends on the complexity of the task. More data generally leads to better AI performance.
Consider data privacy regulations and security measures to protect sensitive information.
4. Start Small, Scale Up:
Resist the temptation to automate everything at once. Begin with a pilot project focused on a specific use case. This allows you to:
Test and Validate: Assess the AI’s performance in a controlled environment.
Identify Challenges: Address any technical or operational issues that arise.
Refine the Solution: Make adjustments based on real-world feedback.
Once the pilot proves successful, you can gradually scale up to other processes and departments.
5. Invest in Talent:
Building a team with AI expertise is crucial. You can:
Develop In-House Talent: Train existing employees in AI skills or hire new talent with specialized knowledge.
Partner with Specialists: Collaborate with AI consulting firms or technology providers to access external expertise.
Ensure your team has a deep understanding of AI, machine learning, and data science to effectively manage and maintain your AI automation systems.
By following this strategic approach, you can successfully navigate the implementation process, minimize risks, and achieve your desired outcomes with AI automation.
Challenges of Implementing AI Automation
AI automation improves the efficiency of work, however, important issues related to ethics such as bias and discrimination arise.
AI systems are trained on big data, and this data can contain specific prejudices. Such biases if not prevented cause unfair outcomes.
1. Data Bias: Statistics hold social bias in them and record the trends in societies’ injustice. For example, if an Artificial intelligence for business is trained on one set of data in any AI agency, it is likely to fail to give a fair result for the other set of people. This can be especially crucial in areas such as employment, credit, and policing.
2. Lack of Algorithmic Transparency: Algorithms, which are at the core of AI for business and related services, aren’t transparent. This makes it difficult to understand how the AI system reached a specific solution. When businesses lack transparency on how their AI systems work, they cannot also handle bias.
3. AI Hallucination: AI hallucinations are defined as situations in which an AI produces outcomes that are erroneous or do not make any sense. These erroneous outputs are not based on the real world, which is especially prevalent in generative models. High-stakes settings and contexts especially those in the health and legal realms are at risk from this phenomenon.
Countering Implementation Challenges
Organizations can adopt some solutions to manage AI hallucinations.
1. Transparency and Explainability: Develop AI systems with any artificial intelligence automation agency that can explain their decision-making processes in a way understandable to humans. This fosters trust and allows for better accountability.
2. Fairness and Bias Mitigation: Proactively identify and rectify biases in data and algorithms to ensure fair and equitable outcomes. Implement rigorous testing and validation procedures to ensure the AI system is unbiased and does not discriminate against any particular group.
3. Robust Cybersecurity and Privacy: Safeguard AI systems from malicious attacks and unauthorized access. Implement robust privacy measures to protect sensitive personal data used in AI applications. Ensure compliance with data protection regulations.
4. Grounding AI: Grounding AI is incorporating knowledge of the physical world and its context into AI systems. To minimize or even prevent occurrences of hallucination, organizations must ensure that the underlying AI models are trained on diverse and high-quality datasets.
5. Human-in-the-Loop(HITL): HITL techniques involve the presence of human oversight over the decisions made by AI systems. Involving human supervision ensures that AI outputs are checked before they are implemented.

How Bionic Can Help?
Bionic can improve the productivity of different organizations by enabling the delegation of various routine tasks to Bionic’s human taskers. They not only perform the tasks but also give feedback that can help ensure the quality of AI systems.
Bionic AI combines AI with human inputs to eliminate contextual misinterpretation. Effective AI grounding techniques and a human-in-the-loop approach empower Bionic AI with specific and relevant information. This seamless integration of AI and human oversight makes Bionic AI change the game of business outsourcing.
Bionic AI adapts to changing human feedback making it hallucination-free and effective in dynamic environments. By mixing AI with human oversight, Bionic promises accurate and relevant results that foster customer satisfaction and trust. This synergy ensures that customer concerns with traditional AI are addressed justly, delivering outstanding customer experience.
Bionic can also help incorporate HITL and Grounding AI techniques in an organization’s AI system. This can save companies from incurring damaging costs caused by AI hallucination. Request a demo now!
Conclusion
In 2024, AI automation is not just a trend; it’s a paradigm shift reshaping the business landscape. The organizations that thrive in this era will be those that proactively embrace AI, integrating it into their strategic plans and leveraging its transformative power to unlock new levels of efficiency, innovation, and growth.
As a leader in your industry, the time to act is now. Seize the opportunities presented by AI automation, and position your organization for a future where intelligent machines work alongside human ingenuity to achieve remarkable results.
Let this be the year you embark on your AI journey. The rewards are substantial, and the possibilities are limitless.
Tired of wasting hours on tedious, repetitive tasks that drain your energy and distract you from your important work? Bionic is an easy-to-use business automation software that takes care of your time-consuming, repetitive work. Request a demo now!
0 notes
Text
Unlocking Flexibility: The Power of Contract Staffing for React Developers
In today's fast-paced digital landscape, businesses often find themselves grappling with the challenge of acquiring top-tier talent, especially in specialized domains like React development. As technology evolves rapidly, so do the demands for skilled professionals who can navigate the intricacies of modern frameworks and languages.
Enter contract staffing, a dynamic solution that offers unparalleled flexibility and agility for companies seeking React developers. Jurysoft, a leading provider of tech staffing solutions, is at the forefront of this paradigm shift, revolutionizing how businesses address their talent needs.
Adaptability in an Ever-Changing Environment
In the world of software development, adaptability is key. Projects ebb and flow, priorities shift, and timelines evolve. Traditional hiring methods often struggle to keep pace with these fluctuations. This is where contract staffing shines. By leveraging Jurysoft's extensive network of React developers, companies can swiftly scale their teams up or down according to project requirements.
Whether it's a short-term sprint to meet a deadline or a long-term engagement for a large-scale application overhaul, contract staffing offers the flexibility to tailor the workforce precisely to the task at hand. With Jurysoft handling the recruitment process, companies can focus on what they do best – delivering exceptional products and services to their clients.
Access to Specialized Expertise
React development is a specialized field that demands specific skills and experience. Finding and hiring top React talent can be a daunting challenge, especially for businesses operating outside tech hubs or facing talent shortages. Jurysoft removes these barriers by providing access to a vast pool of pre-vetted, highly skilled React developers.
Whether it's proficiency in React Native for mobile app development or mastery of cutting-edge React features for web applications, Jurysoft ensures that companies have access to the expertise they need to succeed. By tapping into this talent pool, businesses can accelerate their development cycles, innovate more rapidly, and stay ahead of the competition.
Cost-Effective Solutions
Cost considerations are always top of mind for businesses, especially in today's economic climate. Traditional hiring processes come with a slew of expenses, from recruitment fees to onboarding costs to employee benefits. Contract staffing offers a cost
-effective alternative, allowing companies to access top-tier talent without the overhead associated with full-time employment.
With contract staffing, businesses pay only for the hours worked or the duration of the project, eliminating the need for long-term commitments or costly benefits packages. This pay-as-you-go model not only helps companies manage their budgets more effectively but also ensures that they get the most value out of their investment in talent.
Furthermore, contract staffing can be a strategic tool for managing fluctuations in workload and optimizing resource allocation. Instead of being saddled with a fixed payroll, companies can scale their teams up or down in response to changing demands, maximizing efficiency and minimizing waste.
Mitigating Risk and Enhancing Flexibility
In today's volatile business environment, risk mitigation is paramount. Contract staffing offers a level of flexibility and risk mitigation that traditional hiring models simply can't match. Whether it's navigating uncertain market conditions, responding to unexpected changes in project scope, or mitigating the risk of talent shortages, contract staffing provides companies with the agility they need to adapt and thrive.
By partnering with Jurysoft for their contract staffing needs, businesses gain access to a wealth of resources and expertise, allowing them to focus on driving innovation and growth without being bogged down by the complexities of recruitment and HR management.
In conclusion, contract staffing represents a paradigm shift in how businesses approach talent acquisition, particularly in specialized fields like React development. With Jurysoft as a trusted partner, companies can unlock the full potential of contract staffing, harnessing the power of flexibility, access to specialized expertise, and cost-effective solutions to drive success in today's digital economy.
0 notes