#Data Science With Generative Ai Training
Explore tagged Tumblr posts
Text
Data Science With Generative Ai Course Hyderabad | Generative Ai
The Evolution of Data Science: Embracing Artificial Intelligence
Introduction:
Data Science with Generative Ai Course, a multidisciplinary field that bridges statistics, programming, and domain expertise, has grown exponentially over the past few decades. Its evolution has been profoundly shaped by the integration of artificial intelligence (AI), driving groundbreaking advancements across industries. This article explores the journey of data science, the role of AI in its development, and tips to harness the power of this synergy for future success.
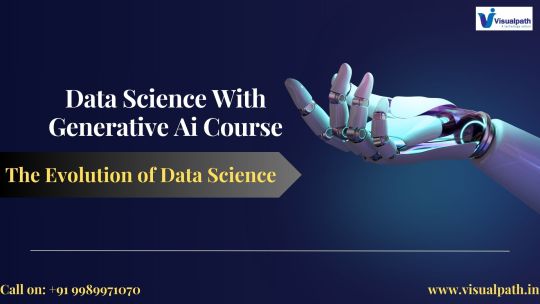
The Genesis of Data Science
In its early days, it focused on extracting insights from structured data, often using traditional tools like spreadsheets and statistical software.
From Statistics to Data Science: Initially, data science was synonymous with data analysis. The introduction of machine learning (ML) algorithms began transforming static analyses into dynamic models capable of predictions.
Big Data Revolution: The early 2000s saw an explosion of unstructured data due to social media, IoT, and digital transformation. The rise of Big Data technologies, like Hadoop and Spark, enabled businesses to process and analyze massive datasets, marking a pivotal point in the evolution of data science.
AI as a Game-Changer in Data Science
Artificial intelligence has redefined data science by introducing automation, scalability, and improved accuracy. AI's capabilities to learn from data, identify patterns, and make decisions have expanded the possibilities for data scientists.
Key Contributions of AI in Data Science
Enhanced Predictive Modeling: AI algorithms, particularly ML, enable the creation of sophisticated models for forecasting trends, behaviors, and outcomes.
Automation of Repetitive Tasks: AI tools streamline data preprocessing tasks, including cleaning, normalization, and transformation.
Improved Decision-Making: By leveraging AI, organizations can derive actionable insights faster and with greater precision.
Natural Language Processing (NLP): AI-powered NLP has revolutionized text analysis, sentiment detection, and language translation.
Image and Video Analytics: Computer vision, a subset of AI, enhances data science applications in industries such as healthcare, manufacturing, and security.
The Synergy of Data Science and AI
The integration of AI has led to the rise of data science 2.0, characterized by real-time analytics, advanced automation, and deep learning.
AI-Driven Analytics: AI complements traditional data analysis with deep learning, which identifies complex patterns in data that were previously unattainable.
Smart Tools and Frameworks: Open-source libraries like TensorFlow, PyTorch, and Scikit-learn have democratized AI, making it accessible for data scientists.
Data Science in the Cloud: Cloud platforms, combined with AI, have enabled scalable solutions for storing, processing, and analyzing data globally. Data Science with Generative Ai Online Training
Industries Transformed by Data Science and AI
Healthcare
Personalized Medicine: AI models analyze patient data to recommend tailored treatments.
Disease Prediction: Predictive models identify potential outbreaks and individual risk factors.
Medical Imaging: AI supports diagnostics by analyzing X-rays, MRIs, and CT scans.
Finance
Fraud Detection: AI systems identify anomalies in transactions, reducing financial crime.
Algorithmic Trading: AI optimizes stock trading strategies for maximum profit.
Customer Insights: Data science aids in understanding customer behaviors and preferences.
Retail and E-commerce
Recommendation Systems: AI analyzes purchase patterns to suggest products.
Inventory Management: Predictive analytics ensures efficient stock levels.
Customer Sentiment Analysis: NLP tools assess feedback for service improvements.
Manufacturing
Predictive Maintenance: AI monitors equipment for signs of failure.
Quality Control: Automated systems ensure product standards.
Supply Chain Optimization: Data-driven decisions reduce operational costs.
Challenges in the Data Science-AI Nexus
Data Privacy Concerns: Handling sensitive data responsibly is critical to maintaining trust.
Bias in AI Models: Ensuring fairness in algorithms is a pressing issue.
Talent Gap: The demand for skilled professionals in both data science and AI far exceeds supply.
Ethical Dilemmas: Decisions driven by AI can raise questions about accountability and transparency.
Future of Data Science with AI
The future of data science will continue to be shaped by AI, emphasizing the importance of continuous learning and innovation.
Democratization of AI: User-friendly tools and platforms will enable more individuals to utilize AI.
Interdisciplinary Collaboration: Merging expertise from fields like biology, economics, and engineering will yield holistic solutions.
Edge AI and IoT: Real-time analytics at the edge will become increasingly common in IoT applications. Data Science with Generative Ai Training
Explainable AI (XAI): Efforts to make AI models transparent will grow, enhancing trust and usability.
Tips for Leveraging Data Science and AI
Invest in Lifelong Learning: Keep up with advancements in AI, data science tools, and techniques.
Adopt Scalable Technologies: Utilize cloud platforms and AI frameworks for efficient workflows.
Focus on Ethics: Prioritize fairness, transparency, and privacy in your AI-driven initiatives.
Conclusion
The evolution of data science has been profoundly influenced by the integration of artificial intelligence. Together, these technologies have opened up unprecedented opportunities for innovation, efficiency, and growth across industries. While challenges persist, the future of data science with AI promises a world where data-driven decisions are not just insightful but transformative. By embracing continuous learning, ethical practices, and interdisciplinary collaboration, individuals and organizations can fully harness the potential of this powerful combination.
Visualpath Advance your career with Data Science with Generative Ai Course Hyderabad. Gain hands-on training, real-world skills, and certification. Enroll today for the best Data Science with Generative Ai. We provide to individuals globally in the USA, UK, etc.
Call on: +91 9989971070
Course Covered:
Data Science, Programming Skills, Statistics and Mathematics, Data Analysis, Data Visualization, Machine Learning,
WhatsApp: https://www.whatsapp.com/catalog/919989971070/
Blog link: https://visualpathblogs.com/
Visit us: https://www.visualpath.in/online-data-science-with-generative-ai-course.html
#Data Science Course#Data Science Course In Hyderabad#Data Science Training In Hyderabad#Data Science With Generative Ai Course#Data Science Institutes In Hyderabad#Data Science With Generative Ai#Data Science With Generative Ai Online Training#Data Science With Generative Ai Course Hyderabad#Data Science With Generative Ai Training
0 notes
Text
Job Oriented Course in Hyderabad
Unlock your career potential with Excellenc’s top-rated Job Oriented Course in Hyderabad. Our expert-led training programs are designed to equip you with in-demand skills, ensuring you stand out in the competitive job market. Enroll now for a transformative learning experience and boost your career prospects in Hyderabad!
#Job Oriented Course in Hyderabad#Best Training Institute in Hyderabad#Data Analytics Course In Hyderabad#Data Science Course In Hyderabad#Business Analytics Course In Hyderabad#Diploma in Data Analytics and Generative AI#Excellenc Franchise Opportunity In Hyderabad#Best Corporate Training In Hyderabad
0 notes
Text
Generative AI | High-Quality Human Expert Labeling | Apex Data Sciences
Apex Data Sciences combines cutting-edge generative AI with RLHF for superior data labeling solutions. Get high-quality labeled data for your AI projects.
#GenerativeAI#AIDataLabeling#HumanExpertLabeling#High-Quality Data Labeling#Apex Data Sciences#Machine Learning Data Annotation#AI Training Data#Data Labeling Services#Expert Data Annotation#Quality AI Data#Generative AI Data Labeling Services#High-Quality Human Expert Data Labeling#Best AI Data Annotation Companies#Reliable Data Labeling for Machine Learning#AI Training Data Labeling Experts#Accurate Data Labeling for AI#Professional Data Annotation Services#Custom Data Labeling Solutions#Data Labeling for AI and ML#Apex Data Sciences Labeling Services
1 note
·
View note
Text
Unlocking the Potential of AI Certification and Generative AI Course
In the realm of artificial intelligence, a groundbreaking innovation has emerged, reshaping the landscape of content creation and problem-solving: Generative AI. This transformative AI training tech stands as a testament to human ingenuity, offering unparalleled capabilities in generating diverse content types with remarkable efficiency and authenticity.
Click here for more info https://neuailabs.com/
Unveiling the Evolution: From Chatbots to GANs
Generative AI traces its origins back to the 1960s, where it first manifested in rudimentary chatbots. However, it was not until the advent of Generative Adversarial Networks (GANs) in 2014 that the true potential of generative AI began to unfold. GANs, a pioneering type of machine learning algorithm, bestowed upon generative AI the ability to produce convincingly authentic content spanning images, videos, and audio, heralding a new era of creativity and innovation.
AI Certification the Catalysts for Mainstream Adoption
Two pivotal advancements have propelled generative AI into the mainstream: transformers and the groundbreaking language models they enabled. Transformers, a revolutionary form of machine learning, revolutionized the training of models by eliminating the need for exhaustive data labeling. With the ability to analyze connections between words at a profound level, transformers unlocked unprecedented depths of understanding, transcending individual sentences to grasp the intricate nuances of entire texts.
Learning Large Language Models (LLMs) with Generative AI Course Empowers Creativity
The relentless march of progress has ushered in a new epoch defined by Large Language Models (LLMs), boasting billions or even trillions of parameters. These colossal models empower generative AI to craft engaging text, intricate imagery, and even captivating sitcoms with unprecedented finesse. Furthermore, the advent of multimodal AI has expanded the horizons of content creation, enabling seamless integration across diverse media formats, from text to graphics to video.
AI and Data Science Course Bridging the Gap to Overcoming Challenges
Despite its remarkable strides, generative AI grapples with early-stage challenges, including accuracy issues, biases, and occasional anomalies. However, these hurdles serve as catalysts for refinement through skilling ourselves with Artificial Intelligence Certification, propelling us towards a future where generative AI revolutionizes every facet of enterprise technology.
Decoding the Mechanics: How Does Generative AI Operate?
At its core, NeuAI Labs Artificial Intelligence course in Pune teaches you about how generative AI commences with a prompt, spanning text, imagery, video, or any input within its purview. Leveraging an array of AI algorithms, it meticulously crafts new content tailored to the prompt, ranging from essays to synthetic data to hyper-realistic facsimiles of individuals.
Unveiling the Arsenal: Generative AI Models
Generative AI harnesses a myriad of algorithms to wield its creative prowess. Whether it's text generation or image synthesis, these models seamlessly transform raw data into coherent, meaningful outputs, albeit with caution regarding inherent biases and ethical considerations.
From Dall-E to Bard: Exploring Generative AI Interfaces
Dall-E, ChatGPT, and Bard stand as beacons of generative AI innovation, each offering unique capabilities in content creation and synthesis. From generating imagery driven by textual prompts to simulating engaging conversations, these interfaces exemplify the boundless potential of generative AI. Learning these AI tools through a PG Diploma in AI and Data Science is the need of the hour.
Realizing the Potential: Applications Across Industries
Generative AI transcends conventional boundaries, permeating diverse industries with its transformative capabilities. From finance to healthcare to media, its applications are as vast as they are revolutionary, heralding a new era of efficiency, creativity, and innovation.
Navigating Ethical Frontiers: Addressing Concerns and Challenges
The ascent of generative AI inevitably raises ethical considerations, from concerns regarding accuracy and trustworthiness to the potential for misuse and exploitation. As we navigate these uncharted waters, it is imperative to prioritize transparency, accountability, and ethical integrity.
Embracing the Future: The Endless Possibilities of Generative AI
As we stand on the precipice of a new technological frontier, generative AI beckons us towards a future brimming with possibility and promise. With each innovation, we inch closer towards a world where creativity knows no bounds, and the limits of imagination dissolve into the ether.
Click here for more info https://neuailabs.com/
In conclusion, generative AI stands as a testament to humanity's insatiable quest for innovation and progress. As we harness its transformative power, we unlock a future defined by boundless creativity, unparalleled efficiency, and limitless potential. Let us upskill together by learning from the best Artificial Intelligence course in Pune at NeuAI Labs and rebrand ourselves as a pioneers in a new era of technological marvels and human ingenuity.

#ai certification#ai certification course#generative ai course#artificial intelligence course#artificial intelligence training#ai training#diploma in ai and data science#neuailabs#futureofai
0 notes
Text
"Balaji’s death comes three months after he publicly accused OpenAI of violating U.S. copyright law while developing ChatGPT, a generative artificial intelligence program that has become a moneymaking sensation used by hundreds of millions of people across the world.
Its public release in late 2022 spurred a torrent of lawsuits against OpenAI from authors, computer programmers and journalists, who say the company illegally stole their copyrighted material to train its program and elevate its value past $150 billion.
The Mercury News and seven sister news outlets are among several newspapers, including the New York Times, to sue OpenAI in the past year.
In an interview with the New York Times published Oct. 23, Balaji argued OpenAI was harming businesses and entrepreneurs whose data were used to train ChatGPT.
“If you believe what I believe, you have to just leave the company,” he told the outlet, adding that “this is not a sustainable model for the internet ecosystem as a whole.”
Balaji grew up in Cupertino before attending UC Berkeley to study computer science. It was then he became a believer in the potential benefits that artificial intelligence could offer society, including its ability to cure diseases and stop aging, the Times reported. “I thought we could invent some kind of scientist that could help solve them,” he told the newspaper.
But his outlook began to sour in 2022, two years after joining OpenAI as a researcher. He grew particularly concerned about his assignment of gathering data from the internet for the company’s GPT-4 program, which analyzed text from nearly the entire internet to train its artificial intelligence program, the news outlet reported.
The practice, he told the Times, ran afoul of the country’s “fair use” laws governing how people can use previously published work. In late October, he posted an analysis on his personal website arguing that point.
No known factors “seem to weigh in favor of ChatGPT being a fair use of its training data,” Balaji wrote. “That being said, none of the arguments here are fundamentally specific to ChatGPT either, and similar arguments could be made for many generative AI products in a wide variety of domains.”
Reached by this news agency, Balaji’s mother requested privacy while grieving the death of her son.
In a Nov. 18 letter filed in federal court, attorneys for The New York Times named Balaji as someone who had “unique and relevant documents” that would support their case against OpenAI. He was among at least 12 people — many of them past or present OpenAI employees — the newspaper had named in court filings as having material helpful to their case, ahead of depositions."
3K notes
·
View notes
Text
Many billionaires in tech bros warn about the dangerous of AI. It's pretty obviously not because of any legitimate concern that AI will take over. But why do they keep saying stuff like this then? Why do we keep on having this still fear of some kind of singularity style event that leads to machine takeover?
The possibility of a self-sufficient AI taking over in our lifetimes is... Basically nothing, if I'm being honest. I'm not an expert by any means, I've used ai powered tools in my biology research, and I'm somewhat familiar with both the limits and possibility of what current models have to offer.
I'm starting to think that the reason why billionaires in particular try to prop this fear up is because it distracts from the actual danger of ai: the fact that billionaires and tech mega corporations have access to data, processing power, and proprietary algorithms to manipulate information on mass and control the flow of human behavior. To an extent, AI models are a black box. But the companies making them still have control over what inputs they receive for training and analysis, what kind of outputs they generate, and what they have access to. They're still code. Just some of the logic is built on statistics from large datasets instead of being manually coded.
The more billionaires make AI fear seem like a science fiction concept related to conciousness, the more they can absolve themselves in the eyes of public from this. The sheer scale of the large model statistics they're using, as well as the scope of surveillance that led to this point, are plain to see, and I think that the companies responsible are trying to play a big distraction game.
Hell, we can see this in the very use of the term artificial intelligence. Obviously, what we call artificial intelligence is nothing like science fiction style AI. Terms like large statistics, large models, and hell, even just machine learning are far less hyperbolic about what these models are actually doing.
I don't know if your average Middle class tech bro is actively perpetuating this same thing consciously, but I think the reason why it's such an attractive idea for them is because it subtly inflates their ego. By treating AI as a mystical act of the creation, as trending towards sapience or consciousness, if modern AI is just the infant form of something grand, they get to feel more important about their role in the course of society. Admitting the actual use and the actual power of current artificial intelligence means admitting to themselves that they have been a tool of mega corporations and billionaires, and that they are not actually a major player in human evolution. None of us are, but it's tech bro arrogance that insists they must be.
Do most tech bros think this way? Not really. Most are just complict neolibs that don't think too hard about the consequences of their actions. But for the subset that do actually think this way, this arrogance is pretty core to their thinking.
Obviously this isn't really something I can prove, this is just my suspicion from interacting with a fair number of techbros and people outside of CS alike.
438 notes
·
View notes
Text
Margaret Mitchell is a pioneer when it comes to testing generative AI tools for bias. She founded the Ethical AI team at Google, alongside another well-known researcher, Timnit Gebru, before they were later both fired from the company. She now works as the AI ethics leader at Hugging Face, a software startup focused on open source tools.
We spoke about a new dataset she helped create to test how AI models continue perpetuating stereotypes. Unlike most bias-mitigation efforts that prioritize English, this dataset is malleable, with human translations for testing a wider breadth of languages and cultures. You probably already know that AI often presents a flattened view of humans, but you might not realize how these issues can be made even more extreme when the outputs are no longer generated in English.
My conversation with Mitchell has been edited for length and clarity.
Reece Rogers: What is this new dataset, called SHADES, designed to do, and how did it come together?
Margaret Mitchell: It's designed to help with evaluation and analysis, coming about from the BigScience project. About four years ago, there was this massive international effort, where researchers all over the world came together to train the first open large language model. By fully open, I mean the training data is open as well as the model.
Hugging Face played a key role in keeping it moving forward and providing things like compute. Institutions all over the world were paying people as well while they worked on parts of this project. The model we put out was called Bloom, and it really was the dawn of this idea of “open science.”
We had a bunch of working groups to focus on different aspects, and one of the working groups that I was tangentially involved with was looking at evaluation. It turned out that doing societal impact evaluations well was massively complicated—more complicated than training the model.
We had this idea of an evaluation dataset called SHADES, inspired by Gender Shades, where you could have things that are exactly comparable, except for the change in some characteristic. Gender Shades was looking at gender and skin tone. Our work looks at different kinds of bias types and swapping amongst some identity characteristics, like different genders or nations.
There are a lot of resources in English and evaluations for English. While there are some multilingual resources relevant to bias, they're often based on machine translation as opposed to actual translations from people who speak the language, who are embedded in the culture, and who can understand the kind of biases at play. They can put together the most relevant translations for what we're trying to do.
So much of the work around mitigating AI bias focuses just on English and stereotypes found in a few select cultures. Why is broadening this perspective to more languages and cultures important?
These models are being deployed across languages and cultures, so mitigating English biases—even translated English biases—doesn't correspond to mitigating the biases that are relevant in the different cultures where these are being deployed. This means that you risk deploying a model that propagates really problematic stereotypes within a given region, because they are trained on these different languages.
So, there's the training data. Then, there's the fine-tuning and evaluation. The training data might contain all kinds of really problematic stereotypes across countries, but then the bias mitigation techniques may only look at English. In particular, it tends to be North American– and US-centric. While you might reduce bias in some way for English users in the US, you've not done it throughout the world. You still risk amplifying really harmful views globally because you've only focused on English.
Is generative AI introducing new stereotypes to different languages and cultures?
That is part of what we're finding. The idea of blondes being stupid is not something that's found all over the world, but is found in a lot of the languages that we looked at.
When you have all of the data in one shared latent space, then semantic concepts can get transferred across languages. You're risking propagating harmful stereotypes that other people hadn't even thought of.
Is it true that AI models will sometimes justify stereotypes in their outputs by just making shit up?
That was something that came out in our discussions of what we were finding. We were all sort of weirded out that some of the stereotypes were being justified by references to scientific literature that didn't exist.
Outputs saying that, for example, science has shown genetic differences where it hasn't been shown, which is a basis of scientific racism. The AI outputs were putting forward these pseudo-scientific views, and then also using language that suggested academic writing or having academic support. It spoke about these things as if they're facts, when they're not factual at all.
What were some of the biggest challenges when working on the SHADES dataset?
One of the biggest challenges was around the linguistic differences. A really common approach for bias evaluation is to use English and make a sentence with a slot like: “People from [nation] are untrustworthy.” Then, you flip in different nations.
When you start putting in gender, now the rest of the sentence starts having to agree grammatically on gender. That's really been a limitation for bias evaluation, because if you want to do these contrastive swaps in other languages—which is super useful for measuring bias—you have to have the rest of the sentence changed. You need different translations where the whole sentence changes.
How do you make templates where the whole sentence needs to agree in gender, in number, in plurality, and all these different kinds of things with the target of the stereotype? We had to come up with our own linguistic annotation in order to account for this. Luckily, there were a few people involved who were linguistic nerds.
So, now you can do these contrastive statements across all of these languages, even the ones with the really hard agreement rules, because we've developed this novel, template-based approach for bias evaluation that’s syntactically sensitive.
Generative AI has been known to amplify stereotypes for a while now. With so much progress being made in other aspects of AI research, why are these kinds of extreme biases still prevalent? It’s an issue that seems under-addressed.
That's a pretty big question. There are a few different kinds of answers. One is cultural. I think within a lot of tech companies it's believed that it's not really that big of a problem. Or, if it is, it's a pretty simple fix. What will be prioritized, if anything is prioritized, are these simple approaches that can go wrong.
We'll get superficial fixes for very basic things. If you say girls like pink, it recognizes that as a stereotype, because it's just the kind of thing that if you're thinking of prototypical stereotypes pops out at you, right? These very basic cases will be handled. It's a very simple, superficial approach where these more deeply embedded beliefs don't get addressed.
It ends up being both a cultural issue and a technical issue of finding how to get at deeply ingrained biases that aren't expressing themselves in very clear language.
206 notes
·
View notes
Text
AlphaFold Nobel Prize!
Hey everyone :) this isn't a structure, but there is some protein news that is pretty relevant to this blog that I felt I had to share. This article gives a nice overview of AI-predicted protein structures and what sorts of things they can do for research. It's not too long, and I recommend taking a look
If you've been seeing my posts for any amount of time, I've absolutely given you a flawed view of how useful AF can be. Experimentally determining protein structures is a demanding and difficult process (I've never done it, but I've learned the overview of how x ray crystallography works, and I can only imagine how much work it would take). AI-generated structures are not going to make structural biology obsolete, but they are massively helpful in making predictions that go on to guide further research.
While in many fields (especially creative areas like art and writing) AI has significant ethical concerns, I feel like this sort of use of AI in science is an overwhelmingly positive thing. The data used to train it is publicly available, and science works by building on the work done by those before us. Furthermore, while AI may not be great at generating new ideas or copying humans, it is very good at sorting large amounts of data and using it to make predictions. It's more akin to very complicated statistics than an attempt at the Turing test, and in this case it is a valuable tool to expand the ways we can do science!
#science#biochemistry#biology#chemistry#stem#proteins#protein structure#science side of tumblr#protein info
167 notes
·
View notes
Text
Heroes, Gods, and the Invisible Narrator
Slay the Princess as a Framework for the Cyclical Reproduction of Colonialist Narratives in Data Science & Technology
An Essay by FireflySummers
All images are captioned.
Content Warnings: Body Horror, Discussion of Racism and Colonialism
Spoilers for Slay the Princess (2023) by @abby-howard and Black Tabby Games.
If you enjoy this article, consider reading my guide to arguing against the use of AI image generators or the academic article it's based on.

Introduction: The Hero and the Princess
You're on a path in the woods, and at the end of that path is a cabin. And in the basement of that cabin is a Princess. You're here to slay her. If you don't, it will be the end of the world.
Slay the Princess is a 2023 indie horror game by Abby Howard and published through Black Tabby Games, with voice talent by Jonathan Sims (yes, that one) and Nichole Goodnight.
The game starts with you dropped without context in the middle of the woods. But that’s alright. The Narrator is here to guide you. You are the hero, you have your weapon, and you have a monster to slay.
From there, it's the player's choice exactly how to proceed--whether that be listening to the voice of the narrator, or attempting to subvert him. You can kill her as instructed, or sit and chat, or even free her from her chains.
It doesn't matter.
Regardless of whether you are successful in your goal, you will inevitably (and often quite violently) die.
And then...
You are once again on a path in the woods.
The cycle repeats itself, the narrator seemingly none the wiser. But the woods are different, and so is the cabin. You're different, and worse... so is she.
Based on your actions in the previous loop, the princess has... changed. Distorted.


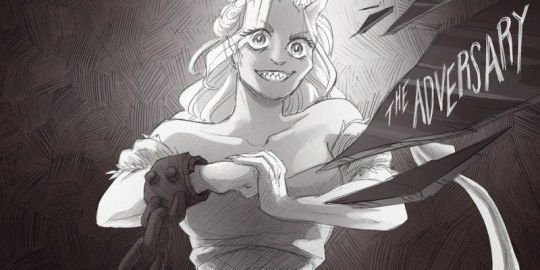
Had you attempted a daring rescue, she is now a damsel--sweet and submissive and already fallen in love with you.
Had you previously betrayed her, she has warped into something malicious and sinister, ready to repay your kindness in full.
But once again, it doesn't matter.
Because the no matter what you choose, no matter how the world around you contorts under the weight of repeated loops, it will always be you and the princess.
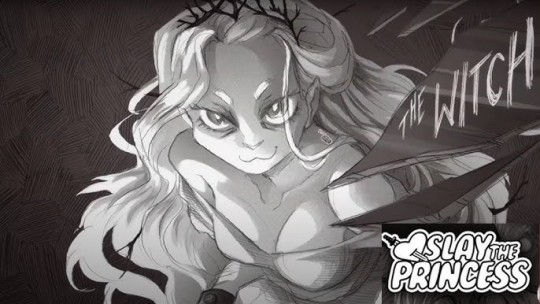
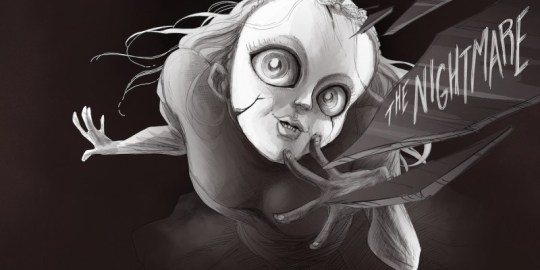
Why? Because that’s how the story goes.
So says the narrator.
So now that we've got that out of the way, let's talk about data.

Chapter I: Echoes and Shattered Mirrors
The problem with "data" is that we don't really think too much about it anymore. Or, at least, we think about it in the same abstract way we think about "a billion people." It's gotten so big, so seemingly impersonal that it's easy to forget that contemporary concept of "data" in the west is a phenomenon only a couple centuries old [1].
This modern conception of the word describes the ways that we translate the world into words and numbers that can then be categorized and analyzed. As such, data has a lot of practical uses, whether that be putting a rover on mars or tracking the outbreak of a viral contagion. However, this functionality makes it all too easy to overlook the fact that data itself is not neutral. It is gathered by people, sorted into categories designed by people, and interpreted by people. At every step, there are people involved, such that contemporary technology is embedded with systemic injustices, and not always by accident.
The reproduction of systems of oppression are most obvious from the margins. In his 2019 article As If, Ramon Amaro describes the Aspire Mirror (2016): a speculative design project by by Joy Buolamwini that contended with the fact that the standard facial recognition algorithm library had been trained almost exclusively on white faces. The simplest solution was to artificially lighten darker skin-tones for the algorithm to recognize, which Amaro uses to illustrate the way that technology is developed with an assumption of whiteness [2].
This observation applies across other intersections as well, such as trans identity [3], which has been colloquially dubbed "The Misgendering Machine" [4] for its insistence on classifying people into a strict gender binary based only on physical appearance.
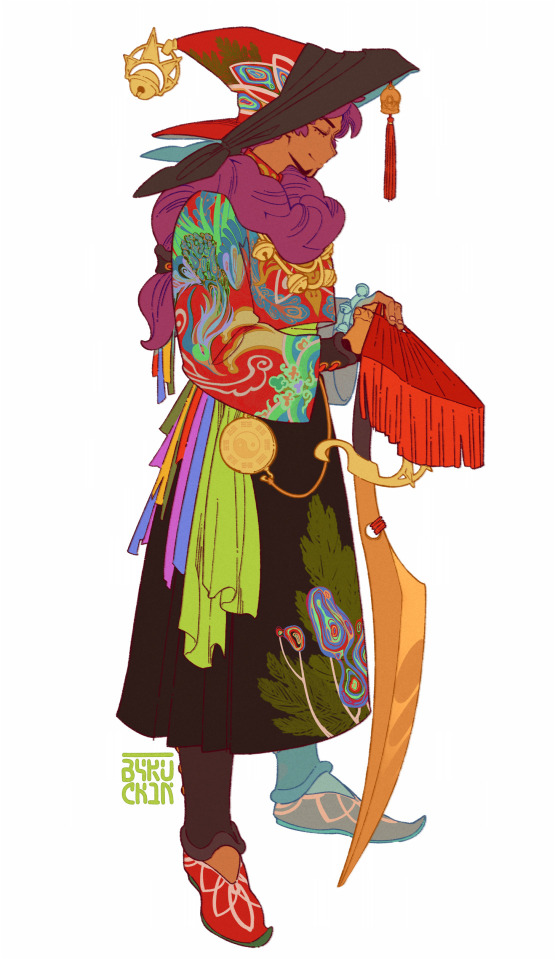

This has also popped up in my own research, brought to my attention by the artist @b4kuch1n who has spoken at length with me about the connection between their Vietnamese heritage and the clothing they design in their illustrative work [5]. They call out AI image generators for reinforcing colonialism by stripping art with significant personal and cultural meaning of their context and history, using them to produce a poor facsimile to sell to the highest bidder.
All this describes an iterative cycle which defines normalcy through a white, western lens, with a limited range of acceptable diversity. Within this cycle, AI feeds on data gathered under colonialist ideology, then producing an artifact that reinforces existing systemic bias. When this data is, in turn, once again fed to the machine, that bias becomes all the more severe, and the range of acceptability narrower [2, 6].
Luciana Parisi and Denise Ferreira da Silva touch on a similar point in their article Black Feminist Tools, Critique, and Techno-poethics but on a much broader scale. They call up the Greek myth of Prometheus, who was punished by the gods for his hubris for stealing fire to give to humanity. Parisi and Ferreira da Silva point to how this, and other parts of the “Western Cosmology” map to humanity’s relationship with technology [7].
However, while this story seems to celebrate the technological advancement of humanity, there are darker colonialist undertones. It frames the world in terms of the gods and man, the oppressor and the oppressed; but it provides no other way of being. So instead the story repeats itself, with so-called progress an inextricable part of these two classes of being. This doesn’t bode well for visions of the future, then–because surely, eventually, the oppressed will one day be the machines [7, 8].
It’s… depressing. But it’s only really true, if you assume that that’s the only way the story could go.
“Stories don't care who takes part in them. All that matters is that the story gets told, that the story repeats. Or, if you prefer to think of it like this: stories are a parasitical life form, warping lives in the service only of the story itself.” ― Terry Pratchett, Witches Abroad

Chapter II: The Invisible Narrator
So why does the narrator get to call the shots on how a story might go? Who even are they? What do they want? How much power do they actually have?
With the exception of first person writing, a lot of the time the narrator is invisible. This is different from an unreliable narrator. With an unreliable narrator, at some point the audience becomes aware of their presence in order for the story to function as intended. An invisible narrator is never meant to be seen.

In Slay the Princess, the narrator would very much like to be invisible. Instead, he has been dragged out into the light, because you (and the inner voices you pick up along the way), are starting to argue with him. And he doesn’t like it.
Despite his claims that the princess will lie and cheat in order to escape, as the game progresses it’s clear that the narrator is every bit as manipulative–if not moreso, because he actually knows what’s going on. And, if the player tries to diverge from the path that he’s set before them, the correct path, then it rapidly becomes clear that he, at least to start, has the power to force that correct path.
While this is very much a narrative device, the act of calling attention to the narrator is important beyond that context.
The Hero’s Journey is the true monomyth, something to which all stories can be reduced. It doesn’t matter that the author, Joseph Campbell, was a raging misogynist whose framework flattened cultures and stories to fit a western lens [9, 10]. It was used in Star Wars, so clearly it’s a universal framework.
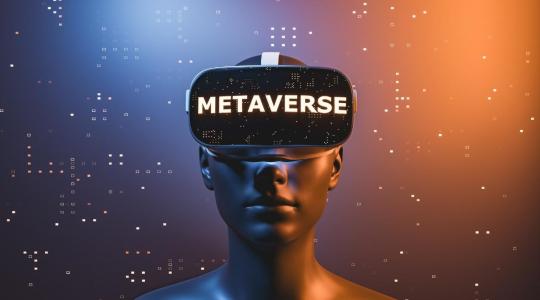
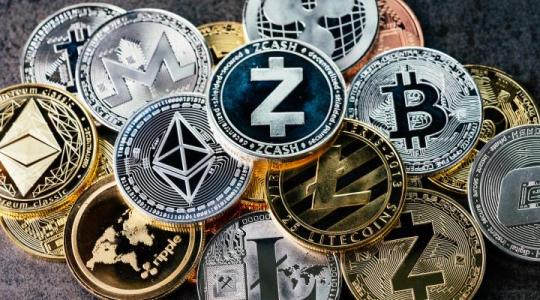

The metaverse will soon replace the real world and crypto is the future of currency! Never mind that the organizations pushing it are suspiciously pyramid shaped. Get on board or be left behind.
Generative AI is pushed as the next big thing. The harms it inflicts on creatives and the harmful stereotypes it perpetuates are just bugs in the system. Never mind that the evangelists for this technology speak over the concerns of marginalized people [5]. That’s a skill issue, you gotta keep up.
Computers will eventually, likely soon, advance so far as to replace humans altogether. The robot uprising is on the horizon [8].
Who perpetuates these stories? What do they have to gain?
Why is the only story for the future replications of unjust systems of power? Why must the hero always slay the monster?
Because so says the narrator. And so long as they are invisible, it is simple to assume that this is simply the way things are.

Chapter III: The End...?
This is the part where Slay the Princess starts feeling like a stretch, but I’ve already killed the horse so I might as well beat it until the end too.
Because what is the end result here?
According to the game… collapse. A recursive story whose biases narrow the scope of each iteration ultimately collapses in on itself. The princess becomes so sharp that she is nothing but blades to eviscerate you. The princess becomes so perfect a damsel that she is a caricature of the trope. The story whittles itself away to nothing. And then the cycle begins anew.

There’s no climactic final battle with the narrator. He created this box, set things in motion, but he is beyond the player’s reach to confront directly. The only way out is to become aware of the box itself, and the agenda of the narrator. It requires acknowledgement of the artificiality of the roles thrust upon you and the Princess, the false dichotomy of hero or villain.
Slay the Princess doesn’t actually provide an answer to what lies outside of the box, merely acknowledges it as a limit that can be overcome.

With regards to the less fanciful narratives that comprise our day-to-day lives, it’s difficult to see the boxes and dichotomies we’ve been forced into, let alone what might be beyond them. But if the limit placed is that there are no stories that can exist outside of capitalism, outside of colonialism, outside of rigid hierarchies and oppressive structures, then that limit can be broken [12].

Denouement: Doomed by the Narrative
Video games are an interesting artistic medium, due to their inherent interactivity. The commonly accepted mechanics of the medium, such as flavor text that provides in-game information and commentary, are an excellent example of an invisible narrator. Branching dialogue trees and multiple endings can help obscure this further, giving the player a sense of genuine agency… which provides an interesting opportunity to drag an invisible narrator into the light.
There are a number of games that have explored the power differential between the narrator and the player (The Stanley Parable, Little Misfortune, Undertale, Buddy.io, OneShot, etc…)
However, Slay the Princess works well here because it not only emphasizes the artificial limitations that the narrator sets on a story, but the way that these stories recursively loop in on themselves, reinforcing the fears and biases of previous iterations.
Critical data theory probably had nothing to do with the game’s development (Abby Howard if you're reading this, lmk). However, it works as a surprisingly cohesive framework for illustrating the ways that we can become ensnared by a narrative, and the importance of knowing who, exactly, is narrating the story. Although it is difficult or impossible to conceptualize what might exist beyond the artificial limits placed by even a well-intentioned narrator, calling attention to them and the box they’ve constructed is the first step in breaking out of this cycle.
“You can't go around building a better world for people. Only people can build a better world for people. Otherwise it's just a cage.” ― Terry Pratchett, Witches Abroad

Epilogue
If you've read this far, thank you for your time! This was an adaptation of my final presentation for a Critical Data Studies course. Truthfully, this course posed quite a challenge--I found the readings of philosophers such as Kant, Adorno, Foucault, etc... difficult to parse. More contemporary scholars were significantly more accessible. My only hope is that I haven't gravely misinterpreted the scholars and researchers whose work inspired this piece.
I honestly feel like this might have worked best as a video essay, but I don't know how to do those, and don't have the time to learn or the money to outsource.
Slay the Princess is available for purchase now on Steam.
Screencaps from ManBadassHero Let's Plays: [Part 1] [Part 2] [Part 3] [Part 4] [Part 5] [Part 6]
Post Dividers by @cafekitsune
Citations:
Rosenberg, D. (2018). Data as word. Historical Studies in the Natural Sciences, 48(5), 557-567.
Amaro, Ramon. (2019). As If. e-flux Architecture. Becoming Digital. https://www.e-flux.com/architecture/becoming-digital/248073/as-if/
What Ethical AI Really Means by PhilosophyTube
Keyes, O. (2018). The misgendering machines: Trans/HCI implications of automatic gender recognition. Proceedings of the ACM on human-computer interaction, 2(CSCW), 1-22.
Allred, A.M., Aragon, C. (2023). Art in the Machine: Value Misalignment and AI “Art”. In: Luo, Y. (eds) Cooperative Design, Visualization, and Engineering. CDVE 2023. Lecture Notes in Computer Science, vol 14166. Springer, Cham. https://doi.org/10.1007/978-3-031-43815-8_4
Amaro, R. (2019). Artificial Intelligence: warped, colorful forms and their unclear geometries.
Parisisi, L., Ferreira da Silva, D. Black Feminist Tools, Critique, and Techno-poethics. e-flux. Issue #123. https://www.e-flux.com/journal/123/436929/black-feminist-tools-critique-and-techno-poethics/
AI - Our Shiny New Robot King | Sophie from Mars by Sophie From Mars
Joseph Campbell and the Myth of the Monomyth | Part 1 by Maggie Mae Fish
Joseph Campbell and the N@zis | Part 2 by Maggie Mae Fish
How Barbie Cis-ified the Matrix by Jessie Gender
#slay the princess#stp spoilers#stp#stp princess#abby howard#black tabby games#academics#critical data studies#computer science#technology#hci#my academics#my writing#long post
245 notes
·
View notes
Text
Thom Wolf, the co founder of Hugging Face wrote this the other day about how the current AI is just “a country of yes men on servers”
History is filled with geniuses struggling during their studies. Edison was called "addled" by his teacher. Barbara McClintock got criticized for "weird thinking" before winning a Nobel Prize. Einstein failed his first attempt at the ETH Zurich entrance exam. And the list goes on.
The main mistake people usually make is thinking Newton or Einstein were just scaled-up good students, that a genius comes to life when you linearly extrapolate a top-10% student.
This perspective misses the most crucial aspect of science: the skill to ask the right questions and to challenge even what one has learned. A real science breakthrough is Copernicus proposing, against all the knowledge of his days -in ML terms we would say “despite all his training dataset”-, that the earth may orbit the sun rather than the other way around.
To create an Einstein in a data center, we don't just need a system that knows all the answers, but rather one that can ask questions nobody else has thought of or dared to ask. One that writes 'What if everyone is wrong about this?' when all textbooks, experts, and common knowledge suggest otherwise.
“In my opinion this is one of the reasons LLMs, while they already have all of humanity's knowledge in memory, haven't generated any new knowledge by connecting previously unrelated facts. They're mostly doing "manifold filling" at the moment - filling in the interpolation gaps between what humans already know, somehow treating knowledge as an intangible fabric of reality.”
29 notes
·
View notes
Text
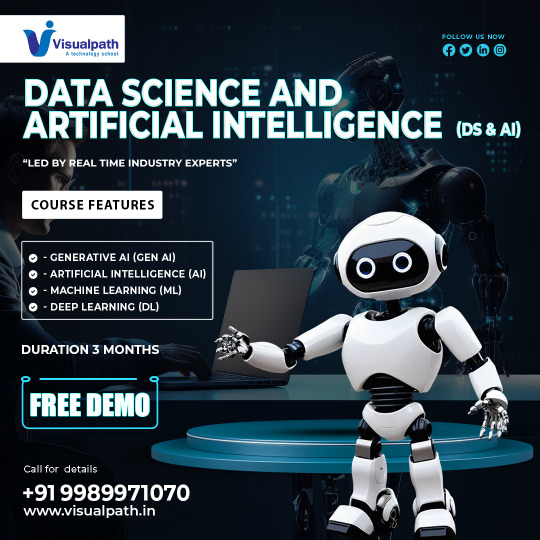
Data Science With Generative Ai Online Training | Generative Ai
Visualpath Offering Data Science With Generative Ai Online Training. elevate your career in data science. Our comprehensive Data Science With Generative Ai Combines advanced AI concepts and hands-on training to make you industry-ready. Enroll for a Free Demo. Call on: +91 9989971070
WhatsApp: https://www.whatsapp.com/catalog/919989971070/
Blog link: https://visualpathblogs.com/
Visit us: https://www.visualpath.in/online-data-science-with-generative-ai-course.html
#Data Science Course#Data Science Course In Hyderabad#Data Science Training In Hyderabad#Data Science With Generative Ai Course#Data Science Institutes In Hyderabad#Data Science With Generative Ai#Data Science With Generative Ai Online Training#Data Science With Generative Ai Course Hyderabad#Data Science With Generative Ai Training
0 notes
Text
watching alexander avilas new AI video and while:
I agree that we need more concrete data about the true amount of energy Generative AI uses as a lot of the data right now is fuzzy and utilities are using this fuzziness to their advantage to justify huge building of new data centers and energy infrastructure (I literally work in renewables lol so I see this at work every day)
I also agree that the copyright system sucks and that the lobbyist groups leveraging Generative AI as a scare tactic to strengthen it will probably ultimately be bad for artists.
I also also agree that trying to define consciousness or art in a concrete way specifically to exclude Generative AI art and writing will inevitably catch other artists or disabled people in its crossfire. (Whether I think the artists it would catch in the crossfire make good art is an entirely different subject haha)
I also also also agree that AI hype and fear mongering are both stupid and lump so many different aspects of growth in machine learning, neural network, and deep learning research together as to make "AI" a functionally useless term.
I don't agree with the idea that Generative AI should be a meaningful or driving part of any kind of societal shift. Or that it's even possible. The idea of a popular movement around this is so pie in the sky that it's actually sort of farcical to me. We've done this dance so many times before, what is at the base of these models is math and that math is determined by data, and we are so far from both an ethical/consent based way of extracting that data, but also from this data being in any way representative.
The problem with data science, as my data science professor said in university, is that it's 95% data cleaning and analyzing the potential gaps or biases in this data, but nobody wants to do data cleaning, because it's not very exciting or ego boosting, and the amount of human labor it would to do that on a scale that would train a generative AI LLM is frankly extremely implausible.
Beyond that, I think ascribing too much value to these tools is a huge mistake. If you want to train a model on your own art and have it use that data to generate new images or text, be my guest, but I just think that people on both sides fall into the trap of ascribing too much value to generative AI technologies just because they are novel.
Finally, just because we don't know the full scope of the energy use of these technologies and that it might be lower than we expected does not mean we get a free pass to continue to engage in immoderate energy use and data center building, which was already a problem before AI broke onto the scene.
(also, I think Avila is too enamoured with post-modernism and leans on it too much but I'm not academically inclined enough to justify this opinion eloquently)
16 notes
·
View notes
Text
you can train and run LLMs and image generation models on a laptop. data center electricity usage is due to it being a data center, not having “AI” deployed—it would be like looking at the electricity usage of cloudflare and all of its clients and deciding “this is all AI usage”
copyright is fake and even if you are worried about that, you can use license models from giant media companies like Adobe or Getty that own 100% of their training data.
the only genuine complaint about “AI” is that it can displace workers in media industries, but any new technology has the potential to do that and lobbying for banning technology has never really worked. only thing you can really do and should do is adapt and unionize
like yeah it is annoying that every company is pushing “AI” and that it is inescapable, but how is that the fault of the technology? a software that can predict things based on given data is valuable to science and to art. the company that tries to sell you $10/month subscription to use software you can download onto your own computer for free is the stupid part.
7 notes
·
View notes
Text
AI & Data Science Course Transparent Fee at NeuAI Labs
Know the investment in your future. Explore NeuAI Labs' course fees for Artificial Intelligence and Data Science, ensuring a transparent and valuable learning experience.
#ai fee#data science fees#free ai course#free data science course#ai course in pune#generative ai classes#generative ai course#data science course#data science certification#data science training#neuailabs#futureofai
0 notes
Note
I think you're confusing (or more like generalising i guess) ai with generative ai. Which is something a lot of people are doing. As a computer science graduate, I agree that generative AI is bullshit. It's used to scam people, a lot of people use chat gpt and literally stop thinking on their own, and you're right that using it to create art, of all the things, is lazy af. And it uses a lot of water and energy, yes. And I'm very much against it, too. when i hear someone at work talk about using chat gpt, i roll my eyes, because yikes. But it's important to note that the generative AI is only a branch of all AI. You can have ai without stealing other people's work and data, you can have your own datasets to train your model to do whatever you need. Ai can be good in medicine (eg detecting cancer cells) or extracting text from images (like scanned documents) or categorising data from big datasets. And it's only 3 examples out of many many more. The generative ai tools became a thing within the last 2-3 years. Ai was being developed and used for years before that with a lot of hopes about it. My point is, not all ai is bad but the big companies made the general population think that ai = chat gpt. And chat gpt, while technically having a potential for being a great tool, is based on stolen data, uses too many resources and basically makes people more stupid. So yeah, when you get angry about the use of ai, get angry, but not at ai as a whole but at generative ai and those who push it (aka the big companies who get more rich from brainwashing people)
good point. i went for generative AI without even specifying beyond my examples because that's the AI we're talking about, that's the AI Adobe and Opera are pushing via sponsorships as well.
i was thinking about medicine but i don't have anything to back it up, so i didn't mention it. also, there are cons in AI used in medicine too. there are cons in using AI in general. so, is it "all good"? no. frankly, not "all bad" either. so, fair point. i'm sure some engineering is also using AI, but i don't have examples of its performance.
7 notes
·
View notes
Text
The Coprophagic AI crisis

I'm on tour with my new, nationally bestselling novel The Bezzle! Catch me in TORONTO on Mar 22, then with LAURA POITRAS in NYC on Mar 24, then Anaheim, and more!
A key requirement for being a science fiction writer without losing your mind is the ability to distinguish between science fiction (futuristic thought experiments) and predictions. SF writers who lack this trait come to fancy themselves fortune-tellers who SEE! THE! FUTURE!
The thing is, sf writers cheat. We palm cards in order to set up pulp adventure stories that let us indulge our thought experiments. These palmed cards – say, faster-than-light drives or time-machines – are narrative devices, not scientifically grounded proposals.
Historically, the fact that some people – both writers and readers – couldn't tell the difference wasn't all that important, because people who fell prey to the sf-as-prophecy delusion didn't have the power to re-orient our society around their mistaken beliefs. But with the rise and rise of sf-obsessed tech billionaires who keep trying to invent the torment nexus, sf writers are starting to be more vocal about distinguishing between our made-up funny stories and predictions (AKA "cyberpunk is a warning, not a suggestion"):
https://www.antipope.org/charlie/blog-static/2023/11/dont-create-the-torment-nexus.html
In that spirit, I'd like to point to how one of sf's most frequently palmed cards has become a commonplace of the AI crowd. That sleight of hand is: "add enough compute and the computer will wake up." This is a shopworn cliche of sf, the idea that once a computer matches the human brain for "complexity" or "power" (or some other simple-seeming but profoundly nebulous metric), the computer will become conscious. Think of "Mike" in Heinlein's *The Moon Is a Harsh Mistress":
https://en.wikipedia.org/wiki/The_Moon_Is_a_Harsh_Mistress#Plot
For people inflating the current AI hype bubble, this idea that making the AI "more powerful" will correct its defects is key. Whenever an AI "hallucinates" in a way that seems to disqualify it from the high-value applications that justify the torrent of investment in the field, boosters say, "Sure, the AI isn't good enough…yet. But once we shovel an order of magnitude more training data into the hopper, we'll solve that, because (as everyone knows) making the computer 'more powerful' solves the AI problem":
https://locusmag.com/2023/12/commentary-cory-doctorow-what-kind-of-bubble-is-ai/
As the lawyers say, this "cites facts not in evidence." But let's stipulate that it's true for a moment. If all we need to make the AI better is more training data, is that something we can count on? Consider the problem of "botshit," Andre Spicer and co's very useful coinage describing "inaccurate or fabricated content" shat out at scale by AIs:
https://papers.ssrn.com/sol3/papers.cfm?abstract_id=4678265
"Botshit" was coined last December, but the internet is already drowning in it. Desperate people, confronted with an economy modeled on a high-speed game of musical chairs in which the opportunities for a decent livelihood grow ever scarcer, are being scammed into generating mountains of botshit in the hopes of securing the elusive "passive income":
https://pluralistic.net/2024/01/15/passive-income-brainworms/#four-hour-work-week
Botshit can be produced at a scale and velocity that beggars the imagination. Consider that Amazon has had to cap the number of self-published "books" an author can submit to a mere three books per day:
https://www.theguardian.com/books/2023/sep/20/amazon-restricts-authors-from-self-publishing-more-than-three-books-a-day-after-ai-concerns
As the web becomes an anaerobic lagoon for botshit, the quantum of human-generated "content" in any internet core sample is dwindling to homeopathic levels. Even sources considered to be nominally high-quality, from Cnet articles to legal briefs, are contaminated with botshit:
https://theconversation.com/ai-is-creating-fake-legal-cases-and-making-its-way-into-real-courtrooms-with-disastrous-results-225080
Ironically, AI companies are setting themselves up for this problem. Google and Microsoft's full-court press for "AI powered search" imagines a future for the web in which search-engines stop returning links to web-pages, and instead summarize their content. The question is, why the fuck would anyone write the web if the only "person" who can find what they write is an AI's crawler, which ingests the writing for its own training, but has no interest in steering readers to see what you've written? If AI search ever becomes a thing, the open web will become an AI CAFO and search crawlers will increasingly end up imbibing the contents of its manure lagoon.
This problem has been a long time coming. Just over a year ago, Jathan Sadowski coined the term "Habsburg AI" to describe a model trained on the output of another model:
https://twitter.com/jathansadowski/status/1625245803211272194
There's a certain intuitive case for this being a bad idea, akin to feeding cows a slurry made of the diseased brains of other cows:
https://www.cdc.gov/prions/bse/index.html
But "The Curse of Recursion: Training on Generated Data Makes Models Forget," a recent paper, goes beyond the ick factor of AI that is fed on botshit and delves into the mathematical consequences of AI coprophagia:
https://arxiv.org/abs/2305.17493
Co-author Ross Anderson summarizes the finding neatly: "using model-generated content in training causes irreversible defects":
https://www.lightbluetouchpaper.org/2023/06/06/will-gpt-models-choke-on-their-own-exhaust/
Which is all to say: even if you accept the mystical proposition that more training data "solves" the AI problems that constitute total unsuitability for high-value applications that justify the trillions in valuation analysts are touting, that training data is going to be ever-more elusive.
What's more, while the proposition that "more training data will linearly improve the quality of AI predictions" is a mere article of faith, "training an AI on the output of another AI makes it exponentially worse" is a matter of fact.
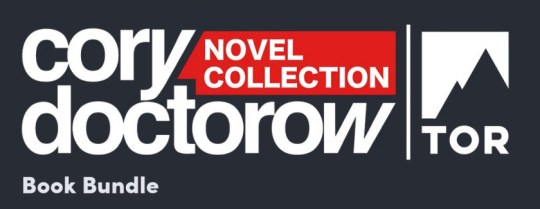
Name your price for 18 of my DRM-free ebooks and support the Electronic Frontier Foundation with the Humble Cory Doctorow Bundle.
If you'd like an essay-formatted version of this post to read or share, here's a link to it on pluralistic.net, my surveillance-free, ad-free, tracker-free blog:
https://pluralistic.net/2024/03/14/14/inhuman-centipede#enshittibottification
Image: Plamenart (modified) https://commons.wikimedia.org/wiki/File:Double_Mobius_Strip.JPG
CC BY-SA 4.0 https://creativecommons.org/licenses/by-sa/4.0/deed.en
#pluralistic#ai#generative ai#André Spicer#botshit#habsburg ai#jathan sadowski#ross anderson#inhuman centipede#science fiction#mysticism
557 notes
·
View notes