#Linear Regression Online Help
Explore tagged Tumblr posts
Note
Agree people and do whatever they want with the pictures but the same people cropping the picture are also hoping that next week episodes will have Tommy refusing to engage in pda with Buck when he sees Gerard so they can realise they’re not meant for each other. Incredibly gross to want racist sexist bigot to have the power which is essentially what that would be accomplishing, all bc you don’t like the pairing. When you take that into consideration the cropping definitely isn’t just a random non issue
okay so. hm how do i want to say this because now you’ve framed it in a way that makes any counter argument bigoted.
i’ll just reiterate something i’ve said before that was met with a lot of agreement: the 911 fandom has a habit of seeing an opinion or fandom take that they disagree with and instead of moving past it or having a conversation about it, they decide they have to find a way to make it a complete moral failure to have this opinion so that it’s acceptable to “other” those who have it.
let’s be clear, there’s nothing wrong with the speculation that tommy might have a little regression in the presence of someone who held an abusive position of power over him for many years. that would be a totally normal thing to have happen because growth is not linear (might i remind you that is a CENTRAL thesis of the very show you are watching and was the HEAVY main theme in the very episode you just watched). this could or could not happen and it could or could not lead to the end of buck and tommy. there is nothing about this potential— i want to reiterate that this hasn’t even happened in canon and you are arguing about made up scenarios in peoples minds— storyline. it is not “giving back the power” to a bigot, its a story.
anon, i’m going to say this with love and hope that it can help you learn to curate your online experience in a way that doesn’t stress you out so bad you feel the need to jump in my ask box about it ever again: use the block button, and use it generously. if someone posts speculation you think is annoying, block them. if someone crops an actor out of a photo: block them. you don’t have to justify your feelings of anger by making up a reason that this person is secretly a bigoted freak, you just need to block them.
#i try to stay out of discourse and put up a mostly kind and nice front#but this is bullshit and you know it is. so fuck off with your virtue signaling ass tomfoolery and do something that matters#911 abc#main tagging cus why the fuck not let’s party
10 notes
·
View notes
Text
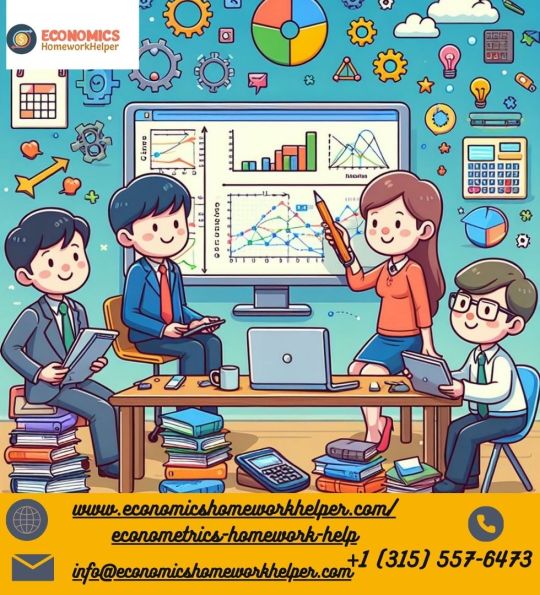
Econometrics Demystified: The Ultimate Compilation of Top 10 Study Aids
Welcome to the world of econometrics, where economic theories meet statistical methods to analyze and interpret data. If you're a student navigating through the complexities of econometrics, you know how challenging it can be to grasp the intricacies of this field. Fear not! This blog is your ultimate guide to the top 10 study aids that will demystify econometrics and make your academic journey smoother.
Economicshomeworkhelper.com – Your Go-To Destination
Let's kick off our list with the go-to destination for all your econometrics homework and exam needs – https://www.economicshomeworkhelper.com/. With a team of experienced experts, this website is dedicated to providing high-quality assistance tailored to your specific requirements. Whether you're struggling with regression analysis or hypothesis testing, the experts at Economicshomeworkhelper.com have got you covered. When in doubt, remember to visit the website and say, "Write My Econometrics Homework."
Econometrics Homework Help: Unraveling the Basics
Before delving into the intricacies, it's crucial to build a strong foundation in the basics of econometrics. Websites offering econometrics homework help, such as Khan Academy and Coursera, provide comprehensive video tutorials and interactive lessons to help you grasp fundamental concepts like linear regression, correlation, and statistical inference.
The Econometrics Academy: Online Courses for In-Depth Learning
For those seeking a more immersive learning experience, The Econometrics Academy offers online courses that cover a wide range of econometrics topics. These courses, often led by seasoned professors, provide in-depth insights into advanced econometric methods, ensuring you gain a deeper understanding of the subject.
"Mastering Metrics" by Joshua D. Angrist and Jörn-Steffen Pischke
No compilation of study aids would be complete without mentioning authoritative books, and "Mastering Metrics" is a must-read for econometrics enthusiasts. Authored by two renowned economists, Joshua D. Angrist and Jörn-Steffen Pischke, this book breaks down complex concepts into digestible chapters, making it an invaluable resource for both beginners and advanced learners.
Econometrics Forums: Join the Conversation
Engaging in discussions with fellow econometrics students and professionals can enhance your understanding of the subject. Platforms like Econometrics Stack Exchange and Reddit's econometrics community provide a space for asking questions, sharing insights, and gaining valuable perspectives. Don't hesitate to join the conversation and expand your econometrics network.
Gretl: Your Free Econometrics Software
Practical application is key in econometrics, and Gretl is the perfect tool for hands-on learning. This free and open-source software allows you to perform a wide range of econometric analyses, from simple regressions to advanced time-series modeling. Download Gretl and take your econometrics skills to the next level.
Econometrics Journal Articles: Stay Updated
Staying abreast of the latest developments in econometrics is essential for academic success. Explore journals such as the "Journal of Econometrics" and "Econometrica" to access cutting-edge research and gain insights from scholars in the field. Reading journal articles not only enriches your knowledge but also equips you with the latest methodologies and approaches.
Econometrics Bloggers: Learn from the Pros
Numerous econometrics bloggers share their expertise and experiences online, offering valuable insights and practical tips. Follow blogs like "The Unassuming Economist" and "Econometrics by Simulation" to benefit from the expertise of professionals who simplify complex econometric concepts through real-world examples and applications.
Econometrics Software Manuals: Master the Tools
While software like STATA, R, and Python are indispensable for econometric analysis, navigating through them can be challenging. Refer to comprehensive manuals and documentation provided by these software platforms to master their functionalities. Understanding the tools at your disposal will empower you to apply econometric techniques with confidence.
Econometrics Webinars and Workshops: Continuous Learning
Finally, take advantage of webinars and workshops hosted by academic institutions and industry experts. These events provide opportunities to deepen your knowledge, ask questions, and engage with professionals in the field. Check out platforms like Econometric Society and DataCamp for upcoming events tailored to econometrics enthusiasts.
Conclusion
Embarking on your econometrics journey doesn't have to be daunting. With the right study aids, you can demystify the complexities of this field and excel in your academic pursuits. Remember to leverage online resources, engage with the econometrics community, and seek assistance when needed. And when the workload becomes overwhelming, don't hesitate to visit Economicshomeworkhelper.com and say, "Write My Econometrics Homework" – your trusted partner in mastering econometrics. Happy studying!
13 notes
·
View notes
Text
I was standing outside my high school waiting to get picked up when I opened Instagram and saw the news, I thought it was a joke. Genuinely. Cut to me looking at @harryflorals on Instagram and all of my other fan accounts I follow to see if it was true. It was. My heart immediately felt heavier than it had in years. I am still filled with grief and shock. I feel for his son, Bear, who woke up without a father. I feel for his entire family, who is feeling one of the most complex, nuanced, and heavy feelings someone can experience: grief. I can’t imagine how the other boys are feeling. Grieving someone you have lost contact with or have bad memories with is so, so so hard and I know that feeling personally. Grief is devastating, it is confusing, it is paralyzing, it is all-consuming. When you’re a kid, in your mind the people you admire or look up to are immortal. They’re on a pedestal and therefore they cannot die. Especially if they are someone who, from a young age, was commodified and molded to be an object you could sell. It is shocking to be so abruptly brought out of that fantasy. I imagined being 50, having my children or my wife say “Did you hear (insert member here) died?”. I never expected to have to deal with this kind of grief until very later in life. I never thought I would be 17, scrolling through Tumblr and Instagram, trying to make sense of one of them dying so, so, so devastatingly young.
My second-grade teacher got me into One Direction, and over that year, I became a massive fan. I brought the boys up in every conversation—anyone who knew me back then can attest to that—and made them my whole personality. Sometime during the end of the second grade, they announced that they would be going on hiatus. I remember feeling numb and in shock, I had just gotten into them. When their History music video came out, my second-grade teacher, whom I still talk to, promised to premiere it on her projector at the end of class. I remember sobbing, my best friend at the time and I were holding each other. I was mourning, and grieving, and processing. That was a big thing for an 8-year-old to go through. What other loss does she know?
While having a family member be sick at home and eventually passing in the sixth grade, I regressed to my 8 year old self. I made One Direction my entire personality again. I lost friends because they “just didn’t understand it” (aka: they were sick of hearing me only talk about them). I would listen to One Direction all day at school, and read fanfiction on the school bus and before I fell asleep. I would watch any media I could get my hands on that involved them. They were my support, and my rock to fall back on during that hardship. After this family member passed away in March of 2020, just a week before lockdown happened, One Direction became even more of a support. I felt isolated and lonely but the online community and the fandom helped me feel like I had a place, and I know I’ll always be thankful for that of course. As I healed and found other coping mechanisms, I still kept them incredibly dear to my heart, leaning on them whenever I needed to.
Once the news broke about Liam's passing and everything that followed, it felt like everyone was coming together to mourn. People who have been active consistently for years, people who have been a bit more inactive (like me), and even people who got a platform from this community (Sarah Baska, Brittany Broski, etc). The community is the strongest it has been in awhile right now. We are all going through something so linear, and so complicated. We didn’t know him, but he was a part of all of our childhoods. I still have all of my old merch I’ve collected over the years. I look to my right and I see the throw pillow and body pillow that are tucked underneath my bed. I see my One Direction stuffed bear and my poster and the old, untouched electric toothbrush I thrifted when I was 12. I may not as big of a fan actively as I once was, but this grief still runs deep. He was infinitely too young and it hurts to think that he felt that way to do something like that.
To everyone who is also feeling this grief, I see you. You are not being dramatic. Your grief and mourning are completely valid.
#one direction#rip liam payne#liam payne#essay#I’m mourning so bad#thank you to the girl who pre read it#this is so devastating
3 notes
·
View notes
Text
How do I learn R, Python and data science?
Learning R, Python, and Data Science: A Comprehensive Guide
Choosing the Right Language
R vs. Python: Both R and Python are very powerful tools for doing data science. R is usually preferred for doing statistical analysis and data visualisations, whereas Python is much more general and currently is very popular for machine learning and general-purpose programming. Your choice of which language to learn should consider your specific goals and preferences.
Building a Strong Foundation
Structured Courses Online Courses and Tutorials: Coursera, edX, and Lejhro offer courses and tutorials in R and Python for data science. Look out for courses that develop theoretical knowledge with practical exercises. Practise your skills with hands-on coding challenges using accompanying datasets, offered on websites like Kaggle and DataCamp.
Books: There are enough books to learn R and Python for data science. You may go through the classical ones: "R for Data Science" by Hadley Wickham, and "Python for Data Analysis" by Wes McKinney.
Learning Data Science Concepts
Statistics: Know basic statistical concepts: probability, distribution, hypothesis testing, and regression analysis.
Cleaning and Preprocessing: Learn how to handle missing data techniques, outliers, and data normalisation.
Data Visualization: Expert libraries to provide informative visualisations, including but not limited to Matplotlib and Seaborn in Python and ggplot2 in R.
Machine Learning: Learn algorithms-Linear Regression, Logistic Regression, Decision Trees, Random Forest, Neural Networks, etc.
Deep Learning: Study deep neural network architecture and how to build and train them using the frameworks TensorFlow and PyTorch.
Practical Experience
Personal Projects: In this, you apply your knowledge to personal projects which help in building a portfolio.
Kaggle Competitions: Participate in Kaggle competitions to solve real-world problems in data science and learn from others.
Contributions to Open-Source Projects: Contribute to some open-source projects for data science in order to gain experience and work with other people.
Other Advice
Join Online Communities: Join online forums or communities such as Stack Overflow and Reddit to ask questions, get help, and connect with other data scientists.
Attend Conferences and Meetups: This is a fantastic way to network with similar working professionals in the field and know the latest trends going on in the industry.
Practice Regularly: For becoming proficient in data science, consistent practice is an indispensable element. Devote some time each day for practising coding challenges or personal projects.
This can be achieved by following the above-mentioned steps and having a little bit of dedication towards learning R, Python, and Data Science.
2 notes
·
View notes
Text
From Math to Machine Learning: A Comprehensive Blueprint for Aspiring Data Scientists
The realm of data science is vast and dynamic, offering a plethora of opportunities for those willing to dive into the world of numbers, algorithms, and insights. If you're new to data science and unsure where to start, fear not! This step-by-step guide will navigate you through the foundational concepts and essential skills to kickstart your journey in this exciting field. Choosing the Best Data Science Institute can further accelerate your journey into this thriving industry.
1. Establish a Strong Foundation in Mathematics and Statistics
Before delving into the specifics of data science, ensure you have a robust foundation in mathematics and statistics. Brush up on concepts like algebra, calculus, probability, and statistical inference. Online platforms such as Khan Academy and Coursera offer excellent resources for reinforcing these fundamental skills.
2. Learn Programming Languages
Data science is synonymous with coding. Choose a programming language – Python and R are popular choices – and become proficient in it. Platforms like Codecademy, DataCamp, and W3Schools provide interactive courses to help you get started on your coding journey.
3. Grasp the Basics of Data Manipulation and Analysis
Understanding how to work with data is at the core of data science. Familiarize yourself with libraries like Pandas in Python or data frames in R. Learn about data structures, and explore techniques for cleaning and preprocessing data. Utilize real-world datasets from platforms like Kaggle for hands-on practice.
4. Dive into Data Visualization
Data visualization is a powerful tool for conveying insights. Learn how to create compelling visualizations using tools like Matplotlib and Seaborn in Python, or ggplot2 in R. Effectively communicating data findings is a crucial aspect of a data scientist's role.
5. Explore Machine Learning Fundamentals
Begin your journey into machine learning by understanding the basics. Grasp concepts like supervised and unsupervised learning, classification, regression, and key algorithms such as linear regression and decision trees. Platforms like scikit-learn in Python offer practical, hands-on experience.
6. Delve into Big Data Technologies
As data scales, so does the need for technologies that can handle large datasets. Familiarize yourself with big data technologies, particularly Apache Hadoop and Apache Spark. Platforms like Cloudera and Databricks provide tutorials suitable for beginners.
7. Enroll in Online Courses and Specializations
Structured learning paths are invaluable for beginners. Enroll in online courses and specializations tailored for data science novices. Platforms like Coursera ("Data Science and Machine Learning Bootcamp with R/Python") and edX ("Introduction to Data Science") offer comprehensive learning opportunities.
8. Build Practical Projects
Apply your newfound knowledge by working on practical projects. Analyze datasets, implement machine learning models, and solve real-world problems. Platforms like Kaggle provide a collaborative space for participating in data science competitions and showcasing your skills to the community.
9. Join Data Science Communities
Engaging with the data science community is a key aspect of your learning journey. Participate in discussions on platforms like Stack Overflow, explore communities on Reddit (r/datascience), and connect with professionals on LinkedIn. Networking can provide valuable insights and support.
10. Continuous Learning and Specialization
Data science is a field that evolves rapidly. Embrace continuous learning and explore specialized areas based on your interests. Dive into natural language processing, computer vision, or reinforcement learning as you progress and discover your passion within the broader data science landscape.
Remember, your journey in data science is a continuous process of learning, application, and growth. Seek guidance from online forums, contribute to discussions, and build a portfolio that showcases your projects. Choosing the best Data Science Courses in Chennai is a crucial step in acquiring the necessary expertise for a successful career in the evolving landscape of data science. With dedication and a systematic approach, you'll find yourself progressing steadily in the fascinating world of data science. Good luck on your journey!
3 notes
·
View notes
Text
Machine Learning Course for Beginners: A Comprehensive Guide to Getting Started
What is Machine Learning?
At its core, machine learning (ML) is a subset of artificial intelligence (AI) that enables computers to learn from data and make decisions or predictions without being explicitly programmed for each task. Instead of coding every rule, ML algorithms identify patterns and relationships within data to generate outcomes.
Why Enroll in an Online Machine Learning Course for Beginners?
Online courses offer flexibility, affordability, and access to expert instructors. A machine learning course online typically covers foundational topics such as supervised and unsupervised learning, data preprocessing, model evaluation, and common algorithms like linear regression, decision trees, and neural networks. These courses often include hands-on projects that reinforce learning and build your portfolio.
Key Topics Covered in a Beginner’s Machine Learning Course
Understanding Data and Features: Learning how to clean, preprocess, and select relevant features.
Supervised Learning: Techniques where models are trained on labeled data, such as classification and regression.
Unsupervised Learning: Methods like clustering and dimensionality reduction applied to unlabeled data.
Model Evaluation: Metrics like accuracy, precision, recall, and F1-score.
Overfitting and Underfitting: Strategies to improve model generalization.
Recommended Resources for Beginners
Several platforms offer beginner-friendly courses, including Coursera, edX, Udacity, and DataCamp. Many of these platforms provide free trials and introductory courses suitable for newcomers.
Practical Machine Learning Projects
To solidify your understanding, engaging in real-world projects is essential. Some popular machine learning projects for beginners include:
Iris Flower Classification: Using the classic Iris dataset to classify flower species.
Titanic Survival Prediction: Predicting passenger survival based on features like age, sex, and class.
Handwritten Digit Recognition: Using the MNIST dataset to recognize handwritten numbers.
Customer Churn Prediction: Analyzing customer data to predict churn rates.
These projects help you apply theoretical knowledge, learn to handle data, and fine-tune models.
AI Tutorial for Beginners: Understanding the Basics
Artificial Intelligence (AI) is a broader field that encompasses machine learning, natural language processing, computer vision, and more. For beginners, an AI tutorial for beginners provides a gentle introduction to these concepts, demystifying how machines can be taught to perform intelligent tasks.
What is AI?
AI involves creating systems that can perform tasks that typically require human intelligence, such as understanding language, recognizing images, making decisions, or solving problems.
Types of AI
Narrow AI: Systems designed for specific tasks (e.g., virtual assistants like Siri or Alexa).
General AI: Hypothetical systems with human-like intelligence (not yet realized).
How AI Relates to Machine Learning
While AI is the overarching field, machine learning is a subset focused on algorithms that learn from data. Many modern AI applications rely heavily on ML techniques, making understanding both essential.
Basic Concepts in AI
Natural Language Processing (NLP): Teaching machines to understand and generate human language.
Computer Vision: Enabling machines to interpret visual information.
Robotics: Designing intelligent robots capable of perception and action.
Getting Started with AI
Beginners can start with free tutorials, videos, and introductory courses that cover fundamental concepts, popular algorithms, and basic programming skills in Python or R.
Combining Learning: Online Machine Learning Course and Projects
Enrolling in a machine learning course for beginners is an excellent way to systematically learn the concepts, gain practical experience, and work on machine learning projects that enhance your skills. These projects often involve datasets from Kaggle or UCI Machine Learning Repository, providing real-world scenarios to apply your knowledge.
Why Practical Projects Matter
Projects help you understand data handling, feature engineering, model selection, and evaluation. They also build your portfolio, which is valuable when seeking internships or jobs in AI and ML.
Resources to Find Projects
Many online courses include project modules, and platforms like Kaggle host competitions suitable for beginners. Additionally, GitHub repositories often showcase beginner-friendly ML projects.
0 notes
Text
Intellipaat Course Review: My Journey From Novice to Data Scientist
Embarking on a Data Science Adventure
When I first decided to transition into the world of data science, I felt both excited and overwhelmed. As a tech professional with a background in software development, I knew I had a solid foundation, but the vast landscape of data science seemed daunting. After researching various online learning platforms, I stumbled upon Intellipaat Review of their Data Science course. Little did I know that this decision would change the trajectory of my career.
Why I Chose Intellipaat
Course Structure and Curriculum
One of the first things that caught my attention was Intellipaat's comprehensive curriculum. The course promised to cover everything from basic statistics to advanced machine learning algorithms. As someone who learns best with a structured approach, I appreciated the logical progression of topics.
Real-World Projects
What really sealed the deal for me was the emphasis on practical, real-world projects. Intellipaat's course offered hands-on experience with actual datasets, which I found crucial for bridging the gap between theory and application.
My Learning Experience
The Initial Challenges
I won't sugarcoat it – the first few weeks were tough. Coming from a programming background, I found the statistical concepts particularly challenging. However, Intellipaat's instructors were patient and always available to clarify doubts.
Breakthrough Moments
As I progressed through the course, I started to have those "aha!" moments. Concepts that once seemed abstract began to click into place. I remember the satisfaction of successfully implementing my first machine learning model – it was a simple linear regression, but it felt like a major achievement.
The Power of Community Learning
One unexpected benefit of the Intellipaat course was the vibrant community of fellow learners. Through discussion forums and group projects, I found myself collaborating with data enthusiasts from around the globe. This not only enhanced my learning but also helped me build a professional network.
Applying My Skills in the Real World
From Theory to Practice
As I neared the end of the course, I felt confident enough to apply for data science positions. The projects I had completed during the Intellipaat course became the cornerstone of my portfolio, demonstrating my newly acquired skills to potential employers.
Landing My Dream Job
Within a few months of completing the course, I secured a position as a junior data scientist at a tech startup. The skills I learned through Intellipaat – from data preprocessing to advanced visualization techniques – proved invaluable in my new role.
Final Thoughts on My Intellipaat Journey
Looking back, I can confidently say that enrolling in Intellipaat's Data Science course was one of the best data science course decisions I've made. The course not only equipped me with technical skills but also instilled the confidence to tackle complex data problems.
"Intellipaat didn't just teach me data science; it transformed me into a data scientist."
For any tech professional considering a move into data science, I wholeheartedly recommend giving Intellipaat a try. It's more than just an online course – it's a comprehensive learning experience that can truly launch your data science career.
0 notes
Text
IIT JAM Mathematical Statistics Exam Pattern 2026: Complete Guide for Success
The IIT JAM Mathematical Statistics exam is one of the most competitive postgraduate entrance tests in India for students who aspire to study in prestigious institutes like the IITs and IISc. As the competition grows stronger each year, a well-informed preparation strategy becomes critical. The first step in that direction is to understand the IIT JAM Mathematical Statistics Exam Pattern in detail.
This blog provides a complete and SEO-friendly overview of the IIT JAM 2026 Mathematical Statistics Exam Pattern. Whether you are starting early or in the revision phase, this guide will help you prepare smartly and effectively.
What is IIT JAM Mathematical Statistics?
The IIT JAM (Joint Admission Test for M.Sc.) is an all-India examination administered by various Indian Institutes of Technology (IITs) on a rotational basis. One of the key papers in this exam is Mathematical Statistics (MS), which is a combination of undergraduate-level mathematics and statistics. This paper is ideal for students from a statistics, mathematics, or economics background and is designed to test a candidate’s conceptual and problem-solving skills.
Before you begin preparing, it is essential to understand the structure of the IIT JAM Mathematical Statistics Exam Pattern 2026 so you can approach your study plan with precision.
IIT JAM 2026 Mathematical Statistics Exam Pattern
The IIT JAM 2026 Mathematical Statistics Exam Pattern consists of a single paper conducted in an online computer-based test format. The duration of the exam is three hours, and the total marks are 100.
The question paper is divided into three sections: Section A, Section B, and Section C. Each section contains different types of questions and follows specific marking schemes.
Section A consists of Multiple Choice Questions (MCQs). There are a total of 30 questions in this section. Ten questions carry one mark each, while the remaining 20 carry two marks each. This section has negative marking for incorrect answers—one-third mark is deducted for each wrong answer in the one-mark questions, and two-thirds mark is deducted for incorrect answers in the two-mark questions.
Section B includes Multiple Select Questions (MSQs). It contains 10 questions, each worth two marks. In this section, more than one option can be correct, and all correct options must be selected to score full marks. There is no negative marking in this section, but partial marks are not awarded.
Section C features Numerical Answer Type (NAT) questions. This section has 20 questions, 10 of which carry one mark each, and 10 carry two marks each. These questions require candidates to enter a numerical value as an answer. There is no negative marking for NAT questions.
Altogether, the paper has 60 questions across all three sections. The key to scoring well is not only knowing the subject but also mastering the types of questions and practicing a lot of mock tests.
Topics Covered in the IIT JAM Mathematical Statistics Exam
The IIT JAM Mathematical Statistics Exam Pattern 2026 covers both Mathematics and Statistics topics from the undergraduate level. Understanding the distribution and importance of each subject area can significantly impact your preparation.
The Mathematics portion includes topics such as sequences and series, calculus, matrices, linear algebra, and differential equations. These topics contribute to about 40% of the total questions in the exam.
The Statistics portion, which comprises the remaining 60%, includes topics like probability theory, random variables, distributions, estimation, hypothesis testing, and regression. These are more concept-driven and require strong analytical skills.
By analyzing the IIT JAM 2026 Mathematical Statistics Exam Pattern, it becomes evident that the paper tests both theoretical understanding and numerical problem-solving.
Why Understanding the Exam Pattern Is Important
Many aspirants jump straight into studying the syllabus without giving much thought to the IIT JAM Mathematical Statistics Exam Pattern. However, knowing the pattern offers several strategic advantages. It allows you to allocate time and effort based on the weightage of each section. It also helps you avoid penalties through careful planning, especially in sections that include negative marking.
Moreover, by understanding which sections carry no negative marking, such as MSQs and NATs, you can take calculated risks and improve your overall score. In a competitive exam like JAM, smart preparation makes all the difference.
Preparation Tips Based on the IIT JAM Mathematical Statistics Exam Pattern 2026
Start with a clear study plan based on the topics covered in both mathematics and statistics. Prioritize high-weightage topics and begin early to allow time for multiple revisions.
Focus more on conceptual clarity, especially in topics like probability, estimation theory, and regression. These are not only scoring areas but also feature prominently in the exam.
Practice previous year papers and mock tests that simulate the IIT JAM exam pattern. This will help you become familiar with question formats, time pressure, and overall difficulty level.
Improve your accuracy to avoid losing marks in Section A due to negative marking. In Sections B and C, where there's no penalty for wrong answers, ensure you attempt all questions after a careful evaluation.
Make use of shortcut techniques and formulae lists for revision. Create flashcards or mind maps to help you revise critical points in less time.
Stay updated with any notifications or changes in the IIT JAM Mathematical Statistics Exam Pattern 2026 released by the official organizing institute.
In conclusion, cracking the IIT JAM exam begins with understanding the IIT JAM Mathematical Statistics Exam Pattern. The paper is thoughtfully designed to test your comprehensive knowledge of mathematical and statistical concepts through multiple question formats. Each section requires a unique strategy, and consistent practice is the key to mastering them.
With the IIT JAM 2026 Mathematical Statistics Exam Pattern now clearly explained, you're better equipped to begin your preparation in the right direction. Combine your subject knowledge with a smart exam strategy, and you'll be on your way to securing a seat in one of India’s top institutions.
If you're looking for expert guidance, mock tests, and structured study material, consider enrolling in IFAS Online's IIT JAM Mathematical Statistics coaching program. Get access to expert faculty, personalized mentorship, and comprehensive preparation tools all in one place.
0 notes
Text
Mastering Data Science: A Roadmap for Beginners and Aspiring Professionals
Understanding the Foundation of Data Science
Data science has emerged as one of the most sought-after career paths in today’s digital economy. It combines statistics, computer science, and domain knowledge to extract meaningful insights from data. Before diving deep into complex topics, it’s crucial to understand the foundational concepts that shape this field. From data cleaning to basic data visualization techniques, beginners must grasp these essential skills. Additionally, programming languages like Python and R are the primary tools used by data scientists worldwide. Building a strong base in these languages can set the stage for more advanced learning. It’s also important to familiarize yourself with databases, as querying and manipulating data efficiently is a key skill in any data-driven role. Solidifying these basics ensures a smoother transition to more complex areas such as artificial intelligence and machine learning.
Machine Learning for Beginners: The Essential Guide
Once you have a solid foundation, the next logical step is to explore machine learning. Machine Learning for Beginners is an exciting journey filled with numerous algorithms and techniques designed to help computers learn from data. Beginners should start with supervised learning models like linear regression and decision trees before progressing to unsupervised learning and reinforcement learning. Understanding the mathematical intuition behind algorithms such as k-nearest neighbors (KNN) and support vector machines (SVM) can enhance your analytical skills significantly. Online resources, workshops, and hands-on projects are excellent ways to strengthen your knowledge. It’s also vital to practice with real-world datasets, as this will expose you to the challenges and nuances faced in actual data science projects. Remember, mastering machine learning is not just about memorizing algorithms but about understanding when and why to use them.
Interview Preparation for Data Scientists: Key Strategies
Entering the job market as a data scientist can be both thrilling and intimidating. Effective interview preparation for data scientists requires more than just technical knowledge; it demands strategic planning and soft skill development. Candidates should be prepared to tackle technical interviews that test their understanding of statistics, machine learning, and programming. Additionally, behavioral interviews are equally important, as companies seek individuals who can collaborate and communicate complex ideas clearly. Mock interviews, coding challenges, and portfolio projects can significantly boost your confidence. It is beneficial to review common interview questions, such as explaining the bias-variance tradeoff or detailing a machine learning project you have worked on. Networking with professionals and seeking mentorship opportunities can also open doors to valuable insights and career advice. A strong preparation strategy combines technical mastery with effective storytelling about your experiences.
Advancing Your Data Science Career Through Specialization
After entering the field, data scientists often find themselves gravitating towards specialized roles like machine learning engineer, data analyst, or AI researcher. Specializing allows professionals to deepen their expertise and stand out in a competitive job market. Those passionate about prediction models might specialize in machine learning, while others who enjoy working with big data might lean towards data engineering. Continuous learning is essential in this rapidly evolving field. Enrolling in advanced courses, attending industry conferences, and contributing to open-source projects can all accelerate your career growth. Furthermore, staying updated with the latest tools and technologies, such as cloud-based machine learning platforms and advanced data visualization libraries, can give you an edge. A proactive approach to career development ensures you remain adaptable and competitive, regardless of how the industry changes.
Conclusion: Your Gateway to Success in Data Science
The journey to becoming a successful data scientist is both challenging and rewarding. It requires a balance of technical knowledge, practical experience, and continuous learning. Building a strong foundation, mastering machine learning basics, strategically preparing for interviews, and eventually specializing in a niche area are all key steps toward achieving your career goals. For those seeking comprehensive resources to guide them through every phase of their journey, visiting finzebra.com offers access to valuable tools and insights tailored for aspiring data science professionals. By following a structured learning path and leveraging the right resources, anyone can transform their passion for data into a fulfilling career.
0 notes
Text
How AI Agents Learn and Adapt Over Time
Artificial Intelligence is no longer a static set of rules; it's becoming increasingly dynamic, capable of learning and adapting much like living organisms. This ability to evolve over time is a cornerstone of intelligent AI agents, allowing them to tackle complex tasks in ever-changing environments. But how exactly do these digital minds learn and adapt? Let's delve into the fascinating world of AI agent learning.
At the heart of an AI agent's learning process lie several key mechanisms:
1. Data, the Lifeblood of Learning:
Just as humans learn from experience, AI agents learn from data. The more relevant and diverse the data they are exposed to, the better they become at their designated tasks. This data can take various forms:
Labeled Data: This data comes with predefined answers or categories, used in supervised learning. For example, images of cats and dogs labeled accordingly help an AI agent learn to classify new images.
Unlabeled Data: This data lacks explicit labels and is used in unsupervised learning. An AI agent might analyze customer purchase patterns to discover natural groupings without prior knowledge of these groups.
Interaction Data: In scenarios like game playing or robotics, AI agents learn through direct interaction with their environment, receiving feedback (rewards or penalties) for their actions. This is the realm of reinforcement learning.
2. The Power of Algorithms:
Algorithms are the recipes that guide an AI agent's learning process. Different types of algorithms are suited for different tasks and learning paradigms:
Supervised Learning Algorithms: These include linear regression, logistic regression, support vector machines, decision trees, and neural networks. They learn to map inputs to outputs based on labeled training data.
Unsupervised Learning Algorithms: Techniques like clustering (e.g., k-means), dimensionality reduction (e.g., PCA), and association rule mining help AI agents find hidden structures and patterns in unlabeled data.
Reinforcement Learning Algorithms: Algorithms like Q-learning, Deep Q-Networks (DQNs), and policy gradient methods enable AI agents to learn optimal behaviors through trial and error, maximizing cumulative rewards in an environment.
3. The Feedback Loop: Guiding the Learning Process:
Learning isn't a one-way street. AI agents constantly receive feedback on their performance, which drives adaptation:
Error Signals: In supervised learning, the difference between the agent's prediction and the actual label (the error) is used to adjust the internal parameters of the model, gradually improving accuracy.
Reward Signals: In reinforcement learning, the agent receives positive or negative rewards based on its actions in the environment. This feedback guides the agent to learn actions that lead to higher cumulative rewards.
Validation and Testing: After training, the agent's performance is evaluated on unseen data (validation and test sets) to ensure it generalizes well and doesn't just memorize the training data (overfitting). Poor performance triggers further adjustments and retraining.
4. Adaptation Over Time: Continuous Learning:
The real magic happens when AI agents can adapt to new information and changing environments over time. This can occur through several mechanisms:
Online Learning: The AI agent continuously learns from new data streams as they arrive, allowing it to adapt to evolving patterns without requiring complete retraining from scratch. Think of a spam filter that gets better at identifying new types of spam as users mark emails.
Transfer Learning: Knowledge gained from solving one task is applied to a new but related task. For example, an AI trained to recognize cats might learn to recognize dogs more quickly with less new data.
Fine-tuning: A pre-trained AI model (which has learned general features from a large dataset) is further trained on a smaller, specific dataset to adapt it to a particular task. This is common in natural language processing and computer vision.
Lifelong Learning: The ambitious goal of enabling AI agents to continuously learn and retain knowledge across multiple tasks and over extended periods, mimicking human-like learning. This is an active area of research.
Examples in Action:
Recommendation Systems: Platforms like Netflix and Spotify learn your preferences over time based on your viewing/listening history and feedback (likes, dislikes, skips), adapting their recommendations to your evolving taste.
Autonomous Vehicles: Self-driving cars continuously learn from sensor data, adapting their driving behavior to different road conditions, traffic patterns, and unexpected events.
Chatbots: Modern chatbots learn from their interactions with users, improving their understanding of language, their ability to answer questions accurately, and their overall conversational flow.
Spam Filters: As mentioned earlier, spam filters adapt to new spam techniques by analyzing the characteristics of newly identified spam emails.
The Future of Adaptive AI:
The ability of AI agents to learn and adapt is crucial for building truly intelligent systems that can operate effectively in dynamic and unpredictable real-world scenarios. Future advancements will likely focus on:
More efficient and robust learning algorithms.
Developing AI agents that can learn with less data.
Improving the ability of AI to generalize knowledge across different tasks.
Creating AI agents with better reasoning and problem-solving capabilities.
Addressing challenges related to bias and fairness in continuously learning systems.
In conclusion, AI agents learn and adapt through a continuous cycle of data ingestion, algorithmic processing, feedback reception, and model adjustment. This ever-evolving nature is what makes them so powerful and promises a future where AI seamlessly integrates into our lives, constantly improving and adapting to our needs and the complexities of the world around us.
1 note
·
View note
Text
3 Reasons APOTAC is Perfect for Beginners in Data Science
In a world flooded with data, the ability to extract insights has become one of the most valuable skills. Whether you're a student, working professional, or someone planning a career switch, you've likely heard of data science—and how promising it is. But here's the catch: most people don’t know where to start.
That’s where APOTAC comes in.
APOTAC’s Data Science course has been a game-changer for countless beginners. It’s more than just another online course—it's a guided pathway from confusion to confidence. If you’re wondering whether it’s the right fit for you, here are 3 powerful reasons APOTAC is perfect for beginners.
✅ 1. No Prior Coding or Math Experience Required
Let’s be honest—terms like “Python,” “machine learning,” or “linear regression” can sound intimidating when you’re just starting. Many platforms assume you already have a tech background or are comfortable with programming. APOTAC doesn’t.
From the very first module, APOTAC teaches everything from scratch. You’ll begin by learning:
Basic Python programming (even if you've never coded before)
Fundamental math & statistics concepts simplified for real understanding
What data science actually is—with real-life use cases to make it relatable
Every concept is broken down with beginner-friendly explanations, hands-on examples, and quizzes that help reinforce your learning.
💬 "I came from a B.Com background and had never written a line of code. But APOTAC helped me not only understand the logic but also made me love coding!" – Anjali S., APOTAC Learner
🛠️ 2. Real-World Projects, Not Just Theory
Learning theory is good, but doing is better. Many beginners struggle because they watch tutorials but never apply their knowledge. APOTAC solves this by integrating hands-on projects from the very start.
Each topic is followed by real-world datasets and use cases like:
Predicting customer churn
Analyzing COVID-19 data
Building recommendation systems
Visualizing e-commerce data
You’ll work with tools that real data scientists use daily: 🖥️ Python | 📊 Pandas & NumPy | 📈 Matplotlib & Seaborn | 🤖 Scikit-learn | 🛢️ SQL
The best part? By the time you complete the course, you'll have a portfolio of 5–8 projects that you can proudly showcase to employers.
💬 "Every time I finished a project, I felt more confident. It wasn’t just code—it was solving actual problems, just like in a real job." – Mohan D., APOTAC Graduate
🧑🏫 3. Mentorship & Job-Focused Learning
What truly sets APOTAC apart is the support system. Most online courses leave you on your own. Not this one.
Every student is assigned a personal mentor who:
Answers doubts (even the small ones!)
Reviews your projects
Prepares you for interviews
Helps with resume building & portfolio polish
You also get access to:
Live doubt-clearing sessions
Mock interviews with experts
Career guidance and placement support
A vibrant student community to collaborate with
This kind of hand-holding and motivation is crucial for beginners. It’s like having someone guide you through a jungle—step by step, without letting you get lost.
Important Link
Python Course
Data Science Course
Data Analytics Course
AI Course
1 note
·
View note
Text
Artificial Intelligence and Machine Learning for Programmers
Artificial Intelligence (AI) and Machine Learning (ML) are transforming the software landscape. For programmers, learning these technologies can open doors to building intelligent systems, automating tasks, and solving real-world problems. This post will help you understand the basics of AI/ML and how to get started as a developer.
What is Artificial Intelligence (AI)?
AI is a broad field of computer science focused on building smart machines that can perform tasks that typically require human intelligence. These include decision-making, speech recognition, visual perception, and language understanding.
What is Machine Learning (ML)?
ML is a subset of AI that allows computers to learn from data without being explicitly programmed. The system improves its performance over time as it processes more data.
Key Concepts in Machine Learning
Supervised Learning: The model is trained on labeled data (e.g., email spam detection).
Unsupervised Learning: The model finds patterns in data without labels (e.g., customer segmentation).
Reinforcement Learning: The model learns by interacting with an environment and receiving feedback (e.g., game-playing agents).
Neural Networks: Algorithms inspired by the human brain, used in deep learning for complex tasks like image recognition.
Popular Tools and Libraries
Python: The most widely used programming language in AI/ML.
TensorFlow: A powerful framework developed by Google for building ML models.
PyTorch: A flexible and beginner-friendly deep learning library by Facebook.
scikit-learn: Great for classical ML algorithms like linear regression and decision trees.
Keras: A high-level API that runs on top of TensorFlow.
How Programmers Can Start with AI/ML
Learn the Basics of Python: If you haven’t already, master Python programming.
Understand Math Fundamentals: Brush up on linear algebra, statistics, and calculus.
Study Core ML Concepts: Learn about data preprocessing, model training, overfitting, and evaluation.
Take Online Courses: Try platforms like Coursera, edX, Udacity, and freeCodeCamp.
Work on Projects: Start with simple projects like house price prediction or digit recognition.
Explore Real Datasets: Use sources like Kaggle, UCI Machine Learning Repository, or Google Dataset Search.
Applications of AI/ML
Recommendation Systems (e.g., Netflix, Amazon)
Speech and Voice Assistants (e.g., Siri, Alexa)
Autonomous Vehicles
Healthcare Diagnostics
Financial Fraud Detection
Natural Language Processing (NLP) for chatbots and translators
Best Practices
Start small and build gradually.
Focus on clean, high-quality datasets.
Document and test your models like you do with any software project.
Stay up to date with research and new tools.
Join communities and competitions (like Kaggle).
Conclusion
AI and ML are revolutionizing the tech world, and programmers with these skills are in high demand. Whether you're building chatbots, recommendation engines, or smart automation, the possibilities are endless. Start learning today and become part of the future of intelligent software development!
0 notes
Text
Artificial Intelligence Course in Delhi: Everything You Need to Know Before You Enroll
Artificial Intelligence (AI) is not just a buzzword anymore—it’s a driving force behind innovation in every industry, from finance and healthcare to e-commerce and entertainment. As the demand for skilled AI professionals continues to rise, Delhi has emerged as a hotbed for high-quality AI education and career opportunities. If you're searching for a top-rated Artificial Intelligence course in Delhi, you're already on the right path toward future-proofing your career.
In this guide, we’ll explore why Delhi is a prime location to study AI, what a top-tier course should offer, and how to select the best training program to suit your goals.
Why Choose Delhi for an Artificial Intelligence Course?
1. India’s Capital of Opportunities
Delhi is not only the political capital but also a growing technological and innovation hub. With the presence of MNCs, government-backed AI projects, tech startups, and leading universities, the city offers ample scope for practical exposure and job opportunities post-course completion.
2. Expanding AI Ecosystem
From smart city projects and fintech solutions to defense tech and healthcare AI tools, Delhi is actively integrating artificial intelligence across sectors. This growth is fueling the demand for AI professionals who can develop, deploy, and maintain intelligent systems.
3. Access to Quality Education
Delhi houses several reputable institutes offering AI and ML courses—many of which combine academic rigor with real-world project experience. Whether you're a college student, working professional, or tech enthusiast, you’ll find programs tailored to your learning needs.
Who Should Take an AI Course in Delhi?
AI is a multidisciplinary field. Whether you're from a tech or non-tech background, you can pursue AI training if you have curiosity and commitment. Ideal candidates include:
Computer Science and Engineering Students
Data Analysts and Software Developers
Working Professionals in IT, Banking, Healthcare, or Marketing
Business Analysts & Product Managers
Entrepreneurs looking to automate or innovate their businesses
No prior AI experience is mandatory for beginner-level courses, but familiarity with mathematics (linear algebra, probability) and basic programming (especially Python) will give you an advantage.
What Does an Artificial Intelligence Course in Delhi Typically Cover?
Delhi, being one of India’s leading education and tech hubs, offers a wide range of Artificial Intelligence (AI) courses catering to students, working professionals, and tech enthusiasts. These courses are designed to provide both foundational knowledge and practical experience. Whether offered by universities, private institutes, or online platforms with Delhi-based mentorship, here's what an AI course typically includes:
1. Introduction to Artificial Intelligence
Most courses begin with an overview of what AI is, its evolution, and where it stands today. Topics generally include:
Definition and scope of AI
History and evolution of AI technologies
Real-world applications in healthcare, finance, e-commerce, and robotics
This section helps learners understand the potential and future of AI in various industries.
2. Mathematics and Programming Foundations
A strong grasp of mathematics is essential in AI. Courses usually cover:
Linear Algebra (matrices, vectors)
Probability and Statistics
Calculus basics (used in training models)
Additionally, learners are introduced to Python programming, which is the most commonly used language in AI, along with tools like Jupyter Notebooks, NumPy, and Pandas.
3. Machine Learning Techniques
Machine Learning (ML), a subset of AI, forms the core of most courses. Key topics include:
Supervised and Unsupervised Learning
Regression and Classification
Clustering and Decision Trees
Model evaluation metrics
Many courses use hands-on projects to reinforce learning with tools like Scikit-learn, TensorFlow, or Keras.
4. Deep Learning and Neural Networks
Advanced AI courses in Delhi typically dive into:
Artificial Neural Networks (ANN)
Convolutional Neural Networks (CNN) for image processing
Recurrent Neural Networks (RNN) for sequence data
Natural Language Processing (NLP) using tools like spaCy and NLTK
These modules help students build intelligent systems capable of handling speech, images, and text.
What Makes a Good AI Course in Delhi?
When choosing an Artificial Intelligence course in Delhi, ensure the program offers these five essentials:
1. Industry-Relevant Curriculum
The AI landscape evolves rapidly. Make sure the course includes Generative AI, LLMs (like GPT), MLOps, and recent advances in computer vision or reinforcement learning.
2. Project-Based Learning
You should build a minimum of 5–7 projects, such as:
AI Chatbots for customer support
Fake news detection with NLP
Image classification using CNNs
Resume screening automation
Voice-enabled virtual assistants
3. Experienced Faculty
Look for trainers with real-world AI experience. Faculty involved in AI startups or research labs add immense value beyond textbook teaching.
4. Certification with Credibility
Courses that offer certifications recognized by industry or affiliated with global tech firms stand out to recruiters.
5. Placement Support
Some institutions in Delhi offer dedicated career services, resume polishing, mock interviews, and job referrals across companies in Gurugram, Noida, and Delhi.
Career Scope After Completing an AI Course in Delhi
Delhi, being one of the top tech hubs in India, is witnessing a growing demand for professionals skilled in Artificial Intelligence (AI). With major industries adopting AI to streamline operations and enhance services, completing an AI course in Delhi opens up various career paths. From software development to research, here’s an overview of the career scope available after completing an AI course in Delhi.
1. Machine Learning Engineer
Machine Learning (ML) Engineers are in high demand across tech companies, financial institutions, and e-commerce firms. These professionals build AI systems that enable computers to learn from data and make decisions. With Delhi’s thriving tech ecosystem, including startups and large enterprises, ML Engineers can find roles at companies like Microsoft, Amazon, and local tech startups.
2. Data Scientist
Data Scientists combine statistical analysis, machine learning, and data visualization techniques to extract insights and support decision-making. As businesses in Delhi, especially in finance, healthcare, and retail, rely heavily on data, skilled Data Scientists are highly sought after. Roles at top firms like Accenture, IBM, and Adobe offer lucrative opportunities.
3. AI Research Scientist
AI Research Scientists push the boundaries of existing AI technologies by creating innovative algorithms and improving current models. This role is ideal for individuals passionate about research and development. Delhi, with its prestigious educational institutions like IIT Delhi and research labs, provides ample opportunities for AI researchers, particularly in fields like computer vision, natural language processing (NLP), and robotics.
4. Business Intelligence (BI) Analyst
BI Analysts in AI use machine learning techniques to analyze business data and provide actionable insights. They play a crucial role in helping businesses make data-driven decisions. With many multinational companies in Delhi’s commercial sectors, including finance and retail, the demand for AI-savvy BI Analysts is increasing.
5. AI/ML Consultant
AI/ML Consultants advise companies on how to integrate AI into their business models. From improving customer experiences to automating tasks, consultants use their AI expertise to design and implement solutions. With Delhi being home to numerous consulting firms and startups, the role of an AI/ML Consultant has great prospects.
6. AI Product Manager
AI Product Managers oversee the development of AI-powered products from ideation to launch. They work closely with developers, designers, and business teams to ensure that the product aligns with market needs. Delhi’s growing startup ecosystem, especially in fintech and edtech, requires AI Product Managers to drive innovation.
Final Thoughts
Taking anArtificial Intelligence course in Delhi is more than just upskilling—it’s a strategic move toward becoming part of a future-ready workforce. With its world-class infrastructure, dynamic tech environment, and academic credibility, Delhi offers everything you need to succeed in AI.
Whether you're starting out or transitioning your career, Delhi has the mentorship, community, and opportunities to help you become an AI professional capable of solving real-world problems. The next generation of AI experts will not just use tools—they will build them. Your journey starts with the right course, in the right city.
#Artificial Intelligence Course in Delhi#Machine Learning Course in Delhi#Artificial Intelligence Classroom Course in Delhi
0 notes
Text
Unleashing Potential: Your Guide to Machine Learning Through Engaging Online Classes
In today's rapidly evolving technological landscape, the demand for skilled professionals in the field of machine learning is surging. This is not just a trend; it’s a paradigm shift that is reshaping industries across the globe. If you’re keen to enter this exciting field, harnessing the power of online resources can be a game-changer. This article will guide you through the essentials of machine learning lessons online, exploring the best online classes, courses designed specifically for beginners, and the benefits of hands-on learning through live projects.
Understanding Machine Learning: The New Frontier
Machine learning is a subset of artificial intelligence that focuses on the development of algorithms that allow computers to learn from and make predictions based on data. This technology powers numerous applications, from recommendation systems to autonomous vehicles. As businesses strive to tap into the full potential of data, there is an ongoing need for professionals who can effectively harness machine learning techniques.
Many aspiring data scientists and machine learning engineers are turning to online education to gain the necessary skills. Online classes provide flexibility, accessibility, and the opportunity to learn at your own pace, making them ideal for busy professionals or students.
The Rise of Machine Learning Lessons Online
Online platforms have begun offering structured machine learning lessons that cater to a diverse audience. These lessons cover a variety of topics, including fundamental concepts, statistical methods, and machine learning algorithms. Unlike traditional education models, online learning environments often combine theoretical knowledge with practical sessions.
With countless platforms available, learners can find lessons tailored to their specific interests, skill levels, and career aspirations. Websites like Styrishai are among those paving the way for comprehensive online education, making it easier to learn about machine learning without the constraints of time and location.
Exploring Machine Learning Online Classes
When choosing a suitable machine learning online class, several factors should be taken into consideration. The curriculum quality, teaching methodology, duration, and student support systems can significantly influence the learning experience.
Top-tier online classes typically feature a systematic approach, beginning with the basics and gradually progressing to advanced topics. Some notable components to look for in these classes include:
Interactive Content: Engaging video lectures, quizzes, and forums help maintain student interest and facilitate interaction.
Instructor Support: The availability of experienced instructors for doubt clarification and guidance is crucial.
Community: Access to a vibrant community for networking with peers can enhance the learning experience and provide invaluable insights.
Machine learning online classes can range from short courses designed to provide a quick overview to extensive programs that offer in-depth knowledge and skills covering various aspects of the field.
A Tailored Path: The Machine Learning Course for Beginners
For beginners, diving into the world of machine learning can be daunting. However, a well-structured machine learning course for beginners can serve as a roadmap to understanding core principles and applications. These courses often begin with foundational topics such as:
Introduction to machine learning concepts and classifications,
Data preprocessing techniques,
Basic algorithms including linear regression, decision trees, and neural networks.
Many courses also provide practical exercises, allowing learners to apply their knowledge to real datasets. By gradually increasing complexity, beginners can develop confidence in their abilities and gain critical insights into how to solve real-world problems using machine learning.
Hands-On Experience: Engaging with Machine Learning Live Projects
One of the most effective ways to reinforce theoretical knowledge is through practical application. Machine learning live projects offer students a vital opportunity to work on real challenges, transforming theoretical concepts into practical skills. This hands-on experience can significantly enhance a learner's portfolio, making them more attractive to potential employers.
Engaging in live projects typically includes:
Collaboration: Working in teams on projects allows students to share knowledge and learn from each other.
Feedback: Instant feedback from instructors can guide learners and enhance their problem-solving skills.
Portfolio Development: Successfully completing projects contributes to a learner's portfolio, showcasing their
abilities to future employers.
Live projects can cover a wide range of topics, from predicting stock market trends to creating recommendation systems for e-commerce platforms. This not only solidifies learning but also provides a sense of accomplishment as students see the practical implications of their work.
The Future of Learning: Combining Theory with Practice
With the landscape of education continually evolving, the shift toward online learning is a testament to technology’s potential in enhancing education quality. The rise of machine learning is transforming industries, and hence, equipping oneself with appropriate skills is essential not just for career advancement but for thriving in a fast-paced world.
By participating in machine learning lessons online, enrolling in well-structured online classes, undertaking machine learning courses tailored for beginners, and engaging with live projects, learners can fully embrace the opportunities presented by this dynamic field.
Conclusion
Embarking on your machine learning journey can be both exciting and challenging. Whether you’re a complete novice or someone looking to upgrade your skills, there are numerous resources available to help you navigate the world of machine learning effectively. Styrishai and other platforms provide essential online classes, comprehensive courses, and real-world projects that pave the way for a bright future in machine learning.
0 notes
Text
Best Machine Learning Classes in Pune Your Guide to Becoming a Data Science Expert with Ethans Tech
In today’s data-driven world, machine learning classes in Pune have become increasingly popular for students and professionals alike. Pune, known as the “Oxford of the East,” offers a thriving tech ecosystem with numerous institutes providing top-notch training in machine learning. Whether you're a beginner looking to start your career or an experienced professional aiming to upskill, enrolling in the right course is crucial.
Why Choose Pune for Machine Learning Classes?
Pune’s booming IT industry, combined with its strong educational infrastructure, makes it an ideal location for pursuing machine learning classes in Pune. The city is home to numerous tech companies, startups, and educational institutions, ensuring ample opportunities for learning and career growth.
Moreover, Pune's vibrant tech community offers numerous meetups, hackathons, and workshops that enhance practical knowledge and networking opportunities.
Key Concepts Covered in Machine Learning Classes
Most reputable institutes offering machine learning classes in Pune focus on the following essential topics:
1. Introduction to Machine Learning
Understanding supervised, unsupervised, and reinforcement learning
Key algorithms like linear regression, decision trees, and k-nearest neighbors
2. Data Preprocessing and Analysis
Handling missing data, feature scaling, and encoding categorical variables
Exploratory data analysis (EDA) using Python libraries like Pandas and Matplotlib
3. Model Training and Evaluation
Building models using frameworks like Scikit-learn, TensorFlow, and PyTorch
Techniques such as cross-validation, hyperparameter tuning, and model evaluation metrics
4. Deep Learning and Neural Networks
Understanding artificial neural networks (ANN), convolutional neural networks (CNN), and recurrent neural networks (RNN)
5. Real-World Projects
Hands-on projects are crucial in machine learning classes in Pune to apply theoretical knowledge. Institutes often provide case studies and practical applications in fields like healthcare, finance, and e-commerce.
What to Look for in a Machine Learning Institute in Pune
When selecting the best training institute, consider the following factors:
Experienced Faculty: Instructors with industry experience provide valuable insights and guidance.
Practical Learning Approach: Institutes offering hands-on projects, assignments, and real-world datasets ensure better understanding.
Placement Support: Look for institutes with strong industry connections and dedicated placement assistance.
Flexible Learning Options: Institutes offering both online and offline classes provide greater flexibility for working professionals.
For more courses - https://ethans.co.in/course/machine-learning-training-in-pune/
Top Skills You Will Gain from Machine Learning Classes
Enrolling in professional machine learning classes in Pune helps you develop a wide range of skills, including:
Strong programming knowledge in Python or R
Data manipulation and visualization skills
Expertise in machine learning algorithms and model evaluation
Experience with tools like Jupyter Notebook, TensorFlow, and Scikit-learn
Problem-solving abilities through real-world case studies
Career Opportunities After Completing Machine Learning Classes
Machine learning professionals are in high demand across various industries. Upon completing your training, you can explore roles such as:
Data Scientist
Machine Learning Engineer
AI Specialist
Business Intelligence Analyst
Data Analyst
The thriving tech industry in Pune ensures ample job opportunities for trained professionals, making it an ideal place to pursue your education.
Conclusion
If you’re looking for comprehensive machine learning classes in Pune, Ethans Tech is your go-to institute. Known for its expert trainers, hands-on learning approach, and impressive placement track record, Ethans Tech offers one of the best learning experiences for aspiring machine learning professionals.
Enrolling in the right training program will empower you with the skills required to excel in the competitive field of machine learning. Take the first step toward a rewarding career by joining a reputed institute in Pune today!
1 note
·
View note
Text
The Importance of Statistics and Mathematics in Data Analytics
At the heart of data analytics lies statistics and mathematics. These two disciplines provide the foundation for interpreting data, identifying patterns, and making data-driven decisions. While tools like SQL and Python help analysts manipulate and visualize data, it is statistical and mathematical principles that allow them to extract meaningful insights from the best Data Analytics Online Training.
Without a strong understanding of statistical concepts, data analysis becomes superficial, lacking the depth needed to make informed business decisions. In this blog, we will explore the critical role of statistics and mathematics in data analytics and why every aspiring data analyst should master these subjects.
Key Statistical Concepts for Data Analysts
Statistics helps analysts summarize data, detect trends, and validate hypotheses. Descriptive statistics, such as mean, median, mode, and standard deviation, provide a basic understanding of data distributions. Inferential statistics, including hypothesis testing, confidence intervals, and regression analysis, allow analysts to make predictions and draw conclusions from samples. Probability theory plays a crucial role in risk assessment, helping businesses make strategic decisions based on likely outcomes. If you want to learn more about Data Analytics, consider enrolling in an Best Online Training & Placement programs . They often offer certifications, mentorship, and job placement opportunities to support your learning journey.
Mathematics in Data Analytics
Mathematics is equally important in data analytics, as it provides the framework for performing calculations and transformations on data. Linear algebra is used in machine learning and data manipulation, while calculus is crucial for optimization problems and forecasting. Analysts working in finance and supply chain management often use mathematical techniques like linear programming to optimize resource allocation and predictive modeling for trend forecasting.
Applications of Statistics and Mathematics in Business Decisions
Businesses rely on statistical models to forecast sales, optimize marketing strategies, and assess operational performance. Regression analysis helps companies understand the relationship between different factors, such as how advertising spend affects sales. A/B testing, a statistical experiment, allows businesses to test different strategies and implement data-driven improvements. Probability models assist in risk assessment, such as predicting customer churn and fraud detection in financial transactions.
Conclusion
Statistics and mathematics are the backbone of data analytics, enabling analysts to draw meaningful conclusions from raw data. Without these skills, data analysts may struggle to validate their findings and make accurate predictions. Companies depend on data-driven insights for strategic decision-making, making statistical and mathematical knowledge invaluable. By mastering key concepts like probability, regression, and optimization, data analysts can enhance their ability to solve complex business problems. Investing time in learning these fundamentals will strengthen an analyst’s expertise and increase their career opportunities.
0 notes