#datascience
Explore tagged Tumblr posts
Text
I got a bee in my bonnet and spent last night crunching these numbers to confirm a long-held suspicion of mine, and now I'm going to do something with them even if it's only interesting to me. So.
I went through and tallied up all of the fics AO3 currently (as of 3/27/24) has under the tags "Trans Tim Drake," "Nonbinary Tim Drake," "Genderfluid Tim Drake" and "Genderqueer Tim Drake," since I figured that cast a wide enough net without committing myself to reading every fic vaguely tagged Trans Character to figure out which character they were talking about.
I then did the same for Dick, Jason, Damian and Bruce and, after comparing those numbers against each other and against the total number of fics each character has under their general tag, followed up with Duke, Babs, Cass, Steph and Kate, and then Kon, Cassie, and Bart for good measure.
The results confirm the suspicions I was going into check and are really interesting, to me at least:
Despite having far fewer stories overall than Jason, Bruce or Dick, Tim has by far the most stories tagging him under the trans umbrella (653 out of 58,395) and is the only member of the Bats for whom at least one full percent of his stories fall under that category (1.12% to be exact.) He actually has more total trans stories than Jason and Damian combined (308 out of 71,120 and 255 out of 42,607, equaling 0.43% and 0.59%, respectively) and outstretches the 2nd place ranker, Dick, by over a hundred (who clocks in at 438 out of 79,057 -- 0.55%). Bruce amusingly has by far the most stories overall (90,305) but the fewest trans stories (185) for the lowest percentage among the boys (0.2%).
The only one who comes anywhere close to matching Tim percentage-wise is Bart, who has far fewer stories to his name but a ratio of 62 out of 5,717 for 1.08%. I was thinking maybe Young Justice might have a higher percentage than the Bats due to their strong queer fandom but that only really proved true for Bart, with both Cassie and Kon coming in at only 0.2% and 0.28% trans umbrella percentage respectively (actual count 6 out of 2,874 and 39 out of 13,746).
Cassie's numbers correspond with the fact that women just, do not get a lot of these stories, at all, even compared to the general lack of attention they're paid by fanfiction spheres in general. Steph and Kate both clocked in at falling 0.17% under the trans umbrella (29 out of 16,638 for Steph, 5 out of 2,897 for Kate); Cass got 0.13% (21 out of 15,769) and Babs only 0.07%, the lowest percentage out of anyone I calculated for (11 out of 15,785). Duke's showing was a respectable 0.55% (34 out of 6,166) which puts him about even with the rest of the boys.
All of which I just went through to confirm a gut instinct I've had for a while: even in light of the noticeable trend in fandom towards increased visibility for trans and other queer-gendered people over the last decade and a half or so, it's a notable Thing for the DC comics fandom to explore with Tim Drake in specific.
And that doesn't even take into account things like the over 200 "Tim Drake is Catlad | Stray" fics, which almost always have some element of queered gender or at least femme'd sexuality to them, far outstripping any of the other Robin boys' spins in that AU (those counts stand at, respectively: Damian - 11, Dick - 33, Jason - 79, Tim - 242). Or the 11 fics logged under the "Tim Drake is Batgirl" tag, a category that doesn't even exist for any of the other male Robins.
(What makes that last one extra hilarious to me that most people don't know one canonical version of Tim has been a member of the Batgirls.) Part of me wants to use that parenthetic detail as a segway to ramble about the various canon snippets I think probably contributed to this, from Tim being presented as "the pretty one" who most often gets the "looks like his mother" comments to the fact that he is the only male Robin who's ever cross-dressed for an undercover mission and even though it only happened once the Internet will never forget Caroline Hill.
But this post is long enough as it is and I don't really have a point beyond I think this is interesting and cool so I'm going to leave off here for now and put my numbers under a cut so people have the raw data to look at if they'd like to.
TL;DR - Based on the numbers, the internet believes Tim Drake is more likely to be trans than any other member of the Bat-family or Young Justice, and I think that has interesting implications about his character and fandom. It's neat.
Data Taken: 3/27/24
Tim Drake: 58,395 Trans Tim Drake: 513 Nonbinary Tim Drake: 46 Genderfluid Tim Drake: 89 Genderqueer Tim Drake: 5
Dick Grayson: 79,057 Trans Dick Grayson: 399 Nonbinary Dick Grayson: 15 Genderfluid Dick Grayson: 23 Genderqueer Dick Grayson: 1
Jason Todd: 71,120 Trans Jason Todd: 286 Nonbinary Jason Todd: 17 Genderqueer/Genderfluid Jason Todd: 5 (4 have both tags and are the only ones tagged Genderqueer Jason Todd)
Damian Wayne: 42,607 Trans Damian Wayne: 215 Nonbinary Damian Wayne: 37 Genderfluid Damian Wayne: 3 Genderqueer Damian Wayne: 0
Bruce Wayne: 90,305 Trans Bruce Wayne: 180 Nonbinary Bruce Wayne: 5 (2 also tagged Trans Bruce Wayne) Genderfluid Bruce Wayne: 1 Genderqueer Bruce Wayne: 1
-
Total Trans Umbrella Tim Drake: 653 Total Trans Umbrella Dick Grayson: 438 Total Trans Umbrella Jason Todd: 308 (313 if you count the GQ tag separately) Total Trans Umbrella Damian Wayne: 255 Total Trans Umbrella Bruce Wayne: 185 (187)
Percentage Trans Umbrella Tim Drake: 1.12% (1.11825) Percentage Trans Umbrella Dick Grayson: 0.55% (0.55403) Percentage Trans Umbrella Jason Todd: 0.43% (0.43307 or 0.44010) Percentage Trans Umbrella Damian Wayne: 0.59% (0.59849) Percentage Trans Umbrella Bruce Wayne: 0.2% (0.20466)
----
Duke Thomas: 6,166 Trans Duke Thomas: 20 Nonbinary Duke Thomas: 14 Genderfluid Duke Thomas: 0 Genderqueer Duke Thomas: 0
Barbara Gordon: 15,785 Trans Barbara Gordon: 11 Nonbinary Barbara Gordon: 0 Genderfluid Barbara Gordon: 0 Genderqueer Barbara Gordon: 0
Cassandra Cain: 15,769 Trans Cassandra Cain: 15 Nonbinary Cassandra Cain: 6 Genderfluid Cassandra Cain: 0 Genderqueer Cassandra Cain: 0
Stephanie Brown: 16,638 Trans Stephanie Brown: 27 Nonbinary Stephanie Brown: 2 Genderfluid Stephanie Brown: 0 Genderqueer Stephanie Brown: 0
Kate Kane (DCU): 2,897 Trans Kate Kane: 4 Nonbinary Kate Kane: 0 Genderfluid Kate Kane: 1 Genderqueer Kate Kane: 0
-
Total Trans Umbrella Duke Thomas: 34 Total Trans Umbrella Barbara Gordon: 11 Total Trans Umbrella Cassandra Cain: 21 Total Trans Umbrella Stephanie Brown: 29 Total Trans Umbrella Kate Kane: 5
Percentage Trans Umbrella Duke Thomas: 0.55% (0.55141) Percentage Trans Umbrella Barbara Gordon: 0.07% (0.06968) Percentage Trans Umbrella Cassandra Cain: 0.13% (0.13317) Percentage Trans Umbrella Stephanie Brown: 0.17% (0.17429) Percentage Trans Umbrella Kate Kane: 0.17% (0.17259)
----
Kon-El | Conner Kent: 13,746 Trans Kon-El | Conner Kent: 19 Nonbinary Kon-El | Conner Kent: 19 Genderfluid Kon-El | Conner Kent: 1 Genderqueer Kon-El | Conner Kent: 0
Bart Allen: 5,717 Trans Bart Allen: 40 Nonbinary Bart Allen: 20 Genderfluid Bart Allen: 1 Genderqueer Bart Allen: 1
Cassie Sandsmark: 2,874 Trans Cassie Sandsmark: 4 Nonbinary Cassie Sandsmark: 2 Genderfluid Cassie Sandsmark: 0 Genderqueer Cassie Sandsmark: 0
-
Total Trans Umbrella Kon-El: 39 Total Trans Umbrella Bart Allen: 62 Total Trans Umbrella Cassie Sandsmark: 6
Percentage Trans Umbrella Kon-El: 0.28% (0.28371) Percentage Trans Umbrella Bart Allen: 1.08% (1.08448) Percentage Trans Umbrella Cassie Sandsmark: 0.2% (0.20876)
#transgender#tim drake#dick grayson#jason todd#damian wayne#bruce wayne#statistics#fanfic#ao3#dc comics#batfamily#duke thomas#barbara gordon#cassandra cain#stephanie brown#kon-el#cassandra sandsmark#bart allen#batman#robin#genderqueer#queer gender#gender issues#genderfluid#nonbinary#data#datascience#maybe? this isn't usually my area of expertise I just suddenly got the urge to crunch numbers on this#and my brain would not let go#the results are really interesting though!
299 notes
·
View notes
Text

Trans Lady looking for a serious relationship 💋😘 how do I look
#dating#datin#trans dating#trans nsft#transfem#trans pride#datascience#transgender#date night#dating sim#transformers#traaaaaaannnnnnnnnns#transgirl#gay men#gay#gayhot#so hot and sexy#sexy babygirl#sexy chick#sexy and beautiful#sexy pose
27 notes
·
View notes
Text
A colleague has a student collecting data on bad handwriting, if you could take 2 minutes to decide between some 1s and 7s ✍🏻👀
23 notes
·
View notes
Text
AI exists and there's nothing any of us can do to change that.
If you have concerns about how AI is being/will be used the solution is not to abstain - it's to get involved.
Learn about it, practice utilising AI tools, understand it. Ignorance will not protect you, and putting your fingers in your ears going 'lalalala AI doesn't exist I don't acknowledge it' won't stop it from affecting your life.
The more the general population fears and misunderstands this technology, the less equipped they will be to resist its influence.
#ai#artificial intelligence#ai technology#tech#technology#singularity#futurism#datascience#data analytics#data harvesting#manipulation#civil rights#civil disobedience#ai discourse
168 notes
·
View notes
Text

one churro with chocolate sauce (๑ᵔ⤙ᵔ๑)
30 December 2024, Monday
Uni diaries II day 51/-
Mondays are low effort days. Today I-
Prayed 5/5
Had a nutritious breakfast but late lunch and no dinner
Attended 3 classes
Helped make club poster
Went printer servicing (need new cartridges)
Bought new earphone (•‿•)
Read for 1+ hour (Proven Guilty by Jim Butcher)
Walked 4.5k steps
Slept well (7 hours)
#altin posts#studyblr#studyspo#study motivation#study inspiration#study aesthetic#study#studying#study hard#datablr#statblr#stemblr#datascience#statistics
31 notes
·
View notes
Text
poll time! please share!
54 notes
·
View notes
Text
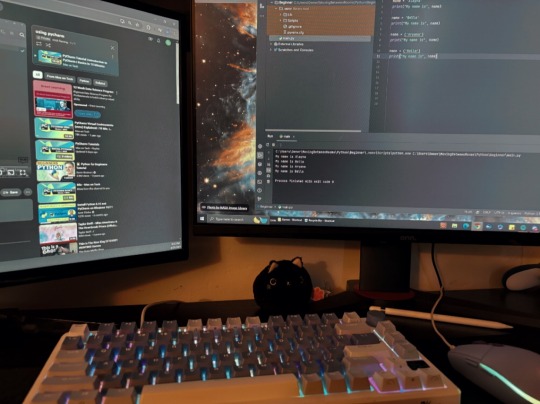
Hey everyone! enjoying my (two) week break of uni, so I've been lazy and playing games. Today, working on Python, I'm just doing repetition of learning the basics; Variables, Data types, Logic statements, etc. Hope everyone has a good week!
#codeblr#coding#python#university#uni life#studying#datascience#data analytics#data analysis#studyblr#student life#study motivation#study blog#student
77 notes
·
View notes
Text
It's #BiScienceFriday and today we're doing a deep-dive into some delicious data!
#lgbt#queer#bisexuality#bi#lgbtq#bi pride#lgbtqia#bi visibility#representationmatters#bisexual#lgbt pride#state of the union#bivisibility#research#data#datascience#data analytics#queer community
30 notes
·
View notes
Text
Hey stats or linguistics nerds!
(Explanation below)
Explanation:
Countable and singular means you hear “data” and think there’s exactly one thing in discussion. You would use it like “this data is an outlier, whereas that one is not.” Examples of this form: a cat, an artwork
Countable and plural means you hear “data” and think there’s more than one thing being talked about. You would use it like “these data show a positive trend, whereas those do not.” When discussing just one, you would use the term “datum”. Examples: cars, planets
Uncountable means you hear “data” and think that there’s some quantity of it, but it’s not necessarily a numbered amount, in a similar way to a fluid. You would use it like “this data doesn’t prove my hypothesis, but some of that data does.” Examples: water, air
Bias alert:
My father is a database engineer and has always used the word “data” as uncountable. I believe the “correct” way to use it is countable plural with the singular form being “datum”, though there’s no real answer because it’s linguistics and languages shift with use. That’s why I’m asking the people, in order to know how the term is used
24 notes
·
View notes
Text
#tua#the umbrella academy#klaus hargreeves#luthor hargreeves#diego hargreeves#allison hargreeves#five hargreeves#ben hargreeves#viktor hargreeves#victor hargreeves#pogo hargreeves#grace hargreeves#reginald hargreeves#statistic#statistics#datascience#data analytics#data analysis#data
20 notes
·
View notes
Text
Life update -
Hi, sorry for being MIA for a while and I'll try to update here more frequently. Here's a general update of what I've been up to.
Changed my Tumblr name from studywithmeblr to raptorstudiesstuff. Changed my blog name as well. I don't feel comfortable putting my real name on my social media platforms so I'm going by 'Raptor' now.
💻 Finished the Machine Learning-2 and Unsupervised Learning module along with projects. Got a pretty good grade in both of them and my overall grade went up a bit.
📝 Started applying for data science internships and jobs but got rejected from most of the companies I applied to... 😬
I'll start applying again in a week or two with a new resume. Let me know any tips I can use to not get rejected. 😅
💻 Started SQL last week and really enjoying it. I did get a bad grade on an assignment though. Hope I can make up for it in the final quiz. 🤞
🏥 Work has been alright. We're a little less staffed than usual this week but I'm trying not to stress too much about it.
📖 Currently reading Discworld #1 - The Color of Magic. More than halfway through.
📺 Re-watched the Lord of The Rings movies and now I'm compelled to read the books or rewatch the Hobbit movies.
"There's good in this world, Mr Frodo, and it's worth fighting for." This scene had me in tears and I really needed to hear that..
📺 Watched the first 4 episodes of First Kill on Netflix and I don't know what I was doing to myself. The writing and dialogue is so cheesy and terrible. The acting is okay-ish. It's so bad that it turned out to be quite hilarious. Laughed the whole time.
🎧 Discovered a new (for me) song that I'm obsessed with right now - Mirrors by Justin Timberlake.
📷 Took some really cool pics on my camera..





Might start the 100 days productivity challenge soon as that is the only way I find myself to be consistent.
Peace ✌️
Raptor
PS. Please don't repost any of my pictures without permission.
#study with me#study blog#studyblr#study motivation#study#study inspiration#student#100 days of productivity#student life#life update#update#raptor#photography#nature#original photographers#currently reading#reading#lotr#the hobbit#books and reading#books#tv shows#tv series#netflix#datascience#data analytics#machine learning#sql
8 notes
·
View notes
Text
Free online courses for bioinformatics beginners
🔬 Free Online Courses for Bioinformatics Beginners 🚀
Are you interested in bioinformatics but don’t know where to start? Whether you're from a biotechnology, biology, or computer science background, learning bioinformatics can open doors to exciting opportunities in genomics, drug discovery, and data science. And the best part? You can start for free!
Here’s a list of the best free online bioinformatics courses to kickstart your journey.
📌 1. Introduction to Bioinformatics – Coursera (University of Toronto)
📍 Platform: Coursera 🖥️ What You’ll Learn:
Basic biological data analysis
Algorithms used in genomics
Hands-on exercises with biological datasets
🎓 Why Take It? Ideal for beginners with a biology background looking to explore computational approaches.
📌 2. Bioinformatics for Beginners – Udemy (Free Course)
📍 Platform: Udemy 🖥️ What You’ll Learn:
Introduction to sequence analysis
Using BLAST for genomic comparisons
Basics of Python for bioinformatics
🎓 Why Take It? Short, beginner-friendly course with practical applications.
📌 3. EMBL-EBI Bioinformatics Training
📍 Platform: EMBL-EBI 🖥️ What You’ll Learn:
Genomic data handling
Transcriptomics and proteomics
Data visualization tools
🎓 Why Take It? High-quality training from one of the most reputable bioinformatics institutes in Europe.
📌 4. Introduction to Computational Biology – MIT OpenCourseWare
📍 Platform: MIT OCW 🖥️ What You’ll Learn:
Algorithms for DNA sequencing
Structural bioinformatics
Systems biology
🎓 Why Take It? A solid foundation for students interested in research-level computational biology.
📌 5. Bioinformatics Specialization – Coursera (UC San Diego)
📍 Platform: Coursera 🖥️ What You’ll Learn:
How bioinformatics algorithms work
Hands-on exercises in Python and Biopython
Real-world applications in genomics
🎓 Why Take It? A deep dive into computational tools, ideal for those wanting an in-depth understanding.
📌 6. Genomic Data Science – Harvard Online (edX) 🖥️ What You’ll Learn:
RNA sequencing and genome assembly
Data handling using R
Machine learning applications in genomics
🎓 Why Take It? Best for those interested in AI & big data applications in genomics.
📌 7. Bioinformatics Courses on BioPractify (100% Free)
📍 Platform: BioPractify 🖥️ What You’ll Learn:
Hands-on experience with real datasets
Python & R for bioinformatics
Molecular docking and drug discovery techniques
🎓 Why Take It? Learn from domain experts with real-world projects to enhance your skills.
🚀 Final Thoughts: Start Learning Today!
Bioinformatics is a game-changer in modern research and healthcare. Whether you're a biology student looking to upskill or a tech enthusiast diving into genomics, these free courses will give you a strong start.
📢 Which course are you excited to take? Let me know in the comments! 👇💬
#Bioinformatics#FreeCourses#Genomics#BiotechCareers#DataScience#ComputationalBiology#BioinformaticsTraining#MachineLearning#GenomeSequencing#BioinformaticsForBeginners#STEMEducation#OpenScience#LearningResources#PythonForBiologists#MolecularBiology
7 notes
·
View notes
Text

How do I look
#dating#trans dating#dating sim#trans pride#trans nsft#transgender#transfem#date night#datascience#datin#trans germany#transgirl#transg
22 notes
·
View notes
Text
8 fresh Chinese models with easy guides,
plus Europe’s surprising synergy
your AI approach might never look the same
#machinelearning#artificialintelligence#art#digitalart#mlart#datascience#ai#algorithm#deepseek#china ai
9 notes
·
View notes
Text

We innovate beyond expectations to deliver solutions that propel your #business forward. How are you planning to integrate AI & ML into your strategy? Let’s discuss how these technologies can help you stay ahead.
Let’s discuss: 👉🌐 https://www.pranathiss.com 👉📧 [email protected] 👉📲 +1 732 333 3037
#AI#MachineLearning#Innovation#TechSolutions#DataScience#BusinessGrowth#Automation#FutureOfWork#SmartTech#DigitalTransformation
7 notes
·
View notes