#MultimodalAi
Explore tagged Tumblr posts
Text
Learn how Aria, the open-source multimodal Mixture-of-Experts model, is revolutionizing AI. With a 64K token context and 3.9 billion parameters per token, Aria outperforms models like Llama3.2-11B and even rivals proprietary giants like GPT-4o mini. Discover its unique capabilities and architecture that make it a standout in AI technology.
#Aria#MultimodalAI#MixtureOfExperts#AI#MachineLearning#OpenSource#RhymesAI#open source#artificial intelligence#software engineering#nlp#machine learning#programming#python
4 notes
·
View notes
Text
Investment and M&A Trends in the Multimodal AI Market
Global Multimodal AI Market: Growth, Trends, and Forecasts for 2024-2034
The Global Multimodal AI Market is witnessing explosive growth, driven by advancements in artificial intelligence (AI) technologies and the increasing demand for systems capable of processing and interpreting diverse data types.
The Multimodal AI market is projected to grow at a compound annual growth rate (CAGR) of 35.8% from 2024 to 2034, reaching an estimated value of USD 8,976.43 million by 2034. In 2024, the market size is expected to be USD 1,442.69 million, signaling a promising future for this cutting-edge technology. In this blog, we will explore the key components, data modalities, industry applications, and regional trends that are shaping the growth of the Multimodal AI market.
Request Sample PDF Copy :https://wemarketresearch.com/reports/request-free-sample-pdf/multimodal-ai-market/1573
Key Components of the Multimodal AI Market
Software: The software segment of the multimodal AI market includes tools, platforms, and applications that enable the integration of different data types and processing techniques. This software can handle complex tasks like natural language processing (NLP), image recognition, and speech synthesis. As AI software continues to evolve, it is becoming more accessible to organizations across various industries.
Services: The services segment encompasses consulting, system integration, and maintenance services. These services help businesses deploy and optimize multimodal AI solutions. As organizations seek to leverage AI capabilities for competitive advantage, the demand for expert services in AI implementation and support is growing rapidly.
Multimodal AI Market by Data Modality
Image Data: The ability to process and understand image data is critical for sectors such as healthcare (medical imaging), retail (visual search), and automotive (autonomous vehicles). The integration of image data into multimodal AI systems is expected to drive significant market growth in the coming years.
Text Data: Text data is one of the most common data types used in AI systems, especially in applications involving natural language processing (NLP). Multimodal AI systems that combine text data with other modalities, such as speech or image data, are enabling advanced search engines, chatbots, and automated content generation tools.
Speech & Voice Data: The ability to process speech and voice data is a critical component of many AI applications, including virtual assistants, customer service bots, and voice-controlled devices. Multimodal AI systems that combine voice recognition with other modalities can create more accurate and interactive experiences.
Multimodal AI Market by Enterprise Size
Large Enterprises: Large enterprises are increasingly adopting multimodal AI technologies to streamline operations, improve customer interactions, and enhance decision-making. These companies often have the resources to invest in advanced AI systems and are well-positioned to leverage the benefits of integrating multiple data types into their processes.
Small and Medium Enterprises (SMEs): SMEs are gradually adopting multimodal AI as well, driven by the affordability of AI tools and the increasing availability of AI-as-a-service platforms. SMEs are using AI to enhance their customer service, optimize marketing strategies, and gain insights from diverse data sources without the need for extensive infrastructure.
Key Applications of Multimodal AI
Media & Entertainment: In the media and entertainment industry, multimodal AI is revolutionizing content creation, recommendation engines, and personalized marketing. AI systems that can process text, images, and video simultaneously allow for better content discovery, while AI-driven video editing tools are streamlining production processes.
Banking, Financial Services, and Insurance (BFSI): The BFSI sector is increasingly utilizing multimodal AI to improve customer service, detect fraud, and streamline operations. AI-powered chatbots, fraud detection systems, and risk management tools that combine speech, text, and image data are becoming integral to financial institutions’ strategies.
Automotive & Transportation: Autonomous vehicles are perhaps the most high-profile application of multimodal AI. These vehicles combine data from cameras, sensors, radar, and voice commands to make real-time driving decisions. Multimodal AI systems are also improving logistics and fleet management by optimizing routes and analyzing traffic patterns.
Gaming: The gaming industry is benefiting from multimodal AI in areas like player behavior prediction, personalized content recommendations, and interactive experiences. AI systems are enhancing immersive gameplay by combining visual, auditory, and textual data to create more realistic and engaging environments.
Regional Insights
North America: North America is a dominant player in the multimodal AI market, particularly in the U.S., which leads in AI research and innovation. The demand for multimodal AI is growing across industries such as healthcare, automotive, and IT, with major companies and startups investing heavily in AI technologies.
Europe: Europe is also seeing significant growth in the adoption of multimodal AI, driven by its strong automotive, healthcare, and financial sectors. The region is focused on ethical AI development and regulations, which is shaping how AI technologies are deployed.
Asia-Pacific: Asia-Pacific is expected to experience the highest growth rate in the multimodal AI market, fueled by rapid technological advancements in countries like China, Japan, and South Korea. The region’s strong focus on AI research and development, coupled with growing demand from industries such as automotive and gaming, is propelling market expansion.
Key Drivers of the Multimodal AI Market
Technological Advancements: Ongoing innovations in AI algorithms and hardware are enabling more efficient processing of multimodal data, driving the adoption of multimodal AI solutions across various sectors.
Demand for Automation: Companies are increasingly looking to automate processes, enhance customer experiences, and gain insights from diverse data sources, fueling demand for multimodal AI technologies.
Personalization and Customer Experience: Multimodal AI is enabling highly personalized experiences, particularly in media, healthcare, and retail. By analyzing multiple types of data, businesses can tailor products and services to individual preferences.
Conclusion
The Global Multimodal AI Market is set for Tremendous growth in the coming decade, with applications spanning industries like healthcare, automotive, entertainment, and finance. As AI technology continues to evolve, multimodal AI systems will become increasingly vital for businesses aiming to harness the full potential of data and automation. With a projected CAGR of 35.8%, the market will see a sharp rise in adoption, driven by advancements in AI software and services, as well as the growing demand for smarter, more efficient solutions across various sectors.
#MultimodalAI#MultimodalAIMarket#ArtificialIntelligence#AITrends#GenerativeAI#DeepLearning#AIMarketAnalysis#FutureOfAI#AIInnovation#AIApplications#AIForecast
0 notes
Text
Next-Gen AI: Beyond ChatGPT


Beyond ChatGPT: Exploring the Next Generation of Language Models
Next-Gen AI: Beyond ChatGPT has captured global attention with its ability to generate human-like responses, assist in creative writing, automate coding, and much more. But as impressive as it is, ChatGPT represents just one phase in the rapidly evolving world of artificial intelligence. The next generation of language models promises to go far beyond current capabilities, ushering in a new era of advanced reasoning, real-time interaction, and deeper understanding. From Language Models to Language Agents Next-gen models are not just designed to generate text; they're being trained to understand context, reason through problems, and interact autonomously with digital environments. This shift moves AI from being a passive responder (like ChatGPT) to an active problem-solver or AI agent—capable of performing tasks, managing workflows, and making decisions across complex systems. Key Advances Driving the Next Generation 1. Multimodal Capabilities Future models won’t be limited to text. Next-Gen AI: Beyond ChatGPT. They're being trained to interpret and respond to images, audio, video, and even code simultaneously. This makes them ideal for use in fields like medicine (interpreting scans), education (personalized learning), and design (generating visual content based on verbal cues). 2. Long-Term Memory and Personalization Newer models are being developed with persistent memory—enabling them to recall past conversations, user preferences, and personal contexts. This unlocks highly personalized assistance and smarter automation over time, unlike current models that operate in a single-session memory window. 3. Autonomous Reasoning and Planning Future language models will exhibit better decision-making and goal-setting abilities. Instead of just following instructions, they’ll be able to break down complex tasks into steps, adjust to changes, and refine their strategies—paving the way for autonomous agents that can manage schedules, conduct research, and even write code end-to-end. Real-World Applications on the Horizon AI Assistants with Agency: Imagine an assistant that not only answers emails but also schedules meetings, negotiates appointments, and books travel—all without constant user input. Advanced Tutoring Systems: AI tutors capable of explaining math, interpreting student expressions via video, and adjusting teaching strategies in real time. Creative Collaborators: Artists and filmmakers will be able to co-create with AI that understands narrative flow, visual aesthetics, and emotional impact. Ethical and Societal Considerations With power comes responsibility. As language models become more autonomous and human-like, questions around privacy, misinformation, bias, and control become even more critical. Developers, regulators, and society must work together to ensure these tools are safe, transparent, and aligned with human values. Conclusion Next-Gen AI: Beyond ChatGPT. The next generation of language models will be more than just smarter versions of ChatGPT—they’ll be proactive, multimodal, personalized digital partners capable of transforming how we work, learn, and create. As we look beyond ChatGPT, one thing is clear: we're just scratching the surface of what AI can achieve. Read the full article
#AIAdvancements#AIandHumanCollaboration#AIandSociety#AIinCreativity#AIinEducation#AILanguageAgents#AIPersonalization#Artificialintelligence#AutonomousAISystems#BeyondChatGPT#Content#design#EthicalAI#FutureofArtificialIntelligence#FutureofLanguageModels#LanguageModelEvolution#MultimodalAI#Next-GenAI
0 notes
Text
Intel MCP Model Context Protocol For Scalable & Modular AI

This blog shows how to construct modular AI agents without cumbersome frameworks for the Intel MCP and Intel accelerators. Multi-modal recipe production systems that analyse food photographs, identify ingredients, find relevant recipes, and generate tailored cooking instructions are examples.
MCP: Model Context Protocol?
Intel and other AI researchers created the Model Context Protocol (MCP) to help multi-modal AI agents manage and share context across multiple AI models and activities. Intel MCP prioritises scalability, modularity, and context sharing in distributed AI systems.
Contextual Orchestration
MCP relies on a context engine or orchestrator to manage AI component information flow. This monitors:
Current goal or job
The relevant context (conversations, scenario, user choices)
Which models need which data?
This orchestrator gives each model the most relevant context rather than the full history or data.
Communication using Modular Models
Modularising AI components is encouraged by Intel MCP.
Each speech, language, or vision module exposes a standard interface.
Modellers can request or receive modality-specific “context packs.”
This simplifies plug-and-playing models and tools from multiple vendors and architectures, improving interoperability.
Effective Context Packaging
MCP automatically compresses or summaries material instead of sending large amounts (pictures, transcripts, etc.):
For a reasoning model, a summariser model may summarise a document.
Vision models may output object embeddings instead of pictures.
Therefore, models can run more efficiently with less memory, computing power, and bandwidth.
Multiple-Mode Alignment
Intel MCP often uses shared memory or embedding space to align data types. This allows:
Fusing graphics and text is easier.
Cross-modal reasoning (including text-based inference to answer visual questions).
Security and Privacy Layers
Since context may contain private user data, Intel MCP frameworks often include:
Access control regulates which models can access which data.
Anonymisation: Changing or removing personal data.
Auditability tracks which models accessed which environments and when.
MCP Uses
MCP is useful in many situations:
Conversational AI (multi-turn assistants that remember prior conversations)
Speech and vision-based robotics Healthcare systems that integrate imaging, medical data, and patient contact
Intelligent context sharing between edge and cloud devices is edge AI.
Significance of Intel MCP
Multi-modal systems typically train models individually and communicate inefficiently. Intel MCP organises this communication methodically:
More efficient with less resources
Easy scaling of complex AI agents
Stronger, clearer, safer systems
Conclusion
With the Model Context Protocol, intelligent, scalable, and effective multi-modal AI systems have improved. MCP facilitates context-aware communication, flexible integration, and intelligent data processing to ensure every AI component uses the most relevant data.
Higher performance, lower computational overhead, and better model cooperation result. As AI becomes more human-like in interactions and decision-making, protocols like MCP will be essential for organising smooth, safe, and flexible AI ecosystems.
#IntelMCP#ModelContextProtocol#MCP#MCPModelContextProtocol#MCPApplications#multimodalAI#technology#technews#news#technologynews#technologytrends#govindhtech
0 notes
Text

Multimodal AI refers to artificial intelligence systems capable of processing, understanding, and generating outputs across multiple forms of data simultaneously. Unlike traditional AI models that specialize in a single data type (like text-only or image-only systems), multimodal AI integrates information from various sources to form a comprehensive understanding of its environment.
1 note
·
View note
Text
Google Unveils Gemini 2.0: A Leap Forward in AI Capabilities

Google Unveils Gemini 2.0: A Leap Forward in AI Capabilities Mountain View, CA - Recently Google announced the release of Gemini 2.0, its most advanced AI model yet, designed for the "agentic era." This signifies a significant step forward in AI technology, with Gemini 2.0 demonstrating enhanced reasoning, planning, and memory capabilities, enabling it to act more independently and proactively. Key Features of Gemini 2.0: Agentic Capabilities: Gemini 2.0 is designed to be more than just a tool; it's positioned as a collaborative partner. It can anticipate needs, plan multi-step actions, and even take initiative under user supervision. This "agentic" approach aims to revolutionize how we interact with AI, making it more integrated into our workflows. Enhanced Multimodality: Building upon the foundation of previous models, this model boasts advanced multimodal features. It can natively generate images and audio output, seamlessly integrating these capabilities into its responses. This allows for more creative and expressive interactions, opening up new possibilities for content creation and communication. Advanced Reasoning and Planning: Gemini 2.0 excels in complex reasoning tasks, including solving advanced math equations and tackling multi-step inquiries. Its improved planning capabilities enable it to effectively strategize and execute complex projects, making it a valuable asset for various applications. Seamless Tool Integration: Gemini 2.0 can natively utilize tools like Google Search and Maps, allowing it to access and process real-world information in a more integrated manner. This enhances its ability to provide accurate and up-to-date information, making it a more reliable source for knowledge and insights. Early Access and Future Plans: Gemini 2.0 Flash: An experimental version of the model, known as "Flash," is now available to developers through the Gemini API. This provides early access to the model's capabilities and allows developers to explore its potential for building innovative AI-powered applications. Broader Availability: Google plans to expand the availability of the new model to more Google products in the coming months. This will allow users to experience the benefits of this advanced AI technology across a wider range of services. Conclusion: The release of this model marks a significant milestone in the evolution of AI. Its agentic capabilities, enhanced multimodality, and advanced reasoning and planning abilities position it as a leading AI model with the potential to transform how we interact with technology and solve complex challenges. As Google continues to refine and expand this amazing new model, we can expect to see even more innovative applications of this powerful technology in the years to come.
Model variants
The Gemini API offers different models that are optimized for specific use cases. Here's a brief overview of Gemini variants that are available: Model variant Input(s) Output Optimized for Gemini 2.0 Flash gemini-2.0-flash-exp Audio, images, videos, and text Text, images (coming soon), and audio (coming soon) Next generation features, speed, and multimodal generation for a diverse variety of tasks Gemini 1.5 Flash gemini-1.5-flash Audio, images, videos, and text Text Fast and versatile performance across a diverse variety of tasks Gemini 1.5 Flash-8B gemini-1.5-flash-8b Audio, images, videos, and text Text High volume and lower intelligence tasks Gemini 1.5 Pro gemini-1.5-pro Audio, images, videos, and text Text Complex reasoning tasks requiring more intelligence Gemini 1.0 Pro gemini-1.0-pro (Deprecated on 2/15/2025) Text Text Natural language tasks, multi-turn text and code chat, and code generation Text Embedding text-embedding-004 Text Text embeddings Measuring the relatedness of text strings AQA aqa Text Text Providing source-grounded answers to questions Read the full article
#agenticAI#AIadvancements#AIagentcapabilities#AIcollaborationtools#AIplanningtools#AIreasoningcapabilities#AI-poweredapplications#audiogenerationAI#enhancedAImemory#experimentalAIAPI#Gemini2.0#Gemini2.0Flash#GoogleAImodel#GoogleGemini2.0#GoogleSearchintegration#imagegenerationAI#multimodalAI#multimodalliveAPI#proactiveAIsystems
0 notes
Text
Perplexity AI: Unlocking the Power of Advanced AI
Introduction
In the age of artificial intelligence, staying informed and efficient with data processing is essential. Perplexity AI, a cutting-edge AI model, promises just that—efficiency and innovation combined. But what is Perplexity AI, and how does it work? In this article, we’ll dive deep into what Perplexity AI is all about, its key features, and benefits, and why it's making waves in the world of technology. Whether you’re a tech enthusiast, data analyst, or business owner, you’ll find out how this AI tool can transform the way you understand and utilize data.
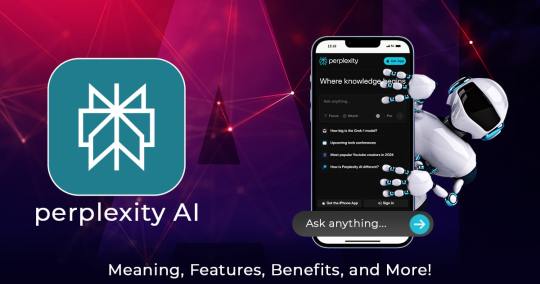
What is Perplexity AI?
Perplexity AI is a specialized artificial intelligence tool designed to understand, analyze, and generate content with a high degree of accuracy. Unlike traditional AI tools that may struggle with nuanced data, Perplexity AI uses a "language model" trained on vast amounts of text data, allowing it to interpret context and complexity. This AI tool doesn’t just stop at processing text; it also supports image input, making it a “multimodal” model. By incorporating various data types, Perplexity AI provides a richer and more versatile experience for users.
Key Features of Perplexity AI
Perplexity AI comes with a set of powerful features that set it apart from other AI tools. Here’s a breakdown of the most significant:
Contextual Understanding Perplexity AI doesn’t just analyze words; it understands context, allowing for more accurate and relevant responses. For example, if you ask it a question with a complex or nuanced answer, it can interpret the layers of meaning and provide a well-rounded response.
Multimodal Capabilities Beyond text, Perplexity AI also supports image inputs. You can prompt it with an image and receive information or answers based on the visual content. This feature is invaluable for fields where both text and image data are used, such as marketing, research, and education.
High-Speed Data Processing Time is of the essence in data processing, and Perplexity AI delivers results quickly. Whether analyzing documents, generating reports, or interpreting data, this AI tool saves time and effort, making it ideal for both professionals and students.
Customizable Responses Perplexity AI can tailor its responses based on user needs. If you need an explanation to be more in-depth or simplified, it can adjust accordingly. This adaptability is perfect for various users, from beginners to advanced professionals.
Continuous Learning Perplexity AI evolves and improves over time. By constantly learning from interactions, it refines its responses and keeps up with the latest information, ensuring that you receive relevant and up-to-date insights.
Benefits of Using Perplexity AI
Perplexity AI offers a multitude of benefits that make it a valuable tool for individuals and businesses alike. Here’s why you should consider incorporating it into your workflow:
Enhanced Productivity By automating repetitive tasks like data analysis, report generation, and even content creation, Perplexity AI frees up valuable time. Users can focus on more complex or strategic activities, boosting overall productivity.
Accurate Insights and Analysis One of Perplexity AI’s strengths is its ability to provide accurate insights, which is essential for businesses making data-driven decisions. Its advanced algorithms can identify patterns, spot trends, and offer recommendations that enhance decision-making.
Versatile Applications Across Industries Perplexity AI isn’t just for tech experts. From education and healthcare to finance and marketing, it has practical uses across numerous industries. Teachers can use it to create educational content, marketers for audience insights, and healthcare professionals for data interpretation.
Improved Content Creation Content creators, writers, and marketers can rely on Perplexity AI to generate high-quality content. By understanding context and nuances, it can produce relevant, on-topic, and grammatically correct content, saving time and ensuring quality.
User-Friendly and Accessible Even with its advanced features, Perplexity AI remains easy to use. Its interface is designed for accessibility, making it suitable for users of all levels, even those new to AI technology.
Frequently Asked Questions (FAQs) About Perplexity AI
1. How does Perplexity AI differ from other AI models? Perplexity AI stands out with its strong contextual understanding and multimodal capabilities. Its ability to process both text and images makes it more versatile compared to many traditional AI tools that handle only one data type.
2. Is Perplexity AI suitable for small businesses? Absolutely! Perplexity AI can benefit businesses of all sizes. It streamlines tasks, generates insights, and automates content creation—key functions that help small businesses enhance productivity without additional resources.
3. Can Perplexity AI handle complex topics? Yes. With its advanced language model, Perplexity AI is designed to tackle complex subjects. Whether it’s scientific research, technical topics, or detailed reports, it can provide relevant and accurate information.
4. How secure is Perplexity AI? Security is a priority. Perplexity AI follows stringent data protection protocols, ensuring that all user data remains private and secure.
5. What are the pricing options for Perplexity AI? Pricing varies based on usage levels and features. While free versions may offer basic access, premium subscriptions unlock advanced functionalities and are ideal for businesses or professionals needing more robust capabilities.
Why Perplexity AI Is Worth Trying
Whether you’re a professional looking to save time, a content creator seeking assistance, or a business aiming to make data-driven decisions, Perplexity AI can provide immense value. It’s not just a tool but a solution that addresses multiple needs, from generating reports to creating content and gaining insights. By bringing advanced AI directly into your workflow, Perplexity AI lets you stay ahead of the curve, making it a worthy addition to any productivity toolkit.
Conclusion
Perplexity AI combines cutting-edge technology with user-friendly features, making it an impressive tool for anyone seeking to enhance productivity and insights. Its versatility and ease of use ensure that it appeals to a wide audience, from beginners to advanced users. If you want a competitive edge in understanding data or improving efficiency, Perplexity AI is worth exploring.
As AI continues to shape our world, tools like Perplexity AI empower us to harness its full potential. Give Perplexity AI a try and see how it can transform your workflow today!
0 notes
Text
Generative AI is increasingly emphasizing the role of context in creating accurate responses. The next major leap in this field appears to be multimodal AI, where a single model processes various types of data—such as speech, video, audio, and text—simultaneously. This approach enhances context comprehension, allowing AI models to deliver more insightful and relevant outputs.
Explore our latest blog to learn more about the rising significance of multimodal AI and the exciting new opportunities it opens up.
0 notes
Text
Learn how Open-FinLLMs is setting new benchmarks in financial applications with its multimodal capabilities and comprehensive financial knowledge. Finetuned from a 52 billion token financial corpus and powered by 573K financial instructions, this open-source model outperforms LLaMA3-8B and BloombergGPT. Discover how it can transform your financial data analysis.
#OpenFinLLMs#FinancialAI#LLMs#MultimodalAI#MachineLearning#ArtificialIntelligence#AIModels#artificial intelligence#open source#machine learning#software engineering#programming#ai#python
2 notes
·
View notes
Link
#AdrenoGPU#AI#artificialintelligence#automateddriving#digitalcockpits#Futurride#generativeAI#Google#Hawaii#largelanguagemodels#LiAuto#LLMs#Maui#Mercedes-Benz#multimodalAI#neuralprocessingunit#NPU#OryonCPUSnapdragonDigitalChassis#Qualcomm#QualcommOryon#QualcommSnapdragonSummit#QualcommTechnologies#SDVs#SnapdragonCockpitElite#SnapdragonRideElite#SnapdragonSummit#software-definedvehicles#sustainablemobility
0 notes
Text
Nvidia Enters Open-Source AI Arena with NVLM
NVIDIA introduces NVLM 1.0, a multi-model redefining both vision-language and text-based AI tasks.

NVLM 1.0, a cutting-edge family of multimodal large language models (LLMs), is making waves in AI by setting new standards for vision-language tasks. Outperforming proprietary models like GPT-4o and open-access competitors such as Llama 3-V 405B, NVLM 1.0 delivers top-tier results across domains without compromise.
Post-multimodal training, NVLM 1.0 shows unprecedented accuracy in text-only tasks, surpassing its historical performance. Its open-access model, available through Megatron-Core, encourages global collaboration in AI research. NVLM 72B leads with the highest industry scores in benchmarks such as OCRBench and VQAv2, competing with GPT-4o on key tests.
Uniquely, NVLM 1.0 improves its text capabilities during multimodal training, achieving a 4.3-point increase in accuracy on key text-based benchmarks. This positions it as a powerful alternative not just for vision-language applications but also for complex tasks like mathematics and coding, outperforming models like Gemini 1.5 Pro.
By bridging multiple AI domains through an open-source design, NVLM 1.0 is set to spark innovation across academic and industrial sectors.

For more news like this: thenextaitool.com/news
0 notes
Text
Azure AI Content Understanding: Mastering Multimodal AI

Use Azure AI Content Understanding to turn unstructured data into multimodal app experiences.
To better reflect input and material that reflects our real world, artificial intelligence (AI) capabilities are rapidly developing and going beyond traditional text. To make creating multimodal applications containing text, music, photos, and video quicker, simpler, and more affordable, Microsoft Azure launching Azure AI Content Understanding. This service, which is currently in preview, extracts information into adaptable structured outputs using generative AI.
A simplified workflow and the ability to personalize results for a variety of use cases, like call center analytics, marketing automation, content search, and more, are provided via pre-built templates. Additionally, by simultaneously processing data from many modalities, this service can assist developers in simplifying the process of creating AI applications while maintaining accuracy and security.
Develop Multimodal AI Solutions More Quickly with Azure AI Content Understanding.
Overview
Quicken the creation of multimodal AI apps
Businesses may convert unstructured multimodal data into insights with the aid of Azure AI Content Understanding.
Obtain valuable insights from a variety of input data formats, including text, audio, photos, and video.
Use advanced artificial intelligence techniques like scheme extraction and grounding to produce accurate, high-quality data for use in downstream applications.
Simplify and combine pipelines with different kinds of data into a single, efficient process to cut expenses and speed up time to value.
Learn how call center operators and businesses may use call records to extract insightful information that can be used to improve customer service, track key performance indicators, and provide faster, more accurate answers to consumer questions.
Features
Using multimodal AI to turn data into insights
Ingestion of data in multiple modes
Consume a variety of modalities, including documents, photos, voice, and video, and then leverage Azure AI’s array of AI models to convert the incoming data into structured output that downstream applications can readily handle and analyze.
Tailored output schemas
To suit your needs, modify the collected results’ schemas. Make sure that summaries, insights, or features are formatted and structured to only include the most pertinent information from video or audio files, such as timestamps or important points.
Confidence ratings
With user feedback, confidence scores can be used to increase accuracy and decrease the need for human intervention.
Ready-made output for use in subsequent processes
The output can be used by downstream applications to automate business processes using agentic workflows or to develop enterprise generative AI apps using retrieval-augmentation generation (RAG).
Getting grounded
A representation of the extracted, inferred, or abstracted information should be included in the underlying content.
Automated labeling
By employing large language models (LLMs) to extract fields from different document types, you may develop models more quickly and save time and effort on human annotation.
FAQs
What is Azure AI Content Understanding?
In the era of generative AI, Content Understanding is a new Azure AI service that helps businesses speed up the development of multimodal AI apps. Using a variety of input data formats, including text, audio, photos, documents, and video, Content Understanding helps businesses create generative AI solutions with ease using the newest models on the market. AI already recognizes faces, builds bots, and analyzes documents. Without ever needing specialized generative AI skills like prompt engineering, Content Understanding gives businesses a new way to create applications that can integrate all of these using pre-built templates made to address the most common use-cases or by creating custom models to address use-cases that are specific to a given domain or enterprise. With the help of the service, businesses may contribute their domain knowledge and create automated processes that consistently improve output while guaranteeing strong accuracy. Using Azure’s industry-leading enterprise security, data privacy, and ethical AI rules, this new AI service was developed.
What are the benefits of using Azure AI Content Understanding?
Developers can use Content Understanding to develop unique models for their organization and integrate data kinds from multiple modalities into their current apps. For multimodal scenarios, it greatly streamlines the development of generative AI solutions and eliminates the need for manual switching to the most recent model when it is released. It speeds up time-to-value by analyzing several modalities at once in a single workflow.
Where can I learn to use Azure AI Content Understanding?
Check out the Azure AI Studio‘s Azure AI Content Understanding feature.
Read more on Govindhtech.com
#AzureAI#AzureAIContent#MultimodalAI#AI#generativeAI#AzureAIContentUnderstanding#News#Technews#Technology#Technologynews#Technologytrends#Govindhtech
0 notes
Text
Unlocking the Power of Llama 3.2: Meta's Revolutionary Multimodal AI
Dive into the latest advancements in AI technology with Llama 3.2. Explore its multimodal capabilities, combining text and voice for seamless user interactions. Discover how this innovative model can transform industries and enhance user experiences.
#Llama32 #MetaAI #MultimodalAI #VoiceTechnology #AIInnovation
0 notes
Text
Top 7 Platforms to Quickly Build Multimodal AI Agents in 2025
Explore the leading platforms revolutionizing the development of multimodal AI agents in 2025. From versatile tools like LangChain and Microsoft AutoGen to user-friendly options like Bizway, discover solutions that cater to various technical needs and budgets. These platforms enable seamless integration of text, images, and audio, enhancing applications across industries such as customer service, analytics, and education.
0 notes