#PyTorch Geometric
Explore tagged Tumblr posts
Text
Peran Alat Pembelajaran Mesin dalam Meningkatkan Kemampuan AI di 2025
Pembelajaran mesin (machine learning) adalah cabang dari kecerdasan buatan (AI) yang memungkinkan sistem untuk belajar dan meningkatkan performa mereka tanpa pemrograman eksplisit. Dalam beberapa tahun terakhir, perkembangan pembelajaran mesin telah menjadi pendorong utama kemajuan AI. Pada tahun 2025, peran alat pembelajaran mesin semakin signifikan dalam meningkatkan kemampuan AI, baik dalam…
#advanced AI frameworks#AI collaboration#AI development#AI ethics#AI for business#AI in 2025#AI research#AI scalability#AI tools#artificial intelligence trends#deep learning#federated learning#future of AI#generative AI#graph-based machine learning#machine learning#multimodal learning#PyTorch Geometric#TensorFlow Federated
0 notes
Link
0 notes
Link
Author(s): Marco Lomele Originally published on Towards AI. Graph Neural Networks and Transformers for Time Series Forecasting on Heterogeneous Graphs to Predict Butte Trade Volumes. Source: Image by the author. Graphs and Time Graphs are becoming o #AI #ML #Automation
0 notes
Text
Anime Character Drawing
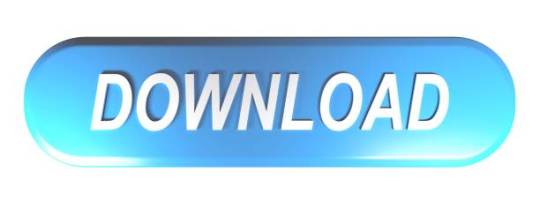
Learning how to draw an anime character is very easy, you just need to read and repeat the steps from this drawing lesson created by the team of Drawingforall.net. In one of the previous guides, the team of Drawingforall.net has already shown how to draw anime, and in this instruction we want to show how to draw an anime character from a slightly different angle. This lesson will also be a kind of basic guide with the help of which our readers will be able to learn to draw a wide variety of anime persons. Using this simple instruction you can also draw an anime version of yourself or your friends. So, to start creating you will need such classic things as paper, pencil, preferably automatic since it is easier to work with it, and an eraser.
Step 1
In order to properly draw an anime person we must draw his skeleton and begin, of course, from the head. Next, using the most simple, light and almost transparency line depict the spine. On the spine sketch the chest and pelvis. The arms we also sketch with light lines. We did all these operations in order not to get confused in the proportions in the future and that our anime character would be beautiful and proportional.
Free interactive 3D characters for reference poses. Breathe life into your art. Give depth to your characters with the best pose reference tool on the web. Anime is one of those drawing styles that makes it fairly easy to change the expressions of the characters. Get 300+ freebies in your inbox! Subscribe to our newsletter and receive 300+ design resources in your first 5 minutes as a subscriber. A character for a manga or an animation should have a reasonably simple design (it can become too time consuming to draw a complex character multiple times). You need to consider how a manga or anime character will look from all views/angles (you will likely have to draw them from multiple views multiple times). A simple PyTorch Implementation of Generative Adversarial Networks, focusing on anime face drawing. Randomly Generated Images. The images are generated from a DCGAN model trained on 143,000 anime character faces for 100 epochs. Image Interpolation. Manipulating latent codes, enables the transition from images in the first row to the last row.
Drawing basic shapes. Drawing anime characters is great. But if you are a total beginner to drawing in general you should start by learning the basics. Practice drawing simple things like straight lines and basic shapes like circles and squares. This can be boring at times but will help you improve. Continue drawing anime or anything else you might like. Practice drawing basic lines and shapes a few minutes a day and then draw anything you find fun or interesting after that.
Step 2
Now we need to make our anime person drawing more voluminous, for this we will have to work with simple geometric shapes. For us it is very important that the face of the anime person was symmetrical and there were no mistakes in it. For these purposes, we will draw two lines intersecting in the center of the face in the place where the nose bridge will be located. To draw the neck we use a simple cylinder and to draw a torso we use a smooth geometric shape resembling a large cylinder.
Step 3
Now we need to add volume to the limbs of our anime character. For drawing arms and legs, we use elongated cylindrical shapes. And for drawing the shoulders, elbow and knee joints, we use circles and ovals. A small life hack to check the proportionality of the sketch. You can look at it through the mirror and it will show you all the errors in the ratio of details.
Step 4
The head of anime characters is one of the most difficult. And in order to properly draw the anime head, first of all we need to add the most basic details and for this we will be guided by the intersecting lines that we drew in the second step. Gently sketch the location of your character’s eyes, mark nose with a short line and the mouth with another one. In the same step, you need to outline the contours of the jaw and hair.
Step 5
Now we start indicating lines of clothing, it is actually very easy. If you draw clothes from a photo or from imagination, then try to transfer all the lines on paper as the artists of Drawingforall.net did in the example below. To start adding details some artists use a new sheet to put it on top of an old sheet with the sketch in order not to use an eraser and immediately get rid of them. Anime characters often wear slim fit pants, so most of the folds will be located in the area of groin, knees and at the very bottom of the pants.
Step 6
Circle the face details with dark and clear lines, add the necessary details if you missed them earlier. The jaw of an anime person can be narrowed like ours, round or more angular. Now circle the hair with the same dark lines. The more lines the hair will look more realistic. Of course, there are an infinite amount of types of people in this world, and their faces will also be very different, but the general principles of drawing remain unchanged.
Anime Character Creator
Step 7
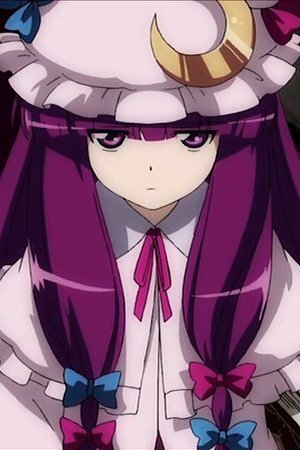
Let’s get down to the torso. But first, draw the neck muscles with two slightly curved lines and the clavicle with two more lines. Circle clothing using very smooth and sharp lines. Anime persons have a great variety of clothes and styles. You can wear your drawn character in a T-shirt or something more complicated. To make your anime person drawing look prettier and cleaner, remove all unnecessary auxiliary lines from the torso.
Step 8
Anime Character Drawing Outline
Now, dear artists, let’s repeat the same actions, but with the arms of the anime man. We also need to circle them using clear and dark lines. By the way, you can make lines thinner and thicker in order to focus on certain areas and make the lines more juicy and more interesting. Circle the hands and fingers, remove all unnecessary guidelines. By the way, in the guide about anime hands you can learn about it in detail.
Step 9
The basic guidelines for the shape of the jeans are already drawn, and here we just need to deep into the details. So, here we need to indicate the folds that look like simple and short strokes and bumps located in the area of the pelvis, knees and in the place where the pants are tucked into shoes. And to clean your anime person drawing, take the eraser and remove all remaining unnecessary auxiliary lines which we no longer need.
Step 10
Anime Character Drawing Easy
To correctly draw the shadows, first map out their location. Then start smoothly filling the necessary areas with hatching, adjusting the intensity of shadows by changing the pressing on the pencil and changing the density of the hatching. Try to keep the drawing clean from unnecessary lines, for this you can use a piece of paper that you can put under the working hand.
As we said, you are free to use the guide about how to draw an anime character, to portray yourself or or anyone else. For this purpose, you first need a photo that will serve as the basis for the future work of art. You will need to painstakingly transfer the photo to a sheet of paper, simultaneously turning realistic features and details into a more anime version. One more thing you should remember, is that the team of Drawingforall.net is waiting for your suggestions and criticism. This is a very important aspect, which helps us not to lose touch with the audience, which is also interested in the subject of art. So write your comments under our articles, subscribe to us on social networks and share our articles with everyone who, just like you, wants to become a real artist.
Anime Character Drawing Base
Recommended
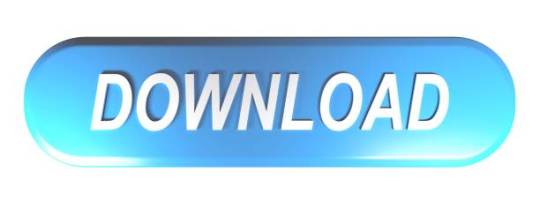
1 note
·
View note
Text
Microsoft Researchers Unlock New Avenues In Image-Generation Research With Manifold Matching Via Metric Learning
By developing fresh images, generative image models provide a distinct value. These photos could be clear super-resolution copies of current images or even manufactured shots that look realistic. The framework of training two networks against each other has shown pioneering success with Generative Adversarial Networks (GANs) and their variants: a generator network learns to generate realistic fake data that can fool a discriminator network, and the discriminator network learns to correctly tell apart the generated counterfeit data from the actual data.
The research community must address two issues in order to use the most recent advances in computer vision for GANs. First, rather than using geometric metrics, GANs model data distributions using statistical measures such as the mean and moments. Second, in classic GANs, the discriminator network loss is solely represented as a 1D scalar value corresponding to the Euclidean distance between the genuine and fake data distributions. The research community has been unable to utilize breakthrough metric learning methods directly or experiment with novel loss functions and training strategies to continue to develop generative models due to these two limitations.
Microsoft researchers offer a novel framework for generative models called Manifold Matching via Metric Learning in a recent paper titled “Manifold Matching via Deep Metric Learning for Generative Modeling” (MvM). Two networks are trained against each other in the MvM framework. For the distribution generator network’s manifold matching objective, the metric generator network learns to define a better metric. The distribution generator network learns to provide more hard negative samples for the metric generator network’s metric learning objective. MvM creates a distribution generator network that can generate fake data distributions that are very close to the accurate data distributions, as well as a metric generator network that can provide an effective metric for capturing the data distribution’s internal geometric structure using adversarial training. In October, this research was accepted at the International Conference on Computer Vision (ICCV 2021).
Quick Read: https://www.marktechpost.com/2021/11/30/microsoft-researchers-unlock-new-avenues-in-image-generation-research-with-manifold-matching-via-metric-learning/
Paper: https://arxiv.org/pdf/2106.10777.pdf
Github:https://github.com/dzld00/pytorch-manifold-matching
Microsoft Blog: https://www.microsoft.com/en-us/research/blog/unlocking-new-dimensions-in-image-generation-research-with-manifold-matching-via-metric-learning/
submitted by /u/techsucker [link] [comments] from Ai https://ift.tt/3lmL93e
0 notes
Photo
"[R] [1907.10830] U-GAT-IT: Unsupervised Generative Attentional Networks with Adaptive Layer-Instance Normalization for Image-to-Image Translation"- Detail: 1st row : input, 2nd row : attention map, 3rd row : outputEach column dataset is "selfie2anime", "horse2zebra", "cat2dog", "photo2vangogh", "photo2portrait"& "portrait2photo", "vangogh2photo", "dog2cat", "zebra2horse", "anime2selfie"AbstractWe propose a novel method for unsupervised image-to-image translation, which incorporates a new attention module and a new learnable normalization function in an end-to-end manner. The attention module guides our model to focus on more important regions distinguishing between source and target domains based on the attention map obtained by the auxiliary classifier. Unlike previous attention-based methods which cannot handle the geometric changes between domains, our model can translate both images requiring holistic changes and images requiring large shape changes. Moreover, our new AdaLIN (Adaptive Layer-Instance Normalization) function helps our attention-guided model to flexibly control the amount of change in shape and texture by learned parameters depending on datasets. Experimental results show the superiority of the proposed method compared to the existing state-of-the-art models with a fixed network architecture and hyper-parameters.paper : https://ift.tt/2K7llpI Tensorflow : https://ift.tt/2YuHnqh Pytorch : https://ift.tt/2K3N1vA. Caption by taki0112. Posted By: www.eurekaking.com
0 notes
Text
Robust.AI launches to build an industrial-grade cognitive platform for robots
Despite the seemingly fantastical demonstration of walking and jumping robots, today’s robots are often stupid, brittle and inflexible, only capable of working in carefully-engineered environments, and unable to respond dynamically and sensibly in unexpected circumstances.
Deep learning has been spectacularly successful in certain problems (facial recognition, object recognition, etc) but the “smart robots” that we have promised still haven’t arrived. The promised robotic future is still a long way off.
New Silicon Valley robotics startup Robust.AI aims, firstly, to build the world’s first industrial-grade cognitive platform for robots. Secondly, it will aim to help companies in a wide range of areas, from construction to eldercare and domestic robots, towards the goal of making robots that are smarter, safer, more robust, more context-aware, and more collaborative.
Initially located in Palo Alto, California, Robust.AI has secured a “substantial” undisclosed seed round from Playground Global, among other undisclosed investors.
The market for intelligent robots is worth potentially several hundred billion dollars a year, once robots can enter new markets (e.g., construction, eldercare, to-the-door delivery) that historically have been too challenging for traditional robotics.
Robust.AI plans to make money by licensing its cognitive platform, and by helping companies solve robotics problems that would otherwise be out of reach of current technology.
To create this vision, the company has two stellar founders: Rodney Brooks, co-founder of iRobot and Rethink Robotics, co-inventor of Roomba, the best-selling consumer robot of all time, and former chair of the MIT AI lab (CSAIL); and Gary Marcus (CEO co-founder of Geometric Intelligence, acquired by Uber, bestselling author and cognitive scientist at NYU). Brooks will be CTO, Marcus will be the CEO.
Coming from different perspectives, Marcus (cognitive science) and Brooks (robotics) have been writing for the last several years about the perils of deep learning, and why it had been overhyped; they also independently reached similar conclusions about the need for developing machine-interpretable common sense as a prerequisite for reaching the next level of AI.
When Marcus decided to take the plunge into robotics, he says he realized immediately that Brooks, a legend in robotics, would be the perfect collaborator, and Marcus spent months recruiting him. The two say they are excited by the mission and the commercial potential.
“We are building an industrial-strength cognitive platform — the first of its kind — to enable robots to be smart, collaborative, robust, safe and genuinely autonomous, with applications in a very broad range of verticals from construction and delivery to warehouses and domestic robots,” Marcus told me.
“We will be synthesizing a wide range of advances in AI, including both deep learning and classical approaches, with a focus on building machines with a toolbox for common sense,” he continued.
As for competitors, Marcus seems to think there aren’t many: “We don’t know anyone else trying to do this. Most often what happens nowadays is that when one wants to build a robot, one hacks together a mixture of ROS [Robot Operating System] and TensorFlow or PyTorch, tailored to a very specific problem. We don’t know of anyone building the kind of general-purpose cognitive tools we have in mind. There is no extant tool that can deliver the kind of complex, flexible cognition that we are focusing on.”
0 notes
Text
確率的プログラミングPyro入門
from https://developers.eure.jp/tech/ppl-pyro/
確率的プログラミングPyro入門
はじめまして。eureka-BIチームの小林です。 普段は卓球とスプラトゥーンをやっています。
この記事は eureka Engineering Advent Calendar 2017 – Qiita の17日目の記事です。 16日目は サマーインターン参加者かつSREでインターン中のdatchこと原田くんの 「Pairsのテキストデータを学習させたword2vecを使って、コミュニティを分類してみた」です。
はじめに
BIチームでは、様々な数字を分析することで、プロダクトの意思決定に貢献しています。 その中で、データからモデルを作成し、予測を立てるといった業務をすることがあります。 今までは、簡単な線形回帰でのモデル作成に留まりがちで、知識としてもMCMCで止まっていたので、 今後、確率的プログラミングを取り入れたモデリングをしていきたいと思い、最近発表されたばかりのPyroを触ってみました。
実際に業務に用いられるレベルにはマスターできていないので、今回は導入から簡単な使い方までを、 公式のIntroductionをなぞっていくことで説明します。
確率的プログラミング言語Pyro
PyroはUBERが公開している確率的プログラミング言語です。 プログラミング言語、とは言うものの実際はPythonのライブラリとして公開されています。 確率的プログラミングについては、ここに書くと長くなってしまうので割愛しますが、以下の記事が詳しいです。 確率的プログラミング | POSTD PyroはPyTorchをバックエンドに使い、高速なテンソル計算と自動微分を実現しています。 Pyroに似たものにEdwardがあり、こちらはTensorflowをバックエンドに利用しています。
Pyroの導入
PyroではPyTorchが必要になるので、インストールしておいてください。 PyTorchが入っていれば、Pyro自体はpip install pyro-pplでインストールできます。
Pyroの基礎
変数は全てtorchのtensorをVariableで包んだ形で保持します。 言葉で表現すると意味不明なのですが、コードで書くとすなわち
import torch from torch.autograd import Variable hoge = Variable(torch.Tensor(hage))
こうなります。 つまり、mu = 0 と sigma = 1 を表現すると
mu = Variable(torch.zeros(1)) # zerosは0のみのtensorを生成する sigma = Variable(torch.ones(1))
こうなります。 平均 mu 分散 sigma の正規分布にしたがう x は以下で表現できます。
import pyro.distributions as dist x = dist.normal(mu, sigma)
または、
import pyro x = pyro.sample("my_sample", dist.normal, mu, sigma)
とすることで、my_sample という名前を用いたサンプリングとして定義することもできます。
また、この x の時の対数確率密度の値は
log_p_x = dist.normal.log_pdf(x, mu, sigma)
で取得できます。
Pyroでのモデリング
上記の基礎構文を用いて、天気と気温の関係を表現するモデルを作成します。
def weather(): cloudy = pyro.sample('cloudy', dist.bernoulli, Variable(torch.Tensor([0.3]))) cloudy = 'cloudy' if cloudy.data[0] == 1.0 else 'sunny' mean_temp = {'cloudy': [55.0], 'sunny': [75.0]}[cloudy] sigma_temp = {'cloudy': [10.0], 'sunny': [15.0]}[cloudy] temp = pyro.sample('temp', dist.normal, Variable(torch.Tensor(mean_temp)), Variable(torch.Tensor(sigma_temp))) return cloudy, temp.data[0]
順を追って説明すると、 ・ 2~4行目では、cloudyはベルヌーイ分布により30%の確率で曇り、70%の確率で晴れとなることを表しています。 ・ 5,6行目では気温の従う分布の平均と分散を、天気を条件にして定めます。(変数はテンソルで扱うために、配列として渡しています。) ・ 最後に、上で定めた値を元に正規分布より気温の値を決め、天気と気温を返します。
このように、統計的な分布に基づくランダムな値を生成するモデルを作成することができます。
Pyroは、Pythonで作成されているので、統計的な関数はPythonで用いるような複雑な書き方もできます。 例えば、再帰的な書き方をしたモデルの例として、
def geometric(p, t=None): if t is None: t = 0 x = pyro.sample("x_{}".format(t), dist.bernoulli, p) if torch.equal(x.data, torch.zeros(1)): return x else: return x + geometric(p, t+1)
このように書けます。 サンプリングする際は、必ずユニークな名前を付ける必要があるので、再帰回数ごとに、x_1, x_2 …としています。
また、以下のように他の確率的関数を入力としたり、出力とすることもできます。
def normal_product(mu, sigma): z1 = pyro.sample("z1", dist.normal, mu, sigma) z2 = pyro.sample("z2", dist.normal, mu, sigma) y = z1 * z2 return y def make_normal_normal(): mu_latent = pyro.sample("mu_latent", dist.normal, Variable(torch.zeros(1)), Variable(torch.ones(1))) fn = lambda sigma: normal_product(mu_latent, sigma) return fn print(make_normal_normal()(Variable(torch.ones(1))))
Pyroによる推定
重点サンプリングによって、周辺分布を求めることができます。 例えば、毎回測定誤差が出るような秤のモデルを例に置くと、
def scale(guess): weight = pyro.sample("weight", dist.normal, guess, Variable(torch.ones(1))) return pyro.sample("measurement", dist.normal, weight, Variable(torch.Tensor([0.75]))
と定義した秤のモデルに対して、
posterior = pyro.infer.Importance(scale, num_samples=100)
とすると、重点サンプリングがなされます。 しかし、posterior単体では有用なオブジェクトではなく、pyro.infer.Marginalによる周辺化に用いられます。
guess = Variable(torch.Tensor([8.5])) marginal = pyro.infer.Marginal(posterior) print(marginal(guess))
marginalは、scaleを重点サンプリングしたposteriorからヒストグラムを生成し、 それを元に、guessの値が与えられた場合の分布から値をサンプリングします。 同じ引数を持つmarginalを��数回呼び出すと、同じヒストグラムからサンプリングされるので、
plt.hist([marginal(guess).data[0] for _ in range(100)], range=(5.0, 12.0)) plt.title("P(measurement | guess)") plt.xlabel("weight") plt.ylabel("#")
とすると、同一のヒストグラムを元にサンプリングされるので、元の形が再現されていきます。
パラメータ調整
確率的プログラミングによるモデリングの有用性は、 観測値によってモデルを調整することで、データ生成における潜在的な要因を推定する能力にあります。
例えば、秤のモデルにおいて,計測値が8.5になる場合はこのように表現します。
conditioned_scale = pyro.condition( scale, data={"measurement": Variable(torch.Tensor([8.5]))})
パラメータ調整において、下記のように引数を与えられるようにもできます。
def deferred_conditioned_scale(measurement, *args, **kwargs): return pyro.condition(scale, data={"measurement": measurement})(*args, **kwargs)
また、conditionメソッドではなく、obsパラメータを用いる書き方や、pyro.observeを用いた下記からも存在します。
## equivalent to pyro.condition(scale, data={"measurement": Variable(torch.ones(1))}) def scale_obs(guess): z = pyro.sample("weight", dist.normal, guess, Variable(torch.ones(1))) # here we attach an observation measurement == 1 return pyro.sample("measurement", dist.normal, weight, Variable(torch.ones(1)), obs=Variable(torch.Tensor([0.1]))) ## equivalent to scale_obs: def scale_obs(guess): z = pyro.sample("weight", dist.normal, guess, Variable(torch.ones(1))) # here we attach an observation measurement == 1 return pyro.observe("measurement", dist.normal, Variable(torch.ones(1)), weight, Variable(torch.Tensor([0.1])))
ただし、モデル中でハードコーディングすることはあまり推奨されないので、 pyro.conditionによって、モデルを変更することなく条件を与える方が良いです。
また、複数の条件を与える書き方は複数あり、
def scale2(guess): weight = pyro.sample("weight", dist.normal, guess, Variable(torch.ones(1))) tolerance = torch.abs( pyro.sample("tolerance", dist.normal, Variable(torch.zeros(1)), Variable(torch.ones(1)))) return pyro.sample("measurement", dist.normal, weight, tolerance) conditioned_scale2_1 = pyro.condition( pyro.condition(scale2, data={"weight": Variable(torch.ones(1))}), data={"measurement": Variable(torch.ones(1))}) conditioned_scale2_2 = pyro.condition( pyro.condition(scale2, data={"measurement": Variable(torch.ones(1))}), data={"weight": Variable(torch.ones(1))}) conditioned_scale2_3 = pyro.condition( scale2, data={"weight": Variable(torch.ones(1)), "measurement": Variable(torch.ones(1))})
3つのconditionメソッドは同質です。
秤のモデルにおいて、pyro.conditionを使ってguessとmeasurementを与えた時のweightの値について推測したいときは、先ほどの重点サンプリングの例と同様にして、
guess = Variable(torch.Tensor([8.5])) measurement = Variable(torch.Tensor([9.5])) conditioned_scale = pyro.condition(scale, data={"measurement": measurement}) marginal = pyro.infer.Marginal( pyro.infer.Importance(conditioned_scale, num_samples=100), sites=["weight"]) print(marginal(guess)) plt.hist([marginal(guess)["weight"].data[0] for _ in range(100)], range=(5.0, 12.0)) plt.title("P(weight | measurement, guess)") plt.xlabel("weight") plt.ylabel("#")
とすることで分布が求められます。
ただし、これらのやり方は、事前分布に関する情報や制約がないため計算効率が悪いです。 そこで、Pyroでは、Guideを利用して効率化することができます。 例えば以下のように書くことで、推定を効率化できます。
def scale_prior_guide(guess): return pyro.sample("weight", dist.normal, guess, Variable(torch.ones(1))) posterior = pyro.infer.Importance(conditioned_scale, guide=scale_prior_guide, num_samples=10) marginal = pyro.infer.Marginal(posterior, sites=["weight"])
または、weightの事後分布は、guessとmeasurementによって表されるので、
def scale_posterior_guide(measurement, guess): a = (guess + torch.sum(measurement)) / (measurement.size(0) + 1.0) b = Variable(torch.ones(1)) / (measurement.size(0) + 1.0) return pyro.sample("weight", dist.normal, a, b) posterior = pyro.infer.Importance(deferred_conditioned_scale, guide=scale_posterior_guide, num_samples=20) marginal = pyro.infer.Marginal(posterior, sites=["weight"])
と書くことができます。 今回の秤のモデルは、自ら中の仕組みを組み上げているため、 正確な事後分布を書くことができますが、 一般的には正確な事後分布を推定するのは難しいです。 そのため、変分推論と呼ばれるアプローチによって、近似的な事後確率を求めます。
PyroによるSVI(簡���説明)
pyro.paramはpyro.sampleのように、第一引数で名前をつけられます。 初回呼び出し時には、名前とその引数が結びつけられ、その後の呼び出しでは、 他の引数にかかわらず、名前によって値が返されます。
def scale_parametrized_guide(guess): a = pyro.param("a", Variable(torch.randn(1) + guess.data.clone(), requires_grad=True)) b = pyro.param("b", Variable(torch.randn(1), requires_grad=True)) return pyro.sample("weight", dist.normal, a, torch.abs(b))
PyroのSVIについて、公式でSVIのためのチュートリアルが用意されているので、 今回は詳しい説明は省きますが、秤のモデルに適応した簡単なものは以下のように書けます。
pyro.clear_param_store() svi = pyro.infer.SVI(model=conditioned_scale, guide=scale_parametrized_guide, loss="ELBO") losses = [] for t in range(1000): losses.append(svi.step(guess)) plt.plot(losses) plt.title("ELBO") plt.xlabel("step") plt.ylabel("loss")
今回は、optimによる最適化手法の選択と、 lossでの損失関数の指定については説明しません。
また、以下のように最適化されたガイドを重点サンプリングの重要度分布として使用すると、 以前よりも少ないサンプルで周辺分布を推定できます。
posterior = pyro.infer.Importance(conditioned_scale, scale_parametrized_guide, num_samples=10) marginal = pyro.infer.Marginal(posterior, sites=["weight"]) plt.hist([marginal(guess)["weight"].data[0] for _ in range(100)], range=(5.0, 12.0)) plt.title("P(weight | measurement, guess)") plt.xlabel("weight") plt.ylabel("#")
ガイドから直接、事後分布の近似としてサンプリングすることもできます。
plt.hist([scale_parametrized_guide(guess).data[0] for _ in range(100)], range=(5.0, 12.0)) plt.title("P(weight | measurement, guess)") plt.xlabel("weight") plt.ylabel("#")
まとめ
以上、簡単にではありますがPyroの導入から基本的な使い方を、Introductionに沿って説明させていただきました。 本当は、SVIの解説を詳しくやっていくつもりだったのですが、文量が10倍になりそうでしたので、今回は省かせていただきました。 また、Edwardとの比較や、pystanなどとの速度比較もしたかったのですが、次回のお楽しみとさせていただきます。 ちなみに、pyroを日本語に訳すと、「火」「熱」「高温」という意味があるようです。 プロジェクトの炎上を連想させる「火」をチョイスするセンスは見習いたいものですね。
明日は、BIチームでもっともホスピタリティのある鈴木さん aka ミニオンさんによる 「非エンジニアがSQLを学習する際の10の心得」です。お楽しみに!
https://i0.wp.com/developers.eure.jp/wp-content/uploads/2017/12/hist1.png
0 notes
Text
What’s going on on PyPI
Scanning all new published packages on PyPI I know that the quality is often quite bad. I try to filter out the worst ones and list here the ones which might be worth a look, being followed or inspire you in some way. • phovea-data-hdf Data provider plugin for loading data stored in the hierarchical data format (HDF). • sumgram sumgram is a tool that summarizes a collection of text documents by generating the most frequent sumgrams (multiple ngrams) • yamconv yamconv converts the file formats of machine learning datasets • algorithm-x An efficient implementation of Algorithm X • apache-superset-johan078 A modern, enterprise-ready business intelligence web application • batchflows-pporto library for executing batches of data processing sequentially or asynchronously to python 3 • coffeeshop A python package that sends your deep learning training loss to your slack channel after every specified epoch. • ctpbee_converter Data converter base pandas and numpy • dbloy Continuous Delivery tool for PySpark Notebooks based jobs on Databricks. • dvc-cc This connector is used to combine the work of CC (www.curious-containers.cc) and DVC (Open-source Version Control System for Machine Learning Projects). • graph-articulations Extension of PyTorch Geometric and Open 3d for learning kinematic articulations • HyPSTER HyPSTER is a brand new Python package that helps you find compact and accurate ML Pipelines while staying light and efficient. • jetstreamer Image and inference metadata recording utility for NVIDIA Tegra. JetStreamer is a command line utility* to record frames and perform inferences from a camera on NVIDIA Tegra. It uses the Jetson Inference library which is comprised of utilities and wrappers around lower level NVIDIA inference libraries. • JLpyUtils Custom methodes for various data science, computer vision, and machine learning operations in python • Lutil Assist small-scale machine learning. http://bit.ly/2sAkLu7
0 notes
Text
Hands on Graph Neural Networks with PyTorch & PyTorch Geometric
http://dlvr.it/R5lTv5
0 notes
Photo
"[N] Deep Graph Library v0.3 release"- Detail: Graph Neural Network has become the new fashion in many graph-based learning problems. Deep Graph Library (DGL) is a Python package built for easy implementation of graph neural network model family, on top of existing DL frameworks (e.g. PyTorch, MXNet, Gluon etc.). As the team behind this library, we want to share with you the new release of DGL (v0.3) that is much faster (up to 19x faster) and more scalable for training GNNs on large graphs (up to 8x larger). Checkout our full release note here: http://bit.ly/2XJI53k .For whom have never heard of DGL or Graph Neural Network, maybe it is worth to take a look at this new trend of geometric deep learning. Some links here:Checkout this 10-minute tutorial about how to use Graph Neural Network to predict community membership (http://bit.ly/2x1icjX more about how a variety of models can be unified under the message passing framework and can be implemented in DGL (http://bit.ly/2XFwYs6 github repo: http://bit.ly/2wRv3F3 project site: https://www.dgl.ai/ . We publish many blogs about the new findings in this area.. Caption by jermainewang. Posted By: www.eurekaking.com
0 notes
Photo
"[D] Hands-on Graph Neural Networks with PyTorch & PyTorch Geometric"- Detail: PyTorch Geometric is one of the fastest Graph Neural Networks frameworks in the world. In this article, I talked about the basic usage of PyTorch Geometric and how to use it on real-world data.http://bit.ly/2Miolmg. Caption by steeveHuang. Posted By: www.eurekaking.com
0 notes
Text
Fresh from the Python Package Index
• torchmeta Dataloaders for meta-learning in Pytorch. A collection of extensions and data-loaders for few-shot learning & meta-learning in [PyTorch](https://pytorch.org ). The package contains popular meta-learning benchmarks, fully compatible with both [`torchvision`](https://…/index.html ) and PyTorch’s [`DataLoader`](https://…/data.html#torch.utils.data.DataLoader ). • cluster-over-sampling Clustering based over-sampling. • csdmpy A python module for importing and exporting CSD model file-format. The `csdmpy` package is a Python support for the core scientific dataset (CSD) model file exchange-format. The package is based on the core scientific dataset (CSD) model which is designed as a building block in the development of a more sophisticated portable scientific dataset file standard. The CSD model is capable of handling a wide variety of scientific datasets both within and across disciplinary fields. • fastrank A set of learning-to-rank algorithms. • lisc Literature Scanner. LISC, or ‘literature scanner’ is a package for collecting and analyzing scientific literature. LISC acts as a wrapper and connector between available APIs, allowing users to collect data from and about scientific articles, and to do analyses on this data, such as performing automated meta-analyses. • pylift Python implementation of uplift modeling. • pythonGraph simple graphics in Python. pythonGraph is a simple graphics package for Python. It’s design is loosely descended from Adagraph and RAPTOR Graph. pythonGraph supports the creation of a graphics window, the display of simple geometric shapes, and simple user interactions using the mouse and keyboard. • pytorch-transformers-pvt-nightly Repository of pre-trained NLP Transformer models: BERT & RoBERTa, GPT & GPT-2, Transformer-XL, XLNet and XLM • qubu A Simple Database Query Builder • rl-musician Composition of music with reinforcement learning. • shapeletpy Time series classification Using Shapelets • torch-radiate Automatic deep learning research report generator • torch-stethoscope Automatic deep learning research report generator • yahoo-finance-hdd Download historical financial data from yahoo finance • affnine-deltaleaf Easy way to create MAP (Undirected Graph)and find shortest path from one Node to another. • apai ML & DL helper library. Helper library built on Tensorflow. Provides utility and helper functions for the machine and deep learning tasks. • easyagents-v1 reinforcement learning for practitioners. Use EasyAgents if • you are looking for an easy and simple way to get started with reinforcement learning • you have implemented your own environment and want to experiment with it • you want mix and match different implementations and algorithms • ignis Intuitive library for training neural nets in PyTorch. `ignis` is a high-level library that helps you write compact but full-featured training loops with metrics, early stops, and model checkpoints for deep learning library [PyTorch](https://pytorch.org ). http://bit.ly/34foQ4J
0 notes
Text
Hands on Graph Neural Networks with PyTorch & PyTorch Geometric
http://dlvr.it/R5lFdn
0 notes
Text
Hands on Graph Neural Networks with PyTorch & PyTorch Geometric
http://dlvr.it/R5kS41
0 notes