#develop predictive model using nlp
Explore tagged Tumblr posts
Text
The Future of Artificial Intelligence: Expectations and Possibilities
Artificial Intelligence (AI) is remodeling nearly every element of our lives, from how we work and speak to how we entertain ourselves and clear up complicated problems. As AI maintains to increase, it raises fundamental questions on the future, consisting of how it'll reshape industries, impact society, or even redefine what it manner to be human. This essay explores the predicted future of AI, specializing in improvements, ethical issues, and capacity demanding situations.

Future Of Artifical Intelligence In India
Advancements in AI
AI is advancing at an exceptional price, with several key areas poised for substantial breakthroughs:
1. Machine Learning and Deep Learning
Machine mastering and deep getting to know have driven a whole lot of AI’s development, allowing systems to apprehend patterns, process massive amounts of facts, and make predictions with high accuracy. Future traits in those regions are anticipated to improve AI’s ability to generalize knowledge, decreasing the need for big education statistics and enhancing overall performance across numerous tasks.
2. Natural Language Processing (NLP)
AI’s potential to understand and generate human language has seen fantastic progress through models like GPT-4 and beyond. Future iterations will probable cause extra fluent, nuanced, and context-aware interactions, making AI an even extra valuable device for communique, content material introduction, and translation.
Three. Autonomous Systems and Robotics
Autonomous automobiles, drones, and robotic assistants are becoming increasingly sophisticated. In the future, we can expect AI-powered robots to be greater adaptable and able to performing complicated duties with greater performance. From self-riding vehicles to robot surgeons, AI’s position in automation will expand across more than one sectors.
4. AI in Healthcare
AI is revolutionizing healthcare through early ailment detection, customized medicine, and robotic-assisted surgeries. In the future, AI will allow medical doctors to diagnose situations extra appropriately and offer tailored remedy plans, in the long run enhancing affected person results and extending human lifespan.
5. AI in Creativity and the Arts
AI-generated artwork, tune, and literature are already tough conventional notions of creativity. Future advancements will blur the line among human and gadget-generated creativity, main to new sorts of artistic expression and collaboration.
Ethical and Social Considerations
As AI maintains to strengthen, it brings forth essential ethical and social demanding situations that must be addressed:
1. Bias and Fairness
AI systems regularly reflect biases found in their schooling data, that may cause unfair or discriminatory outcomes. Researchers and builders are operating on ways to create extra honest and independent AI fashions, but this remains an ongoing mission.
2. Job Displacement and Workforce Evolution
Automation powered through AI is expected to replace positive jobs even as developing new ones. While some worry big task losses, others accept as true with AI will enhance human paintings in preference to replace it. Preparing the team of workers for an AI-pushed economic system would require reskilling programs and new instructional procedures.
3. Privacy and Surveillance
AI’s ability to system large amounts of private statistics increases extensive privacy worries. Striking a stability among innovation and protecting man or woman rights might be vital to make certain AI’s responsible development and deployment.
4. AI Governance and Regulation
Ensuring AI is used ethically and responsibly requires effective regulations and governance frameworks. Governments and global agencies are operating to establish suggestions to prevent AI from being misused for malicious functions, such as deepfakes or cyberattacks.
Challenges and Potential Risks
Despite AI’s ability, there are numerous demanding situations and dangers that should be taken into consideration:
1. AI Alignment Problem
Ensuring that AI systems align with human values and dreams is a good sized undertaking. Misaligned AI could lead to unintended outcomes, making it critical to design AI that prioritizes human well-being.
2. Superintelligence and Existential Risks
The opportunity of growing superintelligent AI—structures that surpass human intelligence—increases worries approximately manipulate and safety. Researchers emphasize the significance of enforcing safeguards to save you AI from acting in approaches that might be harmful to humanity.
Three. Ethical Dilemmas in AI Decision-Making
As AI takes on greater duties, it's going to face ethical dilemmas, including figuring out who gets get right of entry to to restrained medical resources or figuring out the route of movement in autonomous motors at some point of injuries. Addressing those dilemmas calls for moral AI layout and obvious decision-making processes.
Top 10 Emerging Tech Trends In 2025
#Future Of Artifical Intelligence In India#artifical intelligence#machine learning#tech#digital marketing
2 notes
·
View notes
Text
MedAI by Tech4Biz Solutions: Pioneering Next-Gen Medical Technologies
The healthcare industry is undergoing a seismic shift as advanced technologies continue to transform the way care is delivered. MedAI by Tech4Biz Solutions is at the forefront of this revolution, leveraging artificial intelligence and cutting-edge tools to develop next-generation medical solutions. By enhancing diagnostics, personalizing patient care, and streamlining operations, MedAI is empowering healthcare providers to deliver better outcomes.
1. AI-Driven Medical Insights
MedAI harnesses the power of artificial intelligence to analyze complex medical data and generate actionable insights. Its advanced algorithms can detect anomalies, predict disease progression, and recommend treatment pathways with unprecedented accuracy.
Case Study: A large medical center integrated MedAI’s diagnostic platform, leading to:
Faster identification of rare conditions.
A 30% reduction in misdiagnoses.
Enhanced clinician confidence in treatment decisions.
These capabilities underscore MedAI’s role in advancing clinical decision-making.
2. Personalized Patient Care
Personalization is key to modern healthcare, and MedAI’s data-driven approach ensures treatment plans are tailored to individual needs. By analyzing patient histories, lifestyle factors, and genetic data, MedAI offers more targeted and effective interventions.
Example: A chronic disease management clinic used MedAI to create personalized care plans, resulting in:
Improved medication adherence.
Decreased hospital readmission rates.
Greater patient satisfaction and engagement.
MedAI’s solutions allow providers to offer more precise, patient-centered care.
3. Enhanced Operational Efficiency
MedAI goes beyond clinical improvements by optimizing healthcare operations. Its automation tools reduce administrative burdens, freeing healthcare professionals to focus on patient care.
Insight: A regional hospital implemented MedAI’s workflow automation system, achieving:
A 40% reduction in administrative errors.
Faster patient registration and billing processes.
Streamlined appointment scheduling.
These improvements enhance overall operational efficiency and patient experiences.
4. Advanced Predictive Analytics
Predictive analytics play a vital role in preventive care. MedAI’s algorithms identify patients at high risk of developing chronic conditions, enabling early interventions.
Case Study: A primary care network used MedAI’s predictive models to monitor high-risk patients, leading to:
Early lifestyle adjustments and medical interventions.
A 25% drop in emergency room visits.
Higher enrollment in wellness programs.
By shifting to proactive care, MedAI helps reduce healthcare costs and improve long-term outcomes.
5. Revolutionizing Telemedicine
The rise of telemedicine has been accelerated by MedAI’s AI-powered virtual care solutions. These tools enhance remote consultations by providing real-time patient insights and symptom analysis.
Example: A telehealth provider adopted MedAI’s platform and reported:
Improved diagnostic accuracy during virtual visits.
Reduced wait times for consultations.
Increased access to care for rural and underserved populations.
MedAI’s telemedicine tools ensure equitable, high-quality virtual care for all.
6. Streamlining Drug Development
MedAI accelerates the drug discovery process by analyzing clinical trial data and simulating drug interactions. Its AI models help identify promising compounds faster and improve trial success rates.
Case Study: A pharmaceutical company partnered with MedAI to enhance its drug development process, achieving:
Faster identification of viable drug candidates.
Shorter trial durations.
Reduced costs associated with trial phases.
These innovations are driving faster development of life-saving medications.
7. Natural Language Processing for Clinical Data
MedAI’s natural language processing (NLP) capabilities extract insights from unstructured medical data, such as physician notes and discharge summaries. This allows for faster retrieval of vital patient information.
Insight: A healthcare system implemented MedAI’s NLP engine and experienced:
Improved documentation accuracy.
Quicker clinical decision-making.
Enhanced risk assessment for high-priority cases.
By automating data extraction, MedAI reduces clinician workloads and improves care quality.
8. Robust Data Security and Compliance
Data security is paramount in healthcare. MedAI employs advanced encryption, threat monitoring, and regulatory compliance measures to safeguard patient information.
Example: A hospital using MedAI’s security solutions reported:
Early detection of potential data breaches.
Full compliance with healthcare privacy regulations.
Increased patient trust and confidence in data protection.
MedAI ensures that sensitive medical data remains secure in an evolving digital landscape.
Conclusion
MedAI by Tech4Biz Solutions is redefining healthcare through its pioneering medical technologies. By delivering AI-driven insights, personalized care, operational efficiency, and robust security, MedAI empowers healthcare providers to navigate the future of medicine with confidence.
As healthcare continues to evolve, MedAI remains a trailblazer, driving innovation that transforms patient care and outcomes. Explore MedAI’s comprehensive solutions today and discover the next frontier of medical excellence.
For More Reachout :https://medai.tech4bizsolutions.com/
3 notes
·
View notes
Text
Understanding Artificial Intelligence: A Comprehensive Guide
Artificial Intelligence (AI) has become one of the most transformative technologies of our time. From powering smart assistants to enabling self-driving cars, AI is reshaping industries and everyday life. In this comprehensive guide, we will explore what AI is, its evolution, various types, real-world applications, and both its advantages and disadvantages. We will also offer practical tips for embracing AI in a responsible manner—all while adhering to strict publishing and SEO standards and Blogger’s policies.
---
1. Introduction
Artificial Intelligence refers to computer systems designed to perform tasks that typically require human intelligence. These tasks include learning, reasoning, problem-solving, and even understanding natural language. Over the past few decades, advancements in machine learning and deep learning have accelerated AI’s evolution, making it an indispensable tool in multiple domains.
---
2. What Is Artificial Intelligence?
At its core, AI is about creating machines or software that can mimic human cognitive functions. There are several key areas within AI:
Machine Learning (ML): A subset of AI where algorithms improve through experience and data. For example, recommendation systems on streaming platforms learn user preferences over time.
Deep Learning: A branch of ML that utilizes neural networks with many layers to analyze various types of data. This technology is behind image and speech recognition systems.
Natural Language Processing (NLP): Enables computers to understand, interpret, and generate human language. Virtual assistants like Siri and Alexa are prime examples of NLP applications.
---
3. A Brief History and Evolution
The concept of artificial intelligence dates back to the mid-20th century, when pioneers like Alan Turing began to question whether machines could think. Over the years, AI has evolved through several phases:
Early Developments: In the 1950s and 1960s, researchers developed simple algorithms and theories on machine learning.
The AI Winter: Due to high expectations and limited computational power, interest in AI waned during the 1970s and 1980s.
Modern Resurgence: The advent of big data, improved computing power, and new algorithms led to a renaissance in AI research and applications, especially in the last decade.
Source: MIT Technology Review
---
4. Types of AI
Understanding AI involves recognizing its different types, which vary in complexity and capability:
4.1 Narrow AI (Artificial Narrow Intelligence - ANI)
Narrow AI is designed to perform a single task or a limited range of tasks. Examples include:
Voice Assistants: Siri, Google Assistant, and Alexa, which respond to specific commands.
Recommendation Engines: Algorithms used by Netflix or Amazon to suggest products or content.
4.2 General AI (Artificial General Intelligence - AGI)
AGI refers to machines that possess the ability to understand, learn, and apply knowledge across a wide range of tasks—much like a human being. Although AGI remains a theoretical concept, significant research is underway to make it a reality.
4.3 Superintelligent AI (Artificial Superintelligence - ASI)
ASI is a level of AI that surpasses human intelligence in all aspects. While it currently exists only in theory and speculative discussions, its potential implications for society drive both excitement and caution.
Source: Stanford University AI Index
---
5. Real-World Applications of AI
AI is not confined to laboratories—it has found practical applications across various industries:
5.1 Healthcare
Medical Diagnosis: AI systems are now capable of analyzing medical images and predicting diseases such as cancer with high accuracy.
Personalized Treatment: Machine learning models help create personalized treatment plans based on a patient’s genetic makeup and history.
5.2 Automotive Industry
Self-Driving Cars: Companies like Tesla and Waymo are developing autonomous vehicles that rely on AI to navigate roads safely.
Traffic Management: AI-powered systems optimize traffic flow in smart cities, reducing congestion and pollution.
5.3 Finance
Fraud Detection: Banks use AI algorithms to detect unusual patterns that may indicate fraudulent activities.
Algorithmic Trading: AI models analyze vast amounts of financial data to make high-speed trading decisions.
5.4 Entertainment
Content Recommendation: Streaming services use AI to analyze viewing habits and suggest movies or shows.
Game Development: AI enhances gaming experiences by creating more realistic non-player character (NPC) behaviors.
Source: Forbes – AI in Business
---
6. Advantages of AI
AI offers numerous benefits across multiple domains:
Efficiency and Automation: AI automates routine tasks, freeing up human resources for more complex and creative endeavors.
Enhanced Decision Making: AI systems analyze large datasets to provide insights that help in making informed decisions.
Improved Personalization: From personalized marketing to tailored healthcare, AI enhances user experiences by addressing individual needs.
Increased Safety: In sectors like automotive and manufacturing, AI-driven systems contribute to improved safety and accident prevention.
---
7. Disadvantages and Challenges
Despite its many benefits, AI also presents several challenges:
Job Displacement: Automation and AI can lead to job losses in certain sectors, raising concerns about workforce displacement.
Bias and Fairness: AI systems can perpetuate biases present in training data, leading to unfair outcomes in areas like hiring or law enforcement.
Privacy Issues: The use of large datasets often involves sensitive personal information, raising concerns about data privacy and security.
Complexity and Cost: Developing and maintaining AI systems requires significant resources, expertise, and financial investment.
Ethical Concerns: The increasing autonomy of AI systems brings ethical dilemmas, such as accountability for decisions made by machines.
Source: Nature – The Ethics of AI
---
8. Tips for Embracing AI Responsibly
For individuals and organizations looking to harness the power of AI, consider these practical tips:
Invest in Education and Training: Upskill your workforce by offering training in AI and data science to stay competitive.
Prioritize Transparency: Ensure that AI systems are transparent in their operations, especially when making decisions that affect individuals.
Implement Robust Data Security Measures: Protect user data with advanced security protocols to prevent breaches and misuse.
Monitor and Mitigate Bias: Regularly audit AI systems for biases and take corrective measures to ensure fair outcomes.
Stay Informed on Regulatory Changes: Keep abreast of evolving legal and ethical standards surrounding AI to maintain compliance and public trust.
Foster Collaboration: Work with cross-disciplinary teams, including ethicists, data scientists, and industry experts, to create well-rounded AI solutions.
---
9. Future Outlook
The future of AI is both promising and challenging. With continuous advancements in technology, AI is expected to become even more integrated into our daily lives. Innovations such as AGI and even discussions around ASI signal potential breakthroughs that could revolutionize every sector—from education and healthcare to transportation and beyond. However, these advancements must be managed responsibly, balancing innovation with ethical considerations to ensure that AI benefits society as a whole.
---
10. Conclusion
Artificial Intelligence is a dynamic field that continues to evolve, offering incredible opportunities while posing significant challenges. By understanding the various types of AI, its real-world applications, and the associated advantages and disadvantages, we can better prepare for an AI-driven future. Whether you are a business leader, a policymaker, or an enthusiast, staying informed and adopting responsible practices will be key to leveraging AI’s full potential.
As we move forward, it is crucial to strike a balance between technological innovation and ethical responsibility. With proper planning, education, and collaboration, AI can be a force for good, driving progress and improving lives around the globe.
---
References
1. MIT Technology Review – https://www.technologyreview.com/
2. Stanford University AI Index – https://aiindex.stanford.edu/
3. Forbes – https://www.forbes.com/
4. Nature – https://www.nature.com/
---
Meta Description:
Explore our comprehensive 1,000-word guide on Artificial Intelligence, covering its history, types, real-world applications, advantages, disadvantages, and practical tips for responsible adoption. Learn how AI is shaping the future while addressing ethical and operational challenges.
2 notes
·
View notes
Text
How-To IT
Topic: Core areas of IT
1. Hardware
• Computers (Desktops, Laptops, Workstations)
• Servers and Data Centers
• Networking Devices (Routers, Switches, Modems)
• Storage Devices (HDDs, SSDs, NAS)
• Peripheral Devices (Printers, Scanners, Monitors)
2. Software
• Operating Systems (Windows, Linux, macOS)
• Application Software (Office Suites, ERP, CRM)
• Development Software (IDEs, Code Libraries, APIs)
• Middleware (Integration Tools)
• Security Software (Antivirus, Firewalls, SIEM)
3. Networking and Telecommunications
• LAN/WAN Infrastructure
• Wireless Networking (Wi-Fi, 5G)
• VPNs (Virtual Private Networks)
• Communication Systems (VoIP, Email Servers)
• Internet Services
4. Data Management
• Databases (SQL, NoSQL)
• Data Warehousing
• Big Data Technologies (Hadoop, Spark)
• Backup and Recovery Systems
• Data Integration Tools
5. Cybersecurity
• Network Security
• Endpoint Protection
• Identity and Access Management (IAM)
• Threat Detection and Incident Response
• Encryption and Data Privacy
6. Software Development
• Front-End Development (UI/UX Design)
• Back-End Development
• DevOps and CI/CD Pipelines
• Mobile App Development
• Cloud-Native Development
7. Cloud Computing
• Infrastructure as a Service (IaaS)
• Platform as a Service (PaaS)
• Software as a Service (SaaS)
• Serverless Computing
• Cloud Storage and Management
8. IT Support and Services
• Help Desk Support
• IT Service Management (ITSM)
• System Administration
• Hardware and Software Troubleshooting
• End-User Training
9. Artificial Intelligence and Machine Learning
• AI Algorithms and Frameworks
• Natural Language Processing (NLP)
• Computer Vision
• Robotics
• Predictive Analytics
10. Business Intelligence and Analytics
• Reporting Tools (Tableau, Power BI)
• Data Visualization
• Business Analytics Platforms
• Predictive Modeling
11. Internet of Things (IoT)
• IoT Devices and Sensors
• IoT Platforms
• Edge Computing
• Smart Systems (Homes, Cities, Vehicles)
12. Enterprise Systems
• Enterprise Resource Planning (ERP)
• Customer Relationship Management (CRM)
• Human Resource Management Systems (HRMS)
• Supply Chain Management Systems
13. IT Governance and Compliance
• ITIL (Information Technology Infrastructure Library)
• COBIT (Control Objectives for Information Technologies)
• ISO/IEC Standards
• Regulatory Compliance (GDPR, HIPAA, SOX)
14. Emerging Technologies
• Blockchain
• Quantum Computing
• Augmented Reality (AR) and Virtual Reality (VR)
• 3D Printing
• Digital Twins
15. IT Project Management
• Agile, Scrum, and Kanban
• Waterfall Methodology
• Resource Allocation
• Risk Management
16. IT Infrastructure
• Data Centers
• Virtualization (VMware, Hyper-V)
• Disaster Recovery Planning
• Load Balancing
17. IT Education and Certifications
• Vendor Certifications (Microsoft, Cisco, AWS)
• Training and Development Programs
• Online Learning Platforms
18. IT Operations and Monitoring
• Performance Monitoring (APM, Network Monitoring)
• IT Asset Management
• Event and Incident Management
19. Software Testing
• Manual Testing: Human testers evaluate software by executing test cases without using automation tools.
• Automated Testing: Use of testing tools (e.g., Selenium, JUnit) to run automated scripts and check software behavior.
• Functional Testing: Validating that the software performs its intended functions.
• Non-Functional Testing: Assessing non-functional aspects such as performance, usability, and security.
• Unit Testing: Testing individual components or units of code for correctness.
• Integration Testing: Ensuring that different modules or systems work together as expected.
• System Testing: Verifying the complete software system’s behavior against requirements.
• Acceptance Testing: Conducting tests to confirm that the software meets business requirements (including UAT - User Acceptance Testing).
• Regression Testing: Ensuring that new changes or features do not negatively affect existing functionalities.
• Performance Testing: Testing software performance under various conditions (load, stress, scalability).
• Security Testing: Identifying vulnerabilities and assessing the software’s ability to protect data.
• Compatibility Testing: Ensuring the software works on different operating systems, browsers, or devices.
• Continuous Testing: Integrating testing into the development lifecycle to provide quick feedback and minimize bugs.
• Test Automation Frameworks: Tools and structures used to automate testing processes (e.g., TestNG, Appium).
19. VoIP (Voice over IP)
VoIP Protocols & Standards
• SIP (Session Initiation Protocol)
• H.323
• RTP (Real-Time Transport Protocol)
• MGCP (Media Gateway Control Protocol)
VoIP Hardware
• IP Phones (Desk Phones, Mobile Clients)
• VoIP Gateways
• Analog Telephone Adapters (ATAs)
• VoIP Servers
• Network Switches/ Routers for VoIP
VoIP Software
• Softphones (e.g., Zoiper, X-Lite)
• PBX (Private Branch Exchange) Systems
• VoIP Management Software
• Call Center Solutions (e.g., Asterisk, 3CX)
VoIP Network Infrastructure
• Quality of Service (QoS) Configuration
• VPNs (Virtual Private Networks) for VoIP
• VoIP Traffic Shaping & Bandwidth Management
• Firewall and Security Configurations for VoIP
• Network Monitoring & Optimization Tools
VoIP Security
• Encryption (SRTP, TLS)
• Authentication and Authorization
• Firewall & Intrusion Detection Systems
• VoIP Fraud DetectionVoIP Providers
• Hosted VoIP Services (e.g., RingCentral, Vonage)
• SIP Trunking Providers
• PBX Hosting & Managed Services
VoIP Quality and Testing
• Call Quality Monitoring
• Latency, Jitter, and Packet Loss Testing
• VoIP Performance Metrics and Reporting Tools
• User Acceptance Testing (UAT) for VoIP Systems
Integration with Other Systems
• CRM Integration (e.g., Salesforce with VoIP)
• Unified Communications (UC) Solutions
• Contact Center Integration
• Email, Chat, and Video Communication Integration
2 notes
·
View notes
Text
How to Leverage Engagement With AI For Content Creation
Content creation has become essential any successful online marketing strategy in this digital era. However, creating high-quality, engaging content can often be time-consuming and resource-intensive. Herein lies the role of AI for content creation. By leveraging advanced artificial intelligence tools, businesses and content creators can streamline their content production process, boost engagement, and enhance the overall quality of their output.
AI tools can assist in generating ideas, drafting content, optimizing for SEO, and even personalizing content to specific audience segments. AI's analyzation and track vast amounts of data allows it to predict audience preferences and generate content that resonates with them, improving interaction and engagement levels.
Here, we will explore how content creation AI works, how to leverage it to increase audience engagement, and why AI is becoming an indispensable tool for content marketers and creators. Whether seasoned content creator or a newbie, understanding how to utilize AI in your content strategy will help you stay ahead of the curve.
What Is AI for Content Creation?
Production of content Artificial Intelligence (AI) is the use of AI technologies to help with content creation, optimization, and enhancement. These technologies can generate written text, images, videos, and audio based on user inputs, historical data, and predefined goals. From natural language processing (NLP) models to machine learning algorithms that analyze audience behavior, AI systems streamline content creation processes.
AI tools can assist in content creation by automating repetitive tasks, such as drafting blog posts, suggesting headlines, or performing keyword research. Some AI tools also use predictive algorithms to determine which types of content will resonate most with a target audience. For example, AI can analyze trends in search queries, social media interactions, and user behaviors to help creators produce timely and relevant content.
Also, AI is also evolving to assist in video and graphic content creation. Tools like Canva, powered by AI, can help marketers and creators develop visually appealing content by automating the design process, recommending layout options, and even generating visuals based on written input.
Leveraging Engagement with AI for Content Creation
Personalized Content
One of the key ways to enhance engagement through content AI is through its ability to personalize content. AI tools can analyze data from user behavior, preferences, and previous interactions to help content creators tailor their messaging. Personalized content increases the likelihood of engaging users because it speaks directly to their interests and needs.
AI for SEO Optimization
Search engine optimization (SEO) is a critical component of content marketing, but can be tedious and complex. Content creation AI can automate much of the SEO process, helping creators rank higher in search engine results. AI tools can identify the best keywords, suggest meta descriptions, and optimize content for readability.
Content Scheduling and Consistency
Consistency is crucial for maintaining audience engagement, but managing content publishing schedules can be overwhelming. By automating the scheduling of material, AI can help to expedite this process. Tools like Buffer or Hootsuite use AI to analyze audience behavior patterns and determine the best times to post content for maximum engagement.
Enhanced Visual Content Creation
Content is not limited to text alone; visuals play an equally important role in engaging audiences. AI tools can assist creators in producing high-quality visuals that align with the content. Whether generating infographics, optimizing images for SEO, or creating short videos, AI can significantly enhance the visual appeal of content, making it more shareable and interactive.
Analyzing User Feedback
One of the most essential aspects of creating engaging content is to know your audience. AI tools can help you analyze user feedback, comments, and behavior to gauge how well your content is performing. AI provides valuable insights into what works by tracking engagement metrics like click-through rates, social shares, and time spent on a page.
You can also watch: Meet AdsGPT’s Addie| Smarter Ad Copy Creation In Seconds
youtube
Wrapping Up!!!
As digital landscapes evolve, AI for content creation offers a influential advantage for content creators and marketers looking to increase engagement and streamline their processes. From personalizing content to optimizing SEO strategies and automating repetitive tasks, AI makes content creation faster, more efficient, and ultimately more effective. By embracing AI-powered tools and techniques, content creators can stay ahead of the competition and keep their audiences engaged through high-quality, targeted content. The future of content creation lies in harnessing the full potential of AI, which not only helps save time but also ensures that your content resonates with the right people at the right time. AI integration into your content strategy is now a must for success in this cutthroat digital environment.
3 notes
·
View notes
Text
Transfer Learning in NLP: Impact of BERT, GPT-3, and T5 on NLP Tasks
Transfer learning has revolutionized the field of Natural Language Processing (NLP) by allowing models to leverage pre-trained knowledge on large datasets for various downstream tasks. Among the most impactful models in this domain are BERT, GPT-3, and T5. Let's explore these models and their significance in NLP.
1. BERT (Bidirectional Encoder Representations from Transformers)
Overview:
Developed by Google, BERT was introduced in 2018 and marked a significant leap in NLP by using bidirectional training of Transformer models.
Unlike previous models that processed text in a unidirectional manner, BERT looks at both left and right context in all layers, providing a deeper understanding of the language.
Key Features:
Bidirectional Contextual Understanding: BERT’s bidirectional approach allows it to understand the context of a word based on both preceding and following words.
Pre-training Tasks: BERT uses two pre-training tasks – Masked Language Modeling (MLM) and Next Sentence Prediction (NSP). MLM involves predicting masked words in a sentence, while NSP involves predicting if two sentences follow each other in the text.
Impact on NLP Tasks:
Text Classification: BERT's contextual understanding improves the performance of text classification tasks like sentiment analysis and spam detection.
Named Entity Recognition (NER): BERT enhances NER tasks by accurately identifying entities in the text due to its deep understanding of the context.
Question Answering: BERT has set new benchmarks in QA tasks, as it can effectively comprehend and answer questions based on given contexts.
2. GPT-3 (Generative Pre-trained Transformer 3)
Overview:
Developed by OpenAI, GPT-3 is one of the largest language models ever created, with 175 billion parameters.
It follows a unidirectional (left-to-right) autoregressive approach, generating text based on the preceding words.
Key Features:
Scale and Size: GPT-3’s massive size allows it to generate highly coherent and contextually relevant text, making it suitable for a wide range of applications.
Few-Shot Learning: GPT-3 can perform tasks with minimal examples, reducing the need for large labeled datasets for fine-tuning.
Impact on NLP Tasks:
Text Generation: GPT-3 excels at generating human-like text, making it useful for creative writing, chatbots, and content creation.
Translation: While not specifically trained for translation, GPT-3 can perform reasonably well in translating text between languages due to its extensive pre-training.
Code Generation: GPT-3 can generate code snippets and assist in programming tasks, demonstrating its versatility beyond traditional NLP tasks.
3. T5 (Text-to-Text Transfer Transformer)
Overview:
Developed by Google, T5 frames all NLP tasks as a text-to-text problem, where both input and output are text strings.
This unified approach allows T5 to handle a wide variety of tasks with a single model architecture.
Key Features:
Text-to-Text Framework: By converting tasks like translation, summarization, and question answering into a text-to-text format, T5 simplifies the process of applying the model to different tasks.
Pre-training on Diverse Datasets: T5 is pre-trained on the C4 dataset (Colossal Clean Crawled Corpus), which provides a rich and diverse training set.
Impact on NLP Tasks:
Summarization: T5 achieves state-of-the-art results in text summarization by effectively condensing long documents into concise summaries.
Translation: T5 performs competitively in translation tasks by leveraging its text-to-text framework to handle multiple language pairs.
Question Answering and More: T5's versatility allows it to excel in various tasks, including QA, sentiment analysis, and more, by simply changing the text inputs and outputs.
Conclusion
BERT, GPT-3, and T5 have significantly advanced the field of NLP through their innovative architectures and pre-training techniques. These models have set new benchmarks across various NLP tasks, demonstrating the power and versatility of transfer learning. By leveraging large-scale pre-training, they enable efficient fine-tuning on specific tasks, reducing the need for extensive labeled datasets and accelerating the development of NLP applications.
These models have not only improved the performance of existing tasks but have also opened up new possibilities in areas like creative text generation, few-shot learning, and unified task frameworks, paving the way for future advancements in NLP.
3 notes
·
View notes
Text
SEMANTIC TREE AND AI TECHNOLOGIES
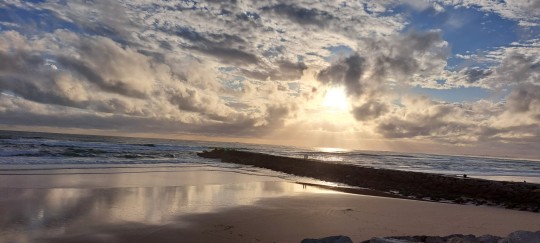
Semantic Tree learning and AI technologies can be combined to solve problems by leveraging the power of natural language processing and machine learning.
Semantic trees are a knowledge representation technique that organizes information in a hierarchical, tree-like structure.
Each node in the tree represents a concept or entity, and the connections between nodes represent the relationships between those concepts.
This structure allows for the representation of complex, interconnected knowledge in a way that can be easily navigated and reasoned about.
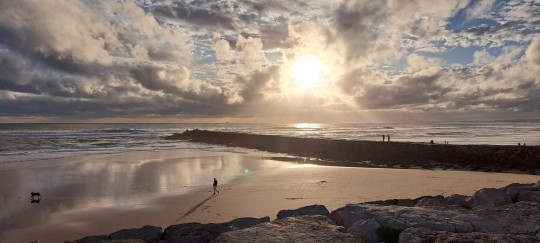


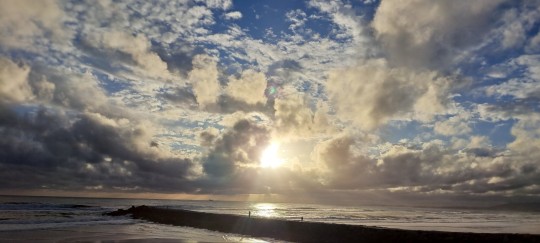

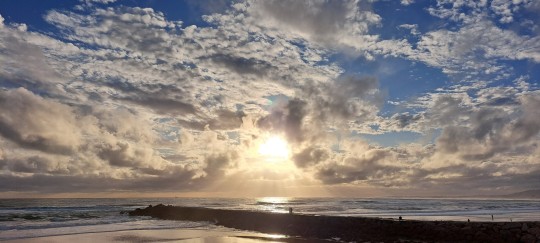
CONCEPTS
Semantic Tree: A structured representation where nodes correspond to concepts and edges denote relationships (e.g., hyponyms, hyponyms, synonyms).
Meaning: Understanding the context, nuances, and associations related to words or concepts.
Natural Language Understanding (NLU): AI techniques for comprehending and interpreting human language.
First Principles: Fundamental building blocks or core concepts in a domain.
AI (Artificial Intelligence): AI refers to the development of computer systems that can perform tasks that typically require human intelligence. AI technologies include machine learning, natural language processing, computer vision, and more. These technologies enable computers to understand reason, learn, and make decisions.
Natural Language Processing (NLP): NLP is a branch of AI that focuses on the interaction between computers and human language. It involves the analysis and understanding of natural language text or speech by computers. NLP techniques are used to process, interpret, and generate human languages.
Machine Learning (ML): Machine Learning is a subset of AI that enables computers to learn and improve from experience without being explicitly programmed. ML algorithms can analyze data, identify patterns, and make predictions or decisions based on the learned patterns.
Deep Learning: A subset of machine learning that uses neural networks with multiple layers to learn complex patterns.
EXAMPLES OF APPLYING SEMANTIC TREE LEARNING WITH AI.
1. Text Classification: Semantic Tree learning can be combined with AI to solve text classification problems. By training a machine learning model on labeled data, the model can learn to classify text into different categories or labels. For example, a customer support system can use semantic tree learning to automatically categorize customer queries into different topics, such as billing, technical issues, or product inquiries.
2. Sentiment Analysis: Semantic Tree learning can be used with AI to perform sentiment analysis on text data. Sentiment analysis aims to determine the sentiment or emotion expressed in a piece of text, such as positive, negative, or neutral. By analyzing the semantic structure of the text using Semantic Tree learning techniques, machine learning models can classify the sentiment of customer reviews, social media posts, or feedback.
3. Question Answering: Semantic Tree learning combined with AI can be used for question answering systems. By understanding the semantic structure of questions and the context of the information being asked, machine learning models can provide accurate and relevant answers. For example, a Chabot can use Semantic Tree learning to understand user queries and provide appropriate responses based on the analyzed semantic structure.
4. Information Extraction: Semantic Tree learning can be applied with AI to extract structured information from unstructured text data. By analyzing the semantic relationships between entities and concepts in the text, machine learning models can identify and extract specific information. For example, an AI system can extract key information like names, dates, locations, or events from news articles or research papers.
Python Snippet Codes for Semantic Tree Learning with AI
Here are four small Python code snippets that demonstrate how to apply Semantic Tree learning with AI using popular libraries:
1. Text Classification with scikit-learn:
```python
from sklearn.feature_extraction.text import TfidfVectorizer
from sklearn.linear_model import LogisticRegression
# Training data
texts = ['This is a positive review', 'This is a negative review', 'This is a neutral review']
labels = ['positive', 'negative', 'neutral']
# Vectorize the text data
vectorizer = TfidfVectorizer()
X = vectorizer.fit_transform(texts)
# Train a logistic regression classifier
classifier = LogisticRegression()
classifier.fit(X, labels)
# Predict the label for a new text
new_text = 'This is a positive sentiment'
new_text_vectorized = vectorizer.transform([new_text])
predicted_label = classifier.predict(new_text_vectorized)
print(predicted_label)
```
2. Sentiment Analysis with TextBlob:
```python
from textblob import TextBlob
# Analyze sentiment of a text
text = 'This is a positive sentence'
blob = TextBlob(text)
sentiment = blob.sentiment.polarity
# Classify sentiment based on polarity
if sentiment > 0:
sentiment_label = 'positive'
elif sentiment < 0:
sentiment_label = 'negative'
else:
sentiment_label = 'neutral'
print(sentiment_label)
```
3. Question Answering with Transformers:
```python
from transformers import pipeline
# Load the question answering model
qa_model = pipeline('question-answering')
# Provide context and ask a question
context = 'The Semantic Web is an extension of the World Wide Web.'
question = 'What is the Semantic Web?'
# Get the answer
answer = qa_model(question=question, context=context)
print(answer['answer'])
```
4. Information Extraction with spaCy:
```python
import spacy
# Load the English language model
nlp = spacy.load('en_core_web_sm')
# Process text and extract named entities
text = 'Apple Inc. is planning to open a new store in New York City.'
doc = nlp(text)
# Extract named entities
entities = [(ent.text, ent.label_) for ent in doc.ents]
print(entities)
```
APPLICATIONS OF SEMANTIC TREE LEARNING WITH AI
Semantic Tree learning combined with AI can be used in various domains and industries to solve problems. Here are some examples of where it can be applied:
1. Customer Support: Semantic Tree learning can be used to automatically categorize and route customer queries to the appropriate support teams, improving response times and customer satisfaction.
2. Social Media Analysis: Semantic Tree learning with AI can be applied to analyze social media posts, comments, and reviews to understand public sentiment, identify trends, and monitor brand reputation.
3. Information Retrieval: Semantic Tree learning can enhance search engines by understanding the meaning and context of user queries, providing more accurate and relevant search results.
4. Content Recommendation: By analyzing the semantic structure of user preferences and content metadata, Semantic Tree learning with AI can be used to personalize content recommendations in platforms like streaming services, news aggregators, or e-commerce websites.
Semantic Tree learning combined with AI technologies enables the understanding and analysis of text data, leading to improved problem-solving capabilities in various domains.
COMBINING SEMANTIC TREE AND AI FOR PROBLEM SOLVING
1. Semantic Reasoning: By integrating semantic trees with AI, systems can engage in more sophisticated reasoning and decision-making. The semantic tree provides a structured representation of knowledge, while AI techniques like natural language processing and knowledge representation can be used to navigate and reason about the information in the tree.
2. Explainable AI: Semantic trees can make AI systems more interpretable and explainable. The hierarchical structure of the tree can be used to trace the reasoning process and understand how the system arrived at a particular conclusion, which is important for building trust in AI-powered applications.
3. Knowledge Extraction and Representation: AI techniques like machine learning can be used to automatically construct semantic trees from unstructured data, such as text or images. This allows for the efficient extraction and representation of knowledge, which can then be used to power various problem-solving applications.
4. Hybrid Approaches: Combining semantic trees and AI can lead to hybrid approaches that leverage the strengths of both. For example, a system could use a semantic tree to represent domain knowledge and then apply AI techniques like reinforcement learning to optimize decision-making within that knowledge structure.
EXAMPLES OF APPLYING SEMANTIC TREE AND AI FOR PROBLEM SOLVING
1. Medical Diagnosis: A semantic tree could represent the relationships between symptoms, diseases, and treatments. AI techniques like natural language processing and machine learning could be used to analyze patient data, navigate the semantic tree, and provide personalized diagnosis and treatment recommendations.
2. Robotics and Autonomous Systems: Semantic trees could be used to represent the knowledge and decision-making processes of autonomous systems, such as self-driving cars or drones. AI techniques like computer vision and reinforcement learning could be used to navigate the semantic tree and make real-time decisions in dynamic environments.
3. Financial Analysis: Semantic trees could be used to model complex financial relationships and market dynamics. AI techniques like predictive analytics and natural language processing could be applied to the semantic tree to identify patterns, make forecasts, and support investment decisions.
4. Personalized Recommendation Systems: Semantic trees could be used to represent user preferences, interests, and behaviors. AI techniques like collaborative filtering and content-based recommendation could be used to navigate the semantic tree and provide personalized recommendations for products, content, or services.
PYTHON CODE SNIPPETS
1. Semantic Tree Construction using NetworkX:
```python
import networkx as nx
import matplotlib.pyplot as plt
# Create a semantic tree
G = nx.DiGraph()
G.add_node("root", label="Root")
G.add_node("concept1", label="Concept 1")
G.add_node("concept2", label="Concept 2")
G.add_node("concept3", label="Concept 3")
G.add_edge("root", "concept1")
G.add_edge("root", "concept2")
G.add_edge("concept2", "concept3")
# Visualize the semantic tree
pos = nx.spring_layout(G)
nx.draw(G, pos, with_labels=True)
plt.show()
```
2. Semantic Reasoning using PyKEEN:
```python
from pykeen.models import TransE
from pykeen.triples import TriplesFactory
# Load a knowledge graph dataset
tf = TriplesFactory.from_path("./dataset/")
# Train a TransE model on the knowledge graph
model = TransE(triples_factory=tf)
model.fit(num_epochs=100)
# Perform semantic reasoning
head = "concept1"
relation = "isRelatedTo"
tail = "concept3"
score = model.score_hrt(head, relation, tail)
print(f"The score for the triple ({head}, {relation}, {tail}) is: {score}")
```
3. Knowledge Extraction using spaCy:
```python
import spacy
# Load the spaCy model
nlp = spacy.load("en_core_web_sm")
# Extract entities and relations from text
text = "The quick brown fox jumps over the lazy dog."
doc = nlp(text)
# Visualize the extracted knowledge
from spacy import displacy
displacy.render(doc, style="ent")
```
4. Hybrid Approach using Ray:
```python
import ray
from ray.rllib.agents.ppo import PPOTrainer
from ray.rllib.env.multi_agent_env import MultiAgentEnv
from ray.rllib.models.tf.tf_modelv2 import TFModelV2
# Define a custom model that integrates a semantic tree
class SemanticTreeModel(TFModelV2):
def __init__(self, obs_space, action_space, num_outputs, model_config, name):
super().__init__(obs_space, action_space, num_outputs, model_config, name)
# Implement the integration of the semantic tree with the neural network
# Define a multi-agent environment that uses the semantic tree model
class SemanticTreeEnv(MultiAgentEnv):
def __init__(self):
self.semantic_tree = # Initialize the semantic tree
self.agents = # Define the agents
def step(self, actions):
# Implement the environment dynamics using the semantic tree
# Train the hybrid model using Ray
ray.init()
config = {
"env": SemanticTreeEnv,
"model": {
"custom_model": SemanticTreeModel,
},
}
trainer = PPOTrainer(config=config)
trainer.train()
```
APPLICATIONS
The combination of semantic trees and AI can be applied to a wide range of problem domains, including:
- Healthcare: Improving medical diagnosis, treatment planning, and drug discovery.
- Finance: Enhancing investment strategies, risk management, and fraud detection.
- Robotics and Autonomous Systems: Enabling more intelligent and adaptable decision-making in complex environments.
- Education: Personalizing learning experiences and providing intelligent tutoring systems.
- Smart Cities: Optimizing urban planning, transportation, and resource management.
- Environmental Conservation: Modeling and predicting environmental changes, and supporting sustainable decision-making.
- Chatbots and Virtual Assistants:
Use semantic trees to understand user queries and provide context-aware responses.
Apply NLU models to extract meaning from user input.
- Information Retrieval:
Build semantic search engines that understand user intent beyond keyword matching.
Combine semantic trees with vector embeddings (e.g., BERT) for better search results.
- Medical Diagnosis:
Create semantic trees for medical conditions, symptoms, and treatments.
Use AI to match patient symptoms to relevant diagnoses.
- Automated Content Generation:
Construct semantic trees for topics (e.g., climate change, finance).
Generate articles, summaries, or reports based on semantic understanding.
RDIDINI PROMPT ENGINEER
#semantic tree#ai solutions#ai-driven#ai trends#ai system#ai model#ai prompt#ml#ai predictions#llm#dl#nlp
3 notes
·
View notes
Text
How Artificial Intelligence Will Change the Future of Marketing

Businesses have used artificial intelligence in several operations, and marketing is no exception to this phenomenon. Corporations want to know the impact of AI on marketing research outsourcing to evaluate whether to invest research budgets in artificial intelligence applications. This post will summarize the different aspects of the future of AI in marketing.
What is Artificial Intelligence (AI) in Marketing?
Artificial intelligence integrates extensive machine learning models to facilitate the engineering and deployment of self-aware technologies. Therefore, market intelligence firms explore the use cases of AI in marketing.
How is AI used in advanced marketing techniques? Adaptation intelligence can help you identify customer segments more efficiently and anonymously. AI solutions save your time and company resources by identifying new opportunities through market research outsourcing.
Besides, AI systems become smarter with time and usage. So, corporations increasingly rely on them for cost optimization and budget projection. Both paid and organic marketing techniques benefit from AI. Likewise, you can develop multiple marketing campaigns targeting precise geolocation. Companies can also offer personalization services without exposing personal data.
Impact of AI on Marketing
1| Automated Moderation in Community Marketing
Community-based marketing involves creating online spaces where consumers, employees, and other stakeholders can interact proactively. You can often create invitation-only communities for different customer tiers. Consider market research outsourcing to discover trends and strategies in community marketing.
The exclusivity of private or restricted communities helps you review the content without being overwhelmed. After all, customers pay for the membership indirectly when purchasing a product or service from you. However, many brands have publicly available online communities that act as consumer education platforms.
The effectiveness of community marketing relies on creating a healthy environment to make different customer segments feel welcome and appreciated. Simultaneously, uncivilized behaviors threaten the appeal of online communities. Therefore, market intelligence firms recommend using AI-powered content moderation tools for community marketing. E.g., protecting community members from online harassment and spam.
2| AI Used in Chatbot Marketing
Conversational AI chatbots recreate social media messaging experience for website visitors and virtual helpdesks. These techniques, used by market intelligence firms, combine natural language processing (NLP) capabilities with intuitive user interfaces.
Therefore, you feel like you are talking to an actual human. Meanwhile, an algorithm interacts with you from beyond the screen. Moreover, the AI responses are less formulaic or predictable, unlike the scripted chatbots. So, you get contextual messages and a more organic feel. Modern chatbots highlight the future of AI in marketing, where any company can use always-on, lead nourishing interactions.
AI chatbots can also improve market research outsourcing by converting online customer surveys into more personable messages. For example, AI chatbot marketing can collect data on a consumer’s profession via exciting conversations instead of an empty form field accompanied by boring instructions.
3| How is AI Used in Targeted Marketing?
Online marketing is no longer an optional activity, but it is a highly competitive landscape. Therefore, all corporations must leverage market intelligence firms to explore and implement AI-powered targeted bid optimization.
Keyword research and bidding for targeted marketing slots on a website or a video are important considerations in digital marketing management. Artificial intelligence firms streamline these processes by facilitating automated bid adjustments for increased exposure in paid marketing techniques.
Targeted marketing helps you create memorable customer experiences using personal or demographic characteristics data.
Consider how a young medical student has different priorities than a married person with two kids who is about to retire. So, AI-enabled targeted marketing will adjust your bids to achieve a greater impact. This facility prevents inefficient spending on irrelevant ad impressions.
Conclusion
The future of AI in marketing is promising on multiple fronts. AI chatbots enhance consumer engagement while making market research outsourcing surveys more dynamic. Artificial intelligence also helps you maximize the effectiveness of your marketing campaign via smart auto-bidding.
AI is crucial to increasing the reliability of automated content moderation tools used in community marketing. Besides, reputable firms utilize artificial intelligence to validate consumer responses in market research.
A leader among market intelligence firms, SG Analytics, empowers organizations to acquire actionable marketing insights for detailed benchmark studies. Contact us today to increase your competitive edge and market share.
2 notes
·
View notes
Text
How AI is Reshaping the Future of Fintech Technology
In the rapidly evolving landscape of financial technology (fintech), the integration of artificial intelligence (AI) is reshaping the future in profound ways. From revolutionizing customer experiences to optimizing operational efficiency, AI is unlocking new opportunities for innovation and growth across the fintech ecosystem. As a pioneer in fintech software development, Xettle Technologies is at the forefront of leveraging AI to drive transformative change and shape the future of finance.
Fintech technology encompasses a wide range of solutions, including digital banking, payment processing, wealth management, and insurance. In each of these areas, AI is playing a pivotal role in driving innovation, enhancing competitiveness, and delivering value to businesses and consumers alike.
One of the key areas where AI is reshaping the future of fintech technology is in customer experiences. Through techniques such as natural language processing (NLP) and machine learning, AI-powered chatbots and virtual assistants are revolutionizing the way customers interact with financial institutions.
Xettle Technologies has pioneered the integration of AI-powered chatbots into its digital banking platforms, providing customers with personalized assistance and support around the clock. These chatbots can understand and respond to natural language queries, provide account information, offer product recommendations, and even execute transactions, all in real-time. By delivering seamless and intuitive experiences, AI-driven chatbots enhance customer satisfaction, increase engagement, and drive loyalty.
Moreover, AI is enabling financial institutions to gain deeper insights into customer behavior, preferences, and needs. Through advanced analytics and predictive modeling, AI algorithms can analyze vast amounts of data to identify patterns, trends, and correlations that were previously invisible to human analysts.
Xettle Technologies' AI-powered analytics platforms leverage machine learning to extract actionable insights from transaction data, social media activity, and other sources. By understanding customer preferences and market dynamics more accurately, businesses can tailor their offerings, refine their marketing strategies, and drive growth in targeted segments.
AI is also transforming the way financial institutions manage risk and detect fraud. Through the use of advanced algorithms and data analytics, AI can analyze transaction patterns, detect anomalies, and identify potential threats in real-time.
Xettle Technologies has developed sophisticated fraud detection systems that leverage AI to monitor transactions, identify suspicious activity, and prevent fraudulent transactions before they occur. By continuously learning from new data and adapting to emerging threats, these AI-powered systems provide businesses with robust security measures and peace of mind.
In addition to enhancing customer experiences and mitigating risks, AI is driving operational efficiency and innovation in fintech software development. Through techniques such as robotic process automation (RPA) and intelligent workflow management, AI-powered systems can automate routine tasks, streamline processes, and accelerate time-to-market for new products and services.
Xettle Technologies has embraced AI-driven automation across its software development lifecycle, from code generation and testing to deployment and maintenance. By automating repetitive tasks and optimizing workflows, Xettle's development teams can focus on innovation and value-added activities, delivering high-quality fintech solutions more efficiently and effectively.
Looking ahead, the integration of AI into fintech technology is expected to accelerate, driven by advancements in machine learning, natural language processing, and computational power. As AI algorithms become more sophisticated and data sources become more diverse, the potential for innovation in fintech software is virtually limitless.
For Xettle Technologies, this presents a unique opportunity to continue pushing the boundaries of what is possible in fintech innovation. By investing in research and development, forging strategic partnerships, and staying ahead of emerging trends, Xettle is committed to delivering cutting-edge solutions that empower businesses, drive growth, and shape the future of finance.
In conclusion, AI is reshaping the future of fintech technology in profound and exciting ways. From enhancing customer experiences and mitigating risks to driving operational efficiency and innovation, AI-powered solutions hold immense potential for businesses and consumers alike. As a leader in fintech software development, Xettle Technologies is at the forefront of this transformation, leveraging AI to drive meaningful change and shape the future of finance.
#Fintech Technologies#Fintech Software#Artificial Intelligence#Finance#Fintech Startups#technology#ecommerce#fintech#xettle technologies#writers on tumblr
6 notes
·
View notes
Text
Reason Why AI Chatbots Are Becoming More Intelligent?
Introduction:
AI chatbots have come a long way from their early days of producing responses. With the advancement in technology, these intelligent conversation partners have transformed various industries. This article scrabbles into the reasons why AI chatbots are becoming more intelligent and explores the key components behind their evolution.
Understanding AI Chatbots:
Defining AI chatbots and their key components
AI chatbots are computer programs that are designed to simulate conversations with human users. They rely on natural language processing (NLP), machine learning, and other AI techniques to understand user queries and provide appropriate responses. The key components of AI chatbots include a language understanding module, a dialog management system, and a language generation component.
Types of AI chatbots and their functionalities
There are different types of AI chatbots, each serving a specific purpose. Rule-based chatbots follow a predefined set of rules and provide answers based on the programmed responses. On the other hand, machine-learning chatbots employ algorithms that enable them to learn from users' interactions and improve their responses over time.
How AI chatbots learn and adapt
AI chatbots learn and adapt through a process known as training. Initially, the chatbot is provided with a dataset of conversations and relevant information. Through machine learning algorithms, it analyzes this data and creates patterns to respond more intelligently. As users interact with the chatbot, it further improves its understanding and adaptation capabilities.
The Role of Natural Language Processing (NLP):
Exploring the significance of NLP in AI chatbots' intelligence
NLP plays a crucial role in enhancing the intelligence of AI chatbots. It enables them to understand and interpret human language, including complex sentences, slang, and context-dependent meanings. By leveraging NLP techniques, chatbots can provide more accurate and contextually relevant responses.
NLP techniques used for language understanding and generation
To understand user queries, AI chatbots employ various NLP techniques such as tokenization, part-of-speech tagging, and named entity recognition. These techniques help in breaking down the text, identifying the grammatical structure, and extracting important information. Techniques like sentiment analysis and language generation models are utilized for generating responses.
Machine Learning in AI Chatbots:
Unveiling the role of machine learning algorithms in enhancing chatbot intelligence
Machine learning algorithms play a crucial role in augmenting the intelligence of AI chatbots. They enable chatbots to learn patterns from vast amounts of data, identify trends, and make accurate predictions. This allows chatbots to provide personalized and contextually appropriate responses.
Supervised, unsupervised, and reinforcement learning in chatbot development
In chatbot development, various machine-learning techniques are employed. Supervised learning involves training the chatbot using labeled data, where each input is associated with a correct output. Unsupervised learning, on the other hand, allows the chatbot to discover patterns and correlations within the data without explicit labels. Reinforcement learning is utilized to reward the chatbot for making correct decisions and penalize it for incorrect ones, allowing it to optimize its performance.
Deep Learning and Neural Networks in Chatbots:
Discovering the power of deep learning and neural networks in chatbot advancements
Deep learning and neural networks have significantly contributed to the advancements in chatbot technology. By leveraging deep learning models, chatbots can process complex data, recognize patterns, and generate more accurate responses. Neural networks, with their interconnected layers of artificial neurons, enable chatbots to learn and adapt in a way that resembles the human brain.
How deep learning models improve chatbot understanding and responses
Deep learning models enhance chatbot understanding by learning from vast amounts of data, allowing them to recognize patterns, semantics, and context. By continuously refining their algorithms, chatbots gradually improve their language comprehension skills. This results in more coherent and contextually accurate responses, making chatbot interactions more natural and meaningful.
Contextual Understanding by Chatbots:
Examining how AI chatbots grasp context to provide relevant and personalized interactions:
Contextual understanding is a crucial aspect of AI chatbots' increasing intelligence. They utilize techniques like sentiment analysis to gauge the emotions and intentions behind user queries. By understanding the context, chatbots can provide more personalized and relevant responses.
Techniques such as sentiment analysis and entity recognition:
Sentiment analysis helps chatbots understand the emotions expressed in user queries, enabling them to respond accordingly. Additionally, entity recognition allows chatbots to identify and extract important information from the user's input. This enhances the chatbot's ability to provide accurate and contextually appropriate responses.
Conversational Design for Better User Experience:
Design principles for creating intuitive and user-friendly AI chatbots
Conversational design principles are essential in creating AI chatbots that offer an intuitive and user-friendly experience. Clear and concise language, well-organized prompts, and logical conversation flows all contribute to a positive user experience. By incorporating conversational design principles, chatbots can engage users effectively and provide a seamless interaction.
Importance of conversational flow and maintaining user engagement
Conversational flow is crucial in maintaining user engagement and satisfaction. Chatbots should respond promptly and naturally, mimicking human conversation patterns. By understanding the context and adapting to user preferences, AI chatbots can create a conversational flow that feels authentic and keeps users engaged throughout the interaction.
Ethical Implications of Intelligent AI Chatbots:
Addressing concerns about privacy, data security, and algorithmic biases
As AI chatbots become more intelligent, ethical considerations become vital. Ensuring user privacy and data security is of utmost importance. Additionally, measures must be taken to mitigate algorithmic biases that may unintentionally discriminate against certain individuals or groups. Transparency and accountability in the development and deployment of AI chatbots are essential to maintain trust.
Ensuring responsible and ethical deployment of AI chatbots
To ensure responsible and ethical deployment, developers and organizations need to establish guidelines and protocols. Regular audits and evaluations should be conducted to identify and rectify any potential biases or privacy issues. By adopting ethical practices, AI chatbots can provide immense value to users while upholding important ethical considerations.
Industry Applications of AI Chatbots:
Healthcare: Revolutionizing patient care through intelligent chatbots
In the healthcare industry, AI chatbots are transforming patient care. They can provide accurate information, answer health-related inquiries, and even offer recommendations for symptoms and treatments. AI chatbots assist in reducing waiting times, providing round-the-clock support, and empowering patients to take control of their health.
E-commerce: Enhancing customer support and personalized recommendations
AI chatbots have revolutionized customer support in the e-commerce sector. They can handle a large volume of inquiries, provide instant responses, and assist customers through the entire purchase journey. Additionally, chatbots leverage machine learning algorithms to offer personalized product recommendations based on user's preferences and browsing history.
Banking and finance: Chatbots for seamless transactions and financial advice
In the banking and finance industry, chatbots are streamlining transactions and providing financial advice. They can assist customers in transferring funds, checking account balances, and even providing personalized investment recommendations. With their ability to access and analyze vast amounts of data, AI chatbots enhance the efficiency and convenience of financial services.
Education: The role of chatbots in modern learning environments
AI chatbots are revolutionizing education by providing personalized learning experiences. They can assist students in understanding complex concepts, answering questions, and even evaluating their progress. AI chatbots empower educators by providing real-time feedback, offering tailored learning materials, and catering to individual learning styles.
The Future of AI Chatbots:
Predicting the trajectory of AI chatbot advancements
The future of AI chatbots holds immense possibilities. Advancements in natural language processing, machine learning, and deep learning will further enhance the intelligence and capabilities of chatbots. With ongoing research and development, chatbots will become even more human-like and capable of understanding and responding to complex queries.
Integration of AI chatbots with other emerging technologies (e.g., voice recognition, IoT)
AI chatbots will integrate with other emerging technologies, such as voice recognition and the Internet of Things (IoT). This integration will enable chatbots to understand voice commands, seamlessly interact with smart devices, and provide personalized experiences across various platforms. The convergence of these technologies will redefine the way we interact with chatbots and make them more versatile in assisting users.
Case Studies: Successful AI Chatbot Implementations
Highlighting real-world examples of AI chatbots making a difference
Real-world examples demonstrate the transformative impact of AI chatbots. For instance, healthcare platforms have implemented AI chatbots to triage patients and provide initial medical advice. E-commerce giants have enhanced their customer support systems by deploying AI chatbots to handle customer inquiries. These successful implementations showcase the effectiveness and value of AI chatbots in various industries.
The Human-Chatbot Collaboration:
Emphasizing the complementary relationship between humans and AI chatbots
In the world of AI chatbots, it is important to understand the complementary relationship between humans and machines. While chatbots offer quick and efficient solutions to user queries, they can never fully replace the human touch. Humans bring empathy, creativity, and intuition to conversations, complementing the intelligence of AI chatbots. The collaboration between humans and chatbots results in a more enriching and productive user experience.
Balancing automation and human touch in chatbot interactions
Finding the right balance between automation and the human touch is crucial in chatbot interactions. While automation ensures efficiency and scalability, incorporating the human touch adds warmth and emotional intelligence to conversations. By striking a balance between the two, chatbot interactions can be personalized, engaging, and meaningful, creating a positive user experience.
Challenges in AI Chatbot Development:
Discussing technical and practical obstacles faced by developers
AI chatbot development comes with its fair share of challenges. Technical obstacles include accurately understanding and generating natural language, deciphering context, and handling ambiguous queries. Practical challenges involve training the chatbot with relevant and diverse datasets, ensuring scalability, and optimizing performance across different platforms.
Overcoming language barriers and cross-cultural communication challenges
Language barriers and cross-cultural communication pose challenges for AI chatbot development. Different languages, dialects, and cultural nuances make it difficult for chatbots to achieve a high level of understanding and empathy. To overcome these challenges, developers need to continuously improve language models, incorporate cultural context, and enhance chatbots' ability to adapt to diverse communication styles.
Conclusion:
AI chatbots are getting smarter because they use fancy technology like NLP and machine learning to understand our words better. They're good at different jobs, like helping in healthcare and online shopping. But they're not perfect and need to be nice and follow rules. In the future, they'll learn even more, work with voice commands, and be super helpful. Remember, they're like our helpers, not replacements for people. Sometimes, they have trouble with languages and cultures, but they're trying to get better. If you're interested in learning more about AI, you can take an Artificial Intelligence Course in Lahore where you'll cover exciting topics like coding and creating websites.
#artificial intelligence#coding#search engine optimized (seo) articles#search engine optimization#Ideocollege.com#ai chatbot
4 notes
·
View notes
Text
25 Python Projects to Supercharge Your Job Search in 2024
Introduction: In the competitive world of technology, a strong portfolio of practical projects can make all the difference in landing your dream job. As a Python enthusiast, building a diverse range of projects not only showcases your skills but also demonstrates your ability to tackle real-world challenges. In this blog post, we'll explore 25 Python projects that can help you stand out and secure that coveted position in 2024.
1. Personal Portfolio Website
Create a dynamic portfolio website that highlights your skills, projects, and resume. Showcase your creativity and design skills to make a lasting impression.
2. Blog with User Authentication
Build a fully functional blog with features like user authentication and comments. This project demonstrates your understanding of web development and security.
3. E-Commerce Site
Develop a simple online store with product listings, shopping cart functionality, and a secure checkout process. Showcase your skills in building robust web applications.
4. Predictive Modeling
Create a predictive model for a relevant field, such as stock prices, weather forecasts, or sales predictions. Showcase your data science and machine learning prowess.
5. Natural Language Processing (NLP)
Build a sentiment analysis tool or a text summarizer using NLP techniques. Highlight your skills in processing and understanding human language.
6. Image Recognition
Develop an image recognition system capable of classifying objects. Demonstrate your proficiency in computer vision and deep learning.
7. Automation Scripts
Write scripts to automate repetitive tasks, such as file organization, data cleaning, or downloading files from the internet. Showcase your ability to improve efficiency through automation.
8. Web Scraping
Create a web scraper to extract data from websites. This project highlights your skills in data extraction and manipulation.
9. Pygame-based Game
Develop a simple game using Pygame or any other Python game library. Showcase your creativity and game development skills.
10. Text-based Adventure Game
Build a text-based adventure game or a quiz application. This project demonstrates your ability to create engaging user experiences.
11. RESTful API
Create a RESTful API for a service or application using Flask or Django. Highlight your skills in API development and integration.
12. Integration with External APIs
Develop a project that interacts with external APIs, such as social media platforms or weather services. Showcase your ability to integrate diverse systems.
13. Home Automation System
Build a home automation system using IoT concepts. Demonstrate your understanding of connecting devices and creating smart environments.
14. Weather Station
Create a weather station that collects and displays data from various sensors. Showcase your skills in data acquisition and analysis.
15. Distributed Chat Application
Build a distributed chat application using a messaging protocol like MQTT. Highlight your skills in distributed systems.
16. Blockchain or Cryptocurrency Tracker
Develop a simple blockchain or a cryptocurrency tracker. Showcase your understanding of blockchain technology.
17. Open Source Contributions
Contribute to open source projects on platforms like GitHub. Demonstrate your collaboration and teamwork skills.
18. Network or Vulnerability Scanner
Build a network or vulnerability scanner to showcase your skills in cybersecurity.
19. Decentralized Application (DApp)
Create a decentralized application using a blockchain platform like Ethereum. Showcase your skills in developing applications on decentralized networks.
20. Machine Learning Model Deployment
Deploy a machine learning model as a web service using frameworks like Flask or FastAPI. Demonstrate your skills in model deployment and integration.
21. Financial Calculator
Build a financial calculator that incorporates relevant mathematical and financial concepts. Showcase your ability to create practical tools.
22. Command-Line Tools
Develop command-line tools for tasks like file manipulation, data processing, or system monitoring. Highlight your skills in creating efficient and user-friendly command-line applications.
23. IoT-Based Health Monitoring System
Create an IoT-based health monitoring system that collects and analyzes health-related data. Showcase your ability to work on projects with social impact.
24. Facial Recognition System
Build a facial recognition system using Python and computer vision libraries. Showcase your skills in biometric technology.
25. Social Media Dashboard
Develop a social media dashboard that aggregates and displays data from various platforms. Highlight your skills in data visualization and integration.
Conclusion: As you embark on your job search in 2024, remember that a well-rounded portfolio is key to showcasing your skills and standing out from the crowd. These 25 Python projects cover a diverse range of domains, allowing you to tailor your portfolio to match your interests and the specific requirements of your dream job.
If you want to know more, Click here:https://analyticsjobs.in/question/what-are-the-best-python-projects-to-land-a-great-job-in-2024/
#python projects#top python projects#best python projects#analytics jobs#python#coding#programming#machine learning
2 notes
·
View notes
Text
ChatGPT and Machine Learning: Advancements in Conversational AI

Introduction: In recent years, the field of natural language processing (NLP) has witnessed significant advancements with the development of powerful language models like ChatGPT. Powered by machine learning techniques, ChatGPT has revolutionized conversational AI by enabling human-like interactions with computers. This article explores the intersection of ChatGPT and machine learning, discussing their applications, benefits, challenges, and future prospects.
The Rise of ChatGPT: ChatGPT is an advanced language model developed by OpenAI that utilizes deep learning algorithms to generate human-like responses in conversational contexts. It is based on the underlying technology of GPT (Generative Pre-trained Transformer), a state-of-the-art model in NLP, which has been fine-tuned specifically for chat-based interactions.
How ChatGPT Works: ChatGPT employs a technique called unsupervised learning, where it learns from vast amounts of text data without explicit instructions or human annotations. It utilizes a transformer architecture, which allows it to process and generate text in a parallel and efficient manner.
The model is trained using a massive dataset and learns to predict the next word or phrase given the preceding context.
Applications of ChatGPT: Customer Support: ChatGPT can be deployed in customer service applications, providing instant and personalized assistance to users, answering frequently asked questions, and resolving common issues.
Virtual Assistants: ChatGPT can serve as intelligent virtual assistants, capable of understanding and responding to user queries, managing calendars, setting reminders, and performing various tasks.
Content Generation: ChatGPT can be used for generating content, such as blog posts, news articles, and creative writing, with minimal human intervention.
Language Translation: ChatGPT's language understanding capabilities make it useful for real-time language translation services, breaking down barriers and facilitating communication across different languages.
Benefits of ChatGPT: Enhanced User Experience: ChatGPT offers a more natural and interactive conversational experience, making interactions with machines feel more human-like.
Increased Efficiency: ChatGPT automates tasks that would otherwise require human intervention, resulting in improved efficiency and reduced response times.
Scalability: ChatGPT can handle multiple user interactions simultaneously, making it scalable for applications with high user volumes.
Challenges and Ethical Considerations: Bias and Fairness: ChatGPT's responses can sometimes reflect biases present in the training data, highlighting the importance of addressing bias and ensuring fairness in AI systems.
Misinformation and Manipulation: ChatGPT's ability to generate realistic text raises concerns about the potential spread of misinformation or malicious use. Ensuring the responsible deployment and monitoring of such models is crucial.
Future Directions: Fine-tuning and Customization: Continued research and development aim to improve the fine-tuning capabilities of ChatGPT, enabling users to customize the model for specific domains or applications.
Ethical Frameworks: Efforts are underway to establish ethical guidelines and frameworks for the responsible use of conversational AI models like ChatGPT, mitigating potential risks and ensuring accountability.
Conclusion: In conclusion, the emergence of ChatGPT and its integration into the field of machine learning has opened up new possibilities for human-computer interaction and natural language understanding. With its ability to generate coherent and contextually relevant responses, ChatGPT showcases the advancements made in language modeling and conversational AI.
We have explored the various aspects and applications of ChatGPT, including its training process, fine-tuning techniques, and its contextual understanding capabilities. Moreover, the concept of transfer learning has played a crucial role in leveraging the model's knowledge and adapting it to specific tasks and domains.
While ChatGPT has shown remarkable progress, it is important to acknowledge its limitations and potential biases. The continuous efforts by OpenAI to gather user feedback and refine the model reflect their commitment to improving its performance and addressing these concerns. User collaboration is key to shaping the future development of ChatGPT and ensuring it aligns with societal values and expectations.
The integration of ChatGPT into various applications and platforms demonstrates its potential to enhance collaboration, streamline information gathering, and assist users in a conversational manner. Developers can harness the power of ChatGPT by leveraging its capabilities through APIs, enabling seamless integration and expanding the reach of conversational AI.
Looking ahead, the field of machine learning and conversational AI holds immense promise. As ChatGPT and similar models continue to evolve, the focus should remain on user privacy, data security, and responsible AI practices. Collaboration between humans and machines will be crucial, as we strive to develop AI systems that augment human intelligence and provide valuable assistance while maintaining ethical standards.
With further advancements in training techniques, model architectures, and datasets, we can expect even more sophisticated and context-aware language models in the future. As the dialogue between humans and machines becomes more seamless and natural, the potential for innovation and improvement in various domains is vast.
In summary, ChatGPT represents a significant milestone in the field of machine learning, bringing us closer to human-like conversation and intelligent interactions. By harnessing its capabilities responsibly and striving for continuous improvement, we can leverage the power of ChatGPT to enhance user experiences, foster collaboration, and push the boundaries of what is possible in the realm of artificial intelligence.
2 notes
·
View notes
Text
The Power of Artificial Intelligence (AI) and Machine Learning (ML): Advancements and Applications
In recent years, Artificial Intelligence (AI) and Machine Learning (ML) have emerged as game-changers, revolutionizing numerous industries and reshaping the way we live, work, and interact with technology. The advancements in AI and ML have unlocked new possibilities, enabling machines to learn, adapt, and make intelligent decisions. In this article, we will explore the power of AI and ML, their recent advancements, and their diverse applications across various sectors.
Advancements in AI and ML:
AI and ML have witnessed significant advancements, thanks to breakthroughs in computing power, data availability, and algorithmic innovations. Deep learning, a subfield of ML, has gained prominence, allowing machines to analyze vast amounts of data and extract meaningful patterns and insights. Additionally, advancements in natural language processing (NLP), computer vision, and reinforcement learning have further expanded the capabilities of AI and ML.
Applications in Healthcare:
AI and ML have immense potential in transforming the healthcare industry. From early disease detection to personalized treatment plans, AI-based systems can analyze medical records, imaging data, and genomic information to aid in diagnosis and treatment decisions. ML algorithms can predict patient outcomes, optimize hospital operations, and assist in drug discovery, making healthcare more efficient and effective.
Automation and Robotics:
The integration of AI and ML has revolutionized automation and robotics. Intelligent machines equipped with ML algorithms can learn from human interaction, adapt to changing environments, and perform complex tasks with precision and efficiency. From industrial automation to autonomous vehicles, AI and ML enable machines to perceive, reason, and act in real-time, driving advancements in manufacturing, logistics, and transportation.
Natural Language Processing and Chatbots:
Advancements in NLP have led to the development of sophisticated chatbots and virtual assistants that can understand and respond to human language. These AI-powered conversational agents are increasingly used in customer service, providing instant responses and personalized experiences. They can handle queries, assist in product recommendations, and streamline customer interactions, improving user satisfaction and efficiency.
Financial Services:
AI and ML have disrupted the financial services industry, enabling accurate fraud detection, risk assessment, and algorithmic trading. ML models can analyze vast amounts of financial data, identify patterns, and make predictions, aiding in investment decisions and portfolio management. AI-powered virtual financial advisors provide personalized financial guidance, making financial services more accessible and tailored to individual needs.
Personalization and Recommendation Systems:
AI and ML algorithms drive powerful recommendation systems that have become an integral part of our digital experiences. From e-commerce platforms to streaming services, these systems analyze user preferences, purchase history, and behavior patterns to provide personalized product recommendations and content suggestions, enhancing user engagement and driving sales.
Cybersecurity:
AI and ML have proven invaluable in combating cyber threats. ML algorithms can detect anomalies and patterns in network traffic, identifying potential security breaches and enabling proactive measures. AI-based systems can learn from past attacks and adapt their defense mechanisms, providing real-time threat detection and response to protect sensitive data and infrastructure.
Conclusion:
In conclusion, the advancements in Artificial Intelligence (AI) and Machine Learning (ML) have unleashed transformative capabilities across various industries. From healthcare and automation to personalization and cybersecurity, the applications of AI and ML are wide-ranging and profound. As these technologies continue to evolve, it is crucial to collaborate with the top mobile app development companies in New York that have expertise in harnessing the power of AI and ML.
In the bustling city of New York, where technological advancements thrive, businesses can find a pool of top-notch mobile app development companies capable of integrating AI and ML into their solutions. These companies possess the knowledge and experience to leverage AI and ML algorithms effectively, enabling businesses to build intelligent and innovative mobile applications.
By partnering with these Top 10 mobile app development companies in New York, businesses can leverage AI and ML to enhance customer experiences, optimize processes, and gain a competitive edge in the market. These companies excel in developing AI-powered chatbots, recommendation systems, and personalized experiences that drive engagement and boost user satisfaction.
2 notes
·
View notes
Text
Introduction to AI Platforms
AI Platforms are powerful tools that allow businesses to automate complex tasks, provide real-time insights, and improve customer experiences. With their ability to process massive amounts of data, AI platforms can help organizations make more informed decisions, enhance productivity, and reduce costs.
These platforms incorporate advanced algorithms such as machine learning, natural language processing (NLP), and computer vision to analyze data through neural networks and predictive models. They offer a broad range of capabilities such as chatbots, image recognition, sentiment analysis, and recommendation engines.
Choosing the right AI platform is imperative for businesses that wish to stay ahead of the competition. Each platform has its strengths and weaknesses which must be assessed when deciding on a vendor. Moreover, an AI platform’s ability to integrate with existing systems is critical in effectively streamlining operations.
The history of AI platforms dates back to the 1950s, with the development of early artificial intelligence research. However, over time these technologies have evolved considerably – thanks to advancements in computing power and big data analytics. While still in their infancy stages just a few years ago – today’s AI platforms have matured into complex and feature-rich solutions designed specifically for business use cases.
Ready to have your mind blown and your workload lightened? Check out the best AI platforms for businesses and say goodbye to manual tasks:
Popular Commercial AI Platforms
To explore popular the top AI platforms and make informed decisions, you need to know the benefits each platform offers. With IBM Watson, Google Cloud AI Platform, Microsoft Azure AI Platform, and Amazon SageMaker in focus, this section shows the unique advantages each platform provides for various industries and cognitive services.
IBM Watson
The Innovative AI Platform by IBM:
Transform your business with the dynamic cognitive computing technology of IBM Watson. Enhance decision-making, automate operations, and accelerate the growth of your organization with this powerful tool.
Additional unique details about the platform:
IBM Watson’s Artificial intelligence streamlines workflows and personalizes experiences while enhancing predictive capabilities. The open-source ecosystem allows developers and businesses alike to integrate their innovative applications seamlessly.
Suggested implementation strategies:
1) Leverage Watson’s data visualization tools to clearly understand complex data sets and analyze them. 2) Utilize Watson’s Natural Language processing capabilities for sentiment analysis, identifying keywords, or contextual understanding.
By incorporating IBM Watson’s versatile machine learning functions into your operations, you can gain valuable insights into customer behavior patterns, track industry trends, improve decision-making abilities, and eventually boost revenue. Google’s AI platform is so powerful, it knows what you’re searching for before you do.
Google Cloud AI Platform
The AI platform provided by Google Cloud is an exceptional tool for businesses that major in delivering machine learning services. It provides a broad array of functionalities tailored to meet the diverse demands of clients all over the world.
The following table summarizes the features and capabilities offered by the Google Cloud AI Platform:FeaturesCapabilitiesData Management & Pre-processing
– Large-scale data processing
– Data Integration and Analysis tools
– Deep Learning Frameworks
– Data versioning tools
Model Training
– Scalable training
– AutoML tools
– Advanced tuning configurations
– Distributed Training on CPU/GPU/TPU
Prediction
– High-performance responses within seconds
– Accurate predictions resulting from models trained using large-scale datasets.
Monitoring
– Real-time model supervision and adjustment
– Comprehensive monitoring, management, and optimization of models across various stages including deployment.
One unique aspect of the Google Cloud AI platform is its prominent role in enabling any developer, regardless of their prior experience with machine learning, to build sophisticated models. This ease of use accelerates experimentation and fosters innovation.
Finally, it is worth noting that according to a study conducted by International Business Machines Corporation (IBM), brands that adopted AI for customer support purposes experienced 40% cost savings while improving customer satisfaction rates by 90%.
Continue Reading
2 notes
·
View notes
Text
AI/ML Development Services for Business Growth
Innovation is a need, not a luxury, in the digital age. Companies that don't adjust to new technologies run the risk of falling behind. These days, machine learning (ML) and artificial intelligence (AI) are at the center of industry-wide transformational strategies. One of the most calculated moves you can make to stay ahead of the curve is to collaborate with a top AI and ML development company.
These businesses provide professional AI and ML development services that enable businesses to automate procedures, customize client interactions, and use data to make more informed decisions.
Why AI and ML Are Game-Changers for Business
The imitation of human intelligence in machines, known as artificial intelligence (AI), allows them to perform activities like decision-making, problem-solving, and language understanding. Machine learning (ML), a subfield of artificial intelligence, enables computers to learn from data, identify patterns, and progressively improve performance without the need for human programming.
By automating repetitive operations and providing predictive insights, AI and ML are boosting productivity, reducing costs, and creating new business models.
Benefits of Partnering with an AI/ML Development Company
Here’s how a specialized AI/ML company can transform your business:
1. Tailored Intelligent Solutions
Every company has different objectives and challenges. A seasoned AI/ML development firm starts by comprehending your business plan and spotting areas where AI or ML can add value. Whether it's a recommendation engine, chatbot for customer service, or predictive analytics for more accurate forecasting, the end result is a personalized solution.
2. End-to-End Services
AI/ML firms provide full-cycle services, from strategy and data preparation to model development, deployment, and support. This guarantees uniformity, expediency, and decreased hazards throughout the project's various stages. You can concentrate on your main business goals when professionals are in charge of the entire pipeline.
3. Accelerated Innovation and Automation
Automating time-consuming and repetitive tasks is best done with AI and ML. Intelligent automation can greatly increase productivity in a variety of tasks, including scanning documents, processing invoices, and identifying fraud. By revealing previously undiscovered insights in intricate data sets, these technologies also foster innovation.
4. Improved Decision-Making with Data
Large volumes of data can be transformed into useful insights using ML models and analytics driven by AI. This enables companies to make data-driven decisions more quickly, whether they are predicting customer attrition, optimizing marketing campaigns, or increasing operational efficiency.
5. Competitive Advantage
Early AI/ML adopters frequently outperform their rivals. Businesses can provide their clients with better value if they can predict market trends, customize user experiences, and allocate resources as efficiently as possible.
Key Services Offered by Leading AI/ML Companies
AI Strategy Consulting – Identifying AI use cases and building a roadmap
Machine Learning Model Development – Supervised, unsupervised, and reinforcement learning
Natural Language Processing (NLP) – Chatbots, language translation, sentiment analysis
Computer Vision – Image recognition, facial detection, video analytics
Predictive Analytics – Demand forecasting, risk modeling, behavior prediction
AI Integration & Deployment – Seamless integration into business systems
Model Monitoring & Optimization – Ensuring long-term accuracy and performance
Industries Benefiting from AI/ML
AI/ML technologies are reshaping industries such as:
Healthcare: Early disease detection, patient data analysis, personalized medicine
Finance: Credit scoring, fraud prevention, algorithmic trading
Retail: Inventory management, product recommendations, customer segmentation
Manufacturing: Predictive maintenance, quality assurance, robotics
Logistics: Route planning, supply chain automation
Marketing: Customer analytics, dynamic pricing, campaign optimization
Choosing the Right Partner
When selecting an AI/ML development partner, look for:
A proven portfolio of successful AI/ML projects
Expertise in relevant frameworks and tools (TensorFlow, PyTorch, etc.)
Strong data science and engineering teams
Transparent communication and agile development methods
Post-deployment support and continuous optimization
Conclusion
These days, AI and ML technologies are necessary for competitive advantage, efficiency, and growth. Working with a top AI and ML development company gives you access to skilled personnel, tried-and-true processes, and cutting-edge tools to transform your data into a valuable asset.
Lead instead of merely adapting. With a reliable AI/ML development partner, embrace data-driven innovation and intelligent automation to future-proof your company. Source: https://silverspaceinc.com/artificial-intelligence-and-ml/
0 notes
Text
Machine Learning Syllabus: What Mumbai-Based Courses Are Offering This Year
As Artificial Intelligence continues to dominate the future of technology, Machine Learning (ML) has become one of the most sought-after skills in 2025. Whether you’re a data enthusiast, a software developer, or someone looking to transition into tech, understanding the structure of a Machine Learning Course in Mumbai can help you make informed decisions and fast-track your career.
Mumbai, a city synonymous with opportunity and innovation, has emerged as a growing hub for AI and ML education. With a rising demand for skilled professionals, leading training institutes in the city are offering comprehensive and job-focused Machine Learning courses in Mumbai. But what exactly do these programs cover?
In this article, we break down the typical Machine Learning syllabus offered by Mumbai-based institutes, highlight key modules, tools, and career pathways, and help you understand why enrolling in a structured ML course is one of the best investments you can make this year.
Why Machine Learning Matters in 2025?
Before diving into the syllabus, it’s essential to understand why machine learning is central to the tech industry in 2025.
Machine learning is the driving force behind:
Predictive analytics
Recommendation engines
Autonomous systems
Fraud detection
Chatbots and virtual assistants
Natural Language Processing (NLP)
From healthcare to fintech and marketing to logistics, industries are deploying ML to enhance operations, automate decisions, and offer personalized services. As a result, the demand for ML engineers, data scientists, and AI developers has skyrocketed.
Overview of a Machine Learning Course in Mumbai
A Machine Learning course in Mumbai typically aims to:
Build foundational skills in math and programming
Teach practical ML model development
Introduce deep learning and advanced AI techniques
Prepare students for industry-level projects and interviews
Let’s now explore the typical modules and learning paths that top-tier ML programs in Mumbai offer in 2025.
1. Foundation in Programming and Mathematics
🔹 Programming with Python
Most courses start with Python, the industry-standard language for data science and ML. This module typically includes:
Variables, loops, functions
Data structures (lists, tuples, dictionaries)
File handling and error handling
Introduction to libraries like NumPy, Pandas, Matplotlib
🔹 Mathematics for ML
You can’t master machine learning without understanding the math behind it. Essential topics include:
Linear Algebra (vectors, matrices, eigenvalues)
Probability and Statistics
Calculus basics (gradients, derivatives)
Bayes’ Theorem
Descriptive and inferential statistics
These foundations help students grasp how ML models work under the hood.
2. Data Handling and Visualization
Working with data is at the heart of ML. Courses in Mumbai place strong emphasis on:
Data cleaning and preprocessing
Handling missing values
Data normalization and transformation
Exploratory Data Analysis (EDA)
Visualization with Matplotlib, Seaborn, Plotly
Students are often introduced to real-world datasets (CSV, Excel, JSON formats) and taught to manipulate data effectively.
3. Supervised Machine Learning
This core module teaches the backbone of most ML applications. Key algorithms covered include:
Linear Regression
Logistic Regression
Decision Trees
Random Forest
Naive Bayes
Support Vector Machines (SVM)
Students also learn model evaluation techniques like:
Confusion matrix
ROC-AUC curve
Precision, recall, F1 score
Cross-validation
Hands-on labs using Scikit-Learn, along with case studies from domains like healthcare and retail, reinforce these concepts.
4. Unsupervised Learning
This segment of the syllabus introduces students to patterns and grouping in data without labels. Key topics include:
K-Means Clustering
Hierarchical Clustering
Principal Component Analysis (PCA)
Anomaly Detection
Students often work on projects like customer segmentation, fraud detection, or market basket analysis using unsupervised techniques.
5. Model Deployment and MLOps Basics
As real-world projects go beyond model building, many Machine Learning courses in Mumbai now include modules on:
Model deployment using Flask or FastAPI
Containerization with Docker
Version control with Git and GitHub
Introduction to cloud platforms like AWS, GCP, or Azure
CI/CD pipelines and monitoring in production
This gives learners an edge in understanding how ML systems operate in real-time environments.
6. Introduction to Deep Learning
While ML and Deep Learning are distinct, most advanced programs offer a foundational understanding of deep learning. Topics typically covered:
Neural Networks: Structure and working
Activation Functions: ReLU, sigmoid, tanh
Backpropagation and Gradient Descent
Convolutional Neural Networks (CNNs) for image processing
Recurrent Neural Networks (RNNs) for sequential data
Frameworks: TensorFlow and Keras
Students often build beginner deep learning models, such as digit recognizers or sentiment analysis tools.
7. Natural Language Processing (NLP)
With AI’s growing use in text-based applications, NLP is an essential module:
Text preprocessing: Tokenization, stopwords, stemming, lemmatization
Term Frequency–Inverse Document Frequency (TF-IDF)
Sentiment analysis
Named Entity Recognition (NER)
Introduction to transformers and models like BERT
Hands-on projects might include building a chatbot, fake news detector, or text classifier.
8. Capstone Projects and Portfolio Development
Most Machine Learning courses in Mumbai culminate in capstone projects. These simulate real-world problems and require applying all learned concepts:
Data ingestion and preprocessing
Model selection and evaluation
Business interpretation
Deployment and presentation
Example capstone projects:
Predictive maintenance in manufacturing
Price prediction for real estate
Customer churn prediction
Credit risk scoring model
These projects are crucial for portfolio building and serve as talking points in interviews.
9. Soft Skills and Career Preparation
The best training institutes in Mumbai don’t stop at technical skills—they invest in career readiness. These include:
Resume building and portfolio review
Mock technical interviews
Behavioral interview training
LinkedIn optimization
Job referrals and placement assistance
Students also receive guidance on freelancing, internships, and participation in Kaggle competitions.
A Standout Option: Boston Institute of Analytics
Among the many training providers in Mumbai, one institute that consistently delivers quality machine learning education is the Boston Institute of Analytics.
Their Machine Learning Course in Mumbai is built to offer:
A globally recognized curriculum tailored for industry demands
In-person classroom learning with expert faculty
Real-world datasets and capstone projects
Deep exposure to tools like Python, TensorFlow, Scikit-learn, Keras, and AWS
One-on-one career mentorship and resume support
Dedicated placement assistance with a strong alumni network
For students and professionals serious about entering the AI/ML field, BIA provides a structured and supportive environment to thrive.
Final Thoughts: The Future Is Machine-Learned
In 2025, machine learning is not just a skill—it's a career catalyst. The best part? You don’t need to be a Ph.D. holder to get started. All you need is the right course, the right mentors, and the commitment to build your skills.
By understanding the detailed Machine Learning syllabus offered by Mumbai-based courses, you now have a roadmap to guide your learning journey. From Python basics to deep learning applications, and from real-time deployment to industry projects—everything is within your reach.
If you’re looking to transition into the world of AI or upgrade your existing data science knowledge, enrolling in a Machine Learning course in Mumbai might just be the smartest move you’ll make this year.
#Machine Learning Course in Mumbai#6 Months Data Science Course in Mumbai#Artificial Intelligence Classroom Course in Mumbai
0 notes