#extracting relevant data from OTT media platforms
Explore tagged Tumblr posts
Text
OTT Media Platform Data Scraping | Extract Streaming App Data
Unlock insights with our OTT Media Platform Data Scraping. Extract streaming app data in the USA, UK, UAE, China, India, or Spain. Optimize your strategy today
know more: https://www.mobileappscraping.com/ott-media-app-scraping-services.php
#OTT Media Platform Data Scraping#Extract Streaming App Data#extracting relevant data from OTT media platforms#extracting data from websites
0 notes
Text
Revolutionizing Data Labeling with Video Transcription Services: A GTS.AI Perspective
In the fast-evolving world of AI and ML, accurate and quality data is the linchpin of every discovery in the industry. Video transcription services have emerged as a vital cog in the data-labeling framework, unlocking newer vistas for training cutting-edge AI models. At GTS.AI, we specialize in providing fast, seamless video transcription solutions according to the varying needs of our clients.
Why video transcription services!
Sharpening AI Myopathy More video data is being utilized globally in industries such as entertainment, education, e-commerce, and health. With the wave of overflowing video into daily lives, AI models require precise transcription in order to extract valuable insights, train algorithms, and enhance user experience. Here is where GTS.AI comes in to bridge the gap between raw video data and actionable machine learning insights.
Increased accuracy of machine learning: Top-quality transcriptions assist AI in reading patterns, nuances, and language-specific complications present in video content. Customization according to industry: Be it medical diagnosis, forensic analysis, or e-learning platforms on video, transcriptions are hence crucial in fine-tuning the AI for a specific application. Accessibility across the globe: Transcription enables multilingual support and closed captions, which allow businesses to launch their products all around the globe. The Role of GTS.AI in Video Transcription
At GTS.AI, we are on the mission to update the entire process of data labeling. Through the hybridization of technology and human capability, we provide transcribing services that go beyond mere accuracy-into productivity, conformity towards scalability, and relevancy to industry.
A Human-in-the-Loop System: While automated tools are used throughout our workflow, we endorse human oversight for full comprehension of the context and flawless accuracy in the transcription thereof. Tailored Workflows: We work together with our clients to create custom transcription pipelines that fit the specific requirements of their projects. Scalability: From a start-up to the enterprise, GTS.AI could scale up the solution to meet its ever-growing data requirements. Assured Security: Preservation of data privacy and data integrity is a cardinal priority. We at GTS.AI adopt state-of-the-art security protocol for safeguarding your video data.
Real-World Applications of Our Services
E-Learning Platforms: Serving online educators by providing transcriptions for pedagogical videos that enhance knowledge dissemination. Market Research: Assisting market analysts by converting video interviews, focus group discussions, and customer feedback into transcripts for in-depth analysis. Healthcare: Giving healthcare professionals access to precise transcriptions of medical procedures and appointments to help them conduct research efforts and training. Media and Entertainment: Facilitating the production house and OTT platforms' generation of subtitles and video classification respectively. Engage GTS.AI for a Partnership in Video Transcription
GTS.AI is not just a data labeler; we are, indeed, your partners in shaping the future of AI. We give video transcription the necessary touch to enhance your projects with precision, speed, and efficiency. In a landscape filled with innovation, we redefine the very benchmarks of data labeling excellence with a tremendous zeal for customer satisfaction. To explore the full potential of your video data with complete confidence, feel free to contact GTS.AI and uplift your quality, trust, and expertise.
1 note
·
View note
Text
How Does IMDB Movies Data Scraping Enhance Media Streaming Platforms
How Does IMDB Movies Data Scraping Enhance Media Streaming Platforms?
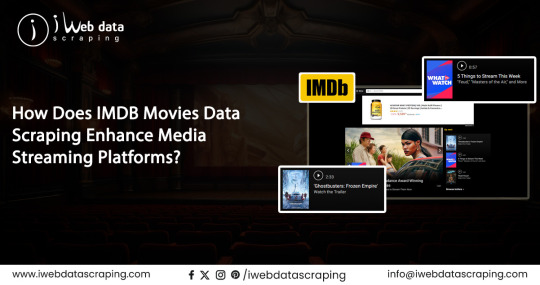
IMDB.com is a veritable treasure trove of information in cinema, housing comprehensive details about movies, including ratings, cast, reviews, and more. Collecting this valuable data through web scraping opens doors to a myriad of possibilities for enthusiasts and analysts. This process involves navigating the web pages, identifying HTML structures, and employing scraping tools in Python like BeautifulSoup or Scrapy. As we embark on the journey of IMDB movie data scraping, we gain access to a treasure trove of insights that help create personalized databases, conduct in-depth analyses, or satisfy our curiosity about the diverse world of film. This guide explores the intricacies of scraping movie data from IMDB.com, unlocking a richer understanding of the cinematic landscape through the lens of data extraction.
List of Data Fields
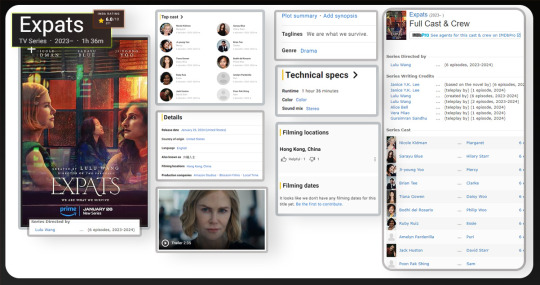
Movie Title
Movie Images Links
Description
Trailer Video Links
Release Year
Genre
Director
Writer
Cast
IMDB Rating
Metascore
Plot Summary
Runtime
Country
Language
Step-by-Step Guide: Extracting Movies Data from IMDB.com
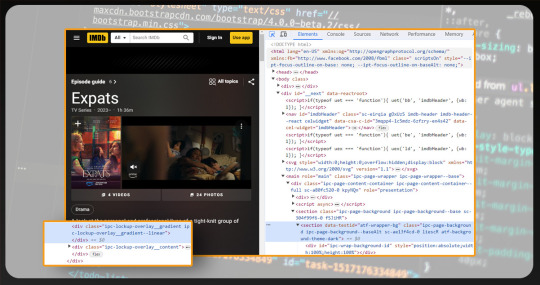
1. Choose a Scraping Tool: Select an IMDB data scraper tailored to your needs. Notable choices include Python's BeautifulSoup or Scrapy. These libraries simplify the intricacies of traversing web pages and extracting desired information efficiently.
2. Identify the Target URL: Navigate to IMDB.com and pinpoint the specific URL representing your area of interest. Identifying the target URL is crucial for focused OTT platform data scraping, whether it's the "Top Rated Movies" section or a particular genre.
3. Inspect the HTML Structure: Utilize your browser's developer tools to scrutinize the HTML structure of the IMDB page. Identify key HTML tags and classes housing pertinent information like movie titles, ratings, and cast details.
4. Set Up Your Script: Develop a Python script using your scraping library. Leverage your script's previously identified HTML tags and classes to locate and extract the relevant data. Incorporate robust error-handling mechanisms to account for potential changes in IMDB.com's website structure.
5. Send HTTP Requests: Utilize your chosen scraping library to send HTTP requests to IMDB's server, fetching the HTML content of the target page. It marks the initiation of data retrieval.
6. Parse HTML Content: Implement parsing mechanisms in your script to extract the desired data from the retrieved HTML content. Transform these HTML elements into structured data, such as a dictionary or a CSV file, facilitating subsequent analysis.
7. Handle Pagination: If your data spans multiple pages, introduce logic to navigate through different search result pages. Handling pagination ensures a comprehensive dataset, capturing a broader spectrum of movies.
8. Store the Data: IMBD data scraping services help decide on the appropriate storage method for your scraped data. This step is crucial for organized data management and seamless retrieval, whether in a CSV file, database, or preferred format.
9. Respect Robots.txt: Adhere to ethical scraping practices by ensuring your activities align with IMDB.com's terms of service and respecting their robots.txt file. Avoid overly aggressive scraping and maintain a harmonious relationship with the website.
10. Regularly Update Your Script: Acknowledge the dynamic nature of websites. Regularly revisit and update your scraping script to accommodate any structural changes on IMDB.com. This proactive approach guarantees your data extraction process's sustained accuracy and effectiveness over time.
Significance of scraping IMDB.com movie data
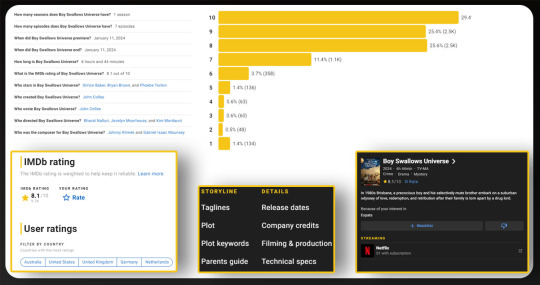
Comprehensive Movie Insights: Scraping data from IMDB.com provides access to a comprehensive repository of information about movies. It includes ratings, cast, crew, release dates, genres, and more, offering a holistic view of the cinematic landscape.
Market Analysis and Trends: The scraped data allows in-depth market analysis and identification of trends. Businesses and analysts can discern patterns, preferences, and the popularity of specific genres or actors by analyzing ratings, reviews, and box office earnings.
Personalized Recommendations: The collected data enables the creation of personalized recommendation systems. By understanding user ratings, preferences, and viewing habits, platforms can tailor movie suggestions, enhancing the user experience.
Content Curation: Media platforms and streaming services can leverage scraped data to curate content. Insights into popular genres, acclaimed directors, or trending actors aid in optimizing content libraries and meeting audience demands.
Competitor Analysis: For film production companies and studios, scraping IMDB.com provides valuable insights into competitors' performance. Analyzing reviews, ratings, and audience feedback helps strategize and position their productions effectively in the market.
Academic Research: Researchers in cinema studies or data science can utilize IMDB data for academic purposes. The detailed movie information and user-generated reviews serve as a rich dataset for various research endeavors.
Enhanced User Engagement: Websites and applications dedicated to movie enthusiasts can leverage IMDB data to enhance user engagement. By incorporating up-to-date information on ratings, reviews, and cast details, these platforms can provide a dynamic and informative user experience, keeping audiences engaged and informed.
Types of Businesses Benefitting from IMDB Data Scraping
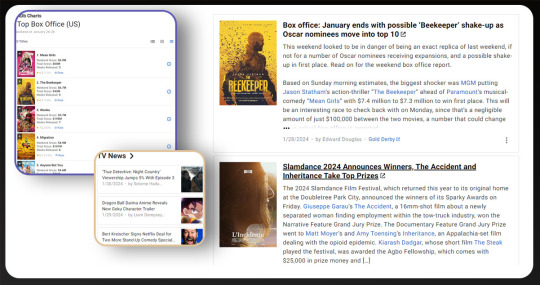
Media Streaming Platforms: Media streaming services can benefit by scraping IMDB data to curate and optimize their content libraries, providing users with personalized recommendations based on movie ratings, genres, and famous actors.
Film Production Companies: Film production companies can utilize IMDB data for competitor analysis, gaining insights into the performance of rival productions, understanding market trends, and making informed decisions about their projects.
Movie Review Websites: Platforms dedicated to movie reviews can enhance their databases by scraping IMDB for the latest ratings, reviews, and user feedback. It ensures their content is up-to-date and comprehensive.
Entertainment News Outlets: Entertainment news outlets can use IMDB data to report trends, box office performances, and the latest developments in the film industry. Access to accurate and timely information increases the credibility of their content.
Academic Researchers: Researchers in cinema studies, data science, and market analysis can leverage IMDB data for academic research. The detailed information on movies and user-generated reviews is a valuable dataset for various research endeavors.
Advertising and Marketing Agencies: Advertising and marketing agencies can use IMDB data to tailor their campaigns. Understanding audience preferences, famous actors, and successful genres allows for more targeted and effective promotional strategies.
E-commerce Platforms: E-commerce platforms specializing in DVDs, Blu-rays, or movie-related merchandise can benefit from IMDB data by ensuring product offerings align with popular and trending movies.
Event Organizers: Event organizers, such as those organizing film festivals or awards ceremonies, can use IMDB data to stay informed about the latest critically acclaimed movies, ensuring their events are as per the current industry trends.
Analytics and Data Companies: Companies specializing in data analytics can offer valuable insights to various clients by utilizing IMDB data. It can include market trends, audience preferences, and the success factors behind popular movies.
Mobile Apps for Movie Enthusiasts: Mobile applications dedicated to movie enthusiasts can integrate IMDB data to provide real-time information on movie ratings, cast details, and reviews, enhancing the overall user experience.
Conclusion: Scrape movie data from IMDB.com to collect ratings, cast details, reviews, and more, offering unparalleled insights into the dynamic world of cinema. Businesses, ranging from media streaming platforms to film production companies, can leverage this data for market analysis, content curation, and strategic decision-making. Academic researchers find a valuable dataset for cinema studies, while entertainment platforms enhance user engagement. As technology advances, the significance of scraping IMDB.com persists, shaping industries and providing a comprehensive lens into the ever-evolving landscape of movies.
Please contact iWeb Data Scraping for a comprehensive range of data services! Our committed team is ready to assist you, whether you need mobile or web data scraping services. Contact us today to discuss your specific needs for scraping retail store location data. Let us showcase how our customized data scraping solutions can deliver efficiency and reliability tailored precisely to meet your unique requirements.
Know More: https://www.iwebdatascraping.com/imdb-movies-data-scraping-enhance-media-streaming-platforms.php
#IMDBMoviesDataScraping#ScrapingEnhanceMediaStreamingPlatforms#ScrapeIMDBMoviesData#IMDBMoviesDataScraper#ExtractIMDBMoviesData#IMDBMoviesDataExtractor
0 notes
Text
How to Extract Insights from OTT Media Platforms: A Guide to Data Scraping?

How to Extract Insights from OTT Media Platforms: A Guide to Data Scraping?
Aug 01, 2023
OTT (Over-The-Top) media platform data scraping refers to extracting relevant data from OTT media platforms. OTT platforms are streaming services that deliver media content directly to users online, bypassing old-style broadcast channels. Some examples of leading OTT media platforms include Netflix, Amazon Prime Video, Hulu, and Disney+.
Data scraping from OTT media platforms involves accessing and extracting various data types, such as user engagement metrics, viewer demographics, content catalogs, ratings and reviews, streaming performance, and more. This data can provide valuable insights for content providers, advertisers, and market researchers to understand audience preferences, track content performance, optimize marketing strategies, and make informed business decisions.
OTT media platform data scraping enables businesses to gather real-time and historical data from multiple platforms. It allows them to analyze trends, identify popular content, target specific audience segments, and enhance content strategies. By leveraging the scraped data, businesses can gain an edge in the highly competitive and rapidly evolving OTT media industry.
How Does OTT Media Platform Data Scraping Work?

OTT Media Platform Data Scraping involves systematically extracting relevant data from OTT media platforms. Here's a simplified overview of how it works:
Identification of Target Platforms: Determine the specific OTT media platforms you want to scrape data from. This could include platforms like Netflix, Hulu, Amazon Prime Video, or any other platform that hosts the content you're interested in.
Data Collection Strategy: Define the scope and parameters of the data you want to scrape. This may include user engagement metrics, content catalogs, ratings and reviews, viewer demographics, streaming performance, and other relevant data points.
Data Scraping Techniques: Employ data scraping techniques to extract the desired data from the OTT platforms. This involves automated software or scripts that navigate the platform's pages, simulate user interactions, and extract data elements based on predefined rules and patterns.
Data Extraction: Use scraping tools to extract the identified data points from the pages. This may involve capturing HTML elements, parsing JSON or XML data, or employing browser automation techniques to interact with the platform's interfaces and retrieve the desired information.
Data Processing and Analysis: Once the data is extracted, it may go through a preprocessing stage to clean and normalize the data for further analysis. This can include removing duplicates, handling missing values, and transforming the data into a structured format suitable for analysis.
Data Storage and Management: Store the scraped data securely and organized, ensuring proper data management practices. This may involve structuring the data into a database, data warehouse, or other storage systems for easy access and retrieval.
Analysis and Insights: Analyze the scraped data to gain actionable insights. This can include performing statistical analysis, visualizing trends, identifying patterns, and deriving meaningful conclusions to inform content strategies, marketing campaigns, or audience targeting.
It's important to note that OTT Media Platform Data Scraping should be conducted ethically and in compliance with the terms of service and legal regulations governing the platforms. Respect user privacy and adhere to data protection guidelines while scraping and handling the extracted data.
What Types Of Data Can Be Scraped From OTT Media Platforms?

Several data types can be scraped from OTT (Over-The-Top) media platforms. The specific data available for scraping may vary depending on the platform and its terms of service. Here are some common types of data that can be scraped from OTT media platforms:
Content Catalog: Information about the available movies, TV shows, documentaries, and other forms of media content, including titles, descriptions, genres, release dates, and duration.
User Engagement Metrics: Data related to user interactions with the platform, such as the number of views, likes, ratings, reviews, comments, and shares for specific content.
Viewer Demographics: Data on the demographic characteristics of platform users, including age, gender, location, and language preferences. This information can help understand the target audience and tailor content strategies accordingly.
Streaming Performance: Metrics related to streaming quality, buffering time, playback errors, and other performance indicators that assess the user experience on the platform.
Recommendations and Personalization: The platform provides data about user preferences, watch history, and personalized recommendations based on user behavior and content consumption patterns.
Ratings and Reviews: User-generated ratings, reviews, and comments on individual movies, TV shows, or episodes. This data can provide insights into audience sentiment and feedback on specific content.
Licensing and Availability: Information regarding content licensing agreements, availability by region, and expiration dates for specific titles. This data is particularly relevant for content acquisition and distribution strategies.
Metadata and Tags: Additional metadata associated with media content, including cast and crew information, production details, keywords, tags, and categorization.
Platform-Specific Data: Each OTT media platform may have unique data points that can be scraped, such as user playlists, recently watched content, or content-specific metrics provided by the platform's API.
By scraping these types of data from OTT media platforms, businesses can gain valuable insights into audience preferences, content performance, and market trends. This information can inform content strategies, marketing campaigns, audience targeting, and other decision-making processes in the dynamic OTT industry.
What Are The Benefits Of Mobile App Scraping's OTT Media Platform Data Scraping Services For Businesses?
Mobile App Scraping's OTT Media Platform Data Scraping Services offer several benefits for businesses in the media industry. Here are some key advantages:
Market Analysis and Audience Insights: Businesses gain valuable market analysis and audience insights by scraping data from OTT media platforms. This includes understanding viewer preferences, consumption patterns, demographic information, and engagement metrics. These insights help make informed decisions about content creation, licensing, marketing, and audience targeting.
Competitive Intelligence: Data scraping allows businesses to gather competitive intelligence by analyzing content catalogs, pricing strategies, user ratings, and reviews of competitors on OTT platforms. This information helps identify market trends, positioning strategies, and opportunities for differentiation.
Content Optimization: Scraped data provides insights into performance, user feedback, and preferences. Businesses can analyze this data to optimize their content offerings, improve user engagement, and tailor their content strategy to meet the evolving demands of their audience.
Personalization and Recommendation Systems: OTT platforms rely on personalized recommendations to enhance user experiences. Businesses can understand user behavior, preferences, and viewing habits by scraping data. This enables them to build more effective recommendation systems, providing personalized content suggestions and improving user satisfaction.
Advertising and Monetization: Data scraping helps businesses identify popular content genres, target relevant audience segments, and optimize advertising campaigns. Businesses can make data-driven decisions to maximize ad revenue and optimize monetization strategies by analyzing user engagement metrics and demographics.
Market Trends and Forecasting: Scraped data from OTT media platforms provide insights into emerging market trends, viewer preferences, and content consumption patterns. This data can be used for market forecasting, predicting future content demand, and making strategic content acquisition and production decisions.
Operational Efficiency: Data scraping automates the process of data collection, allowing businesses to gather large amounts of data from multiple platforms efficiently. This saves time and resources that would otherwise be spent on manual data gathering and analysis.
Data-Driven Decision Making: Businesses can make data-driven decisions based on accurate and up-to-date market information by leveraging scraped data. This reduces guesswork and enhances decision-making processes related to content strategies, marketing campaigns, audience targeting, and business growth.
Overall, Mobile App Scraping's OTT Media Platform Data Scraping Services provide businesses with valuable insights, enabling them to stay competitive, improve content offerings, enhance user experiences, optimize monetization strategies, and make informed decisions in the dynamic and rapidly evolving OTT media industry.
How Can OTT Media Platform Data Scraping From Mobile App Scraping Help In Market Analysis And Audience Insights?
OTT Media Platform Data Scraping from Mobile App Scraping can significantly contribute to market analysis and provide valuable audience insights. Here's how it can help:
Market Trends: By scraping data from various OTT media platforms, businesses can gain insights into market trends, including popular content genres, emerging themes, and viewer preferences. This information allows businesses to identify opportunities for content acquisition, production, and strategic partnerships.
Content Performance Analysis: Data scraping enables businesses to analyze the performance of their content and that of competitors. Metrics such as viewership, ratings, reviews, and engagement statistics provide valuable feedback on content quality, audience reception, and areas for improvement.
Audience Segmentation: Through scraped data, businesses can identify different audience segments based on demographics, viewing habits, and content preferences. This segmentation helps tailor content offerings, marketing campaigns, and personalized recommendations to specific target audiences, enhancing user satisfaction and engagement.
User Behavior Analysis: By scraping data, businesses can gain insights into user behavior, including viewing patterns, session duration, content consumption habits, and user interactions with the platform. This information aids in understanding user preferences, habits, and engagement levels, allowing for more effective content planning and curation.
Content Personalization: Scraped data provides valuable inputs for building robust recommendation systems and personalized content delivery. Businesses can offer tailored content suggestions by analyzing user preferences, watch history, and engagement metrics, improving user experiences and increasing user retention.
Competitor Analysis: OTT Media Platform Data Scraping allows businesses to gather data on competitors' content catalogs, ratings, reviews, and audience engagement. This data provides insights into competitor strategies, content gaps, and areas of potential differentiation, supporting competitive analysis and informed decision-making.
Market Positioning: Scraped data helps businesses understand their position within the market. By comparing their content offerings, performance, and audience engagement metrics with competitors, businesses can identify their strengths, weaknesses, and opportunities for differentiation, refining their market positioning.
User Feedback and Sentiment Analysis: Scraped data includes user reviews, ratings, and comments. Analyzing this feedback gives businesses insights into user sentiments, satisfaction levels, and areas for improvement. It helps address user concerns, refine content strategies, and enhance the overall user experience.
OTT Media Platform Data Scraping from Mobile App Scraping empowers businesses with comprehensive market analysis and audience insights, enabling them to make data-driven decisions, optimize content strategies, improve user experiences, and stay ahead in the competitive OTT media landscape.
What Challenges Or Limitations Are Associated With OTT Media Platform Data Scraping?
OTT Media Platform Data Scraping comes with its own set of challenges and limitations. Here are some common ones:
Platform Restrictions: OTT media platforms often have strict terms of service and may explicitly prohibit data scraping or impose limitations on the extent and frequency of data extraction. Adhering to these restrictions is essential to ensure compliance and maintain a positive relationship with the platforms.
Legal and Ethical Considerations: Data scraping must comply with applicable laws, including copyright, intellectual property, and data protection regulations. Respecting user privacy, obtaining necessary permissions, and handling scraped data responsibly and securely is crucial.
Anti-Scraping Measures: OTT platforms may implement anti-scraping measures to protect their data and prevent unauthorized access. These measures can include CAPTCHAs, IP blocking, session monitoring, or other techniques that make scraping more challenging. Overcoming these measures requires advanced scraping techniques and continuous monitoring.
Data Quality and Accuracy: The scraped data may only sometimes be accurate or consistent. Factors such as variations in data formats, incomplete information, or user-generated content can introduce data quality issues. Data cleaning and validation processes must address these challenges and ensure reliable insights.
Dynamic Data Structures: OTT platforms frequently update their interfaces and underlying technologies, leading to changes in the structure and organization of data. This dynamic nature makes it challenging to maintain scraping scripts and adapt them to new versions of the platforms. Regular monitoring and adjustments are necessary to keep the scraping process current.
Data Volume and Processing: OTT platforms generate vast amounts of data, and scraping them can result in significant data volumes. Managing and processing such large-scale data requires robust infrastructure, storage capacity, and processing capabilities. Efficient data handling and analysis methods are crucial to extract meaningful insights.
Capturing Streaming Content: Scraping video or audio content itself poses additional challenges. Unlike static pages, capturing and extracting streaming media requires specialized techniques and tools to handle media codecs, DRM protection, and streaming protocols.
Constant Monitoring and Maintenance: OTT media platforms and their data structures are subject to frequent changes. To ensure continuous and accurate data scraping, ongoing monitoring and maintenance efforts are required to identify and address any disruptions or updates that affect the scraping process.Despite these challenges, with the proper expertise, technical capabilities, and compliance with legal and ethical standards, businesses can overcome the limitations and leverage the valuable insights derived from OTT Media Platform Data Scraping to drive informed decision-making and achieve a competitive advantage in the market.
How Can Businesses Effectively Utilize The Scraped Data From OTT Media Platforms To Enhance Their Marketing And Content Strategies?
Businesses can effectively utilize the scraped data from OTT Media Platforms to enhance their marketing and content strategies in the following ways:
Audience Segmentation and Targeting: Analyze the scraped data to identify distinct audience segments based on demographics, viewing habits, preferences, and engagement metrics. This segmentation helps businesses create targeted marketing campaigns and personalized content recommendations, increasing user engagement and retention.
Content Optimization: Gain insights into content performance, user feedback, and ratings from the scraped data. Use this information to optimize existing content, identify gaps, and develop new content that aligns with audience preferences. This can lead to improved viewer satisfaction and increased viewership.
Personalized Recommendations: Leverage the scraped data to build robust recommendation systems. By understanding user preferences and viewing patterns, businesses can offer personalized content suggestions, enhancing the user experience, increasing content consumption, and driving customer loyalty.

Marketing Campaign Optimization: Utilize the scraped data to optimize marketing campaigns. Identify the most engaging content, determine the best time to release new content, and tailor promotional strategies based on viewer behavior and preferences. This helps maximize the reach and impact of marketing efforts.
Competitive Analysis: Compare scraped data from competitors to gain insights into their content catalogs, ratings, reviews, and viewer engagement. This analysis helps identify competitive advantages, uncover content gaps, and develop differentiation and market positioning strategies.
User Experience Enhancement: Analyze user feedback, ratings, and reviews from the scraped data to identify areas for improvement in user experience. Address user concerns, enhance platform usability, and optimize features and functionalities to increase user satisfaction and retention.
Advertising Campaign Optimization: Utilize scraped data to understand viewer demographics, preferences, and engagement metrics. This information enables businesses to target relevant audiences more precisely, optimize advertising campaigns, and maximize ad revenue.
Pricing and Monetization Strategies: Analyze pricing models, viewer engagement, and competitor data from the scraped information to optimize pricing strategies. Identify opportunities for revenue growth, determine the optimal pricing points, and make informed decisions about monetization options.
By effectively utilizing the scraped data from OTT Media Platforms, businesses can gain valuable insights into their audience, market trends, content performance, and competitive landscape. These insights empower them to make informed decisions, tailor their marketing and content strategies, and ultimately enhance viewer engagement, retention, and business growth.
OTT Media Platform Data Scraping from Mobile App Scraping offers businesses valuable insights to enhance their marketing and content strategies. By leveraging scraped data, businesses can deeply understand audience preferences, content performance, market trends, and competitor landscape. These insights enable businesses to personalize content recommendations, optimize marketing campaigns, improve user experiences, and make data-driven decisions to stay ahead in the dynamic OTT media industry. Take your business to the next level in the OTT media landscape. Contact Mobile App Scraping today to learn more about our OTT Media Platform Data Scraping services and how we can help you leverage the power of data to transform your marketing and content strategies. Let's collaborate and drive success in the ever-evolving world of OTT media.
know more: https://www.mobileappscraping.com/extract-insights-from-ott-media-platforms.php
#ExtractOttMediaApps#OttMediaAppScraper#OttMediaAppsScraping#OttMediaDataScraping#ScrapeOttMediaData
0 notes
Text
Applications of Data Science: Converting Data into Value
Structured data is way easier for programmers to extract information from; the actual challenge lies within the unstructured data due to its myriad formats and surprisingly we have 80% of such data which is growing rapidly by 55%-65% annually. This is where data science makes its itself useful- to extract useful insights and information from the structured and unstructured data to be used in industries for various discoveries and inventions. Data science is similar to data mining which deploys methods and theories inspired from information science, mathematics, computer science, and statistics. The importance is such, that Harvard Business Review declared Data Science as the hottest job of the 21st century. Although, not in its nascency but the past few years have been more crucial for this technology due to the sudden increase of enormous data from different platforms and has already impacted many applications that are given below.

Advertisement Industry
User experience is the pervasive aim of every business to gain, maintain and retain customers. Data science has allowed the marketers to read and analyse consumer behaviour to introduce any new product or service complementing customer preferences. Meanwhile, digital advertising platform is also achieving lucrative benefits via data science algorithms by successfully displaying banners on numerous websites and digital billboards on different public spaces like airports.
Social Media
Face recognition is yet another inspiring application of data science, an extension into video analytics. The use of high bandwidth data services has made the user experience more personalized by giving suggestions to tag the friends while uploading any photo on Facebook. For any business, security, surveillance, object recognition, expression reading, and gesture recognition has become a cakewalk. Whereas code recognition is also carried out by the data science algorithms and it has made many platforms like web Whatsapp, easier, secured and quicker with the help of scanning the barcode. Similarly, face recognition algorithms backed by data science, has allowed the users to search from the images along with the textual keywords.
Banking Sector
Finance sector pioneered the use of data science after getting depressed by the monotonous frauds, duplicity, bad debts, and losses every year. The organizations had enormous data and hence they came up with the idea of utilizing data science to utilize the existing data for exempting themselves from annual losses. Today, organizations are using data science in many forms including Credit Risk Modelling which helped banks to determine such debtors that won’t be able to pay their debts during maturing year.
Gaming Industry
From Zynga, Sony and Activision-Blizzard to EA sports, gaming industry has been utilizing data science algorithm to take every gamer’s experience to a whole new level. Data science reads and mines heavy sets of data received from the user playtime, interactions between players and peak server times to upgrade the gamer’s level by introducing 360-degrees customer view, enhancing gameplay and optimizing real-time game performance.
OTT Sector
Be it Netflix, Amazon or be it social networking giant- Facebook, recommendation feature has become pivotal to their personalization engine for enhanced user experience. Data science tracks and analyses previous search results and produces results which you might like next. Content relevancy has allowed many companies to reap lucrative benefits by promoting their products or services to the targeted audiences. Next time if you see ‘People you may know’ or ‘Shows you may like’, know that it has data science working behind the scenes.
Speech Recognition
Don’t want to type messages? No problem, just say it out. This method of conveying verbal messages that later gets converted into textual form is known as speech recognition which is carried out by the data science algorithms and systems. Alexa, Cortana and Siri are few of the best examples of speech recognition algorithm that are being used widely today to make the search process easier and quicker. The ability of a machine learning system to read and understand human words and phrases still hold caveats but the past few years have been fortunate for the technology due to the improvement in the data science field. This can be quantified in a recent report where Google has improved its speech recognition accuracy rate by 20% since 2013.
Aviation Industry
Earlier, the aviation industry was bearing heavy losses due to high fuel prices, flight cancellations and heavy discounts until the data science and analytics introduced in the sector. Today many airline companies are benefiting from the data mining techniques to interpret customers’ desires and expectations. Southwest Airlines and Alaska Airlines are among such players who are profiting humongously from data-driven systems. Now, passengers receive more personalized messages and ticket booking discounts are offered to the target customers. With the help of predictive analysis skills, aviation companies track and optimize price in real-time and reap more bookings than ever. Also, it has minimised the hassle to take care of baggage for the customers as airlines are using Radio-Frequency Identification to track down any mishandling of the personal asset.
Data without utilization is futile and data science works on the same methodology to extract information and insights to bring lucrative discoveries and inventions to fetch a smarter civilization with data-driven decisions. This also leads to progress in auto machine learning industry in the near future. Furthermore, the job market is considered to be hottest for the data scientists, buoyed by a 2018 research which stated the year had 2.9 million job vacancies in data science field. The demand doesn’t seem to encounter any kind of downfall and shooting up like anything.
0 notes
Text
Applications of Data Science: Converting Data into Value
Structured data is way easier for programmers to extract information from; the actual challenge lies within the unstructured data due to its myriad formats and surprisingly we have 80% of such data which is growing rapidly by 55%-65% annually. This is where data science makes its itself useful- to extract useful insights and information from the structured and unstructured data to be used in industries for various discoveries and inventions. Data science is similar to data mining which deploys methods and theories inspired from information science, mathematics, computer science, and statistics. The importance is such, that Harvard Business Review declared Data Science as the hottest job of the 21st century. Although, not in its nascency but the past few years have been more crucial for this technology due to the sudden increase of enormous data from different platforms and has already impacted many applications that are given below.

Advertisement Industry
User experience is the pervasive aim of every business to gain, maintain and retain customers. Data science has allowed the marketers to read and analyse consumer behaviour to introduce any new product or service complementing customer preferences. Meanwhile, digital advertising platform is also achieving lucrative benefits via data science algorithms by successfully displaying banners on numerous websites and digital billboards on different public spaces like airports.
Social Media
Face recognition is yet another inspiring application of data science, an extension into video analytics. The use of high bandwidth data services has made the user experience more personalized by giving suggestions to tag the friends while uploading any photo on Facebook. For any business, security, surveillance, object recognition, expression reading, and gesture recognition has become a cakewalk. Whereas code recognition is also carried out by the data science algorithms and it has made many platforms like web Whatsapp, easier, secured and quicker with the help of scanning the barcode. Similarly, face recognition algorithms backed by data science, has allowed the users to search from the images along with the textual keywords.
Banking Sector
Finance sector pioneered the use of data science after getting depressed by the monotonous frauds, duplicity, bad debts, and losses every year. The organizations had enormous data and hence they came up with the idea of utilizing data science to utilize the existing data for exempting themselves from annual losses. Today, organizations are using data science in many forms including Credit Risk Modelling which helped banks to determine such debtors that won’t be able to pay their debts during maturing year.
Gaming Industry
From Zynga, Sony and Activision-Blizzard to EA sports, gaming industry has been utilizing data science algorithm to take every gamer’s experience to a whole new level. Data science reads and mines heavy sets of data received from the user playtime, interactions between players and peak server times to upgrade the gamer’s level by introducing 360-degrees customer view, enhancing gameplay and optimizing real-time game performance.
OTT Sector
Be it Netflix, Amazon or be it social networking giant- Facebook, recommendation feature has become pivotal to their personalization engine for enhanced user experience. Data science tracks and analyses previous search results and produces results which you might like next. Content relevancy has allowed many companies to reap lucrative benefits by promoting their products or services to the targeted audiences. Next time if you see ‘People you may know’ or ‘Shows you may like’, know that it has data science working behind the scenes.
Speech Recognition
Don’t want to type messages? No problem, just say it out. This method of conveying verbal messages that later gets converted into textual form is known as speech recognition which is carried out by the data science algorithms and systems. Alexa, Cortana and Siri are few of the best examples of speech recognition algorithm that are being used widely today to make the search process easier and quicker. The ability of a machine learning system to read and understand human words and phrases still hold caveats but the past few years have been fortunate for the technology due to the improvement in the data science field. This can be quantified in a recent report where Google has improved its speech recognition accuracy rate by 20% since 2013.
Aviation Industry
Earlier, the aviation industry was bearing heavy losses due to high fuel prices, flight cancellations and heavy discounts until the data science and analytics introduced in the sector. Today many airline companies are benefiting from the data mining techniques to interpret customers’ desires and expectations. Southwest Airlines and Alaska Airlines are among such players who are profiting humongously from data-driven systems. Now, passengers receive more personalized messages and ticket booking discounts are offered to the target customers. With the help of predictive analysis skills, aviation companies track and optimize price in real-time and reap more bookings than ever. Also, it has minimised the hassle to take care of baggage for the customers as airlines are using Radio-Frequency Identification to track down any mishandling of the personal asset.
Data without utilization is futile and data science works on the same methodology to extract information and insights to bring lucrative discoveries and inventions to fetch a smarter civilization with data-driven decisions. This also leads to progress in auto machine learning industry in the near future. Furthermore, the job market is considered to be hottest for the data scientists, buoyed by a 2018 research which stated the year had 2.9 million job vacancies in data science field. The demand doesn’t seem to encounter any kind of downfall and shooting up like anything.
SOURCE: https://www.elmens.com/business/applications-of-data-science-converting-data-into-value/
0 notes
Text
Top Trends in Cognitive Computing
As we have arrived in the third decade of computing –the cognitive decade–and it will bring on a fundamental change in the manner humans work with machines. There will be a breakthrough in the way the computers used to function earlier, as with the onset of cognitive computing, various software developers and engineers are bridging the gap between the way the computer functions and the way a human brain thinks. The result is intuitive and interactive however which improves over time and can help humans in expanding their limits of capabilities, increasing knowledge and making well-informed decisions.
Cognitive Computing is a new technology that permits people to communicate with computers using a natural language. Earlier, people used to code or format their text in a manner for the system to understand. For example: if they wish to search something, they used to input the keywords. Natural language processing permits the users to ask questions or speak in sentences while communicating with their systems in the same manner as we talk to another person.
How Cognitive computing works?
Cognitive computing systems are a consistent flow of learning techniques. These techniques employ different processes such as data mining, recognition of patterns and language processing. Although, cognitive computing is witnessing a dramatic change as companies are now engaged in developing Artificial Intelligence(AI) and are competing in the market to get a foothold. Cognitive computing can assist us in realising the accurate AI as it helps in imitating the activity of the human brain. These systems can be useful in developing an automated system for solving complex problems by itself.
IBM is the forerunner of Cognitive computing technology. The company has made a billion-dollar investment in big data analytics and is now on the verge to spend near to one-third of its R&D budget in the development of cognitive computing technology.
Pillars of Cognitive Computing
The three main pillars of Cognitive Computing are:
Machine Learning
Big Data Analytics
Cloud Computing
Machine Learning
Machine Learning is making the algorithms put to use. The objective of the algorithm is to examine the data and estimate the trends. Normally, there is a set of training data that feeds the information.
You are required to examine this data on several data parameters to determine its efficiency. However, a self-learning feature is necessary for Cognitive Computing. From there itself, Machine Learning comes into the picture.
Big Data Analytics
The processing of huge amounts of information is the function of the human brain. Understanding the relation of any question is essential. Machines can make it happen faster once you feed the data in it.
But there are two forms of data – Organized and Unorganized. Here, Big Data Analytics plays a role in processing the huge data for you and finding the relevant one you need.
Cloud Computing
If you have huge data to process, you need to have high computing power. The demand is continuously changing in cognitive computing. However, scalability becomes a big factor.
From there Cloud Computing comes in the limelight which helps in providing you with scalable resources. This type of environment works great for Cognitive Computing.
Phenomenal Trends Seen in Cognitive Computing
Chatbots
The simulation of human behaviour by computer programs are known as Chatbots. It employs the feature of Natural Language Processing (NLP).
NLP is used for taking inputs from a human. The chatbots are the intelligent feature used by Cognitive Computing. It can analyse the situation and answer you accordingly.
For example, Chatbots such as Google Assistant, Alexa, Siri are all intelligent bots using cloud-based natural language processing. The cloud capacity permits the business to store user based relevant information and offer tailor-made solutions, products, and messages subject to the behaviour and user preferences. These chatbots optimize the cloud computing potential to offer personalised context significant customer experiences.
Sentiment Analysis
Sentiment Analysis is associated with determining various forms of emotion. Emotions are a normal thing in a human which comes out naturally. But you need to train a machine by feeding a set of data conversations.
Sentimental analysis can be put to use by many companies for splitting up the social media conversation. You can examine individual posts, likes, replies, comments, etc. very spontaneously.
Face Detection
The image processing that detects your upper face is known as face detection. The cognitive system is put to use to analyse the eye colours, lips, contours, etc. to scan a face. Once a facial image has been generated, one can easily recognize the face.
Normally, 2D images are used for face detection. However, it can also be performed for 3D models. Primarily, the face detection is helpful in any security surveillance system.
Risk Management
Examining the possibility of unforeseen risks via current trends prediction is all about risk management. This feature does analyze data, instincts, behaviour, etc. It is a mixture of both art and science.
Cognitive Computing supports you to amass data and trends. Depending on this collection, it can create actionable insights. Moreover, an analyst would be a better person to contact for any further details in this regard.
Fraud Detection
Identifying any abnormal transaction results in fraud detection. In order to study the frauds happened, you need to go through the historical data for identifying the reason. From there, cognitive computing plays a vital role.
Trends by Industry
The top cognitive computing trends are clearly witnessed in the following segments:
(1) Cognitive Computing in OTT
Cognitive Computing capabilities are also harnessed to produce Over-the-Top-Content for delivering video-based content in the form of web series and movies. The cognitive technologies allow the learning and classifying the information from different organized and unstructured data may at times be cumbersome. But the substance administration can potentially be benefitted from AI innovation as feeling, image and voice recognition has involved media innovation buyers to increasingly depend on AI apparatuses to cluster and search for substance files. So that, AI can provide support in substance labelling (which is a costly affair). With the fundamentals of metadata, go through the substance administration structure which ends up having more precision in this way which empowers monetization avenues. Artificial intelligence, coined as “cognitive computing intelligence” in media industry claims to track the user client habits of utilization.
Now, OTT is no more confined with Android-backed Smartphone, instead, manufacturers are developing Android TV sets along with apps to necessarily assist the users in delivering Omnichannel experiences throughout the devices.
(2) Cognitive Computing in News Industry
To allow natural human interaction to flow with cognitive computing tools, the IBM Watson platform has assemblage various capabilities such as:
Speech and Image recognition
In-depth text understanding
Detecting emotion and expression
Translation tools
Relation extraction
When a user wants to read, listen or hear news – with the already embedded cognitive abilities in the device can present the news in the form of text, image or voice according to the user preferences.
The latest news update, forums, weather forecast, sports, politics, business, finance –are all under the cognitive controls backed by four API categories:
Language: An API combination, but not restricted to, categorizing natural language text, entity extraction, conversations, converting documents, extracting semantic concept, language translation, retrieval of passage and ranking, relationship extraction, tone analysis and much more.
Speech: An API combination for speech to text conversion and text to speech conversion which includes the potential to train with your own language models.
Vision: APIs to search new perspectives, gather relevant value and take actionable insights from images.
Data Insights: News is a pre-enriched content that comes with a natural language processing which allows the highly-targeted search and trend analysis.
(3) Cognitive Computing in Retail
Cognitive computing permits enterprises to increase human expertise vigorously. The cognitive computing technologies enable consumers to assist the retail industry to discover new opportunities and make rational shopping decisions.
The technologies also provide interactive, adaptive and contextual systems for making and permit retailers for creating quality tailor-made experiences for individual customers. The following are some rapid trends that are transforming the retail industry:
IBM Watson Commerce
IBM Watson Commerce is a software program for managing the business processes of both retail and online merchandisers. It also focuses on enhancing the customer involvement initiatives. The software program put Insights Assistant to use which help merchandisers to recognize abnormal situations in their marketplaces and advocate suitable actions.
Predictive Analysis
Predictive analysis helps online retailers anticipate what the customers would like to buy in advance. This would help them to predict the customers buying choices such as knowing the highest amount for a certain item the customer is willing to pay. It assists them in optimizing their customer service. Their analytical tools search at the several determinants that can assist in generating the desired involvement from the client such as clicking an online product promotion or subscribing to a newsletter.
Voice Assistants
It is amazing to know the engagement of activated voice digital assistants revolutionising the retail sector. These devices permit the customers to make orders of their preferred product choices and get them delivered within a few hours at their homes.
Direct Purchase via Social Media
Social media platforms such as Facebook, Twitter, Instagram and Pinterest are also navigating the online shopping sales by offering several purchase options. For example: when you see a product suggestion on your social media platform, upon clicking on the product’s image, an application combined with cognitive capabilities will navigate you to the product page. This will help to drive instant traffic on an e-commerce website and enhance the chances of a customer buying a specific product.
Personalized Recommendations
Online retailers use cognitive technology to offer their clients instant personalized recommendations in the form of mobile notifications or e-mail newsletters regarding products they should consider to purchase. This will help the retailers to develop complex insights into their product and generating customer base for a particular product.
Demand Forecasting
To sustain the immediate demand from their customers, online retailers make estimations regarding future demands with the help of demand forecasting. There are a couple of demand forecasting tools that are based on cognitive technology and machine learning will help the online retailers facilitate the precision of deriving probabilities of future demand.
Price Optimization
It becomes difficult for online retailers to accurately determine the price fluctuations responsiveness in their respective markets. This is due to factors such as competitor pricing, supply constraints and complex predictions of customer demands. To face this situation, online retailers can put price optimization tools to use subject to the cognitive technologies for making predictions on how their clients will respond to the price fluctuations of different products or services. These tools help the online retail industry to fulfil their objective of maximizing profit.
0 notes
Text
Recommendation Search Engine Market Development Trends, Competitive Landscape and Key Regions
Market Highlights:
Over the past two decades, the Internet has emerged as the mainstay for online shopping, social networking, e-mail and many more. Corporations also consider the Web as a potential business accelerator. Thus, we see a huge volume of transactional and interaction data generated by the Internet every day. It becomes a herculean task for the user to find the relevant information. A recommendation search engine system provides a solution for this by allocating suggestions for the items to be of use to a user. Recommendation search engine systems have mathematical roots and are more akin to artificial intelligence (AI) than any other IT discipline. A recommendation search engine system learns from a customer’s behavior and recommends a product in which users may be interested. For instance, Netflix recommends movies according to the interest of the user like drama, comedy, action. Netflix went so far as to offer a $1 million prize to researchers who could improve its recommendation engine. After data collection, recommendation search engine system use machine-learning algorithms to find similarities and affinities between products and users.
Request a Sample report @ https://www.marketresearchfuture.com/sample_request/6086
Key Players
Some of the key players of Recommendation Search Engine market include Google (US), IBM (US), Microsoft (US), SAP (Germany), Salesforce (US), HPE (US), Oracle (US), Intel (US), AWS (US), and Sentient Technologies (US).
The prominent players keep innovating and investing in research and development to present cost-effective offerings. Merger and acquisitions among various players are changing the market structure. For instance, Google has acquired Jetpac, makers of an app that recommends destinations based on an analysis of publicly shared Instagram photos. The technology works automatically, extracting information from large numbers of publicly available photos instead of relying on curation or other manual processes.
According to MRFR, The global Recommendation Search Engine market is expected to reach approximately USD 5,900 Million by 2023 growing at a ~40% CAGR over the forecast period 2018-2023.
Regional Analysis
The geographical analysis of Recommendation Search Engine market is studied for North America, Europe Asia Pacific and the rest of the world.
North America is expected to dominate the Recommendation Search Engine market during the forecast period as many organizations are shifting towards new and upgraded technologies with the increasing adoption of digital business strategies. Also due to the rise in the focus of the companies to enhance consumer experience is major driving for the growth of Recommendation Search Engine Market. Asia Pacific is expected to grow at a faster rate due to rapid digitalization and the increasing presence of over the top players (OTT).
Segmentation
On the basis of type, the market is segmented into Collaborative Filtering, Content-Based Filtering, Hybrid Recommendation. Collaborative Filtering is expected to grow at the highest CAGR during the forecast period. This model uses the collaborative power of the ratings provided by multiple users to make recommendations. Collaborative filtering approach doesn’t need a representation of items in terms of features, it is based only on the judgment of the participating user community which is an advantage. Several industries such as Retail, Media & entertainment, and others have deployed recommendation systems powered by AI and Big data for various applications such as personalizing campaigns.
On the basis of Technology, market is segmented into Context-Aware and Geospatial Aware. Context-aware technology is expected to dominate the market as it helps in giving diverse and accurate recommendations to the user. The contextual information includes the location of the user, Identity of people around, date, season, temperature etc. For instance, a website may recommend songs to a user by asking the current mood of the user.
On the basis of Application, market is segmented into Personalized Campaigns and Customer Discovery, Product Planning, Strategy and Operations Planning, Proactive Asset Management. Personalized campaigns and customer discovery application is expected to account for the largest market size during the forecast period. The more the recommendation system
knows about user’s profile, the better it can help to provide customized search results, recommendations or ads.
On the basis of Deployment Mode, market is segmented into On-cloud and On-premise. The Cloud deployment mode is expected to dominate the market due to high adoption of cloud technologies by SMEs as it is simple, efficient & cost effective.
On the basis of End Users, the market is segmented into Media and Entertainment, Retail, Banking, Financial Services, and Insurance, Transportation, Healthcare, and others. Retail Sector is expected to be a strong contender in Recommendation search engine market.
Browse Complete Report @ https://www.marketresearchfuture.com/reports/recommendation-search-engine-market-6086
Target Audience
AI recommendation engine software and platform providers
Training and consulting service providers
AI System integrators
Recommendation Search Engine vendors
Government Agencies
Managed service providers
Research organizations
Value-added Resellers (VARs)
About Market Research Future:
At Market Research Future (MRFR), we enable our customers to unravel the complexity of various industries through our Cooked Research Report (CRR), Half-Cooked Research Reports (HCRR), Raw Research Reports (3R), Continuous-Feed Research (CFR), and Market Research & Consulting Services.
MRFR team have supreme objective to provide the optimum quality market research and intelligence services to our clients. Our market research studies by products, services, technologies, applications, end users, and market players for global, regional, and country level market segments, enable our clients to see more, know more, and do more, which help to answer all their most important questions.
Contact:
Market Research Future
+1 646 845 9312
Email: [email protected]
0 notes
Text
AI and Computer Vision Are Coming - What Marketers Need to Know
Mohammad Shihadah, the founder of IDENTV discusses that less than two decades into the twenty-first century and AI and marketing are set to be a match made in heaven. Or are they? With so much confusion over emerging technologies, here’s how marketers can keep up with AI advances
The artificial intelligence (AI) revolution is upon us, and advanced machines have begun to make their way into the world of marketing. IBM’s Watson is working with brands to provide weather data and help make relevant recipe recommendations. AI companion Aiden provides marketing execs a holistic view of campaign data. And Google’s AI-powered recurrent neural networks (RNN) look set to replace ad targeting with automated ‘memories’.
Yet still, for most marketers, their AI experiences are more likely to be limited to their iPhone assistant Siri, -- and less applicable to daily operations. While industry leaders wait for their turn, and are starting to predict the effects of AI in marketing, what does the revolution really mean?
AI brings us highly-complex automated decisioning, to replace manual efforts. And in a fragmented industry, it will be the cohesive gel to aggregate data and enable real strategy; across traditional TV, digital, social media and more.
Today, these formats cannot be compared, but emerging tools mean that tomorrow’s world will be driven by synergy. Where TV has been a black hole, artificial intelligence is providing detailed measurements and personalization, allowing it to truly integrate with digital and social media marketing channels. So, how are AI and computer vision shining a light on new forms of ad delivery and brand analytics?
The importance of being interactive
The Internet has transformed into video. Surrounded by screens of varying sizes, the connected viewer consumes over-the-top (OTT) video content like never before.
Take Twitter’s newly launched live-streaming tool, for example. It’s been a resounding success, engaging NFL audiences in a live-stream, against a backdrop of hashtags and impassioned fans in one interface. This year, digital tech publication Mashable emptied a large portion of its newsroom in a pivot to video. And masses of user-generated footage is quickly replacing the ubiquitous selfie.
For brands, a switch to video enables engaging formats, on multiple platforms and devices. According to the Interactive Advertising Bureau (IAB), “marketers and advertisers are spending on average more than $10 million annually on Digital Video”, with average budgets up 85% from just two years ago. This year digital ad spend overtook TV, showing that interactive formats work. Mobile is huge, and video is everything.
But linear television commercials are still incredibly important and there is a need to gain real insight into this form of ad delivery and tie all this information together. Brands need to be able to measure responses to the proliferation of ad formats and platforms, to analyze what works, to look at their competitors and placements, realise and track earned media, and attribution.
Interactive digital formats allow this. They enable measurable response metrics and direct call-to-actions. And the emergence of second screen browsing habits means we can start to connect TV and mobile, using visual content and personalized viewing experiences to incite action. This is where computer vision and AI come in.
Computer vision enables dynamic insights
National Security-level computer vision, machine learning and unique video-fingerprinting technologies are bringing video data analytics for advertising professionals into the future. But, how exactly are they doing it?
Computer vision takes all the information from a video, and tagging footage with this data to create a unique video-fingerprint. This involves techniques such as image and audio recognition, to identify objects, faces, sounds and speech from every frame of video or TV content. Capturing this metadata provides text-based, searchable information that can be used to categorize ads, adding semantics to vast libraries of brand content.
Being able to really understand the anatomy of a video in real time creates an interactive environment, where ad delivery can be adjusted based on context and consumer preference. It’s this smart technology that Google uses to power the aforementioned ‘automated memory’ known as RNN. Advanced computing recognizes the objects within each frame of the video, and it translates language and adds relevant captions to online material. Applying this intelligence to TV content provides transparency, enabling a greater level of insight, so that marketers can compare campaign successes across multiple sources.
For instance, this could mean delivering an effective Christmas iTunes TV commercial, immediately after the X-Factor winner is announced, automatically placing the winner center stage within the ad. It means video ads can be built based on consumer tastes, taking cues from user-generated content (UGC) on social media, like in-image social analytics firm GumGum. Or it could enable a salivating soup ad for Campbell, selecting the best scenes and music, in line with weather analytics; delivering a toasty tomato soup ad on a frosty winter’s night.
What this all means for brand strategy
Computer vision in tandem with intelligent machine learning means real-time decisioning and hyper-personalized video targeting. But, more than just enabling flashy new ad delivery tools based on content cues, it opens a door to TV analytics, and measurable insights to understand TV effectiveness, as a part of your overall brand strategy.
AI and computer vision allows marketers to better measure ad effectiveness using real-time data. Automatic flags that are triggered every time an ad shows, or a user interacts with this content in the same location at the same moment, tracks this data. It means user attribution, that rapidly-shrinking window, can be decided in real-time. TV ad data, coupled with data on web traffic and downloads, can expose whether viewers are following calls to action, or whether the media buy should be altered for a different target demographic. AI also fuels accurate measurement of earned media, also helping to calculate improved brand reputation.
Google has released a library of 8 million YouTube video URLs, tagged with object understanding, and Amazon Web Services cloud computing has also released its AI API, applying natural language understanding (NLU), visual search and image recognition, automatic speech recognition (ASR) to visual content. This technology will extract more meaning from TV and video, and expand opportunities for marketers going forward.
With computer vision, companies can track and trace their brand, competitors, products and other information as it is displayed, in real-time. They can do this on cable streams, live TV, social media and any ad placement. This type of emerging technology can even be used to reveal copyright infringements, or negative product portrayal.
Computer vision technology completely changes the whole equation. When you teach a computer to see something, it’s a whole lot more powerful than tagging it with words. AI is here, and it’s going to add meaning to marketing that enables tailored strategy and scheduling flexibility. Armed with big data, marketers can begin to navigate multi-platform discoveries that are guided by intelligent machines. And as we peer into the abyss, computer vision will lead the way.
This article was first appeared on MarTech Advisor
0 notes
Text
How to Extract Insights from OTT Media Platforms: A Guide to Data Scraping?

How to Extract Insights from OTT Media Platforms: A Guide to Data Scraping?
Aug 01, 2023
OTT (Over-The-Top) media platform data scraping refers to extracting relevant data from OTT media platforms. OTT platforms are streaming services that deliver media content directly to users online, bypassing old-style broadcast channels. Some examples of leading OTT media platforms include Netflix, Amazon Prime Video, Hulu, and Disney+.
Data scraping from OTT media platforms involves accessing and extracting various data types, such as user engagement metrics, viewer demographics, content catalogs, ratings and reviews, streaming performance, and more. This data can provide valuable insights for content providers, advertisers, and market researchers to understand audience preferences, track content performance, optimize marketing strategies, and make informed business decisions.
OTT media platform data scraping enables businesses to gather real-time and historical data from multiple platforms. It allows them to analyze trends, identify popular content, target specific audience segments, and enhance content strategies. By leveraging the scraped data, businesses can gain an edge in the highly competitive and rapidly evolving OTT media industry.
How Does OTT Media Platform Data Scraping Work?

OTT Media Platform Data Scraping involves systematically extracting relevant data from OTT media platforms. Here's a simplified overview of how it works:
Identification of Target Platforms: Determine the specific OTT media platforms you want to scrape data from. This could include platforms like Netflix, Hulu, Amazon Prime Video, or any other platform that hosts the content you're interested in.
Data Collection Strategy: Define the scope and parameters of the data you want to scrape. This may include user engagement metrics, content catalogs, ratings and reviews, viewer demographics, streaming performance, and other relevant data points.
Data Scraping Techniques: Employ data scraping techniques to extract the desired data from the OTT platforms. This involves automated software or scripts that navigate the platform's pages, simulate user interactions, and extract data elements based on predefined rules and patterns.
Data Extraction: Use scraping tools to extract the identified data points from the pages. This may involve capturing HTML elements, parsing JSON or XML data, or employing browser automation techniques to interact with the platform's interfaces and retrieve the desired information.
Data Processing and Analysis: Once the data is extracted, it may go through a preprocessing stage to clean and normalize the data for further analysis. This can include removing duplicates, handling missing values, and transforming the data into a structured format suitable for analysis.
Data Storage and Management: Store the scraped data securely and organized, ensuring proper data management practices. This may involve structuring the data into a database, data warehouse, or other storage systems for easy access and retrieval.
Analysis and Insights: Analyze the scraped data to gain actionable insights. This can include performing statistical analysis, visualizing trends, identifying patterns, and deriving meaningful conclusions to inform content strategies, marketing campaigns, or audience targeting.
It's important to note that OTT Media Platform Data Scraping should be conducted ethically and in compliance with the terms of service and legal regulations governing the platforms. Respect user privacy and adhere to data protection guidelines while scraping and handling the extracted data.
What Types Of Data Can Be Scraped From OTT Media Platforms?

Several data types can be scraped from OTT (Over-The-Top) media platforms. The specific data available for scraping may vary depending on the platform and its terms of service. Here are some common types of data that can be scraped from OTT media platforms:
Content Catalog: Information about the available movies, TV shows, documentaries, and other forms of media content, including titles, descriptions, genres, release dates, and duration.
User Engagement Metrics: Data related to user interactions with the platform, such as the number of views, likes, ratings, reviews, comments, and shares for specific content.
Viewer Demographics: Data on the demographic characteristics of platform users, including age, gender, location, and language preferences. This information can help understand the target audience and tailor content strategies accordingly.
Streaming Performance: Metrics related to streaming quality, buffering time, playback errors, and other performance indicators that assess the user experience on the platform.
Recommendations and Personalization: The platform provides data about user preferences, watch history, and personalized recommendations based on user behavior and content consumption patterns.
Ratings and Reviews: User-generated ratings, reviews, and comments on individual movies, TV shows, or episodes. This data can provide insights into audience sentiment and feedback on specific content.
Licensing and Availability: Information regarding content licensing agreements, availability by region, and expiration dates for specific titles. This data is particularly relevant for content acquisition and distribution strategies.
Metadata and Tags: Additional metadata associated with media content, including cast and crew information, production details, keywords, tags, and categorization.
Platform-Specific Data: Each OTT media platform may have unique data points that can be scraped, such as user playlists, recently watched content, or content-specific metrics provided by the platform's API.
By scraping these types of data from OTT media platforms, businesses can gain valuable insights into audience preferences, content performance, and market trends. This information can inform content strategies, marketing campaigns, audience targeting, and other decision-making processes in the dynamic OTT industry.
What Are The Benefits Of Mobile App Scraping's OTT Media Platform Data Scraping Services For Businesses?
Mobile App Scraping's OTT Media Platform Data Scraping Services offer several benefits for businesses in the media industry. Here are some key advantages:
Market Analysis and Audience Insights: Businesses gain valuable market analysis and audience insights by scraping data from OTT media platforms. This includes understanding viewer preferences, consumption patterns, demographic information, and engagement metrics. These insights help make informed decisions about content creation, licensing, marketing, and audience targeting.
Competitive Intelligence: Data scraping allows businesses to gather competitive intelligence by analyzing content catalogs, pricing strategies, user ratings, and reviews of competitors on OTT platforms. This information helps identify market trends, positioning strategies, and opportunities for differentiation.
Content Optimization: Scraped data provides insights into performance, user feedback, and preferences. Businesses can analyze this data to optimize their content offerings, improve user engagement, and tailor their content strategy to meet the evolving demands of their audience.
Personalization and Recommendation Systems: OTT platforms rely on personalized recommendations to enhance user experiences. Businesses can understand user behavior, preferences, and viewing habits by scraping data. This enables them to build more effective recommendation systems, providing personalized content suggestions and improving user satisfaction.
Advertising and Monetization: Data scraping helps businesses identify popular content genres, target relevant audience segments, and optimize advertising campaigns. Businesses can make data-driven decisions to maximize ad revenue and optimize monetization strategies by analyzing user engagement metrics and demographics.
Market Trends and Forecasting: Scraped data from OTT media platforms provide insights into emerging market trends, viewer preferences, and content consumption patterns. This data can be used for market forecasting, predicting future content demand, and making strategic content acquisition and production decisions.
Operational Efficiency: Data scraping automates the process of data collection, allowing businesses to gather large amounts of data from multiple platforms efficiently. This saves time and resources that would otherwise be spent on manual data gathering and analysis.
Data-Driven Decision Making: Businesses can make data-driven decisions based on accurate and up-to-date market information by leveraging scraped data. This reduces guesswork and enhances decision-making processes related to content strategies, marketing campaigns, audience targeting, and business growth.
Overall, Mobile App Scraping's OTT Media Platform Data Scraping Services provide businesses with valuable insights, enabling them to stay competitive, improve content offerings, enhance user experiences, optimize monetization strategies, and make informed decisions in the dynamic and rapidly evolving OTT media industry.
How Can OTT Media Platform Data Scraping From Mobile App Scraping Help In Market Analysis And Audience Insights?
OTT Media Platform Data Scraping from Mobile App Scraping can significantly contribute to market analysis and provide valuable audience insights. Here's how it can help:
Market Trends: By scraping data from various OTT media platforms, businesses can gain insights into market trends, including popular content genres, emerging themes, and viewer preferences. This information allows businesses to identify opportunities for content acquisition, production, and strategic partnerships.
Content Performance Analysis: Data scraping enables businesses to analyze the performance of their content and that of competitors. Metrics such as viewership, ratings, reviews, and engagement statistics provide valuable feedback on content quality, audience reception, and areas for improvement.
Audience Segmentation: Through scraped data, businesses can identify different audience segments based on demographics, viewing habits, and content preferences. This segmentation helps tailor content offerings, marketing campaigns, and personalized recommendations to specific target audiences, enhancing user satisfaction and engagement.
User Behavior Analysis: By scraping data, businesses can gain insights into user behavior, including viewing patterns, session duration, content consumption habits, and user interactions with the platform. This information aids in understanding user preferences, habits, and engagement levels, allowing for more effective content planning and curation.
Content Personalization: Scraped data provides valuable inputs for building robust recommendation systems and personalized content delivery. Businesses can offer tailored content suggestions by analyzing user preferences, watch history, and engagement metrics, improving user experiences and increasing user retention.
Competitor Analysis: OTT Media Platform Data Scraping allows businesses to gather data on competitors' content catalogs, ratings, reviews, and audience engagement. This data provides insights into competitor strategies, content gaps, and areas of potential differentiation, supporting competitive analysis and informed decision-making.
Market Positioning: Scraped data helps businesses understand their position within the market. By comparing their content offerings, performance, and audience engagement metrics with competitors, businesses can identify their strengths, weaknesses, and opportunities for differentiation, refining their market positioning.
User Feedback and Sentiment Analysis: Scraped data includes user reviews, ratings, and comments. Analyzing this feedback gives businesses insights into user sentiments, satisfaction levels, and areas for improvement. It helps address user concerns, refine content strategies, and enhance the overall user experience.
OTT Media Platform Data Scraping from Mobile App Scraping empowers businesses with comprehensive market analysis and audience insights, enabling them to make data-driven decisions, optimize content strategies, improve user experiences, and stay ahead in the competitive OTT media landscape.
What Challenges Or Limitations Are Associated With OTT Media Platform Data Scraping?
OTT Media Platform Data Scraping comes with its own set of challenges and limitations. Here are some common ones:
Platform Restrictions: OTT media platforms often have strict terms of service and may explicitly prohibit data scraping or impose limitations on the extent and frequency of data extraction. Adhering to these restrictions is essential to ensure compliance and maintain a positive relationship with the platforms.
Legal and Ethical Considerations: Data scraping must comply with applicable laws, including copyright, intellectual property, and data protection regulations. Respecting user privacy, obtaining necessary permissions, and handling scraped data responsibly and securely is crucial.
Anti-Scraping Measures: OTT platforms may implement anti-scraping measures to protect their data and prevent unauthorized access. These measures can include CAPTCHAs, IP blocking, session monitoring, or other techniques that make scraping more challenging. Overcoming these measures requires advanced scraping techniques and continuous monitoring.
Data Quality and Accuracy: The scraped data may only sometimes be accurate or consistent. Factors such as variations in data formats, incomplete information, or user-generated content can introduce data quality issues. Data cleaning and validation processes must address these challenges and ensure reliable insights.
Dynamic Data Structures: OTT platforms frequently update their interfaces and underlying technologies, leading to changes in the structure and organization of data. This dynamic nature makes it challenging to maintain scraping scripts and adapt them to new versions of the platforms. Regular monitoring and adjustments are necessary to keep the scraping process current.
Data Volume and Processing: OTT platforms generate vast amounts of data, and scraping them can result in significant data volumes. Managing and processing such large-scale data requires robust infrastructure, storage capacity, and processing capabilities. Efficient data handling and analysis methods are crucial to extract meaningful insights.
Capturing Streaming Content: Scraping video or audio content itself poses additional challenges. Unlike static pages, capturing and extracting streaming media requires specialized techniques and tools to handle media codecs, DRM protection, and streaming protocols.
Constant Monitoring and Maintenance: OTT media platforms and their data structures are subject to frequent changes. To ensure continuous and accurate data scraping, ongoing monitoring and maintenance efforts are required to identify and address any disruptions or updates that affect the scraping process.Despite these challenges, with the proper expertise, technical capabilities, and compliance with legal and ethical standards, businesses can overcome the limitations and leverage the valuable insights derived from OTT Media Platform Data Scraping to drive informed decision-making and achieve a competitive advantage in the market.
How Can Businesses Effectively Utilize The Scraped Data From OTT Media Platforms To Enhance Their Marketing And Content Strategies?
Businesses can effectively utilize the scraped data from OTT Media Platforms to enhance their marketing and content strategies in the following ways:
Audience Segmentation and Targeting: Analyze the scraped data to identify distinct audience segments based on demographics, viewing habits, preferences, and engagement metrics. This segmentation helps businesses create targeted marketing campaigns and personalized content recommendations, increasing user engagement and retention.
Content Optimization: Gain insights into content performance, user feedback, and ratings from the scraped data. Use this information to optimize existing content, identify gaps, and develop new content that aligns with audience preferences. This can lead to improved viewer satisfaction and increased viewership.
Personalized Recommendations: Leverage the scraped data to build robust recommendation systems. By understanding user preferences and viewing patterns, businesses can offer personalized content suggestions, enhancing the user experience, increasing content consumption, and driving customer loyalty.

Marketing Campaign Optimization: Utilize the scraped data to optimize marketing campaigns. Identify the most engaging content, determine the best time to release new content, and tailor promotional strategies based on viewer behavior and preferences. This helps maximize the reach and impact of marketing efforts.
Competitive Analysis: Compare scraped data from competitors to gain insights into their content catalogs, ratings, reviews, and viewer engagement. This analysis helps identify competitive advantages, uncover content gaps, and develop differentiation and market positioning strategies.
User Experience Enhancement: Analyze user feedback, ratings, and reviews from the scraped data to identify areas for improvement in user experience. Address user concerns, enhance platform usability, and optimize features and functionalities to increase user satisfaction and retention.
Advertising Campaign Optimization: Utilize scraped data to understand viewer demographics, preferences, and engagement metrics. This information enables businesses to target relevant audiences more precisely, optimize advertising campaigns, and maximize ad revenue.
Pricing and Monetization Strategies: Analyze pricing models, viewer engagement, and competitor data from the scraped information to optimize pricing strategies. Identify opportunities for revenue growth, determine the optimal pricing points, and make informed decisions about monetization options.
By effectively utilizing the scraped data from OTT Media Platforms, businesses can gain valuable insights into their audience, market trends, content performance, and competitive landscape. These insights empower them to make informed decisions, tailor their marketing and content strategies, and ultimately enhance viewer engagement, retention, and business growth.
OTT Media Platform Data Scraping from Mobile App Scraping offers businesses valuable insights to enhance their marketing and content strategies. By leveraging scraped data, businesses can deeply understand audience preferences, content performance, market trends, and competitor landscape. These insights enable businesses to personalize content recommendations, optimize marketing campaigns, improve user experiences, and make data-driven decisions to stay ahead in the dynamic OTT media industry. Take your business to the next level in the OTT media landscape. Contact Mobile App Scraping today to learn more about our OTT Media Platform Data Scraping services and how we can help you leverage the power of data to transform your marketing and content strategies. Let's collaborate and drive success in the ever-evolving world of OTT media.
know more: https://www.mobileappscraping.com/extract-insights-from-ott-media-platforms.php
#ExtractOttMediaApps#OttMediaAppScraper#OttMediaAppsScraping#OttMediaDataScraping#ScrapeOttMediaData
0 notes
Text
Recommendation Search Engine Market Supply, Sales, Revenue and Forecast from 2018 to 2023
Market Highlights:
Over the past two decades, the Internet has emerged as the mainstay for online shopping, social networking, e-mail and many more. Corporations also consider the Web as a potential business accelerator. Thus, we see a huge volume of transactional and interaction data generated by the Internet every day. It becomes a herculean task for the user to find the relevant information. A recommendation search engine system provides a solution for this by allocating suggestions for the items to be of use to a user. Recommendation search engine systems have mathematical roots and are more akin to artificial intelligence (AI) than any other IT discipline. A recommendation search engine system learns from a customer’s behavior and recommends a product in which users may be interested. For instance, Netflix recommends movies according to the interest of the user like drama, comedy, action. Netflix went so far as to offer a $1 million prize to researchers who could improve its recommendation engine. After data collection, recommendation search engine system use machine-learning algorithms to find similarities and affinities between products and users.
Request a Sample report @ https://www.marketresearchfuture.com/sample_request/6086
Key Players
Some of the key players of Recommendation Search Engine market include Google (US), IBM (US), Microsoft (US), SAP (Germany), Salesforce (US), HPE (US), Oracle (US), Intel (US), AWS (US), and Sentient Technologies (US).
The prominent players keep innovating and investing in research and development to present cost-effective offerings. Merger and acquisitions among various players are changing the market structure. For instance, Google has acquired Jetpac, makers of an app that recommends destinations based on an analysis of publicly shared Instagram photos. The technology works automatically, extracting information from large numbers of publicly available photos instead of relying on curation or other manual processes.
According to MRFR, The global Recommendation Search Engine market is expected to reach approximately USD 5,900 Million by 2023 growing at a ~40% CAGR over the forecast period 2018-2023.
Regional Analysis
The geographical analysis of Recommendation Search Engine market is studied for North America, Europe Asia Pacific and the rest of the world.
North America is expected to dominate the Recommendation Search Engine market during the forecast period as many organizations are shifting towards new and upgraded technologies with the increasing adoption of digital business strategies. Also due to the rise in the focus of the companies to enhance consumer experience is major driving for the growth of Recommendation Search Engine Market. Asia Pacific is expected to grow at a faster rate due to rapid digitalization and the increasing presence of over the top players (OTT).
Segmentation
On the basis of type, the market is segmented into Collaborative Filtering, Content-Based Filtering, Hybrid Recommendation. Collaborative Filtering is expected to grow at the highest CAGR during the forecast period. This model uses the collaborative power of the ratings provided by multiple users to make recommendations. Collaborative filtering approach doesn’t need a representation of items in terms of features, it is based only on the judgment of the participating user community which is an advantage. Several industries such as Retail, Media & entertainment, and others have deployed recommendation systems powered by AI and Big data for various applications such as personalizing campaigns.
On the basis of Technology, market is segmented into Context-Aware and Geospatial Aware. Context-aware technology is expected to dominate the market as it helps in giving diverse and accurate recommendations to the user. The contextual information includes the location of the user, Identity of people around, date, season, temperature etc. For instance, a website may recommend songs to a user by asking the current mood of the user.
On the basis of Application, market is segmented into Personalized Campaigns and Customer Discovery, Product Planning, Strategy and Operations Planning, Proactive Asset Management. Personalized campaigns and customer discovery application is expected to account for the largest market size during the forecast period. The more the recommendation system
knows about user’s profile, the better it can help to provide customized search results, recommendations or ads.
On the basis of Deployment Mode, market is segmented into On-cloud and On-premise. The Cloud deployment mode is expected to dominate the market due to high adoption of cloud technologies by SMEs as it is simple, efficient & cost effective.
On the basis of End Users, the market is segmented into Media and Entertainment, Retail, Banking, Financial Services, and Insurance, Transportation, Healthcare, and others. Retail Sector is expected to be a strong contender in Recommendation search engine market.
Browse Complete Report @ https://www.marketresearchfuture.com/reports/recommendation-search-engine-market-6086
Target Audience
AI recommendation engine software and platform providers
Training and consulting service providers
AI System integrators
Recommendation Search Engine vendors
Government Agencies
Managed service providers
Research organizations
Value-added Resellers (VARs)
0 notes
Text
Recommendation Search Engine Market – Size, Trends, Growth, Outlook and Forecast to 2023
Market Highlights:
With the beginning of web era, there has been an information overload over the internet which often makes it exhaustive for the user to get the relevant information. This issue is resolved by search engines like Google, Yahoo and many more,however, even they fail to provide personalized data. So, to additionally filter the data we need a recommendation search engine. Recommendation systems are software and techniques, designed with an objective to provide a useful and sensible recommendation to users for items or products that might interest them. Recommendation system typically does not use an explicit query, instead analyzes the user context and user profile, i.e., what the user has recently purchased or read. Then the recommendation mechanism provides the user with one or more specification of objects that may be of interest.
Recommendation search engine system predicts consumer needs based on previous purchase history, online behavior, ratings, reviews, and other personalized attributes. Nowadays companies are investing in real-time technologies to understand consumer behavior at a more granular level across channels and devices. The objective of recommendation search engine is to get more personalized recommendations, customer satisfaction, and increase the sales. Recommendation search engine solves common problems that general search engine face such as inability to scale, generate relevant recommendations, provide flexible processing plans, etc. The recommendation search engine market is gaining momentum to enhance the consumer experience and increase sales.
Request a Sample report @ https://www.marketresearchfuture.com/sample_request/6086
Key Players
Some of the key players of Recommendation Search Engine market include Google (US), IBM (US), Microsoft (US), SAP (Germany), Salesforce (US), HPE (US), Oracle (US), Intel (US), AWS (US), and Sentient Technologies (US).
The prominent players keep innovating and investing in research and development to present cost-effective offerings. Merger and acquisitions among various players are changing the market structure. For instance, Google has acquired Jetpac, makers of an app that recommends destinations based on an analysis of publicly shared Instagram photos. The technology works automatically, extracting information from large numbers of publicly available photos instead of relying on curation or other manual processes.
According to MRFR, The global Recommendation Search Engine market is expected to reach approximately USD 5,900 Million by 2023 growing at a ~40% CAGR over the forecast period 2018-2023.
Regional Analysis
The geographical analysis of Recommendation Search Engine market is studied for North America, Europe Asia Pacific and the rest of the world.
North America is expected to dominate the Recommendation Search Engine market during the forecast period as many organizations are shifting towards new and upgraded technologies with the increasing adoption of digital business strategies. Also due to the rise in the focus of the companies to enhance consumer experience is major driving for the growth of Recommendation Search Engine Market. Asia Pacific is expected to grow at a faster rate due to rapid digitalization and the increasing presence of over the top players (OTT).
Segmentation
By Type, the market is segmented into Collaborative Filtering, Content-Based Filtering, Hybrid Recommendation.
By Technology, the market is segmented into Context-Aware and Geospatial Aware. Context-Aware is further segmented into Machine Learning and Deep Learning, and Natural Language Processing.
By Application, the market is segmented into Personalized Campaigns and Customer Discovery, Product Planning, Strategy and Operations Planning, Proactive Asset Management.
By Deployment, the market is segmented into on Cloud and On-Premise.
By End Users, the market is segmented into Media and Entertainment, Retail, Banking, Financial Services, and Insurance, Transportation, Healthcare, and others.
Browse Complete Report @ https://www.marketresearchfuture.com/reports/recommendation-search-engine-market-6086
Target Audience
AI recommendation engine software and platform providers
Training and consulting service providers
AI System integrators
Recommendation Search Engine vendors
Government Agencies
Managed service providers
Research organizations
Value-added Resellers (VARs)
0 notes