#maxdiff research
Explore tagged Tumblr posts
Text
Unveiling the Power of Market Research Analytics: A Strategic Imperative for Business Success
Introduction -
In today's fast-paced and hyper-competitive business landscape, gaining a competitive edge requires more than just intuition and guesswork. Enter market research analytics â an essential approach that empowers businesses to make informed decisions, uncover hidden insights, and navigate the complex maze of consumer preferences and market trends. In this blog, we take a deep dive into the world of market research analytics, exploring its significance, methodologies, and the transformative impact it can have on your business.

The Significance of Market Research Analytics -
Market research analytics is the art and science of extracting actionable insights from raw data to drive strategic decision-making. It provides a structured approach to understanding consumer behavior, market dynamics, and industry trends. By leveraging data-driven insights, businesses can:
Enhance Customer Understanding: By analyzing consumer preferences, buying patterns, and sentiment, businesses can tailor their products and services to meet customer needs more effectively.
Competitor Analysis: Market research analytics enables companies to assess competitor strengths and weaknesses, identify gaps in the market, and formulate strategies to gain a competitive advantage.
Optimize Marketing Efforts: Precise data analysis allows businesses to target their marketing campaigns with laser-like precision, reducing costs and increasing conversion rates.
Product Innovation: Uncovers latent customer needs and pain points through data analysis, fuels the creation of innovative products that resonate with the target audience.

Methodologies in Market Research Analytics â
In the domain of Market Research Analytics, diverse methodologies play a pivotal role in facilitating informed and sound decision-making. These methodologies empower businesses with the tools to untangle complex market dynamics, cultivate a deeper understanding of consumer preferences and enable the formulation of impactful strategies.
Quantitative Analysis: This approach involves the use of numerical data to measure, quantify, and analyze various aspects of the market. Surveys, polls, and structured questionnaires are common tools used to gather data for quantitative analysis.
Qualitative Analysis: Qualitative research delves into the subjective aspects of consumer behavior, focusing on insights that are not easily quantifiable. Techniques such as focus groups, in-depth interviews, and content analysis provide valuable context and depth to numerical data.
Predictive Analytics: Using historical data and statistical algorithms, predictive analytics helps forecast future trends, customer behavior, and market shifts. This enables businesses to proactively adapt and strategize.
Text and Sentiment Analysis: With the proliferation of online reviews, social media, and user-generated content, extracting insights from text data has become crucial. Text and sentiment analysis tools decipher consumer sentiment, helping businesses gauge public opinion and adjust strategies accordingly.

Transformative Impact on Business-
Market research analytics has different impacts which transforms business into more successful entity. Brands can improve their bottom line and build stronger relationships with their customers by providing high quality products/services. Embracing market research analytics can usher in a myriad of benefits for businesses:
Informed Decision-Making: Accurate data-driven insights provide a solid foundation, reducing the element of risk and uncertainty in strategic decision-making. Â
Cost Efficiency: By focusing resources on targeted strategies and campaigns, businesses can optimize their marketing budgets and operational expenditures.
Agility and Adaptability: Real-time data analysis equips businesses to swiftly respond to changing market conditions, ensuring they remain relevant and adaptable.
Customer-Centric Approach: By understanding consumer preferences and pain points, businesses can align their offerings with customer needs, thereby fostering brand loyalty and customer satisfaction.
Innovation Catalyst: Market research analytics can uncover untapped opportunities, enabling businesses to innovate and stay ahead of the curve.
Conclusion -
In a business landscape driven by data and insights, market research analytics emerges as a strategic imperative for sustainable success. By deciphering the intricate web of consumer behavior, market trends, and competition dynamics, businesses can chart a course towards informed decision-making, innovation, and customer-centricity. Embracing market research analytics isn't just an option; it's a powerful tool that can unlock the doors to unparalleled growth and prosperity in today's dynamic marketplace.
#market research survey#cati market research#sawtooth maxdiff#market study#data validation#market data analysis#maxdiff research#conjoint method
1 note
·
View note
Text
Empowering Growth: Tehrihills Consulting's Market Research Solutions
In today's dynamic landscape of market research and data analysis, Tehrihills Consulting emerges as your strategic partner, focusing on tailoring services to provide industry-specific insights that empower your decision-making process. Our approach involves navigating market trends with precision, ensuring your strategies are backed by robust and relevant data.
Tailored Market Research Expertise
Tehrihills Consulting specializes in conjoint analysis, utilizing advanced tools like confirmit survey programming and decipher survey programming and data modeling. Our commitment extends beyond conventional approaches; we don't just provide insights, but empower you with actionable intelligence. Whether it's Global Data Collection and Analysis or a focus on local market nuances, Tehrihills Consulting is your trusted partner.
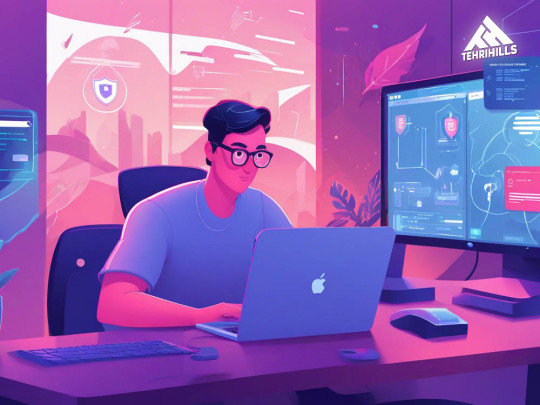
Mastery of Data and Analytics
Our dedication to excellence encompasses mastery of data. Experience the full potential of your data as we craft success-fueling strategies. Leveraging MaxDiff analysis, top survey programming software, and ensuring data validation, we guarantee transformative results. Our precision in understanding market dynamics is reflected in our approach to CATI market research, ensuring your strategies remain at the forefront of innovation.
Global Insights, Local Expertise
Tehrihills Consulting offers a unique blend of global insights and local expertise. Comprehensive global data collection spans borders, providing a panoramic view of market trends. This global perspective, coupled with an understanding of local markets, empowers you to formulate strategies resonating globally while addressing local nuances.
Your Tehrihills Consulting Partnership
Partnering with Tehrihills Consulting means access to industry-leading expertise and innovative solutions. We redefine excellence in every project, ensuring your business not only adapts to market changes but leads the way in innovation. As a dedicated market research company, Tehrihills focuses on MaxDiff analysis, utilizing top survey programming software, and data validation, setting the highest standards in our services.
As we embark on this transformative journey together, Tehrihills Consulting invites you to join us in redefining the boundaries of excellence through innovative market research solutions. Explore myriad possibilities, discover new avenues, and unlock success with Tehrihills Consulting at your side. We believe in not just meeting your expectations but exceeding them, making every project a testament to our commitment to excellence in market research. Elevate your strategies, amplify your successâpartner with Tehrihills Consulting, where every insight becomes a stepping stone toward a brighter future for your business.
Join us on the journey of innovation and excellence!
#market research#decipher#survey programming#researchreports#market research solutions#data validation#MaxDiff analysis#CATI market research
1 note
·
View note
Text
0 notes
Text
MaxDiff RL Algorithm Improves Robotic Learning with âDesigned Randomnessâ
New Post has been published on https://thedigitalinsider.com/maxdiff-rl-algorithm-improves-robotic-learning-with-designed-randomness/
MaxDiff RL Algorithm Improves Robotic Learning with âDesigned Randomnessâ
In a groundbreaking development, engineers at Northwestern University have created a new AI algorithm that promises to transform the field of smart robotics. The algorithm, named Maximum Diffusion Reinforcement Learning (MaxDiff RL), is designed to help robots learn complex skills rapidly and reliably, potentially revolutionizing the practicality and safety of robots across a wide range of applications, from self-driving vehicles to household assistants and industrial automation.
The Challenge of Embodied AI Systems
To appreciate the significance of MaxDiff RL, it is essential to understand the fundamental differences between disembodied AI systems, such as ChatGPT, and embodied AI systems, like robots. Disembodied AI relies on vast amounts of carefully curated data provided by humans, learning through trial and error in a virtual environment where physical laws do not apply, and individual failures have no tangible consequences. In contrast, robots must collect data independently, navigating the complexities and constraints of the physical world, where a single failure can have catastrophic implications.
Traditional algorithms, designed primarily for disembodied AI, are ill-suited for robotics applications. They often struggle to cope with the challenges posed by embodied AI systems, leading to unreliable performance and potential safety hazards. As Professor Todd Murphey, a robotics expert at Northwesternâs McCormick School of Engineering, explains, âIn robotics, one failure could be catastrophic.â
MaxDiff RL: Designed Randomness for Better Learning
To bridge the gap between disembodied and embodied AI, the Northwestern team focused on developing an algorithm that enables robots to collect high-quality data autonomously. At the heart of MaxDiff RL lies the concept of reinforcement learning and âdesigned randomness,â which encourages robots to explore their environments as randomly as possible, gathering diverse and comprehensive data about their surroundings.
By learning through these self-curated, random experiences, robots can acquire the necessary skills to accomplish complex tasks more effectively. The diverse dataset generated through designed randomness enhances the quality of the information robots use to learn, resulting in faster and more efficient skill acquisition. This improved learning process translates to increased reliability and performance, making robots powered by MaxDiff RL more adaptable and capable of handling a wide range of challenges.
Putting MaxDiff RL to the Test
To validate the effectiveness of MaxDiff RL, the researchers conducted a series of tests, pitting the new algorithm against current state-of-the-art models. Using computer simulations, they tasked robots with performing a range of standard tasks. The results were remarkable: robots utilizing MaxDiff RL consistently outperformed their counterparts, demonstrating faster learning speeds and greater consistency in task execution.
Perhaps the most impressive finding was the ability of robots equipped with MaxDiff RL to succeed at tasks in a single attempt, even when starting with no prior knowledge. As lead researcher Thomas Berrueta notes, âOur robots were faster and more agile â capable of effectively generalizing what they learned and applying it to new situations.â This ability to âget it right the first timeâ is a significant advantage in real-world applications, where robots cannot afford the luxury of endless trial and error.
Potential Applications and Impact
The implications of MaxDiff RL extend far beyond the realm of research. As a general algorithm, it has the potential to revolutionize a wide array of applications, from self-driving cars and delivery drones to household assistants and industrial automation. By addressing the foundational issues that have long hindered the field of smart robotics, MaxDiff RL paves the way for reliable decision-making in increasingly complex tasks and environments.
The versatility of the algorithm is a key strength, as co-author Allison Pinosky highlights: âThis doesnât have to be used only for robotic vehicles that move around. It also could be used for stationary robots â such as a robotic arm in a kitchen that learns how to load the dishwasher.â As the complexity of tasks and environments grows, the importance of embodiment in the learning process becomes even more critical, making MaxDiff RL an invaluable tool for the future of robotics.
A Leap Forward in AI and Robotics
The development of MaxDiff RL by Northwestern University engineers marks a significant milestone in the advancement of smart robotics. By enabling robots to learn faster, more reliably, and with greater adaptability, this innovative algorithm has the potential to transform the way we perceive and interact with robotic systems.
As we stand on the cusp of a new era in AI and robotics, algorithms like MaxDiff RL will play a crucial role in shaping the future. With its ability to address the unique challenges faced by embodied AI systems, MaxDiff RL opens up a world of possibilities for real-world applications, from enhancing safety and efficiency in transportation and manufacturing to revolutionizing the way we live and work alongside robotic assistants.
As research continues to push the boundaries of what is possible, the impact of MaxDiff RL and similar advancements will undoubtedly be felt across industries and in our daily lives. The future of smart robotics is brighter than ever, and with algorithms like MaxDiff RL leading the way, we can look forward to a world where robots are not only more capable but also more reliable and adaptable than ever before.
#agile#ai#AI systems#algorithm#Algorithms#applications#arm#Art#automation#bridge#Cars#challenge#chatGPT#complexity#comprehensive#computer#data#development#diffusion#drones#efficiency#engineering#engineers#Environment#Fundamental#Future#gap#hazards#heart#household
1 note
·
View note
Text
How to Spark Customer Interest in Your Business Market Research
Image credit
As you already know, the landscape for businesses is super dynamic. Honestly, this is something thatâs just getting more prevalent. There are more expectations and changes, and trends are coming faster than ever. Seriously, the list could keep going on and on. So, with all of that said, staying ahead of the curve requires more than just great products or services.Â
It demands a deep understanding of your target audience, competitors, and industry trends. Itâs a bit scary to think, but nowadays, itâs something that really needs to be constant. This means that you need to constantly consider things and try to keep it all in mind.
Market research is the compass guiding your business decisions, and engaging your customers in this process can bring invaluable insights. But you also need to keep in mind that market research has to be meaningful research, too. So, how can you achieve that? Well, you need to get your customers involved; they need to actually show interest in helping you out. So, with all of that said, here are some effective strategies to pique customer interest in your market research efforts.
Communicate the Value Proposition
Sometimes, it truly is as simple as just communicating to them (wild, right?) All you really need to do is clearly articulate the benefits of your market research. Be sure to just explain how it will enhance the customer experience, improve product offerings, or address their pain points. When customers see the direct impact on their needs and preferences, they're more likely to engage.
The Surveys You Do Need to be Engaging
So, you really need to keep in mind that you have to try and design the surveys in a way that is not only concise and easy to understand but also engaging. Incorporate interactive elements, visuals, and varied question types to keep respondents interested.Â
It might even help to do the MaxDiff analysis because itâs easier for them to answer the questions and is usually easier for you to break down the answers (and analyze them). So, to sum it all up, really, itâs going to be about creating a well-crafted survey. It needs to be easy to do, not lengthy, and with this, itâs usually more likely to capture and maintain attention.
Offer Some Incentives
Everyone loves a reward. Most market research firms will do this because they know for a fact it gets people to present stuff. So, be sure to just consider offering incentives such as discounts, exclusive access, or even entry into a giveaway for those who participate in your market research. Generally speaking, though, tangible benefits can significantly boost customer motivation to engage.
How You Reach Out Counts
Mass emails donât work; very rarely will a post on social media work either. Instead, youâre going to have to just tailor your communication to individual customers based on their preferences and behaviors. Honestly, itâs as simple as personalized emails or messages that explain how their unique input is valuable and can make customers feel seen and appreciated, increasing their likelihood to participate. You canât make this a mass email; it needs to be personal; this is the only way there is to it!
0 notes
Photo
Research Solutions By Maxdiff Analysis In USA
Max Diff analysis is a new technique by Knowledge Excel used by the researchers for a much easier application and can be used for a large variety of research scenarios. Its application gives the best-worst scaling that can help in sorting out the data much easier and to discriminate among the products and respondents. Max Diff Analysis is a sustainable approach to researchers to obtain the preference over importance scores for any item. It compiles the marketing or the social survey research with a lot of attractive features. MaxDiff Analysis provides ranges from message testing, product features to brand preference and customer satisfaction.
1 note
·
View note
Text
Investigating Consumersâ Preference on Fresh Vegetables in Bangladesh: Best-Worst Scaling Approach- Juniper Publishers
Safety vegetables are nutritionally essential for our good health, but we are daily fighting against to take âSafe and Fresh Vegetablesâ for âsake of âGood Healthâ due to contamination, adulterations and pesticides residues effects. The aim of the study was to examine the consumersâ preference for food quality and safety attributes of fresh vegetables by using quality and safety attributes of fresh vegetables using the best-worst scaling technique. The balanced incomplete block design procedure was employed to obtain a total 180 sample from Dhaka and Mymensingh city of Bangladesh. The data were collected through survey monkey software by using the pre-determined structured questionnaire and analyzed by the MaxDiff scaling and multinomial mixed logit regression model. The results revealed that fresh vegetable attributes were a combination of size, appearance, texture, freshness, and flavor as well as nutritional ingredients and safety aspects that determine their value to the consumer. The fresh vegetables contained vitamins, minerals and dietary fiber that satisfied consumer demand and helped to keep healthy and protect from diseases. The researchers found that nutritional value, purity, freshness, shelf life and safety are the most important attributes of fresh vegetables and variety, size, content, colour are the least important for the consumers. The study concludes that demand for can be significantly stimulated in the study area when these most important attributes have been considered by producers, processors, and marketers.
Keywords: Fresh Vegetables; Consumers âpreferences; Food safety; Products attributes; BWS
  Introduction
Bangladesh is the 3rd position in global vegetable production, but it remained in the lower position in intake. The total vegetables production is 3.73 million but demand annually 13.25 million metric tonnes in Bangladesh (FAO, 2015). According to the World Health Organization (WHO), a healthy individual should take 220 grams of vegetable daily of which Bangladesh is intake only 70 percent of need. Vegetables are the main sources of essential vitamins such as A, C, niacin, riboflavin and thiamin, minerals such as calcium and iron and dietary fiber. They added to eat of crucial nutrients from other foods by making the more taste and dietary fiber necessary for digestion and maintaining health curing nutritional disorders [1]. Vegetables are not only minimizing the malnutrition but also maximize the economic returns.
Farmers are widely using toxic chemicals for vegetables production and pesticides uses to fight against pests. Thatâs why vegetables are adulterated by various harmful chemicals and pesticides. On the other hand, traders are using toxic artificial ingredient and colors to extend its appearance and shelf life. Using chemical preservatives without concern for the health of the consumer is rampant. Now a day, food adulteration is becoming a âSilent Killerâ in Bangladesh. Because, vegetables are contaminated by toxic chemicals and pesticides like carbide, formalin, heavy metal, chemical, textile colors, artificial sweeteners, DDT, urea and so on pose a serious threat to public health especially damaging vital organs like liver, kidney, pancreas etc. It is very difficult to find fresh and adulteration free vegetables in the market. For this reason, the worldwide fresh vegetables market has growing rapidly in recent years to a multi-billion-dollar sector, largely driven by increasing population growth, urbanization and changing consumption pattern as a result increasing consumer demand for healthy, freshly prepared convenient vegetables [2]. The quality of fresh vegetable includes a combination of attributes like appearance, texture and flavor, as well as nutritional and safety aspects that determine their value to the consumer. But challenge for producers, traders and retailers in market is to predict consumer preferences for vegetables, because consumers represent the essential demand for vegetables and this demand is always not stable. Moreover, several studies [3,4] have been undertaken to determine consumer preferences for vegetable attributes and respective willingness to pay for various characteristics, thereby providing vegetables with desirable attributes to consumers and keeping the market fresh and alive. Furthermore, the lack of food safety, food security, nutrition and profitability are a problem no inherent to nature but with unjust societal systems and institutions. Lusk and Briggeman [5] also argued that understanding why consumers prefer a given attribute is important to determine the presence of market failure and therefore the need for public policy intervention. Thatâs why, researchers set the objective of study is to elicit fresh vegetables attribute preferences among quality conscious consumers in Dhaka city and Mymensingh city of Bangladesh.
  Theoretical framework of Best-Worst Scaling (BWS)
Best-Worst Scaling (BWS) is also called Maximum Difference Scaling (MaxDiff.) which is based on random utility theory (Thurstone, 1927; Cohen 2003) [6-8]. It is defined as a choicebased measurement approach that reconciles the need for question parsimony with the advantage of choice tasks that force individuals to make choices. BWS be a way to overcome some key shortcomings of common rating approaches (e.g. ties among items, response style bias, and standardization difficulties [9]. The BWS is superior to other methods, such as rating scales, because it forces respondents to discriminate between items, so results obtained from BWS are easy to interpret [10]. Unlike the rating scale, the BWS can also be used to compare both the intra and inter attributes. It builds on a body of items. The BWS approach effectively permits respondents to evaluate all pairwise combinations of alternatives presented in a particular subset leading to the assumption that their âBestâ and âWorstâ choices represent the maximum difference in utility between all attributes. A respondent gets presented a series of sets and is asked to choose one best item and one worst item in each set. BWS assumes everyone has a latent scale of âutilityâ or âimportanceâ, and the scale value of objects will determine a personâs choice over these objects. This scale value consists of two parts: one systematic part which can be understood as âintrinsicâ, and one random error component. Therefore, the probability of a certain project chosen as the best given other K options can be expressed as:
in which, and represent the scale value, the systematic component of the scale value and the error term respectively. McFadden further developed the conditional logit model under the independently and identical distribution assumption of the error terms, and the choice probability can be expressed as below [6,8].
Compared with other designs there are several advantages of BWS. It is suggested that choosing the âbestâ and the âworstâ from a set of options can generate relatively consistent results compared to the ranking task and it offers more information than simply choosing the âbestâ. Besides, when attributes under evaluation have multiple levels, BWS approach enables a comparison of all attribute levels on a single scale by setting only one level as reference [8,11].
BWS Design is to Estimates of Consumersâ Rank of Preferences
The BWS is widely used for collecting data to analyze the consumersâ preferences and becoming popular over time. It forces respondents to discriminate between scaled items and uses an underlying scale ratio of measurement [12]. This BWS method is consistent with consumer utility maximization. Based on this method, respondents are presented a set of items and they are demanded to indicate which one is the best and which is the worst [12]. For instance, the BWS was applied to investigate the relative importance consumers place on food values and to study preferences for sustainable farming practices [13]. Furthermore, efficient estimates can be obtained when repeated choices are made by the same respondent, which is the case in this present study [14]. Finally, the BWS method is gaining more popularity as a better alternative to the rating system and measuring value [15]. Therefore, the BWS method reported above fit well in this present study related to consumersâ preferences for quality and safety attributes of fresh vegetables. This is captured by the difference between the most preferred and least preferred items chosen from the set, when consumers are making a purchase decision. This can be mathematically represented as in this equation:
Where,
Uij is the utility for consumer i choosing vegetables having attribute, Vij and Δij are the deterministic component and the error term of utility, respectively.
BWS was used to determine values which consumers placed on quality and safety. Thus, the balanced incomplete block design (BIBD) method was used to design the questionnaire administered to respondents. In total 15 (fifteen) attributes of vegetables have been considered in the study (Table 1). Fifteen blocks or questions and eight attributes were randomly assigned to each. This questionnaire was used to collect data from Dhaka and Mymensingh City such as city market, shops, open vendor where vegetables are sold to consumers [16-19]. For each question are asked to choose which option of vegetables attributes they most preferred and which one they least preferred. A sample question for this study based on best-worst scaling method is presented in Table 2.
  Materials and Methods
The study was conducted in Dhaka and Mymensingh City of Bangladesh because of large number of urban populations, quality conscious consumers live in the cities thatâs why a considerable amount of the fresh vegetables are comes here from all over the country. The balanced incomplete block design technique was used to obtain a total 180 sample (100 Dhaka City consumers and 80 Mymensingh City). Survey Monkey software was used to collect by pre-determine structured questionnaire and interviewed in F2F due to lack of reached respondents by e-mail, phone and internet facilities of Bangladeshi people. Descriptive statistics, BWS/MaxDiff and multinomial mixed logit regression model were used to analyze the collected data.
  Results and Discussion
Demographics characteristics of survey consumers
The demographic profiles of respondents are illustrated in the Table 3. Among the total respondents about 63.50% were male and 36.50% were female. The ages were grouped into five categories and found that the highest (40.67%) age group under 30-40 years old and followed by 50-60 years old (25.34%), 40-50 years old (14.48%) and above 60 years old (2.25%) which is indicated most of the young respondents involved in household buying activities. As for matrials status about 83.89% were married, 12.22% were unmarried and 3.89% were unknown about the marital information as per survey questionnaire in the study areas. About 24.30% were employed in Government sectors, 57.30% were in private sectors, 7.50% were unemployed and 4.10% were retired. The result found that 5.21% of respondentsâ income was below BDT 20000 per month, 18.81% was in BDT 20000-40000, 25.46% was BDT 40000-60000, 25.46% was BDT 60000-80000, 19.82% was in BDT 80000-100000 and 12.4% was BDT 100000 and above, respectfully. The household size was 1-10 member with a relatively high share of respondent 37.78% were 4-5 persons per family, 25.00% was 2-3 persons per family, 22.78 % was 6-7 persons per family, 5.00% was 8-9 persons per family, 4.44% were about 10 persons and 0.56% were more than 10 persons per family and 4.44% was living alone. The Table 3 also showed that about 52.22% of the respondent had graduate education. This was followed by 20.00% had postgraduate, 17.78 % had higher secondary education, 6.67% had secondary education. This implies that most of the respondents attained certain level of formal education, which would facilitate understanding of improved selection method of fresh vegetable of the respondents more receptive to advisory services [20-25].
Multinomial Mixed Logit (MML) model Estimates of Vegetables Attribute
The Table 4 shows that parameter estimates from the MML model. The coefficients with positive sign indicate that attributes are preferred, while coefficients with negative signs indicate that attributes are not preferred by consumers. The results found that nutritive value, shelf life, availability, purity and safety are positive and statistically significant, implying that consumers preferred to have vegetables products with these attributes. It also shows that varieties, nutrient content, packaging and handling convenience were negative and statistically significant, indicating consumers significantly discounted vegetables products having these attributes and standard deviation regardless of location (Dhaka city or Mymensingh city) for nutrient content, color, shelf life, purity, safety, hygiene, availability, handling convenience, type of animals, origin, food miles and therapeutic value were statistically significant, implying that these parameters indeed randomly vary over the population.
Relative Important of Consumersâ Perceptions of on Fresh Vegetables
The relative importance of 15 (fifteen) attributes of vegetables is estimated by using MML model (Table 5). Researchers found that nutritive value, on average, is the most important vegetable attributes and significantly more important than hygiene, shelf life, availability, purity and safety are the next most important. Table 5 summarizes the results of simple count analysis. The relative importance of each variable is indicated by its B-W score, and the standard deviation suggests the degree of heterogeneity of consumersâ perceptions on fresh vegetable attributes for food safety. The result shows that âstandardizationâ âfreshnessâ âphysical appearanceâ and âtraceabilityâ are substantially more highly valued than the rest of the attributes, while âaudit during sales life as long as possibleâ and âcold chain with pesticide and chemical used when asked by consumersâ are underrated by most respondents. Most consumers are inclined to hold more positive attitudes towards âphysical appearance to traceabilityâ, âhormoneâ and âfertilizer residueâ, and understate the importance of âfertilizer residueâ and âpesticide residue costs with producersâ. Nevertheless, consumerâ perceptions on the importance of vegetable attributes, such as âhormoneâ, âfertilizer residueâ and âpesticide residue life as long as possibleâ tend to diverge as reflected by the large standard deviations of their B-W scores, which also suggests the need to further exp lore respondent heterogeneity [26-30].
BWS for Consumers Preferences
Table 6 showed that the average BWS scores with high scores corresponding to attributes that were like and the low scores corresponding to attributes that were disliked. The scores reflect some expected patterns which provide some evidences that our consumers appropriately read and interpreted the questionnaire. BWS scores also support quantitative comparison between levels within an attribute, and even across attributes something that is not possible with traditional likert-scale ratings. The Table 6 shows that the average best-worst scaling (BWS) score were -0.806 for food borne pathogens, -0.698 for heavy metals, -0.452 for pesticide residues, 0.415 for food additives, .060 for naturally occurring toxins and -0.340 for veterinary residues of food safety attributes. The average best-worst scaling (BWS) score of nutrition attributes were fat (0.040), calories (0.554), fiber (0.541), vitamins (0.253), sodium (-0.523), and mineral (0.323). Under the value attributes of fresh vegetables, the BWS scores were purity (-0.250), compositional integrity (0.216), appearance (-0.343), taste (0.251), convenience of preparation (-0.542) and size (-0.351). The average best-worst scaling (BWS) scores of production process attributes were genetic modification (-0.351), environmental impact (0.560) and pesticide use (0.140).
  Conclusion
BWS was used to identify the fresh vegetables attribute preference among the quality conscious consumers in the selected areas of Bangladesh. BIBD method was used to design the questionnaires and 180 consumers were purposively sampled and interviewed. The results found that nutritive value, shelf life, availability, safety and purity are the most important attributes, whereas verity, nutrient content, handling convenience and food miles are the least important attributes. This indicates that consumers place a high preference for nutritive value, shelf life, availability, safety and purity attributes of fresh vegetables. It implies that consumers are more concerned about vegetables with these attributes and this can significantly stimulate demand. The output also helps people along the value chain of vegetables to provide consumers with desirable attributes of vegetables, thereby enhance not only the profitability of their business, but also improve the overall welfare of consumers. The BWS technique, however, appropriate in ranking order of importance, had never been used in ranking choice of fresh vegetable purchase. Finally, the study created future direction for ranking of choice setting of consumer behavior analysis to researchers, academician and policymaker in context of Bangladesh as well as developing countries.
  Acknowledgment
The authors gratefully thank the anonymous reviewers of this article for helpful comments for further improvement. The authors also acknowledge the contributions of other study team who are involved in a team. All referees and the journal editor are gratefully appreciated for their valuable comments, consequent recommendation and suggestions on earlier drafts of this manuscript.
To know more about
Journal of Agriculture Research
-
https://juniperpublishers.com/artoaj/index.php
To know more about open access journal publishers click on Juniper publishers Â
0 notes
Text
Ways to Analyze Quantitative Market Research Data
Quantitative market research is the process of collecting large amounts of data through questionnaires, polling methods, and surveys. The market researcher is faced with a large amount of data once quantitative data have been collected. The quantitative market research gives the answers for âwhoâ and âwhat.â
A host of statistical analysis techniques are used by quantitative market research users.
Quantitative Market Research Data Analysis Techniques
Five techniques are commonly used for analyzing quantitative market research data:-
â      TURF Analysis
TURF (Total Unduplicated Reach and Frequency) analysis helps an organization to gain insights on a combination of products and services that will attract the highest number of customers. This is achieved by producing the reach and frequency of unduplicated data from the obtained responses. It analysis the number of customers reached by a particular communication source and the frequency
â      Conjoint Analysis
The method used to identify the value of various attributes such as features, benefits, and costs for the customers that lead to the purchase of a particular product or service is the conjoint analysis method. This analysis is widely used for product pricing, product launch, and marketing placement
A predetermined set of potential products or services is shown to respondents. By analyzing their preferences between these products, the implicit valuation of the individual elements making up the product or service can be determined. These implicit valuations are used to create market models that estimate revenue, market share and even profitability of new designs.
â      MaxDiff Analysis
This model is used to acquire customer preferences of multiple characteristics like brand images, product features, activities around the branding, etc. It is also known as âbest-worstâ scaling. It simply involves survey takers indicating the best and worst options out of a given set. Implemented within an approximate experimental design a relative ranking for each option can be obtained.
â      GAP Analysis
Calculation of the difference between the desired and actual performance of a particular product or service is done through GAP analysis. An organization can make improvements to mend the gap and make the attributes of products/ services more appealing to reduce the gap. Gap here refers to the space between âwhere we areâ and âwhere we want to beâ or the present state and the target state. it is also referred to as a needs analysis, needs assessment or needs gap analysis.
â      Cross tabulation
Comparison of two or more categories In a brief tabular format for convenient data analysis is done through cross-tabulation. It is a method to quantitatively analyze the relationship between multiple variables. Cross tabulation groups variables to understand the correlation between them. Changes of correlation from one variable grouping to another are also shown by this method. It is known by the names of contingency tables or cross tabs also.
Market Research Certification from MICA
To understand market research in more detail, a market research certification from MICA is recommended. Their Market Research and Data Analytics course is one of the best in India. It is a 12-month postgraduate certificate program that is conducted solely through virtual learning tools.
A market research certification from MICA would enable you to understand the correlation between market research and data analytics and provide a firm foundation for your career in the future.
0 notes
Text
max diff analysis
RELEVANT MAX-DIFF
 MaxDiff is a survey research approach for obtaining preference/importance scores for multiple items (brand preferences, brand images, product features, advertising claims, etc.).
 Sometimes researchers want to show only the MaxDiff items that are relevant to a given respondent. Â
 Relevant max diff analysis let us to show a customized and dynamic list for each respondent based on a variety of conditions and logic.  This means  we can customize the MaxDiff items (potentially a different number of items per respondent) to show respondents based on relevance, awareness, importance, preference, etc.
 Example link:
 https://knowledgeexcelsurvey.com/relevantMaxdiff/cgi-bin/ciwweb.pl?studyname=relevantMaxdiff
 Analysis can involve logit, latent class, or HB and you need to specify in the analysis interface how to treat missing items for the respondent.  There are three methods of treating missing items:
 1.Missing items are missing at random and inferred from the population information (default method),
2.Missing items are inferior to included items,
3.Missing items are unavailable and should have near-zero probability of choice for a respondent (we insert a large negative utility such as -20 for the missing utility).
 All three methods lead to a full set of utility scores for all the items (for the group, each latent class, or the respondent if using HB estimation).  If using HB estimation, you can also export the scores with missing items per respondent replaced with blanks, such that the missing items have missing utility scores.
 There are two ways to create the combinations of items to show respondents (the design) for MaxDiff questionnaires: Pre-generated and On-the-fly.
On-The-Fly Design Generation
On-the-fly (during survey) MaxDiff designs contain the combinations of items to show each respondent and are generated in the moment that the respondent takes the survey. Â On-the-fly designs are required when the number of items in the constructed (dynamic) MaxDiff list is different across respondents for the same MaxDiff exercise.
We should take special care that respondents always get a reasonable number of items in their consideration list. Â If too many items are included, each item might not be shown enough times for stable HB estimation. Â If too few items are included, there may be too few items to populate even one MaxDiff question and the entire MaxDiff exercise would be skipped for the respondent. We should ensure that our consideration set has a minimum number of items per respondent.
Researchers also recommend to add a few items to the consideration set that are not relevant/important to the respondent. Â However, given the method we use for augmenting each respondent's choice data with full information when missing items are treated as inferior, this may not be necessary
 Pre-Generated Designs
When the number of items to show each respondent is the same, we can generate the combinations of items to show respondents prior to data collection. This is called a Pre-Generated design. The default is to generate 300 versions (blocks) of the design prior to fielding and respondents are assigned to different versions (often times with multiple respondents receiving the same versions, which is just fine from a statistical standpoint). Â
There are at least two MaxDiff approaches that involve customizing a subset of items to show each respondent that could involve pre-generated designs, because the subset of items always has the same constructed list length: Express MaxDiff and Bandit MaxDiff. Â For example, 30 items could be drawn from a master list of 100 items for each respondent to evaluate, so a pre-generated design accommodating 30 item slots could be employed. Â We should note, however, that prohibitions may not be specified if using pre-generated designs for Express MaxDiff or Bandit MaxDiff. Â Rather, on-the-fly design may be used with Bandit and Express MaxDiff for implementing prohibitions between items.
Visit this website - https://knowledgeexcel.com/maxdiff/
1 note
·
View note