#performance tuning SQL
Explore tagged Tumblr posts
Text
Embracing Efficiency: T-SQL in Azure SQL Database and Elastic Pools
In the dynamic world of cloud computing, managing databases effectively is paramount. Microsoft Azure offers two compelling options for this purpose: Azure SQL Database and Azure SQL Elastic Pools. Both provide robust platforms for leveraging Transact-SQL (T-SQL), a foundational skill for database professionals. This article delves into the practical applications of T-SQL within these…
View On WordPress
#Azure Elastic Pools#Azure SQL Database#cloud database management#performance tuning SQL#T-SQL examples
0 notes
Text
The Skills I Acquired on My Path to Becoming a Data Scientist
Data science has emerged as one of the most sought-after fields in recent years, and my journey into this exciting discipline has been nothing short of transformative. As someone with a deep curiosity for extracting insights from data, I was naturally drawn to the world of data science. In this blog post, I will share the skills I acquired on my path to becoming a data scientist, highlighting the importance of a diverse skill set in this field.
The Foundation — Mathematics and Statistics
At the core of data science lies a strong foundation in mathematics and statistics. Concepts such as probability, linear algebra, and statistical inference form the building blocks of data analysis and modeling. Understanding these principles is crucial for making informed decisions and drawing meaningful conclusions from data. Throughout my learning journey, I immersed myself in these mathematical concepts, applying them to real-world problems and honing my analytical skills.
Programming Proficiency
Proficiency in programming languages like Python or R is indispensable for a data scientist. These languages provide the tools and frameworks necessary for data manipulation, analysis, and modeling. I embarked on a journey to learn these languages, starting with the basics and gradually advancing to more complex concepts. Writing efficient and elegant code became second nature to me, enabling me to tackle large datasets and build sophisticated models.
Data Handling and Preprocessing
Working with real-world data is often messy and requires careful handling and preprocessing. This involves techniques such as data cleaning, transformation, and feature engineering. I gained valuable experience in navigating the intricacies of data preprocessing, learning how to deal with missing values, outliers, and inconsistent data formats. These skills allowed me to extract valuable insights from raw data and lay the groundwork for subsequent analysis.
Data Visualization and Communication
Data visualization plays a pivotal role in conveying insights to stakeholders and decision-makers. I realized the power of effective visualizations in telling compelling stories and making complex information accessible. I explored various tools and libraries, such as Matplotlib and Tableau, to create visually appealing and informative visualizations. Sharing these visualizations with others enhanced my ability to communicate data-driven insights effectively.
Machine Learning and Predictive Modeling
Machine learning is a cornerstone of data science, enabling us to build predictive models and make data-driven predictions. I delved into the realm of supervised and unsupervised learning, exploring algorithms such as linear regression, decision trees, and clustering techniques. Through hands-on projects, I gained practical experience in building models, fine-tuning their parameters, and evaluating their performance.
Database Management and SQL
Data science often involves working with large datasets stored in databases. Understanding database management and SQL (Structured Query Language) is essential for extracting valuable information from these repositories. I embarked on a journey to learn SQL, mastering the art of querying databases, joining tables, and aggregating data. These skills allowed me to harness the power of databases and efficiently retrieve the data required for analysis.
Domain Knowledge and Specialization
While technical skills are crucial, domain knowledge adds a unique dimension to data science projects. By specializing in specific industries or domains, data scientists can better understand the context and nuances of the problems they are solving. I explored various domains and acquired specialized knowledge, whether it be healthcare, finance, or marketing. This expertise complemented my technical skills, enabling me to provide insights that were not only data-driven but also tailored to the specific industry.
Soft Skills — Communication and Problem-Solving
In addition to technical skills, soft skills play a vital role in the success of a data scientist. Effective communication allows us to articulate complex ideas and findings to non-technical stakeholders, bridging the gap between data science and business. Problem-solving skills help us navigate challenges and find innovative solutions in a rapidly evolving field. Throughout my journey, I honed these skills, collaborating with teams, presenting findings, and adapting my approach to different audiences.
Continuous Learning and Adaptation
Data science is a field that is constantly evolving, with new tools, technologies, and trends emerging regularly. To stay at the forefront of this ever-changing landscape, continuous learning is essential. I dedicated myself to staying updated by following industry blogs, attending conferences, and participating in courses. This commitment to lifelong learning allowed me to adapt to new challenges, acquire new skills, and remain competitive in the field.
In conclusion, the journey to becoming a data scientist is an exciting and dynamic one, requiring a diverse set of skills. From mathematics and programming to data handling and communication, each skill plays a crucial role in unlocking the potential of data. Aspiring data scientists should embrace this multidimensional nature of the field and embark on their own learning journey. If you want to learn more about Data science, I highly recommend that you contact ACTE Technologies because they offer Data Science courses and job placement opportunities. Experienced teachers can help you learn better. You can find these services both online and offline. Take things step by step and consider enrolling in a course if you’re interested. By acquiring these skills and continuously adapting to new developments, they can make a meaningful impact in the world of data science.
#data science#data visualization#education#information#technology#machine learning#database#sql#predictive analytics#r programming#python#big data#statistics
14 notes
·
View notes
Text
UNLOCKING THE POWER OF AI WITH EASYLIBPAL 2/2
EXPANDED COMPONENTS AND DETAILS OF EASYLIBPAL:
1. Easylibpal Class: The core component of the library, responsible for handling algorithm selection, model fitting, and prediction generation
2. Algorithm Selection and Support:
Supports classic AI algorithms such as Linear Regression, Logistic Regression, Support Vector Machine (SVM), Naive Bayes, and K-Nearest Neighbors (K-NN).
and
- Decision Trees
- Random Forest
- AdaBoost
- Gradient Boosting
3. Integration with Popular Libraries: Seamless integration with essential Python libraries like NumPy, Pandas, Matplotlib, and Scikit-learn for enhanced functionality.
4. Data Handling:
- DataLoader class for importing and preprocessing data from various formats (CSV, JSON, SQL databases).
- DataTransformer class for feature scaling, normalization, and encoding categorical variables.
- Includes functions for loading and preprocessing datasets to prepare them for training and testing.
- `FeatureSelector` class: Provides methods for feature selection and dimensionality reduction.
5. Model Evaluation:
- Evaluator class to assess model performance using metrics like accuracy, precision, recall, F1-score, and ROC-AUC.
- Methods for generating confusion matrices and classification reports.
6. Model Training: Contains methods for fitting the selected algorithm with the training data.
- `fit` method: Trains the selected algorithm on the provided training data.
7. Prediction Generation: Allows users to make predictions using the trained model on new data.
- `predict` method: Makes predictions using the trained model on new data.
- `predict_proba` method: Returns the predicted probabilities for classification tasks.
8. Model Evaluation:
- `Evaluator` class: Assesses model performance using various metrics (e.g., accuracy, precision, recall, F1-score, ROC-AUC).
- `cross_validate` method: Performs cross-validation to evaluate the model's performance.
- `confusion_matrix` method: Generates a confusion matrix for classification tasks.
- `classification_report` method: Provides a detailed classification report.
9. Hyperparameter Tuning:
- Tuner class that uses techniques likes Grid Search and Random Search for hyperparameter optimization.
10. Visualization:
- Integration with Matplotlib and Seaborn for generating plots to analyze model performance and data characteristics.
- Visualization support: Enables users to visualize data, model performance, and predictions using plotting functionalities.
- `Visualizer` class: Integrates with Matplotlib and Seaborn to generate plots for model performance analysis and data visualization.
- `plot_confusion_matrix` method: Visualizes the confusion matrix.
- `plot_roc_curve` method: Plots the Receiver Operating Characteristic (ROC) curve.
- `plot_feature_importance` method: Visualizes feature importance for applicable algorithms.
11. Utility Functions:
- Functions for saving and loading trained models.
- Logging functionalities to track the model training and prediction processes.
- `save_model` method: Saves the trained model to a file.
- `load_model` method: Loads a previously trained model from a file.
- `set_logger` method: Configures logging functionality for tracking model training and prediction processes.
12. User-Friendly Interface: Provides a simplified and intuitive interface for users to interact with and apply classic AI algorithms without extensive knowledge or configuration.
13.. Error Handling: Incorporates mechanisms to handle invalid inputs, errors during training, and other potential issues during algorithm usage.
- Custom exception classes for handling specific errors and providing informative error messages to users.
14. Documentation: Comprehensive documentation to guide users on how to use Easylibpal effectively and efficiently
- Comprehensive documentation explaining the usage and functionality of each component.
- Example scripts demonstrating how to use Easylibpal for various AI tasks and datasets.
15. Testing Suite:
- Unit tests for each component to ensure code reliability and maintainability.
- Integration tests to verify the smooth interaction between different components.
IMPLEMENTATION EXAMPLE WITH ADDITIONAL FEATURES:
Here is an example of how the expanded Easylibpal library could be structured and used:
```python
import numpy as np
import pandas as pd
from sklearn.model_selection import train_test_split
from sklearn.preprocessing import StandardScaler
from easylibpal import Easylibpal, DataLoader, Evaluator, Tuner
# Example DataLoader
class DataLoader:
def load_data(self, filepath, file_type='csv'):
if file_type == 'csv':
return pd.read_csv(filepath)
else:
raise ValueError("Unsupported file type provided.")
# Example Evaluator
class Evaluator:
def evaluate(self, model, X_test, y_test):
predictions = model.predict(X_test)
accuracy = np.mean(predictions == y_test)
return {'accuracy': accuracy}
# Example usage of Easylibpal with DataLoader and Evaluator
if __name__ == "__main__":
# Load and prepare the data
data_loader = DataLoader()
data = data_loader.load_data('path/to/your/data.csv')
X = data.iloc[:, :-1]
y = data.iloc[:, -1]
X_train, X_test, y_train, y_test = train_test_split(X, y, test_size=0.2, random_state=42)
# Scale features
scaler = StandardScaler()
X_train_scaled = scaler.fit_transform(X_train)
X_test_scaled = scaler.transform(X_test)
# Initialize Easylibpal with the desired algorithm
model = Easylibpal('Random Forest')
model.fit(X_train_scaled, y_train)
# Evaluate the model
evaluator = Evaluator()
results = evaluator.evaluate(model, X_test_scaled, y_test)
print(f"Model Accuracy: {results['accuracy']}")
# Optional: Use Tuner for hyperparameter optimization
tuner = Tuner(model, param_grid={'n_estimators': [100, 200], 'max_depth': [10, 20, 30]})
best_params = tuner.optimize(X_train_scaled, y_train)
print(f"Best Parameters: {best_params}")
```
This example demonstrates the structured approach to using Easylibpal with enhanced data handling, model evaluation, and optional hyperparameter tuning. The library empowers users to handle real-world datasets, apply various machine learning algorithms, and evaluate their performance with ease, making it an invaluable tool for developers and data scientists aiming to implement AI solutions efficiently.
Easylibpal is dedicated to making the latest AI technology accessible to everyone, regardless of their background or expertise. Our platform simplifies the process of selecting and implementing classic AI algorithms, enabling users across various industries to harness the power of artificial intelligence with ease. By democratizing access to AI, we aim to accelerate innovation and empower users to achieve their goals with confidence. Easylibpal's approach involves a democratization framework that reduces entry barriers, lowers the cost of building AI solutions, and speeds up the adoption of AI in both academic and business settings.
Below are examples showcasing how each main component of the Easylibpal library could be implemented and used in practice to provide a user-friendly interface for utilizing classic AI algorithms.
1. Core Components
Easylibpal Class Example:
```python
class Easylibpal:
def __init__(self, algorithm):
self.algorithm = algorithm
self.model = None
def fit(self, X, y):
# Simplified example: Instantiate and train a model based on the selected algorithm
if self.algorithm == 'Linear Regression':
from sklearn.linear_model import LinearRegression
self.model = LinearRegression()
elif self.algorithm == 'Random Forest':
from sklearn.ensemble import RandomForestClassifier
self.model = RandomForestClassifier()
self.model.fit(X, y)
def predict(self, X):
return self.model.predict(X)
```
2. Data Handling
DataLoader Class Example:
```python
class DataLoader:
def load_data(self, filepath, file_type='csv'):
if file_type == 'csv':
import pandas as pd
return pd.read_csv(filepath)
else:
raise ValueError("Unsupported file type provided.")
```
3. Model Evaluation
Evaluator Class Example:
```python
from sklearn.metrics import accuracy_score, classification_report
class Evaluator:
def evaluate(self, model, X_test, y_test):
predictions = model.predict(X_test)
accuracy = accuracy_score(y_test, predictions)
report = classification_report(y_test, predictions)
return {'accuracy': accuracy, 'report': report}
```
4. Hyperparameter Tuning
Tuner Class Example:
```python
from sklearn.model_selection import GridSearchCV
class Tuner:
def __init__(self, model, param_grid):
self.model = model
self.param_grid = param_grid
def optimize(self, X, y):
grid_search = GridSearchCV(self.model, self.param_grid, cv=5)
grid_search.fit(X, y)
return grid_search.best_params_
```
5. Visualization
Visualizer Class Example:
```python
import matplotlib.pyplot as plt
class Visualizer:
def plot_confusion_matrix(self, cm, classes, normalize=False, title='Confusion matrix'):
plt.imshow(cm, interpolation='nearest', cmap=plt.cm.Blues)
plt.title(title)
plt.colorbar()
tick_marks = np.arange(len(classes))
plt.xticks(tick_marks, classes, rotation=45)
plt.yticks(tick_marks, classes)
plt.ylabel('True label')
plt.xlabel('Predicted label')
plt.show()
```
6. Utility Functions
Save and Load Model Example:
```python
import joblib
def save_model(model, filename):
joblib.dump(model, filename)
def load_model(filename):
return joblib.load(filename)
```
7. Example Usage Script
Using Easylibpal in a Script:
```python
# Assuming Easylibpal and other classes have been imported
data_loader = DataLoader()
data = data_loader.load_data('data.csv')
X = data.drop('Target', axis=1)
y = data['Target']
model = Easylibpal('Random Forest')
model.fit(X, y)
evaluator = Evaluator()
results = evaluator.evaluate(model, X, y)
print("Accuracy:", results['accuracy'])
print("Report:", results['report'])
visualizer = Visualizer()
visualizer.plot_confusion_matrix(results['cm'], classes=['Class1', 'Class2'])
save_model(model, 'trained_model.pkl')
loaded_model = load_model('trained_model.pkl')
```
These examples illustrate the practical implementation and use of the Easylibpal library components, aiming to simplify the application of AI algorithms for users with varying levels of expertise in machine learning.
EASYLIBPAL IMPLEMENTATION:
Step 1: Define the Problem
First, we need to define the problem we want to solve. For this POC, let's assume we want to predict house prices based on various features like the number of bedrooms, square footage, and location.
Step 2: Choose an Appropriate Algorithm
Given our problem, a supervised learning algorithm like linear regression would be suitable. We'll use Scikit-learn, a popular library for machine learning in Python, to implement this algorithm.
Step 3: Prepare Your Data
We'll use Pandas to load and prepare our dataset. This involves cleaning the data, handling missing values, and splitting the dataset into training and testing sets.
Step 4: Implement the Algorithm
Now, we'll use Scikit-learn to implement the linear regression algorithm. We'll train the model on our training data and then test its performance on the testing data.
Step 5: Evaluate the Model
Finally, we'll evaluate the performance of our model using metrics like Mean Squared Error (MSE) and R-squared.
Python Code POC
```python
import numpy as np
import pandas as pd
from sklearn.model_selection import train_test_split
from sklearn.linear_model import LinearRegression
from sklearn.metrics import mean_squared_error, r2_score
# Load the dataset
data = pd.read_csv('house_prices.csv')
# Prepare the data
X = data'bedrooms', 'square_footage', 'location'
y = data['price']
# Split the data into training and testing sets
X_train, X_test, y_train, y_test = train_test_split(X, y, test_size=0.2, random_state=42)
# Create and train the model
model = LinearRegression()
model.fit(X_train, y_train)
# Make predictions
predictions = model.predict(X_test)
# Evaluate the model
mse = mean_squared_error(y_test, predictions)
r2 = r2_score(y_test, predictions)
print(f'Mean Squared Error: {mse}')
print(f'R-squared: {r2}')
```
Below is an implementation, Easylibpal provides a simple interface to instantiate and utilize classic AI algorithms such as Linear Regression, Logistic Regression, SVM, Naive Bayes, and K-NN. Users can easily create an instance of Easylibpal with their desired algorithm, fit the model with training data, and make predictions, all with minimal code and hassle. This demonstrates the power of Easylibpal in simplifying the integration of AI algorithms for various tasks.
```python
# Import necessary libraries
import numpy as np
import pandas as pd
import matplotlib.pyplot as plt
from sklearn.linear_model import LinearRegression
from sklearn.linear_model import LogisticRegression
from sklearn.svm import SVC
from sklearn.naive_bayes import GaussianNB
from sklearn.neighbors import KNeighborsClassifier
class Easylibpal:
def __init__(self, algorithm):
self.algorithm = algorithm
def fit(self, X, y):
if self.algorithm == 'Linear Regression':
self.model = LinearRegression()
elif self.algorithm == 'Logistic Regression':
self.model = LogisticRegression()
elif self.algorithm == 'SVM':
self.model = SVC()
elif self.algorithm == 'Naive Bayes':
self.model = GaussianNB()
elif self.algorithm == 'K-NN':
self.model = KNeighborsClassifier()
else:
raise ValueError("Invalid algorithm specified.")
self.model.fit(X, y)
def predict(self, X):
return self.model.predict(X)
# Example usage:
# Initialize Easylibpal with the desired algorithm
easy_algo = Easylibpal('Linear Regression')
# Generate some sample data
X = np.array([[1], [2], [3], [4]])
y = np.array([2, 4, 6, 8])
# Fit the model
easy_algo.fit(X, y)
# Make predictions
predictions = easy_algo.predict(X)
# Plot the results
plt.scatter(X, y)
plt.plot(X, predictions, color='red')
plt.title('Linear Regression with Easylibpal')
plt.xlabel('X')
plt.ylabel('y')
plt.show()
```
Easylibpal is an innovative Python library designed to simplify the integration and use of classic AI algorithms in a user-friendly manner. It aims to bridge the gap between the complexity of AI libraries and the ease of use, making it accessible for developers and data scientists alike. Easylibpal abstracts the underlying complexity of each algorithm, providing a unified interface that allows users to apply these algorithms with minimal configuration and understanding of the underlying mechanisms.
ENHANCED DATASET HANDLING
Easylibpal should be able to handle datasets more efficiently. This includes loading datasets from various sources (e.g., CSV files, databases), preprocessing data (e.g., normalization, handling missing values), and splitting data into training and testing sets.
```python
import os
from sklearn.model_selection import train_test_split
class Easylibpal:
# Existing code...
def load_dataset(self, filepath):
"""Loads a dataset from a CSV file."""
if not os.path.exists(filepath):
raise FileNotFoundError("Dataset file not found.")
return pd.read_csv(filepath)
def preprocess_data(self, dataset):
"""Preprocesses the dataset."""
# Implement data preprocessing steps here
return dataset
def split_data(self, X, y, test_size=0.2):
"""Splits the dataset into training and testing sets."""
return train_test_split(X, y, test_size=test_size)
```
Additional Algorithms
Easylibpal should support a wider range of algorithms. This includes decision trees, random forests, and gradient boosting machines.
```python
from sklearn.tree import DecisionTreeClassifier
from sklearn.ensemble import RandomForestClassifier
from sklearn.ensemble import GradientBoostingClassifier
class Easylibpal:
# Existing code...
def fit(self, X, y):
# Existing code...
elif self.algorithm == 'Decision Tree':
self.model = DecisionTreeClassifier()
elif self.algorithm == 'Random Forest':
self.model = RandomForestClassifier()
elif self.algorithm == 'Gradient Boosting':
self.model = GradientBoostingClassifier()
# Add more algorithms as needed
```
User-Friendly Features
To make Easylibpal even more user-friendly, consider adding features like:
- Automatic hyperparameter tuning: Implementing a simple interface for hyperparameter tuning using GridSearchCV or RandomizedSearchCV.
- Model evaluation metrics: Providing easy access to common evaluation metrics like accuracy, precision, recall, and F1 score.
- Visualization tools: Adding methods for plotting model performance, confusion matrices, and feature importance.
```python
from sklearn.metrics import accuracy_score, classification_report
from sklearn.model_selection import GridSearchCV
class Easylibpal:
# Existing code...
def evaluate_model(self, X_test, y_test):
"""Evaluates the model using accuracy and classification report."""
y_pred = self.predict(X_test)
print("Accuracy:", accuracy_score(y_test, y_pred))
print(classification_report(y_test, y_pred))
def tune_hyperparameters(self, X, y, param_grid):
"""Tunes the model's hyperparameters using GridSearchCV."""
grid_search = GridSearchCV(self.model, param_grid, cv=5)
grid_search.fit(X, y)
self.model = grid_search.best_estimator_
```
Easylibpal leverages the power of Python and its rich ecosystem of AI and machine learning libraries, such as scikit-learn, to implement the classic algorithms. It provides a high-level API that abstracts the specifics of each algorithm, allowing users to focus on the problem at hand rather than the intricacies of the algorithm.
Python Code Snippets for Easylibpal
Below are Python code snippets demonstrating the use of Easylibpal with classic AI algorithms. Each snippet demonstrates how to use Easylibpal to apply a specific algorithm to a dataset.
# Linear Regression
```python
from Easylibpal import Easylibpal
# Initialize Easylibpal with a dataset
Easylibpal = Easylibpal(dataset='your_dataset.csv')
# Apply Linear Regression
result = Easylibpal.apply_algorithm('linear_regression', target_column='target')
# Print the result
print(result)
```
# Logistic Regression
```python
from Easylibpal import Easylibpal
# Initialize Easylibpal with a dataset
Easylibpal = Easylibpal(dataset='your_dataset.csv')
# Apply Logistic Regression
result = Easylibpal.apply_algorithm('logistic_regression', target_column='target')
# Print the result
print(result)
```
# Support Vector Machines (SVM)
```python
from Easylibpal import Easylibpal
# Initialize Easylibpal with a dataset
Easylibpal = Easylibpal(dataset='your_dataset.csv')
# Apply SVM
result = Easylibpal.apply_algorithm('svm', target_column='target')
# Print the result
print(result)
```
# Naive Bayes
```python
from Easylibpal import Easylibpal
# Initialize Easylibpal with a dataset
Easylibpal = Easylibpal(dataset='your_dataset.csv')
# Apply Naive Bayes
result = Easylibpal.apply_algorithm('naive_bayes', target_column='target')
# Print the result
print(result)
```
# K-Nearest Neighbors (K-NN)
```python
from Easylibpal import Easylibpal
# Initialize Easylibpal with a dataset
Easylibpal = Easylibpal(dataset='your_dataset.csv')
# Apply K-NN
result = Easylibpal.apply_algorithm('knn', target_column='target')
# Print the result
print(result)
```
ABSTRACTION AND ESSENTIAL COMPLEXITY
- Essential Complexity: This refers to the inherent complexity of the problem domain, which cannot be reduced regardless of the programming language or framework used. It includes the logic and algorithm needed to solve the problem. For example, the essential complexity of sorting a list remains the same across different programming languages.
- Accidental Complexity: This is the complexity introduced by the choice of programming language, framework, or libraries. It can be reduced or eliminated through abstraction. For instance, using a high-level API in Python can hide the complexity of lower-level operations, making the code more readable and maintainable.
HOW EASYLIBPAL ABSTRACTS COMPLEXITY
Easylibpal aims to reduce accidental complexity by providing a high-level API that encapsulates the details of each classic AI algorithm. This abstraction allows users to apply these algorithms without needing to understand the underlying mechanisms or the specifics of the algorithm's implementation.
- Simplified Interface: Easylibpal offers a unified interface for applying various algorithms, such as Linear Regression, Logistic Regression, SVM, Naive Bayes, and K-NN. This interface abstracts the complexity of each algorithm, making it easier for users to apply them to their datasets.
- Runtime Fusion: By evaluating sub-expressions and sharing them across multiple terms, Easylibpal can optimize the execution of algorithms. This approach, similar to runtime fusion in abstract algorithms, allows for efficient computation without duplicating work, thereby reducing the computational complexity.
- Focus on Essential Complexity: While Easylibpal abstracts away the accidental complexity; it ensures that the essential complexity of the problem domain remains at the forefront. This means that while the implementation details are hidden, the core logic and algorithmic approach are still accessible and understandable to the user.
To implement Easylibpal, one would need to create a Python class that encapsulates the functionality of each classic AI algorithm. This class would provide methods for loading datasets, preprocessing data, and applying the algorithm with minimal configuration required from the user. The implementation would leverage existing libraries like scikit-learn for the actual algorithmic computations, abstracting away the complexity of these libraries.
Here's a conceptual example of how the Easylibpal class might be structured for applying a Linear Regression algorithm:
```python
class Easylibpal:
def __init__(self, dataset):
self.dataset = dataset
# Load and preprocess the dataset
def apply_linear_regression(self, target_column):
# Abstracted implementation of Linear Regression
# This method would internally use scikit-learn or another library
# to perform the actual computation, abstracting the complexity
pass
# Usage
Easylibpal = Easylibpal(dataset='your_dataset.csv')
result = Easylibpal.apply_linear_regression(target_column='target')
```
This example demonstrates the concept of Easylibpal by abstracting the complexity of applying a Linear Regression algorithm. The actual implementation would need to include the specifics of loading the dataset, preprocessing it, and applying the algorithm using an underlying library like scikit-learn.
Easylibpal abstracts the complexity of classic AI algorithms by providing a simplified interface that hides the intricacies of each algorithm's implementation. This abstraction allows users to apply these algorithms with minimal configuration and understanding of the underlying mechanisms. Here are examples of specific algorithms that Easylibpal abstracts:
To implement Easylibpal, one would need to create a Python class that encapsulates the functionality of each classic AI algorithm. This class would provide methods for loading datasets, preprocessing data, and applying the algorithm with minimal configuration required from the user. The implementation would leverage existing libraries like scikit-learn for the actual algorithmic computations, abstracting away the complexity of these libraries.
Here's a conceptual example of how the Easylibpal class might be structured for applying a Linear Regression algorithm:
```python
class Easylibpal:
def __init__(self, dataset):
self.dataset = dataset
# Load and preprocess the dataset
def apply_linear_regression(self, target_column):
# Abstracted implementation of Linear Regression
# This method would internally use scikit-learn or another library
# to perform the actual computation, abstracting the complexity
pass
# Usage
Easylibpal = Easylibpal(dataset='your_dataset.csv')
result = Easylibpal.apply_linear_regression(target_column='target')
```
This example demonstrates the concept of Easylibpal by abstracting the complexity of applying a Linear Regression algorithm. The actual implementation would need to include the specifics of loading the dataset, preprocessing it, and applying the algorithm using an underlying library like scikit-learn.
Easylibpal abstracts the complexity of feature selection for classic AI algorithms by providing a simplified interface that automates the process of selecting the most relevant features for each algorithm. This abstraction is crucial because feature selection is a critical step in machine learning that can significantly impact the performance of a model. Here's how Easylibpal handles feature selection for the mentioned algorithms:
To implement feature selection in Easylibpal, one could use scikit-learn's `SelectKBest` or `RFE` classes for feature selection based on statistical tests or model coefficients. Here's a conceptual example of how feature selection might be integrated into the Easylibpal class for Linear Regression:
```python
from sklearn.feature_selection import SelectKBest, f_regression
from sklearn.linear_model import LinearRegression
class Easylibpal:
def __init__(self, dataset):
self.dataset = dataset
# Load and preprocess the dataset
def apply_linear_regression(self, target_column):
# Feature selection using SelectKBest
selector = SelectKBest(score_func=f_regression, k=10)
X_new = selector.fit_transform(self.dataset.drop(target_column, axis=1), self.dataset[target_column])
# Train Linear Regression model
model = LinearRegression()
model.fit(X_new, self.dataset[target_column])
# Return the trained model
return model
# Usage
Easylibpal = Easylibpal(dataset='your_dataset.csv')
model = Easylibpal.apply_linear_regression(target_column='target')
```
This example demonstrates how Easylibpal abstracts the complexity of feature selection for Linear Regression by using scikit-learn's `SelectKBest` to select the top 10 features based on their statistical significance in predicting the target variable. The actual implementation would need to adapt this approach for each algorithm, considering the specific characteristics and requirements of each algorithm.
To implement feature selection in Easylibpal, one could use scikit-learn's `SelectKBest`, `RFE`, or other feature selection classes based on the algorithm's requirements. Here's a conceptual example of how feature selection might be integrated into the Easylibpal class for Logistic Regression using RFE:
```python
from sklearn.feature_selection import RFE
from sklearn.linear_model import LogisticRegression
class Easylibpal:
def __init__(self, dataset):
self.dataset = dataset
# Load and preprocess the dataset
def apply_logistic_regression(self, target_column):
# Feature selection using RFE
model = LogisticRegression()
rfe = RFE(model, n_features_to_select=10)
rfe.fit(self.dataset.drop(target_column, axis=1), self.dataset[target_column])
# Train Logistic Regression model
model.fit(self.dataset.drop(target_column, axis=1), self.dataset[target_column])
# Return the trained model
return model
# Usage
Easylibpal = Easylibpal(dataset='your_dataset.csv')
model = Easylibpal.apply_logistic_regression(target_column='target')
```
This example demonstrates how Easylibpal abstracts the complexity of feature selection for Logistic Regression by using scikit-learn's `RFE` to select the top 10 features based on their importance in the model. The actual implementation would need to adapt this approach for each algorithm, considering the specific characteristics and requirements of each algorithm.
EASYLIBPAL HANDLES DIFFERENT TYPES OF DATASETS
Easylibpal handles different types of datasets with varying structures by adopting a flexible and adaptable approach to data preprocessing and transformation. This approach is inspired by the principles of tidy data and the need to ensure data is in a consistent, usable format before applying AI algorithms. Here's how Easylibpal addresses the challenges posed by varying dataset structures:
One Type in Multiple Tables
When datasets contain different variables, the same variables with different names, different file formats, or different conventions for missing values, Easylibpal employs a process similar to tidying data. This involves identifying and standardizing the structure of each dataset, ensuring that each variable is consistently named and formatted across datasets. This process might include renaming columns, converting data types, and handling missing values in a uniform manner. For datasets stored in different file formats, Easylibpal would use appropriate libraries (e.g., pandas for CSV, Excel files, and SQL databases) to load and preprocess the data before applying the algorithms.
Multiple Types in One Table
For datasets that involve values collected at multiple levels or on different types of observational units, Easylibpal applies a normalization process. This involves breaking down the dataset into multiple tables, each representing a distinct type of observational unit. For example, if a dataset contains information about songs and their rankings over time, Easylibpal would separate this into two tables: one for song details and another for rankings. This normalization ensures that each fact is expressed in only one place, reducing inconsistencies and making the data more manageable for analysis.
Data Semantics
Easylibpal ensures that the data is organized in a way that aligns with the principles of data semantics, where every value belongs to a variable and an observation. This organization is crucial for the algorithms to interpret the data correctly. Easylibpal might use functions like `pivot_longer` and `pivot_wider` from the tidyverse or equivalent functions in pandas to reshape the data into a long format, where each row represents a single observation and each column represents a single variable. This format is particularly useful for algorithms that require a consistent structure for input data.
Messy Data
Dealing with messy data, which can include inconsistent data types, missing values, and outliers, is a common challenge in data science. Easylibpal addresses this by implementing robust data cleaning and preprocessing steps. This includes handling missing values (e.g., imputation or deletion), converting data types to ensure consistency, and identifying and removing outliers. These steps are crucial for preparing the data in a format that is suitable for the algorithms, ensuring that the algorithms can effectively learn from the data without being hindered by its inconsistencies.
To implement these principles in Python, Easylibpal would leverage libraries like pandas for data manipulation and preprocessing. Here's a conceptual example of how Easylibpal might handle a dataset with multiple types in one table:
```python
import pandas as pd
# Load the dataset
dataset = pd.read_csv('your_dataset.csv')
# Normalize the dataset by separating it into two tables
song_table = dataset'artist', 'track'.drop_duplicates().reset_index(drop=True)
song_table['song_id'] = range(1, len(song_table) + 1)
ranking_table = dataset'artist', 'track', 'week', 'rank'.drop_duplicates().reset_index(drop=True)
# Now, song_table and ranking_table can be used separately for analysis
```
This example demonstrates how Easylibpal might normalize a dataset with multiple types of observational units into separate tables, ensuring that each type of observational unit is stored in its own table. The actual implementation would need to adapt this approach based on the specific structure and requirements of the dataset being processed.
CLEAN DATA
Easylibpal employs a comprehensive set of data cleaning and preprocessing steps to handle messy data, ensuring that the data is in a suitable format for machine learning algorithms. These steps are crucial for improving the accuracy and reliability of the models, as well as preventing misleading results and conclusions. Here's a detailed look at the specific steps Easylibpal might employ:
1. Remove Irrelevant Data
The first step involves identifying and removing data that is not relevant to the analysis or modeling task at hand. This could include columns or rows that do not contribute to the predictive power of the model or are not necessary for the analysis .
2. Deduplicate Data
Deduplication is the process of removing duplicate entries from the dataset. Duplicates can skew the analysis and lead to incorrect conclusions. Easylibpal would use appropriate methods to identify and remove duplicates, ensuring that each entry in the dataset is unique.
3. Fix Structural Errors
Structural errors in the dataset, such as inconsistent data types, incorrect values, or formatting issues, can significantly impact the performance of machine learning algorithms. Easylibpal would employ data cleaning techniques to correct these errors, ensuring that the data is consistent and correctly formatted.
4. Deal with Missing Data
Handling missing data is a common challenge in data preprocessing. Easylibpal might use techniques such as imputation (filling missing values with statistical estimates like mean, median, or mode) or deletion (removing rows or columns with missing values) to address this issue. The choice of method depends on the nature of the data and the specific requirements of the analysis.
5. Filter Out Data Outliers
Outliers can significantly affect the performance of machine learning models. Easylibpal would use statistical methods to identify and filter out outliers, ensuring that the data is more representative of the population being analyzed.
6. Validate Data
The final step involves validating the cleaned and preprocessed data to ensure its quality and accuracy. This could include checking for consistency, verifying the correctness of the data, and ensuring that the data meets the requirements of the machine learning algorithms. Easylibpal would employ validation techniques to confirm that the data is ready for analysis.
To implement these data cleaning and preprocessing steps in Python, Easylibpal would leverage libraries like pandas and scikit-learn. Here's a conceptual example of how these steps might be integrated into the Easylibpal class:
```python
import pandas as pd
from sklearn.impute import SimpleImputer
from sklearn.preprocessing import StandardScaler
class Easylibpal:
def __init__(self, dataset):
self.dataset = dataset
# Load and preprocess the dataset
def clean_and_preprocess(self):
# Remove irrelevant data
self.dataset = self.dataset.drop(['irrelevant_column'], axis=1)
# Deduplicate data
self.dataset = self.dataset.drop_duplicates()
# Fix structural errors (example: correct data type)
self.dataset['correct_data_type_column'] = self.dataset['correct_data_type_column'].astype(float)
# Deal with missing data (example: imputation)
imputer = SimpleImputer(strategy='mean')
self.dataset['missing_data_column'] = imputer.fit_transform(self.dataset'missing_data_column')
# Filter out data outliers (example: using Z-score)
# This step requires a more detailed implementation based on the specific dataset
# Validate data (example: checking for NaN values)
assert not self.dataset.isnull().values.any(), "Data still contains NaN values"
# Return the cleaned and preprocessed dataset
return self.dataset
# Usage
Easylibpal = Easylibpal(dataset=pd.read_csv('your_dataset.csv'))
cleaned_dataset = Easylibpal.clean_and_preprocess()
```
This example demonstrates a simplified approach to data cleaning and preprocessing within Easylibpal. The actual implementation would need to adapt these steps based on the specific characteristics and requirements of the dataset being processed.
VALUE DATA
Easylibpal determines which data is irrelevant and can be removed through a combination of domain knowledge, data analysis, and automated techniques. The process involves identifying data that does not contribute to the analysis, research, or goals of the project, and removing it to improve the quality, efficiency, and clarity of the data. Here's how Easylibpal might approach this:
Domain Knowledge
Easylibpal leverages domain knowledge to identify data that is not relevant to the specific goals of the analysis or modeling task. This could include data that is out of scope, outdated, duplicated, or erroneous. By understanding the context and objectives of the project, Easylibpal can systematically exclude data that does not add value to the analysis.
Data Analysis
Easylibpal employs data analysis techniques to identify irrelevant data. This involves examining the dataset to understand the relationships between variables, the distribution of data, and the presence of outliers or anomalies. Data that does not have a significant impact on the predictive power of the model or the insights derived from the analysis is considered irrelevant.
Automated Techniques
Easylibpal uses automated tools and methods to remove irrelevant data. This includes filtering techniques to select or exclude certain rows or columns based on criteria or conditions, aggregating data to reduce its complexity, and deduplicating to remove duplicate entries. Tools like Excel, Google Sheets, Tableau, Power BI, OpenRefine, Python, R, Data Linter, Data Cleaner, and Data Wrangler can be employed for these purposes .
Examples of Irrelevant Data
- Personal Identifiable Information (PII): Data such as names, addresses, and phone numbers are irrelevant for most analytical purposes and should be removed to protect privacy and comply with data protection regulations .
- URLs and HTML Tags: These are typically not relevant to the analysis and can be removed to clean up the dataset.
- Boilerplate Text: Excessive blank space or boilerplate text (e.g., in emails) adds noise to the data and can be removed.
- Tracking Codes: These are used for tracking user interactions and do not contribute to the analysis.
To implement these steps in Python, Easylibpal might use pandas for data manipulation and filtering. Here's a conceptual example of how to remove irrelevant data:
```python
import pandas as pd
# Load the dataset
dataset = pd.read_csv('your_dataset.csv')
# Remove irrelevant columns (example: email addresses)
dataset = dataset.drop(['email_address'], axis=1)
# Remove rows with missing values (example: if a column is required for analysis)
dataset = dataset.dropna(subset=['required_column'])
# Deduplicate data
dataset = dataset.drop_duplicates()
# Return the cleaned dataset
cleaned_dataset = dataset
```
This example demonstrates how Easylibpal might remove irrelevant data from a dataset using Python and pandas. The actual implementation would need to adapt these steps based on the specific characteristics and requirements of the dataset being processed.
Detecting Inconsistencies
Easylibpal starts by detecting inconsistencies in the data. This involves identifying discrepancies in data types, missing values, duplicates, and formatting errors. By detecting these inconsistencies, Easylibpal can take targeted actions to address them.
Handling Formatting Errors
Formatting errors, such as inconsistent data types for the same feature, can significantly impact the analysis. Easylibpal uses functions like `astype()` in pandas to convert data types, ensuring uniformity and consistency across the dataset. This step is crucial for preparing the data for analysis, as it ensures that each feature is in the correct format expected by the algorithms.
Handling Missing Values
Missing values are a common issue in datasets. Easylibpal addresses this by consulting with subject matter experts to understand why data might be missing. If the missing data is missing completely at random, Easylibpal might choose to drop it. However, for other cases, Easylibpal might employ imputation techniques to fill in missing values, ensuring that the dataset is complete and ready for analysis.
Handling Duplicates
Duplicate entries can skew the analysis and lead to incorrect conclusions. Easylibpal uses pandas to identify and remove duplicates, ensuring that each entry in the dataset is unique. This step is crucial for maintaining the integrity of the data and ensuring that the analysis is based on distinct observations.
Handling Inconsistent Values
Inconsistent values, such as different representations of the same concept (e.g., "yes" vs. "y" for a binary variable), can also pose challenges. Easylibpal employs data cleaning techniques to standardize these values, ensuring that the data is consistent and can be accurately analyzed.
To implement these steps in Python, Easylibpal would leverage pandas for data manipulation and preprocessing. Here's a conceptual example of how these steps might be integrated into the Easylibpal class:
```python
import pandas as pd
class Easylibpal:
def __init__(self, dataset):
self.dataset = dataset
# Load and preprocess the dataset
def clean_and_preprocess(self):
# Detect inconsistencies (example: check data types)
print(self.dataset.dtypes)
# Handle formatting errors (example: convert data types)
self.dataset['date_column'] = pd.to_datetime(self.dataset['date_column'])
# Handle missing values (example: drop rows with missing values)
self.dataset = self.dataset.dropna(subset=['required_column'])
# Handle duplicates (example: drop duplicates)
self.dataset = self.dataset.drop_duplicates()
# Handle inconsistent values (example: standardize values)
self.dataset['binary_column'] = self.dataset['binary_column'].map({'yes': 1, 'no': 0})
# Return the cleaned and preprocessed dataset
return self.dataset
# Usage
Easylibpal = Easylibpal(dataset=pd.read_csv('your_dataset.csv'))
cleaned_dataset = Easylibpal.clean_and_preprocess()
```
This example demonstrates a simplified approach to handling inconsistent or messy data within Easylibpal. The actual implementation would need to adapt these steps based on the specific characteristics and requirements of the dataset being processed.
Statistical Imputation
Statistical imputation involves replacing missing values with statistical estimates such as the mean, median, or mode of the available data. This method is straightforward and can be effective for numerical data. For categorical data, mode imputation is commonly used. The choice of imputation method depends on the distribution of the data and the nature of the missing values.
Model-Based Imputation
Model-based imputation uses machine learning models to predict missing values. This approach can be more sophisticated and potentially more accurate than statistical imputation, especially for complex datasets. Techniques like K-Nearest Neighbors (KNN) imputation can be used, where the missing values are replaced with the values of the K nearest neighbors in the feature space.
Using SimpleImputer in scikit-learn
The scikit-learn library provides the `SimpleImputer` class, which supports both statistical and model-based imputation. `SimpleImputer` can be used to replace missing values with the mean, median, or most frequent value (mode) of the column. It also supports more advanced imputation methods like KNN imputation.
To implement these imputation techniques in Python, Easylibpal might use the `SimpleImputer` class from scikit-learn. Here's an example of how to use `SimpleImputer` for statistical imputation:
```python
from sklearn.impute import SimpleImputer
import pandas as pd
# Load the dataset
dataset = pd.read_csv('your_dataset.csv')
# Initialize SimpleImputer for numerical columns
num_imputer = SimpleImputer(strategy='mean')
# Fit and transform the numerical columns
dataset'numerical_column1', 'numerical_column2' = num_imputer.fit_transform(dataset'numerical_column1', 'numerical_column2')
# Initialize SimpleImputer for categorical columns
cat_imputer = SimpleImputer(strategy='most_frequent')
# Fit and transform the categorical columns
dataset'categorical_column1', 'categorical_column2' = cat_imputer.fit_transform(dataset'categorical_column1', 'categorical_column2')
# The dataset now has missing values imputed
```
This example demonstrates how to use `SimpleImputer` to fill in missing values in both numerical and categorical columns of a dataset. The actual implementation would need to adapt these steps based on the specific characteristics and requirements of the dataset being processed.
Model-based imputation techniques, such as Multiple Imputation by Chained Equations (MICE), offer powerful ways to handle missing data by using statistical models to predict missing values. However, these techniques come with their own set of limitations and potential drawbacks:
1. Complexity and Computational Cost
Model-based imputation methods can be computationally intensive, especially for large datasets or complex models. This can lead to longer processing times and increased computational resources required for imputation.
2. Overfitting and Convergence Issues
These methods are prone to overfitting, where the imputation model captures noise in the data rather than the underlying pattern. Overfitting can lead to imputed values that are too closely aligned with the observed data, potentially introducing bias into the analysis. Additionally, convergence issues may arise, where the imputation process does not settle on a stable solution.
3. Assumptions About Missing Data
Model-based imputation techniques often assume that the data is missing at random (MAR), which means that the probability of a value being missing is not related to the values of other variables. However, this assumption may not hold true in all cases, leading to biased imputations if the data is missing not at random (MNAR).
4. Need for Suitable Regression Models
For each variable with missing values, a suitable regression model must be chosen. Selecting the wrong model can lead to inaccurate imputations. The choice of model depends on the nature of the data and the relationship between the variable with missing values and other variables.
5. Combining Imputed Datasets
After imputing missing values, there is a challenge in combining the multiple imputed datasets to produce a single, final dataset. This requires careful consideration of how to aggregate the imputed values and can introduce additional complexity and uncertainty into the analysis.
6. Lack of Transparency
The process of model-based imputation can be less transparent than simpler imputation methods, such as mean or median imputation. This can make it harder to justify the imputation process, especially in contexts where the reasons for missing data are important, such as in healthcare research.
Despite these limitations, model-based imputation techniques can be highly effective for handling missing data in datasets where a amusingness is MAR and where the relationships between variables are complex. Careful consideration of the assumptions, the choice of models, and the methods for combining imputed datasets are crucial to mitigate these drawbacks and ensure the validity of the imputation process.
USING EASYLIBPAL FOR AI ALGORITHM INTEGRATION OFFERS SEVERAL SIGNIFICANT BENEFITS, PARTICULARLY IN ENHANCING EVERYDAY LIFE AND REVOLUTIONIZING VARIOUS SECTORS. HERE'S A DETAILED LOOK AT THE ADVANTAGES:
1. Enhanced Communication: AI, through Easylibpal, can significantly improve communication by categorizing messages, prioritizing inboxes, and providing instant customer support through chatbots. This ensures that critical information is not missed and that customer queries are resolved promptly.
2. Creative Endeavors: Beyond mundane tasks, AI can also contribute to creative endeavors. For instance, photo editing applications can use AI algorithms to enhance images, suggesting edits that align with aesthetic preferences. Music composition tools can generate melodies based on user input, inspiring musicians and amateurs alike to explore new artistic horizons. These innovations empower individuals to express themselves creatively with AI as a collaborative partner.
3. Daily Life Enhancement: AI, integrated through Easylibpal, has the potential to enhance daily life exponentially. Smart homes equipped with AI-driven systems can adjust lighting, temperature, and security settings according to user preferences. Autonomous vehicles promise safer and more efficient commuting experiences. Predictive analytics can optimize supply chains, reducing waste and ensuring goods reach users when needed.
4. Paradigm Shift in Technology Interaction: The integration of AI into our daily lives is not just a trend; it's a paradigm shift that's redefining how we interact with technology. By streamlining routine tasks, personalizing experiences, revolutionizing healthcare, enhancing communication, and fueling creativity, AI is opening doors to a more convenient, efficient, and tailored existence.
5. Responsible Benefit Harnessing: As we embrace AI's transformational power, it's essential to approach its integration with a sense of responsibility, ensuring that its benefits are harnessed for the betterment of society as a whole. This approach aligns with the ethical considerations of using AI, emphasizing the importance of using AI in a way that benefits all stakeholders.
In summary, Easylibpal facilitates the integration and use of AI algorithms in a manner that is accessible and beneficial across various domains, from enhancing communication and creative endeavors to revolutionizing daily life and promoting a paradigm shift in technology interaction. This integration not only streamlines the application of AI but also ensures that its benefits are harnessed responsibly for the betterment of society.
USING EASYLIBPAL OVER TRADITIONAL AI LIBRARIES OFFERS SEVERAL BENEFITS, PARTICULARLY IN TERMS OF EASE OF USE, EFFICIENCY, AND THE ABILITY TO APPLY AI ALGORITHMS WITH MINIMAL CONFIGURATION. HERE ARE THE KEY ADVANTAGES:
- Simplified Integration: Easylibpal abstracts the complexity of traditional AI libraries, making it easier for users to integrate classic AI algorithms into their projects. This simplification reduces the learning curve and allows developers and data scientists to focus on their core tasks without getting bogged down by the intricacies of AI implementation.
- User-Friendly Interface: By providing a unified platform for various AI algorithms, Easylibpal offers a user-friendly interface that streamlines the process of selecting and applying algorithms. This interface is designed to be intuitive and accessible, enabling users to experiment with different algorithms with minimal effort.
- Enhanced Productivity: The ability to effortlessly instantiate algorithms, fit models with training data, and make predictions with minimal configuration significantly enhances productivity. This efficiency allows for rapid prototyping and deployment of AI solutions, enabling users to bring their ideas to life more quickly.
- Democratization of AI: Easylibpal democratizes access to classic AI algorithms, making them accessible to a wider range of users, including those with limited programming experience. This democratization empowers users to leverage AI in various domains, fostering innovation and creativity.
- Automation of Repetitive Tasks: By automating the process of applying AI algorithms, Easylibpal helps users save time on repetitive tasks, allowing them to focus on more complex and creative aspects of their projects. This automation is particularly beneficial for users who may not have extensive experience with AI but still wish to incorporate AI capabilities into their work.
- Personalized Learning and Discovery: Easylibpal can be used to enhance personalized learning experiences and discovery mechanisms, similar to the benefits seen in academic libraries. By analyzing user behaviors and preferences, Easylibpal can tailor recommendations and resource suggestions to individual needs, fostering a more engaging and relevant learning journey.
- Data Management and Analysis: Easylibpal aids in managing large datasets efficiently and deriving meaningful insights from data. This capability is crucial in today's data-driven world, where the ability to analyze and interpret large volumes of data can significantly impact research outcomes and decision-making processes.
In summary, Easylibpal offers a simplified, user-friendly approach to applying classic AI algorithms, enhancing productivity, democratizing access to AI, and automating repetitive tasks. These benefits make Easylibpal a valuable tool for developers, data scientists, and users looking to leverage AI in their projects without the complexities associated with traditional AI libraries.
2 notes
·
View notes
Text
What It’s Like to Be a Full Stack Developer: A Day in My Life
Have you ever wondered what it’s like to be a full stack developer? The world of full stack development is a thrilling and dynamic one, filled with challenges and opportunities to create end-to-end solutions. In this blog post, I’m going to take you through a day in my life as a full stack developer, sharing the ins and outs of my daily routine, the exciting projects I work on, and the skills that keep me at the forefront of technology.
Morning Ritual: Coffee, Code, and Planning
My day typically begins with a strong cup of coffee and some quiet time for reflection. It’s during this peaceful morning routine that I gather my thoughts, review my task list, and plan the day ahead. Full stack development demands a strategic approach, so having a clear plan is essential.
Once I’m geared up, I dive into code. Mornings are often the most productive time for me, so I use this period to tackle complex tasks that require deep concentration. Whether it’s optimizing database queries or fine-tuning the user interface, the morning is when I make significant progress.
The Balancing Act: Frontend and Backend Work
One of the defining aspects of being a full stack developer is the constant juggling between frontend and backend development. I seamlessly switch between crafting elegant user interfaces and building robust server-side logic.
In the frontend world, I work with HTML, CSS, and JavaScript to create responsive and visually appealing web applications. I make sure that the user experience is smooth, intuitive, and visually appealing. From designing layouts to implementing user interactions, frontend development keeps me creatively engaged.
On the backend, I manage server-side scripting languages like Python and Node.js, ensuring that the data and logic behind the scenes are rock-solid. Databases, both SQL and NoSQL, play a central role in the backend, and I optimize them for performance and scalability. Building APIs, handling authentication, and managing server infrastructure are all part of the backend responsibilities.
Collaboration and Teamwork
Full stack development often involves collaborating with a diverse team of developers, designers, and project managers. Teamwork is a cornerstone of success in our field, and communication is key. I engage in daily stand-up meetings to sync up with the team, share progress, and discuss roadblocks.
Collaborative tools like Git and platforms like GitHub facilitate seamless code collaboration. Code reviews are a regular part of our workflow, ensuring that the codebase remains clean, maintainable, and secure. It’s in these collaborative moments that we learn from each other, refine our skills, and collectively push the boundaries of what’s possible.
Continuous Learning and Staying Updated
Technology evolves at a rapid pace, and staying updated is paramount for a full stack developer. In the afternoon, I set aside time for learning and exploration. Whether it’s delving into a new framework, exploring emerging technologies like serverless computing, or simply catching up on industry news, this dedicated learning time keeps me ahead of the curve. The ACTE Institute offers numerous Full stack developer courses, bootcamps, and communities that can provide you with the necessary resources and support to succeed in this field. Best of luck on your exciting journey!
The Thrill of Problem Solving
As the day progresses, I often find myself tackling unforeseen challenges. Full stack development is, at its core, problem-solving. Debugging issues, optimizing code, and finding efficient solutions are all part of the job. These challenges keep me on my toes and are a source of constant learning.
Evening Reflection: Wrapping Up and Looking Ahead
As the day winds down, I wrap up my work, conduct final code reviews, and prepare for the next day. Full stack development is a fulfilling journey, but it’s important to strike a balance between work and personal life.
Reflecting on the day’s accomplishments and challenges, I’m reminded of the rewarding nature of being a full stack developer. It’s a role that demands versatility, creativity, and adaptability, but it’s also a role that offers endless opportunities for growth and innovation.
Being a full stack developer is not just a job; it’s a way of life. Each day is a new adventure filled with code, collaboration, and the excitement of building end-to-end solutions. While the challenges are real, the satisfaction of creating something meaningful is immeasurable. If you’ve ever wondered what it’s like to be a full stack developer, I hope this glimpse into my daily life has shed some light on the dynamic and rewarding world of full stack development.
#full stack developer#frameworks#web development#web design#education#learning#information#technology
3 notes
·
View notes
Text
In today’s digital era, database performance is critical to the overall speed, stability, and scalability of modern applications. Whether you're running a transactional system, an analytics platform, or a hybrid database structure, maintaining optimal performance is essential to ensure seamless user experiences and operational efficiency.
In this blog, we'll explore effective strategies to improve database performance, reduce latency, and support growing data workloads without compromising system reliability.
1. Optimize Queries and Use Prepared Statements
Poorly written SQL queries are often the root cause of performance issues. Long-running or unoptimized queries can hog resources and slow down the entire system. Developers should focus on:
Using EXPLAIN plans to analyze query execution paths
Avoiding unnecessary columns or joins
Reducing the use of SELECT *
Applying appropriate filters and limits
Prepared statements can also boost performance by reducing parsing overhead and improving execution times for repeated queries.
2. Leverage Indexing Strategically
Indexes are powerful tools for speeding up data retrieval, but improper use can lead to overhead during insert and update operations. Indexes should be:
Applied selectively to frequently queried columns
Monitored for usage and dropped if rarely used
Regularly maintained to avoid fragmentation
Composite indexes can also be useful when multiple columns are queried together.
3. Implement Query Caching
Query caching can drastically reduce response times for frequent reads. By storing the results of expensive queries temporarily, you avoid reprocessing the same query multiple times. However, it's important to:
Set appropriate cache lifetimes
Avoid caching volatile or frequently changing data
Clear or invalidate cache when updates occur
Database proxy tools can help with intelligent query caching at the SQL layer.
4. Use Connection Pooling
Establishing database connections repeatedly consumes both time and resources. Connection pooling allows applications to reuse existing database connections, improving:
Response times
Resource management
Scalability under load
Connection pools can be fine-tuned based on application traffic patterns to ensure optimal throughput.
5. Partition Large Tables
Large tables with millions of records can suffer from slow read and write performance. Partitioning breaks these tables into smaller, manageable segments based on criteria like range, hash, or list. This helps:
Speed up query performance
Reduce index sizes
Improve maintenance tasks such as vacuuming or archiving
Partitioning also simplifies data retention policies and backup processes.
6. Monitor Performance Metrics Continuously
Database monitoring tools are essential to track performance metrics in real time. Key indicators to watch include:
Query execution time
Disk I/O and memory usage
Cache hit ratios
Lock contention and deadlocks
Proactive monitoring helps identify bottlenecks early and prevents system failures before they escalate.
7. Ensure Hardware and Infrastructure Support
While software optimization is key, underlying infrastructure also plays a significant role. Ensure your hardware supports current workloads by:
Using SSDs for faster data access
Scaling vertically (more RAM/CPU) or horizontally (sharding) as needed
Optimizing network latency for remote database connections
Cloud-native databases and managed services also offer built-in scaling options for dynamic workloads.
8. Regularly Update and Tune the Database Engine
Database engines release frequent updates to fix bugs, enhance performance, and introduce new features. Keeping your database engine up-to-date ensures:
Better performance tuning options
Improved security
Compatibility with modern application architectures
Additionally, fine-tuning engine parameters like buffer sizes, parallel execution, and timeout settings can significantly enhance throughput.
0 notes
Text
Reliable Website Maintenance Services In India | NRS Infoways
In today’s hyper‑connected marketplace, a website is far more than a digital brochure—it is the beating heart of your brand experience, your lead‑generation engine, and your most valuable sales asset. Yet many businesses still treat their sites as “launch‑and‑forget” projects, only paying attention when something breaks. At NRS Infoways, we understand that real online success demands continuous care, proactive monitoring, and seamless enhancements. That’s why we’ve built our Reliable Website Maintenance Services In India to deliver round‑the‑clock peace of mind, bulletproof performance, and measurable ROI for forward‑thinking companies like yours.
Why Website Maintenance Matters—And Why “Reliable” Makes All the Difference
Search engines reward fast, secure, and regularly updated sites with higher rankings; customers reward them with trust and loyalty. Conversely, a sluggish, outdated, or vulnerable site can cost you traffic, conversions, and brand reputation—sometimes overnight. Our Reliable Website Maintenance Services In India go beyond the basic “fix‑it‑when‑it‑breaks” model. We combine proactive health checks, performance tuning, security hardening, and content optimization into a single, cohesive program that keeps your digital storefront open, polished, and ready for growth.
What Sets NRS Infoways Apart?
1. Proactive Performance Monitoring
We leverage enterprise‑grade monitoring tools that continuously scan load times, server resources, and user journeys. By identifying bottlenecks before they escalate, we ensure smoother experiences and higher conversion rates—24/7.
2. Robust Security & Compliance
From real‑time threat detection to regular firewall updates and SSL renewals, your site stays impervious to malware, SQL injections, and DDoS attacks. We align with global standards such as GDPR and PCI‑DSS, keeping you compliant and trustworthy.
3. Seamless Content & Feature Updates
Launching a new product line? Running a seasonal promotion? Our dedicated team updates layouts, landing pages, and plugins—often within hours—to keep your messaging sharp and relevant without disrupting uptime.
4. Data‑Driven Optimization
Monthly analytics reviews highlight user behavior, bounce rates, and conversion funnels. We translate insights into actionable tasks—A/B testing CTAs, compressing heavy images, or refining navigation—all folded into our maintenance retainer.
5. Transparent Reporting & SLAs
Every client receives detailed monthly reports covering task logs, incident resolutions, and performance metrics. Our Service Level Agreements guarantee response times as low as 30 minutes for critical issues, underscoring the “Reliable” in our Reliable Website Maintenance Services In India.
Real‑World Impact: A Success Snapshot
A Delhi‑based B2B SaaS provider reached out to NRS Infoways after repeated downtime eroded user trust and slashed demo bookings by 18 %. Within the first month of onboarding, we:
Migrated their site to a high‑availability cloud cluster
Deployed a Web Application Firewall (WAF) to fend off bot attacks
Compressed multimedia assets, cutting average load time from 4.2 s to 1.3 s
Implemented weekly backup protocols with versioned restores
Result? Organic traffic climbed 27 %, demo sign‑ups rebounded 31 %, and support tickets fell by half—proving that consistent, expert care translates directly into revenue.
Flexible Plans That Scale With You
Whether you manage a lean startup site or a sprawling enterprise portal, we offer tiered packages—Basic, Professional, and Enterprise—each customizable with à‑la‑carte add‑ons like e‑commerce catalog updates, multi‑language support, or advanced SEO audits. As your business evolves, our services scale seamlessly, ensuring you never pay for overhead you don’t need or sacrifice features you do.
Partner With NRS Infoways Today
Your website is too important to leave to chance. Join the growing roster of Indian businesses that rely on NRS Infoways for Reliable Website Maintenance Services In India and experience the freedom to innovate while we handle the technical heavy lifting. Ready to protect your digital investment, delight your visitors, and outpace your competition?
Connect with our maintenance experts now and power your growth with reliability you can measure.
0 notes
Text
Automation Product Architect
Job SummaryWe are seeking a talented Automation Product Architect (10 Years) to join our team. If you're passionate about coding, problem-solving, and innovation, wed love to hear from you!About CodeVyasa: We're a fast-growing multinational software company with offices in Florida and New Delhi. Our clientele spans across the US, Australia, and the APAC region. We're proud to collaborate with Fortune 500 companies and offer opportunities to work alongside the top 0.1 percent of developers in the industry. You'll report to IIT/BITS graduates with over 10 years of development experience. Ready to elevate your career? Visit us at codevyasa.com. Must-Have Skills:
Microsoft Power Platform (Power Automate, Power Apps, Power BI)
UiPath (RPA Development, Orchestrator, Bot Management)
Strong understanding of automation design principles and business process optimization
Experience with data sources like SharePoint, SQL, Excel, and Dataverse
Scripting and expression writing (Power Fx, VB.Net, Python, or JavaScript)
API integration and knowledge of REST/JSON services
Good troubleshooting, debugging, and performance tuning skillsGood-to-Have Skills:
Familiarity with Azure Logic Apps or Azure Functions
Experience working with Agile/Scrum teams
Exposure to custom connectors and low-code/no-code governance frameworks
Basic knowledge of Power Virtual Agents
Why Join CodeVyasa?Work on innovative, high-impact projects with a team of top-tier professionals.
Continuous learning opportunities and professional growth.
Flexible work environment with a supportive company culture.
Competitive salary and comprehensive benefits package.
Free healthcare coverage.
Budget- Upto 55 lakhs
Location- Chennai
Must Have skills- Ui Path, Power platforms
Job Type
Payroll
Categories
Product Specialists (Sales)
Systems Analysts (Information Design and Documentaion)
Software Engineer (Software and Web Development)
Data Engineer (Software and Web Development)
Automation Engineer (Software and Web Development)
Business Process Analyst (Information Design and Documentaion)
Architect (Contruction )
Must have Skills
PowerApps - 10 Years
UiPath - 10 Years
Power BI - 8 Years
SQL - 4 YearsIntermediate
SharePoint - 4 YearsIntermediate
REST - 4 YearsIntermediate
Azure - 4 YearsIntermediate
Apply Now: https://crazysolutions.in/job-openings/
0 notes
Text
Should You Change Lock Escalation Behavior to Fix SQL Server Blocking Issues?
Introduction Have you ever encountered blocking problems in your SQL Server databases due to lock escalation? As a DBA, I certainly have! Lock escalation can cause queries to grind to a halt as they wait for locks, slowing down the entire system. It’s a frustrating issue, but luckily there are ways to address it. In this article, we’ll take an in-depth look at lock escalation – what causes it,…
View On WordPress
0 notes
Text
Why Your Business Needs a B2B Marketing Agency in Bangalore

Bangalore — often called the Silicon Valley of India — is no longer just a hub for tech startups. It’s also one of the fastest-growing cities for business-to-business (B2B) innovation. If you're a business aiming to scale, acquire high-quality leads, and build long-term partnerships, partnering with a B2B marketing agency in Bangalore could be your smartest move. These agencies offer the perfect mix of digital expertise, industry knowledge, and performance-driven strategies tailored to B2B needs.
What Makes Bangalore a Hotspot for B2B Marketing?
Bangalore’s strength lies in its unique combination of tech-forward thinking and business diversity. It’s home to thousands of startups, IT giants, manufacturing companies, SaaS platforms, and export-based businesses — all of which require specialized B2B marketing support.
🚀 Strong Tech Ecosystem
With access to advanced tools, data analytics, and marketing automation, agencies in Bangalore are ahead of the curve. They use platforms like HubSpot, Salesforce, SEMrush, and LinkedIn Sales Navigator to deliver high-performing B2B campaigns.
🌍 International Exposure
Many Bangalore-based agencies work with global clients across the US, UK, Australia, and UAE. This experience helps them understand international buyer journeys and compliance regulations like GDPR.
👨💻 Skilled Talent Pool
With leading institutions like IIM Bangalore and NIFT, plus a huge tech workforce, the city offers a pool of marketers, content creators, strategists, and designers — all focused on results.
💡 Startup and Enterprise Mix
Whether you're a new SaaS founder or part of a legacy manufacturing firm, Bangalore agencies are equipped to handle businesses at any scale.
Core Services Offered by B2B Marketing Agencies in Bangalore
A professional B2B marketing agency doesn’t just build brand awareness — it drives measurable outcomes like leads, conversions, and customer retention. Here's what they typically offer:
1. Account-Based Marketing (ABM)
Hyper-targeted outreach to decision-makers in specific companies using personalized content and ads.
2. SEO and Content Marketing
Creating whitepapers, blogs, and case studies that position your brand as an industry expert while improving your search engine rankings.
3. LinkedIn and Email Campaigns
Running automated drip sequences and LinkedIn messaging that attract, engage, and convert high-value B2B leads.
4. Sales Enablement
Providing your sales team with pitch decks, product explainers, and CRM integration to close deals faster.
5. Web Development for B2B
Designing conversion-optimized landing pages, industry-specific websites, and customer portals with clear CTAs and trust elements.
6. Performance Marketing
Google Ads, remarketing, and social ads specifically tuned for B2B products or services with longer sales cycles.
Why B2B Companies Are Choosing Bangalore-Based Agencies
Let’s look at what makes Bangalore agencies ideal for B2B growth.
🎯 Laser-Focused on ROI
Agencies here understand that B2B marketing is not just about impressions but about pipeline value, SQLs (sales-qualified leads), and deal acceleration.
📈 Scalable Strategy for Growing Businesses
From Series A startups to enterprises, Bangalore agencies adapt their strategies as you scale — no one-size-fits-all.
🧠 Data-Driven Decision Making
Using advanced analytics and real-time dashboards, campaigns are continuously optimized for performance.
🤝 Collaborative and Agile Approach
You don’t just get a service provider — you get a growth partner that collaborates with your internal teams.
Case Study Highlights: Real Impact
🌐 SaaS Startup Expands to US Market
A SaaS company based in HSR Layout worked with a B2B marketing agency in Bangalore to penetrate the US market. The agency crafted US-targeted SEO blogs, LinkedIn ads, and demo webinars, resulting in a 40% increase in monthly demos and a 3x boost in MQLs.
🏭 Manufacturing Brand Goes Digital
A Peenya-based manufacturer partnered with a Bangalore B2B agency to shift from offline sales to digital lead gen. Using ABM, email marketing, and trade-specific landing pages, they doubled their B2B lead flow within 3 months.
What to Look for in a B2B Marketing Partner in Bangalore
Before choosing your agency, check if they offer:
Industry Experience in your niche (tech, healthcare, legal, etc.)
Marketing Automation Expertise (HubSpot, Marketo, etc.)
Strong Case Studies and Client References
Multichannel Capabilities across email, SEO, LinkedIn, and PPC
Transparent Reporting and ROI Tracking
Bonus: Many Bangalore agencies also offer workshops and training for your in-house teams to improve alignment between marketing and sales.
Trends Shaping B2B Marketing in Bangalore
Agencies in Bangalore are on the cutting edge of B2B innovation. Here’s what’s trending:
📽️ B2B Video Storytelling
From explainer videos to virtual plant tours, visual content is a powerful lead magnet for complex B2B products.
🤖 AI-Powered Lead Scoring
Smart scoring systems using AI to identify which leads are most likely to convert — improving sales productivity.
📢 Voice & Podcast Marketing
Brands are exploring voice SEO and branded podcasts to build thought leadership and drive deeper engagement.
💼 CEO Branding
Positioning your founder or CEO as a thought leader on LinkedIn is now a strategic B2B move.
Conclusion: Why a B2B Marketing Agency in Bangalore Is Your Next Big Move
In today’s fast-paced, competitive business environment, having the right marketing partner is essential — and a B2B marketing agency in Bangalore brings everything you need to the table. From precision targeting and data-backed strategies to content that educates and converts, these agencies are built to help you grow sustainably and smartly.
Whether you’re looking to expand your presence in India, break into global markets, or build long-term client relationships, a B2B marketing agency in Bangalore offers the strategy, talent, and tools to make it happen. With their deep understanding of both Indian and international B2B dynamics, these agencies deliver tailored campaigns that actually move the needle.
In short, a B2B marketing agency in Bangalore doesn’t just deliver leads — they deliver business outcomes. If you're ready to scale, improve ROI, and future-proof your brand, it’s time to choose a Bangalore-based partner who can take you there.
0 notes
Text
5 Ultimate Industry Trends That Define the Future of Data Science
Data science is a field in constant motion, a dynamic blend of statistics, computer science, and domain expertise. Just when you think you've grasped the latest tool or technique, a new paradigm emerges. As we look towards the immediate future and beyond, several powerful trends are coalescing to redefine what it means to be a data scientist and how data-driven insights are generated.
Here are 5 ultimate industry trends that are shaping the future of data science:
1. Generative AI and Large Language Models (LLMs) as Co-Pilots
This isn't just about data scientists using Gen-AI; it's about Gen-AI augmenting the data scientist themselves.
Automated Code Generation: LLMs are becoming increasingly adept at generating SQL queries, Python scripts for data cleaning, feature engineering, and even basic machine learning models from natural language prompts.
Accelerated Research & Synthesis: LLMs can quickly summarize research papers, explain complex concepts, brainstorm hypotheses, and assist in drafting reports, significantly speeding up the research phase.
Democratizing Access: By lowering the bar for coding and complex analysis, LLMs enable "citizen data scientists" and domain experts to perform more sophisticated data tasks.
Future Impact: Data scientists will shift from being pure coders to being "architects of prompts," validators of AI-generated content, and experts in fine-tuning and integrating LLMs into their workflows.
2. MLOps Maturation and Industrialization
The focus is shifting from building individual models to operationalizing entire machine learning lifecycles.
Production-Ready AI: Organizations realize that a model in a Jupyter notebook provides no business value. MLOps (Machine Learning Operations) provides the practices and tools to reliably deploy, monitor, and maintain ML models in production environments.
Automated Pipelines: Expect greater automation in data ingestion, model training, versioning, testing, deployment, and continuous monitoring.
Observability & Governance: Tools for tracking model performance, data drift, bias detection, and ensuring compliance with regulations will become standard.
Future Impact: Data scientists will need stronger software engineering skills and a deeper understanding of deployment environments. The line between data scientist and ML engineer will continue to blur.
3. Ethical AI and Responsible AI Taking Center Stage
As AI systems become more powerful and pervasive, the ethical implications are no longer an afterthought.
Bias Detection & Mitigation: Rigorous methods for identifying and reducing bias in training data and model outputs will be crucial to ensure fairness and prevent discrimination.
Explainable AI (XAI): The demand for understanding why an AI model made a particular decision will grow, driven by regulatory pressure (e.g., EU AI Act) and the need for trust in critical applications.
Privacy-Preserving AI: Techniques like federated learning and differential privacy will gain prominence to allow models to be trained on sensitive data without compromising individual privacy.
Future Impact: Data scientists will increasingly be responsible for the ethical implications of their models, requiring a strong grasp of responsible AI principles, fairness metrics, and compliance frameworks.
4. Edge AI and Real-time Analytics Proliferation
The need for instant insights and local processing is pushing AI out of the cloud and closer to the data source.
Decentralized Intelligence: Instead of sending all data to a central cloud for processing, AI models will increasingly run on devices (e.g., smart cameras, IoT sensors, autonomous vehicles) at the "edge" of the network.
Low Latency Decisions: This enables real-time decision-making for applications where milliseconds matter, reducing bandwidth constraints and improving responsiveness.
Hybrid Architectures: Data scientists will work with complex hybrid architectures where some processing happens at the edge and aggregated data is sent to the cloud for deeper analysis and model retraining.
Future Impact: Data scientists will need to understand optimization techniques for constrained environments and the challenges of deploying and managing models on diverse hardware.
5. Democratization of Data Science & Augmented Analytics
Data science insights are becoming accessible to a broader audience, not just specialized practitioners.
Low-Code/No-Code (LCNC) Platforms: These platforms empower business analysts and domain experts to build and deploy basic ML models without extensive coding knowledge.
Augmented Analytics: AI-powered tools that automate parts of the data analysis process, such as data preparation, insight generation, and natural language explanations, making data more understandable to non-experts.
Data Literacy: A greater emphasis on fostering data literacy across the entire organization, enabling more employees to interpret and utilize data insights.
Future Impact: Data scientists will evolve into mentors, consultants, and developers of tools that empower others, focusing on solving the most complex and novel problems that LCNC tools cannot handle.
The future of data science is dynamic, exciting, and demanding. Success in this evolving landscape will require not just technical prowess but also adaptability, a strong ethical compass, and a continuous commitment to learning and collaboration.
0 notes
Text
Top 5 Tools for Salesforce Data Migration in 2025

Data migration is a critical aspect of any Salesforce implementation or upgrade. Whether you’re transitioning from legacy systems, merging Salesforce orgs, or simply updating your current Salesforce instance, choosing the right tool can make or break the success of your migration. In 2025, the landscape of Salesforce data migration tools has evolved significantly, offering more automation, better user interfaces, and improved compatibility with complex datasets.
If you're a business looking to ensure a smooth migration process, working with an experienced Salesforce consultant in New York can help you identify the best tools and practices. Here's a detailed look at the top five Salesforce data migration tools in 2025 and how they can help your organization move data efficiently and accurately.
1. Salesforce Data Loader (Enhanced 2025 Edition)
Overview: The Salesforce Data Loader remains one of the most popular tools, especially for companies looking for a free, secure, and reliable way to manage data migration. The 2025 edition comes with a modernized UI, faster processing speeds, and enhanced error logging.
Why It’s Top in 2025:
Improved speed and performance
Enhanced error tracking and data validation
Seamless integration with external databases like Oracle, SQL Server, and PostgreSQL
Support for larger datasets (up to 10 million records)
Best For: Organizations with experienced admins or developers who are comfortable working with CSV files and need a high level of control over their data migration process.
Pro Tip: Engage a Salesforce developer in New York to write custom scripts for automating the loading and extraction processes. This will save significant time during large migrations.
2. Skyvia
Overview: Skyvia has emerged as a go-to cloud-based data integration tool that simplifies Salesforce data migration, especially for non-technical users. With drag-and-drop functionality and pre-built templates, it supports integration between Salesforce and over 100 other platforms.
Why It’s Top in 2025:
No coding required
Advanced transformation capabilities
Real-time sync between Salesforce and other cloud applications
Enhanced data governance features
Best For: Mid-sized businesses and enterprises that need a user-friendly platform with robust functionality and real-time synchronization.
Use Case: A retail company integrating Shopify, Salesforce, and NetSuite found Skyvia especially helpful in maintaining consistent product and customer data across platforms.
Expert Advice: Work with a Salesforce consulting partner in New York to set up your data models and design a migration path that aligns with your business processes.
3. Jitterbit Harmony
Overview: Jitterbit Harmony is a powerful data integration platform that enables users to design, run, and manage integration workflows. In 2025, it remains a favorite for enterprises due to its AI-powered suggestions and robust performance in complex scenarios.
Why It’s Top in 2025:
AI-enhanced mapping and transformation logic
Native Salesforce connector with bulk API support
Real-time data flow monitoring and alerts
Cross-platform compatibility (on-premise to cloud, cloud to cloud)
Best For: Large enterprises and organizations with complex IT ecosystems requiring high-throughput data migration and real-time integrations.
Tip from the Field: A Salesforce consulting firm in New York can help fine-tune your Jitterbit setup to ensure compliance with your industry regulations and data handling policies.
4. Informatica Cloud Data Wizard
Overview: Informatica is well-known in the enterprise data integration space. The Cloud Data Wizard is a lightweight, Salesforce-focused tool designed for business users. In 2025, its intuitive interface and automated field mapping make it a favorite for quick and simple migrations.
Why It’s Top in 2025:
Automatic schema detection and mapping
Pre-built Salesforce templates
Role-based access control for secure collaboration
Integration with Salesforce Flow for process automation
Best For: Companies needing quick, on-the-fly migrations with minimal IT involvement.
Case in Point: A nonprofit organization used Informatica Cloud Data Wizard for migrating donor information from spreadsheets into Salesforce Nonprofit Success Pack (NPSP) with minimal technical assistance.
Pro Insight: Partner with a Salesforce consultant in New York to evaluate whether the Cloud Data Wizard meets your scalability and security needs before committing.
5. Talend Data Fabric
Overview: Talend Data Fabric combines data integration, quality, and governance in one unified platform. In 2025, it leads the way in enterprise-grade data migration for Salesforce users who require deep customization, high security, and data lineage tracking.
Why It’s Top in 2025:
Full data quality and compliance toolset
AI-driven suggestions for data cleaning and transformation
End-to-end data lineage tracking
Integration with AWS, Azure, and Google Cloud
Best For: Industries with strict compliance needs like finance, healthcare, or government, where data accuracy and traceability are paramount.
Strategic Advantage: A Salesforce consulting partner in New York can help configure Talend’s governance tools to align with HIPAA, GDPR, or other regulatory requirements.
Why Choosing the Right Tool Matters
Data migration is more than just moving records from one system to another—it’s about preserving the integrity, security, and usability of your data. Choosing the right tool ensures:
Fewer errors and data loss
Faster deployment timelines
Higher end-user adoption
Better alignment with business goals
Partnering with Salesforce Experts in New York
Working with an experienced Salesforce consultant in New York can help you navigate the complexities of data migration. Local consultants understand both the technical and business landscapes and can offer personalized support throughout the migration journey.
Whether you're a startup looking for lean, cost-effective solutions or a large enterprise needing advanced governance, engaging with Salesforce consultants in New York ensures you make the most informed decisions.
These professionals can:
Conduct data audits and mapping
Recommend the best tool for your specific use case
Build custom scripts or integrations as needed
Ensure a smooth transition with minimal business disruption
Final Thoughts
In 2025, Salesforce data migration is no longer a cumbersome, manual task. With tools like Salesforce Data Loader, Skyvia, Jitterbit, Informatica, and Talend, businesses of all sizes can achieve fast, secure, and seamless migrations. The key lies in selecting the right tool based on your business size, technical capacity, and compliance needs.
Moreover, partnering with a knowledgeable Salesforce consulting partner in New York gives you access to tailored solutions and hands-on support, making your data migration journey smooth and successful.
Ready to migrate your data the right way? Consult with a trusted Salesforce consulting in New York expert and empower your business to scale with confidence.
#salesforce consultant in new york#salesforce consulting in new york#salesforce consulting partner in new york#salesforce consultants in new york#salesforce developer in new york#Top 5 Tools for Salesforce Data Migration in 2025
0 notes
Text
How Our SAP HANA Course Prepares You for Real-World Projects
In today’s fast-paced, data-driven business environment, organizations are increasingly relying on real-time analytics and enterprise-ready solutions to stay competitive. SAP HANA (High-Performance Analytic Appliance) has emerged as a leading in-memory database and application development platform for enterprise data processing. But understanding the theory behind SAP HANA is only part of the equation. To thrive in the job market or perform in a corporate role, real-world project experience is critical.
That’s why our SAP HANA course in Pune is specifically designed to bridge the gap between academic learning and real-world application. This blog explores exactly how our course equips learners with the hands-on skills, problem-solving capabilities, and practical insights needed to confidently tackle live projects using SAP HANA.
1. A Curriculum Built Around Real Business Needs
Unlike generic training programs that focus heavily on theory, our SAP HANA course has been designed in consultation with industry experts and SAP-certified professionals. The course structure aligns closely with business processes and challenges that professionals face on the ground.
From day one, learners are introduced to realistic use cases, such as:
Data modeling for large enterprises
Real-time data processing scenarios
Performance tuning for transactional systems
Predictive analytics for customer behavior
2. Project-Based Learning Modules
One of the most powerful components of our SAP HANA course is the project-based learning approach. Every major topic culminates in a mini-project that simulates actual business challenges. Here are a few examples:
Sales Reporting System: Build a HANA model that tracks real-time sales across multiple regions and products.
HR Analytics Dashboard: Develop a solution to analyze employee attrition, hiring trends, and performance metrics using SAP HANA views.
Inventory Management: Design a system that automates stock-level checks and forecasting using SAP HANA’s advanced analytics.
3. Real-Time Data Integration Training
One of SAP HANA’s core strengths is its ability to process data in real time. Our course emphasizes this by including live data integration tasks where students:
Use SAP SLT (System Landscape Transformation) replication to sync data from SAP ERP to HANA
Connect HANA to third-party data sources (e.g., Excel, flat files, or web services)
Set up and manage data pipelines using Smart Data Integration (SDI) tools
4. Exposure to SAP HANA Studio and Web IDE
Working professionals in SAP environments rely on tools like SAP HANA Studio and SAP Web IDE for development and administration. Our course includes guided labs on how to:
Create calculation views using graphical and SQL scripting
Perform schema-level administration tasks
Monitor system performance and manage memory
Debug and optimize SQL queries
5. Performance Optimization Techniques
It’s not just about building solutions—it’s about building efficient, scalable, and performant systems. Our SAP HANA course includes a dedicated section on performance optimization, where learners explore:
Indexing and partitioning strategies
Query optimization
Memory management and CPU usage
Best practices in HANA data modeling
6. Collaboration and Team-Based Project Work
In the real world, SAP professionals rarely work in silos. Team collaboration is essential, especially in agile or DevOps-driven environments. To mirror this, our course includes team-based capstone projects that require learners to:
Collaborate using shared repositories
Divide responsibilities between developers, modelers, and admins
Present their solution and rationale to a review panel
7. Simulated Client Requirements and Feedback Loops
To make the course even more realistic, we introduce client-like personas and simulate business requirement documents (BRDs). Students receive evolving requirements, just like in a real-world project, and must:
Analyze and clarify needs
Create technical specifications
Iterate on their solution based on mock stakeholder feedback
8. Certification & Job Preparation
While experience is key, certifications still matter in the SAP ecosystem. Our SAP HANA course includes preparation for official SAP certification exams such as:
SAP Certified Application Associate – SAP HANA 2.0 (SPS05)
SAP Certified Technology Associate – SAP HANA 2.0
9. Industry-Relevant Case Studies
We’ve embedded real industry case studies into the course so that learners can see how SAP HANA is being used across:
Retail: Real-time inventory and POS analytics
Healthcare: Patient data integration and predictive care
Banking: Fraud detection and risk assessment
Manufacturing: Supply chain and operations optimization
Final Thoughts
SAP HANA is a game-changer in the enterprise data world, but to truly harness its potential, professionals need more than theoretical knowledge. They need hands-on, real-world experience.
Our SAP HANA course delivers exactly that. By blending project-based learning, tool familiarity, industry case studies, and certification prep, we ensure that learners graduate not just knowing SAP HANA but knowing how to use it to solve real business problems.
Whether you're an IT professional looking to upskill or a newcomer breaking into the SAP ecosystem, our course will prepare you to hit the ground running in any SAP HANA project.
0 notes
Text
How to Improve Database Performance with Smart Optimization Techniques
Database performance is critical to the efficiency and responsiveness of any data-driven application. As data volumes grow and user expectations rise, ensuring your database runs smoothly becomes a top priority. Whether you're managing an e-commerce platform, financial software, or enterprise systems, sluggish database queries can drastically hinder user experience and business productivity.
In this guide, we’ll explore practical and high-impact strategies to improve database performance, reduce latency, and increase throughput.
1. Optimize Your Queries
Poorly written queries are one of the most common causes of database performance issues. Avoid using SELECT * when you only need specific columns. Analyze query execution plans to understand how data is being retrieved and identify potential inefficiencies.
Use indexed columns in WHERE, JOIN, and ORDER BY clauses to take full advantage of the database indexing system.
2. Index Strategically
Indexes are essential for speeding up data retrieval, but too many indexes can hurt write performance and consume excessive storage. Prioritize indexing on columns used in search conditions and join operations. Regularly review and remove unused or redundant indexes.
3. Implement Connection Pooling
Connection pooling allows multiple application users to share a limited number of database connections. This reduces the overhead of opening and closing connections repeatedly, which can significantly improve performance, especially under heavy load.
4. Cache Frequently Accessed Data
Use caching layers to avoid unnecessary hits to the database. Frequently accessed and rarely changing data—such as configuration settings or product catalogs—can be stored in in-memory caches like Redis or Memcached. This reduces read latency and database load.
5. Partition Large Tables
Partitioning splits a large table into smaller, more manageable pieces without altering the logical structure. This improves performance for queries that target only a subset of the data. Choose partitioning strategies based on date, region, or other logical divisions relevant to your dataset.
6. Monitor and Tune Regularly
Database performance isn’t a one-time fix—it requires continuous monitoring and tuning. Use performance monitoring tools to track query execution times, slow queries, buffer usage, and I/O patterns. Adjust configurations and SQL statements accordingly to align with evolving workloads.
7. Offload Reads with Replication
Use read replicas to distribute query load, especially for read-heavy applications. Replication allows you to spread read operations across multiple servers, freeing up the primary database to focus on write operations and reducing overall latency.
8. Control Concurrency and Locking
Poor concurrency control can lead to lock contention and delays. Ensure your transactions are short and efficient. Use appropriate isolation levels to avoid unnecessary locking, and understand the impact of each level on performance and data integrity.
0 notes
Text
Best Laravel Development Services for Fintech App Security & Speed
In 2025, the fintech sector is booming like never before. From digital wallets and neobanks to loan management systems and investment platforms, the demand for secure, fast, and scalable applications is skyrocketing. Behind many of these high-performing platforms lies one key technology: Laravel development services.
Laravel is a PHP-based web framework known for its elegant syntax, built-in security features, and flexibility. It has quickly become a go-to solution for fintech companies looking to build robust and future-ready apps.
In this blog, we’ll dive deep into why Laravel development services are the best choice for fintech applications, especially when it comes to security and speed. We’ll also answer key FAQs to help you make an informed decision.
Why Laravel is the Smart Choice for Fintech Development
1. Bank-Grade Security
Security is non-negotiable in fintech. Laravel offers features like:
CSRF protection
Encrypted password hashing (Bcrypt and Argon2)
SQL injection prevention
Two-factor authentication integrations
Secure session handling
When you hire expert Laravel development services, you ensure that your fintech app is guarded against common cyber threats and vulnerabilities.
2. Speed & Performance Optimization
In fintech, milliseconds matter. Laravel is designed for high performance. With features like:
Built-in caching with Redis or Memcached
Lazy loading of data
Queues for background processing
Lightweight Blade templating engine
Laravel apps are optimized to run fast and efficiently, even with complex data and multiple users.
3. Modular & Scalable Structure
Fintech startups need to evolve quickly. Laravel’s modular architecture allows developers to add new features without rewriting the whole app. Need to add payment gateways, KYC verification, or investment tracking? Laravel makes it easier and more maintainable.
4. API-Ready Backend
Most fintech apps need strong API support for mobile apps, third-party services, or internal dashboards. Laravel offers:
RESTful routing
API authentication with Laravel Sanctum or Passport
Seamless data exchange in JSON format
This makes Laravel development services ideal for creating flexible, API-first applications.
5. Developer Ecosystem & Community
Laravel has one of the strongest developer communities, which means:
Quick access to pre-built packages (e.g., for payments, SMS alerts, OTP login)
Frequent updates and support
Access to Laravel Nova, Horizon, and Echo for admin panels, job queues, and real-time data respectively
This helps fintech businesses reduce time-to-market and focus on innovation.
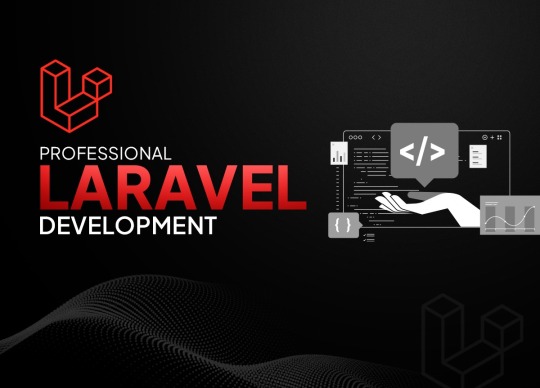
Real-World Use Case: Laravel in Fintech
A Canadian lending startup partnered with a Laravel development services provider to build a loan origination platform. The app included borrower onboarding, KYC checks, EMI tracking, and real-time risk analysis. Using Laravel:
The app handled over 10,000 users in the first 3 months.
Page load times were under 1 second even during peak hours.
The system passed a third-party penetration test with zero critical vulnerabilities.
Key Laravel Features That Fintech Businesses Love
Feature
Why It Matters for Fintech
Blade Templates
Speeds up frontend UI without complex JS
Laravel Sanctum
Easy API token management for mobile apps
Laravel Queue System
Handles transactions, notifications in background
Migration System
Helps keep track of database changes easily
Test Automation Support
Essential for secure and bug-free releases
How to Choose the Right Laravel Development Services
Here are 5 tips to find the best Laravel team for your fintech project:
Check for Security Expertise: Ask how they handle encryption, SSL, and data privacy.
Look for Fintech Experience: Have they built apps in finance, banking, or insurance?
Ask About Performance Tuning: Do they use Redis, CDN, or job queues?
Review Client Testimonials: Look for real business results and successful launches.
Support & Maintenance: Fintech apps need ongoing updates. Make sure they offer it.
FAQs: Laravel Development Services for Fintech
Q1: Can Laravel handle sensitive financial data securely?
Yes. Laravel offers built-in tools for encryption, secure session handling, and protection against OWASP top 10 vulnerabilities. Many fintech platforms successfully use Laravel.
Q2: Is Laravel fast enough for real-time fintech applications?
Absolutely. With caching, queues, and efficient routing, Laravel delivers low-latency responses. For real-time data (like trading apps), Laravel Echo and WebSockets can be used.
Q3: Can Laravel be used for mobile fintech apps?
Yes. Laravel is commonly used as a backend for mobile apps (using Flutter, React Native, or native frameworks). Laravel APIs are easy to connect with mobile frontends.
Final Thoughts
In the fintech world, the margin for error is razor-thin. Security breaches or slow load times can lead to user loss and legal trouble. That’s why choosing the right tech stack and more importantly, the right development team is crucial.
With Laravel, you get a framework that’s powerful, secure, and scalable. By partnering with professional Laravel development services, fintech companies can:
Launch secure and lightning-fast apps
Stay compliant with global standards
Scale features and users effortlessly
Beat the competition in speed and reliability
So, if you're planning to build or upgrade your fintech platform in 2025, now is the perfect time to invest in trusted Laravel development services.
0 notes
Text

Oracle Online Training
Croma Campus offers comprehensive Oracle Online Training designed for beginners and professionals. The course covers key Oracle concepts, including database management, SQL, PL/SQL, and performance tuning. With expert trainers, flexible schedules, and hands-on projects, learners gain real-world skills to excel in database administration and Oracle development roles.
0 notes
Text
The Hidden Hero of Software Success: Inside EDSPL’s Unmatched Testing & QA Framework

When a software product goes live without glitches, users often marvel at its speed, design, or functionality. What they don’t see is the invisible layer of discipline, precision, and strategy that made it possible — Testing and Quality Assurance (QA). At EDSPL, QA isn’t just a step in the process; it’s the very spine that supports software integrity from start to finish.
As digital applications grow more interconnected, especially with advancements in network security, cloud security, application security, and infrastructure domains like routing, switching, and mobility, quality assurance becomes the glue holding it all together. EDSPL’s comprehensive QA and testing framework has been fine-tuned to ensure consistent performance, reliability, and security — no matter how complex the software environment.
Let’s go behind the scenes of EDSPL’s QA approach to understand why it is a hidden hero in modern software success.
Why QA Is More Crucial Than Ever
The software ecosystem is no longer siloed. Enterprises now rely on integrated systems that span cloud platforms, APIs, mobile devices, and legacy systems — all of which need to work in sync without error.
From safeguarding sensitive data through network security protocols to validating business-critical workflows on the cloud, EDSPL ensures that testing extends beyond functionality. It is a guardrail for security, compliance, performance, and user trust.
Without rigorous QA, a minor bug in a login screen could lead to a vulnerability that compromises an entire system. EDSPL prevents these catastrophes by placing QA at the heart of its delivery model.
QA Touchpoints Across EDSPL’s Service Spectrum
Let’s explore how EDSPL’s testing excellence integrates into different service domains.
1. Ensuring Safe Digital Highways through Network Security
In an era where cyber threats can cripple operations, QA isn’t just about validating code — it’s about verifying that security holds up under stress. EDSPL incorporates penetration testing, vulnerability assessments, and simulation-based security testing into its QA model to validate:
Firewall behavior
Data leakage prevention
Encryption mechanisms
Network segmentation efficacy
By integrating QA with network security, EDSPL ensures clients launch digitally fortified applications.
2. Reliable Application Delivery on the Cloud
Cloud-native and hybrid applications are central to enterprise growth, but they also introduce shared responsibility models. EDSPL’s QA ensures that deployment across cloud platforms is:
Secure from misconfigurations
Optimized for performance
Compliant with governance standards
Whether it’s AWS, Azure, or GCP, EDSPL’s QA framework validates data access policies, scalability limits, and containerized environments. This ensures smooth delivery across the cloud with airtight cloud security guarantees.
3. Stress-Testing Application Security
Modern applications are constantly exposed to APIs, users, and third-party integrations. EDSPL includes robust application security testing as part of QA by simulating real-world attacks and identifying:
Cross-site scripting (XSS) vulnerabilities
SQL injection points
Broken authentication scenarios
API endpoint weaknesses
By using both manual and automated testing methods, EDSPL ensures applications are resilient to threat vectors and function smoothly across platforms.
4. Validating Enterprise Network Logic through Routing and Switching
Routing and switching are the operational backbone of any connected system. When software solutions interact with infrastructure-level components, QA plays a key role in ensuring:
Data packets travel securely and efficiently
VLANs are correctly configured
Dynamic routing protocols function without interruption
Failover and redundancy mechanisms are effective
EDSPL’s QA team uses emulators and simulation tools to test against varied network topologies and configurations. This level of QA ensures that software remains robust across different environments.
5. Securing Agile Teams on the Move with Mobility Testing
With a growing mobile workforce, enterprise applications must be optimized for mobile-first use cases. EDSPL’s QA team conducts deep mobility testing that includes:
Device compatibility across Android/iOS
Network condition simulation (3G/4G/5G/Wi-Fi)
Real-time responsiveness
Security over public networks
Mobile-specific security testing (root detection, data sandboxing, etc.)
This ensures that enterprise mobility solutions are secure, efficient, and universally accessible.
6. QA for Integrated Services
At its core, EDSPL offers an integrated suite of IT and software services. QA is embedded across all of them — from full-stack development to API design, cloud deployment, infrastructure automation, and cybersecurity.
Key QA activities include:
Regression testing for evolving features
Functional and integration testing across service boundaries
Automation testing to reduce human error
Performance benchmarking under realistic conditions
Whether it's launching a government portal or a fintech app, EDSPL's services rely on QA to deliver dependable digital experiences.
The QA Framework: Built for Resilience and Speed
EDSPL has invested in building a QA framework that balances speed with precision. Here's what defines it:
1. Shift-Left Testing
QA begins during requirements gathering, not after development. This reduces costs, eliminates rework, and aligns product strategy with user needs.
2. Continuous Integration & Automated Testing
Automation tools are deeply integrated with CI/CD pipelines to support agile delivery. Tests run with every commit, giving developers instant feedback and reducing deployment delays.
3. Security-First QA Culture
Security checks are integrated into every QA cycle, not treated as separate audits. This creates a proactive defense mechanism and encourages developers to write secure code from day one.
4. Test Data Management
EDSPL uses production-simulated datasets to ensure test scenarios reflect real-world user behavior. This improves defect prediction and minimizes surprises post-launch.
5. Reporting & Metrics
QA results are analyzed using KPIs like defect leakage rate, test coverage, mean time to resolve, and user-reported issue rates. These metrics drive continuous improvement.
Case Studies: Impact Through Quality
A National Education Platform
EDSPL was tasked with launching a high-traffic education portal with live video, assessments, and resource sharing. The QA team created an end-to-end test architecture including performance, usability, and application security testing.
Results:
99.9% uptime during national rollout
Zero critical issues in the first 90 days
100K+ concurrent users supported with no lag
A Banking App with Cloud-Native Architecture
A private bank chose EDSPL for QA on a mobile app deployed on the cloud. The QA team validated the app’s security posture, cloud security, and resilience under high load.
Results:
Passed all OWASP compliance checks
Load testing confirmed 5000+ concurrent sessions
Automated testing reduced release cycles by 40%
Future-Ready QA: AI, RPA, and Autonomous Testing
EDSPL’s QA roadmap includes:
AI-based test generation from user behavior patterns
Self-healing automation for flaky test cases
RPA integration for business process validation
Predictive QA using machine learning to forecast defects
These capabilities ensure that EDSPL’s QA framework not only adapts to today’s demands but also evolves with future technologies.
Conclusion: Behind Every Great Software Is Greater QA
While marketing, development, and design get much of the spotlight, software success is impossible without a strong QA foundation. At EDSPL, testing is not a checkbox — it’s a commitment to excellence, safety, and performance.
From network security to cloud security, from routing to mobility, QA is integrated into every layer of the digital infrastructure. It is the thread that ties all services together into a reliable, secure, and scalable product offering.
When businesses choose EDSPL, they’re not just buying software — they’re investing in peace of mind, powered by an unmatched QA framework.
Visit this website to know more — https://www.edspl.net/
0 notes