#tpu cutting machine
Explore tagged Tumblr posts
Text
THROWBACK SUNDAY
18/8/24 The Olympics have come and gone. The highlight of the summer, there was enormous amount of space and connection in both opening ceremonies torch and closing ceremonies medals.
Last year Google posted a video reel of hits based on most frequent searches on its X page. various moments ranging from sports to pop music to protests and peace-advocates made the cut.
Today in sifting through the grain of consumer-apps, we rely on the unobtrusive Play Store. Google understood the mass appeal of social media and mobile support early on, and figured out service models like *GoogleTalk, *Google+ and *V8, all native to Android TM.
● KEEPING DATA TO OURSELVES?
With expansion of machine learning services ( including TENSOR processing unit upgrade and COLAB integration ) there will be unprecedented interest in protecting data from "scrapers".
This interest is in opposition to one of the founding principles of Google, to take the world's knowledge and make it universally accessible. Libraries are taking down books in response to complaints, and even Archive.COM reports losing its legal claim to 500,000 published works, all because the publishers vowed to 'secure' those titles.
● MIDDLECLASS TAX DOLLARS VS MULTIMODAL AGENTS
For better or worse this panic: over A.I. getting in touch with source data, and training on looks, poems, or speech style IS REAL. Pretty much every 12 weeks new models are rolled out and leaderboards construct new tests / build better red teams.
Google, being a W3C leader: is heading the efforts to 'fix the environment' by requiring guardrails in the form of digital watermarks . Second, encouraging banning of bad actors, angry scrapers going behind people's backs just to gain access into their private data. Apple is also motivated to redeem their brand from security flaws either through monitoring or misinformation prone apps.
We grew up with an /Internet which might have been slow, but for the most part there was a reliability in security /protocols and /antiviruses. However, our children will have to cope with a deluge of generated ads, artificial scripts and comments.
2 notes
·
View notes
Text
Top AI Frameworks in 2025: How to Choose the Best Fit for Your Project
AI Development: Your Strategic Framework Partner

1. Introduction: Navigating the AI Framework Landscape
The world of artificial intelligence is evolving at breakneck speed. What was cutting-edge last year is now foundational, and new advancements emerge almost daily. This relentless pace means the tools we use to build AI — the AI frameworks — are also constantly innovating. For software developers, AI/ML engineers, tech leads, CTOs, and business decision-makers, understanding this landscape is paramount.
The Ever-Evolving World of AI Development
From sophisticated large language models (LLMs) driving new generative capabilities to intricate computer vision systems powering autonomous vehicles, AI applications are becoming more complex and pervasive across every industry. Developers and businesses alike are grappling with how to harness this power effectively, facing challenges in scalability, efficiency, and ethical deployment. At the heart of building these intelligent systems lies the critical choice of the right AI framework.
Why Choosing the Right Framework Matters More Than Ever
In 2025, selecting an AI framework isn't just a technical decision; it's a strategic one that can profoundly impact your project's trajectory. The right framework can accelerate development cycles, optimize model performance, streamline deployment processes, and ultimately ensure your project's success and ROI. Conversely, a poor or ill-suited choice can lead to significant bottlenecks, increased development costs, limited scalability, and missed market opportunities. Understanding the current landscape of AI tools and meticulously aligning your choice with your specific project needs is crucial for thriving in the competitive world of AI development.
2. Understanding AI Frameworks: The Foundation of Intelligent Systems

Before we dive into the top contenders of AI frameworks in 2025, let's clarify what an AI framework actually is and why it's so fundamental to building intelligent applications.
What Exactly is an AI Framework?
An AI framework is essentially a comprehensive library or platform that provides a structured set of pre-built tools, libraries, and functions. Its primary purpose is to make developing machine learning (ML) and deep learning (DL) models easier, faster, and more efficient. Think of it as a specialized, high-level toolkit for AI development. Instead of coding every complex mathematical operation, algorithm, or neural network layer from scratch, developers use these frameworks to perform intricate tasks with just a few lines of code, focusing more on model architecture and data.
Key Components and Core Functions
Most AI frameworks come equipped with several core components that underpin their functionality:
Automatic Differentiation: This is a fundamental capability, particularly critical for training deep learning frameworks. It enables the efficient calculation of gradients, which are essential for how neural networks learn from data.
Optimizers: These are algorithms that adjust model parameters (weights and biases) during training to minimize errors and improve model performance. Common examples include Adam, SGD, and RMSprop.
Neural Network Layers: Frameworks provide ready-to-use building blocks (e.g., convolutional layers for image processing, recurrent layers for sequential data, and dense layers) that can be easily stacked and configured to create complex neural network architectures.
Data Preprocessing Tools: Utilities within frameworks simplify the often complex tasks of data cleaning, transformation, augmentation, and loading, ensuring data is in the right format for model training.
Model Building APIs: High-level interfaces allow developers to define, train, evaluate, and save their models with relatively simple and intuitive code.
GPU/TPU Support: Crucially, most modern AI frameworks are optimized to leverage specialized hardware like Graphics Processing Units (GPUs) and Tensor Processing Units (TPUs) for parallel computation, dramatically accelerating the computationally intensive process of deep learning model training.
The Role of Frameworks in Streamlining AI Development
AI frameworks play a pivotal role in streamlining the entire AI development process. They standardize workflows, abstract away low-level programming complexities, and provide a collaborative environment for teams. Specifically, they enable developers to:
Faster Prototyping: Quickly test and refine ideas by assembling models from pre-built components, accelerating the experimentation phase.
Higher Efficiency: Significantly reduce development time and effort by reusing optimized, built-in tools and functions rather than recreating them.
Scalability: Build robust models that can effectively handle vast datasets and scale efficiently for deployment in production environments.
Team Collaboration: Provide a common language, set of tools, and established best practices that streamline teamwork and facilitate easier project handover.
3. The Leading AI Frameworks in 2025: A Deep Dive

The AI development landscape is dynamic, with continuous innovation. However, several AI frameworks have solidified their positions as industry leaders by 2025, each possessing unique strengths and catering to specific ideal use cases.
TensorFlow: Google's Enduring Giant
TensorFlow, developed by Google, remains one of the most widely adopted deep learning frameworks, especially in large-scale production environments.
Key Features & Strengths:
Comprehensive Ecosystem: Boasts an extensive ecosystem, including TensorFlow Lite (for mobile and edge devices), TensorFlow.js (for web-based ML), and TensorFlow Extended (TFX) for end-to-end MLOps pipelines.
Scalable & Production-Ready: Designed from the ground up for massive computational graphs and robust deployment in enterprise-level solutions.
Great Visuals: TensorBoard offers powerful visualization tools for monitoring training metrics, debugging models, and understanding network architectures.
Versatile: Highly adaptable for a wide range of ML tasks, from academic research to complex, real-world production applications.
Ideal Use Cases: Large-scale enterprise AI solutions, complex research projects requiring fine-grained control, production deployment of deep learning models, mobile and web AI applications, and MLOps pipeline automation.
PyTorch: The Research & Flexibility Champion
PyTorch, developed by Meta (formerly Facebook) AI Research, has become the preferred choice in many academic and research communities, rapidly gaining ground in production.
Key Features & Strengths:
Flexible Debugging: Its dynamic computation graph (known as "define-by-run") makes debugging significantly easier and accelerates experimentation.
Python-Friendly: Its deep integration with the Python ecosystem and intuitive API makes it feel natural and accessible to Python developers, contributing to a smoother learning curve for many.
Research-Focused: Widely adopted in academia and research for its flexibility, allowing for rapid prototyping of novel architectures and algorithms.
Production-Ready: Has significantly matured in production capabilities with tools like PyTorch Lightning for streamlined training and TorchServe for model deployment.
Ideal Use Cases: Rapid prototyping, advanced AI research, projects requiring highly customized models and complex neural network architectures, and startups focused on quick iteration and experimentation.
JAX: Google's High-Performance Differentiable Programming
JAX, also from Google, is gaining substantial traction for its powerful automatic differentiation and high-performance numerical computation capabilities, particularly in cutting-edge research.
Key Features & Strengths:
Advanced Autodiff: Offers highly powerful and flexible automatic differentiation, not just for scalars but for vectors, matrices, and even higher-order derivatives.
XLA Optimized: Leverages Google's Accelerated Linear Algebra (XLA) compiler for extreme performance optimization and efficient execution on GPUs and TPUs.
Composable Functions: Enables easy composition of functional transformations like grad (for gradients), jit (for just-in-time compilation), and vmap (for automatic vectorization) to create highly optimized and complex computations.
Research-Centric: Increasingly popular in advanced AI research for exploring novel AI architectures and training massive models.
Ideal Use Cases: Advanced AI research, developing custom optimizers and complex loss functions, high-performance computing, exploring novel AI architectures, and training deep learning models on TPUs where maximum performance is critical.
Keras (with TensorFlow/JAX/PyTorch backend): Simplicity Meets Power
Keras is a high-level API designed for fast experimentation with deep neural networks. Its strength lies in its user-friendliness and ability to act as an interface for other powerful deep learning frameworks.
Key Features & Strengths:
Beginner-Friendly: Offers a simple, intuitive, high-level API, making it an excellent entry point for newcomers to deep learning.
Backend Flexibility: Can run seamlessly on top of TensorFlow, JAX, or PyTorch, allowing developers to leverage the strengths of underlying frameworks while maintaining Keras's ease of use.
Fast Prototyping: Its straightforward design is ideal for quickly building, training, and testing models.
Easy Experimentation: Its intuitive design supports rapid development cycles and iterative model refinement.
Ideal Use Cases: Quick model building and iteration, educational purposes, projects where rapid prototyping is a priority, and developers who prefer a high-level abstraction to focus on model design rather than low-level implementation details.
Hugging Face Transformers (Ecosystem, not just a framework): The NLP Powerhouse
While not a standalone deep learning framework itself, the Hugging Face Transformers library, along with its broader ecosystem (Datasets, Accelerate, etc.), has become indispensable for Natural Language Processing (NLP) and Large Language Model (LLM) AI development.
Key Features & Strengths:
Huge Library of Pre-trained Models: Offers an enormous collection of state-of-the-art pre-trained models for NLP, computer vision (CV), and audio tasks, making it easy to leverage cutting-edge research.
Unified, Framework-Agnostic API: Provides a consistent interface for using various models, compatible with TensorFlow, PyTorch, and JAX.
Strong Community & Documentation: A vibrant community and extensive, clear documentation make it exceptionally easy to get started and find solutions for complex problems.
Ideal Use Cases: Developing applications involving NLP tasks (text generation, sentiment analysis, translation, summarization), fine-tuning and deploying custom LLM applications, or leveraging pre-trained models for various AI tasks with minimal effort.
Scikit-learn: The Machine Learning Workhorse
Scikit-learn is a foundational machine learning framework for traditional ML algorithms, distinct from deep learning but critical for many data science applications.
Key Features & Strengths:
Extensive Classic ML Algorithms: Offers a wide array of battle-tested traditional machine learning algorithms for classification, regression, clustering, dimensionality reduction, model selection, and preprocessing.
Simple API, Strong Python Integration: Known for its user-friendly, consistent API and seamless integration with Python's scientific computing stack (NumPy, SciPy, Matplotlib).
Excellent Documentation: Provides comprehensive and easy-to-understand documentation with numerous examples.
Ideal Use Cases: Traditional machine learning tasks, data mining, predictive analytics on tabular data, feature engineering, statistical modeling, and projects where deep learning is not required or feasible.
4. Beyond the Hype: Critical Factors for Choosing Your AI Framework

Choosing the "best" AI framework isn't about picking the most popular one; it's about selecting the right fit for your AI project. Here are the critical factors that CTOs, tech leads, and developers must consider to make an informed decision:
Project Requirements & Scope
Type of AI Task: Different frameworks excel in specific domains. Are you working on Computer Vision (CV), Natural Language Processing (NLP), Time Series analysis, reinforcement learning, or traditional tabular data?
Deployment Scale: Where will your model run? On a small edge device, a mobile phone, a web server, or a massive enterprise cloud infrastructure? The framework's support for various deployment targets is crucial.
Performance Needs: Does your application demand ultra-low latency, high throughput (processing many requests quickly), or efficient memory usage? Benchmarking and framework optimization capabilities become paramount.
Community & Ecosystem Support
Documentation and Tutorials: Are there clear, comprehensive guides, tutorials, and examples available to help your team get started and troubleshoot issues?
Active Developer Community & Forums: A strong, vibrant community means more shared knowledge, faster problem-solving, and continuous improvement of the framework.
Available Pre-trained Models & Libraries: Access to pre-trained models (like those from Hugging Face) and readily available libraries for common tasks can drastically accelerate development time.
Learning Curve & Team Expertise
Onboarding: How easily can new team members learn the framework's intricacies and become productive contributors to the AI development effort?
Existing Skills: Does the framework align well with your team's current expertise in Python, specific mathematical concepts, or other relevant technologies? Leveraging existing knowledge can boost efficiency.
Flexibility & Customization
Ease of Debugging and Experimentation: A flexible framework allows for easier iteration, understanding of model behavior, and efficient debugging, which is crucial for research and complex AI projects.
Support for Custom Layers and Models: Can you easily define and integrate custom neural network layers or entirely new model architectures if your AI project requires something unique or cutting-edge?
Integration Capabilities
Compatibility with Existing Tech Stack: How well does the framework integrate with your current programming languages, databases, cloud providers, and existing software infrastructure? Seamless integration saves development time.
Deployment Options: Does the framework offer clear and efficient pathways for deploying your trained models to different environments (e.g., mobile apps, web services, cloud APIs, IoT devices)?
Hardware Compatibility
GPU/TPU Support and Optimization: For deep learning frameworks, efficient utilization of specialized hardware like GPUs and TPUs is paramount for reducing training time and cost. Ensure the framework offers robust and optimized support for the hardware you plan to use.
Licensing and Commercial Use Considerations
Open-source vs. Proprietary Licenses: Most leading AI frameworks are open-source (e.g., Apache 2.0, MIT), offering flexibility. However, always review the specific license to ensure it aligns with your commercial use case and intellectual property requirements.
5. Real-World Scenarios: Picking the Right Tool for the Job
Let's look at a few common AI project scenarios and which AI frameworks might be the ideal fit, considering the factors above:
Scenario 1: Rapid Prototyping & Academic Research
Best Fit: PyTorch, Keras (with any backend), or JAX. Their dynamic graphs (PyTorch) and high-level APIs (Keras) allow for quick iteration, experimentation, and easier debugging, which are crucial in research settings. JAX is gaining ground here for its power and flexibility in exploring novel architectures.
Scenario 2: Large-Scale Enterprise Deployment & Production
Best Fit: TensorFlow or PyTorch (with production tools like TorchServe/Lightning). TensorFlow's robust ecosystem (TFX, SavedModel format) and emphasis on scalability make it a strong contender. PyTorch's production readiness has also significantly matured, making it a viable choice for large-scale AI development and deployment.
Scenario 3: Developing a Custom NLP/LLM Application
Best Fit: Hugging Face Transformers (running on top of PyTorch or TensorFlow). This ecosystem provides the fastest way to leverage and fine-tune state-of-the-art large language models (LLMs), significantly reducing AI development time and effort. Its vast collection of pre-trained models is a game-changer for AI tools in NLP.
Scenario 4: Building Traditional Machine Learning Models
Best Fit: Scikit-learn. For tasks like classification, regression, clustering, and data preprocessing on tabular data, Scikit-learn remains the industry standard. Its simplicity, efficiency, and comprehensive algorithm library make it the go-to machine learning framework for non-deep learning applications.
6. Conclusion: The Strategic Imperative of Informed Choice
In 2025, the proliferation of AI frameworks offers incredible power and flexibility to organizations looking to implement AI solutions. However, it also presents a significant strategic challenge. The dynamic nature of these AI tools means continuous learning and adaptation are essential for developers and businesses alike to stay ahead in the rapidly evolving AI development landscape.
Investing in the right AI framework is about more than just following current 2025 AI trends; it's about laying a solid foundation for your future success in the AI-driven world. An informed choice minimizes technical debt, maximizes developer productivity, and ultimately ensures your AI projects deliver tangible business value and a competitive edge.
Navigating this complex landscape, understanding the nuances of each deep learning framework, and selecting the optimal AI framework for your unique requirements can be daunting. If you're looking to leverage AI to revolutionize your projects, optimize your AI development process, or need expert guidance in selecting and implementing the best AI tools, consider partnering with an experienced AI software development company. We can help you build intelligent solutions tailored to your specific needs, ensuring you pick the perfect fit for your AI project and thrive in the future of AI.
#ai gpt chat#open ai chat bot#ai talking#chat ai online#ai chat bot online#google chat bot#google ai chat#chat ai free#gpt chat ai#free ai chat bot#best ai chat#chat bot gpt#ai chat online free#chat ai bot#conversational ai chatbot#chat gpt chatbot#cognigy ai#gpt ai chat#google chat ai#best chat bot#ai chat bot free#best ai chat bot#ai chat website#chat ai google#ai open chat#conversational chatbot#chat gpt bot#bot ai chat#ai chat bot for website#new chat ai
0 notes
Text
Exascale Computing Market Size, Share, Analysis, Forecast, and Growth Trends to 2032: The Race to One Quintillion Calculations Per Second

The Exascale Computing Market was valued at USD 3.47 billion in 2023 and is expected to reach USD 29.58 billion by 2032, growing at a CAGR of 26.96% from 2024-2032.
The Exascale Computing Market is undergoing a profound transformation, unlocking unprecedented levels of computational performance. With the ability to process a billion billion (quintillion) calculations per second, exascale systems are enabling breakthroughs in climate modeling, genomics, advanced materials, and national security. Governments and tech giants are investing aggressively, fueling a race for exascale dominance that’s reshaping industries and redefining innovation timelines.
Exascale Computing Market revolutionary computing paradigm is being rapidly adopted across sectors seeking to harness the immense data-crunching potential. From predictive simulations to AI-powered discovery, exascale capabilities are enabling new frontiers in science, defense, and enterprise. Its impact is now expanding beyond research labs into commercial ecosystems, paving the way for smarter infrastructure, precision medicine, and real-time global analytics.
Get Sample Copy of This Report: https://www.snsinsider.com/sample-request/6035
Market Keyplayers:
Hewlett Packard Enterprise (HPE) [HPE Cray EX235a, HPE Slingshot-11]
International Business Machines Corporation (IBM) [IBM Power System AC922, IBM Power System S922LC]
Intel Corporation [Intel Xeon Max 9470, Intel Max 1550]
NVIDIA Corporation [NVIDIA GH200 Superchip, NVIDIA Hopper H100]
Cray Inc. [Cray EX235a, Cray EX254n]
Fujitsu Limited [Fujitsu A64FX, Tofu interconnect D]
Advanced Micro Devices, Inc. (AMD) [AMD EPYC 64C 2.0GHz, AMD Instinct MI250X]
Lenovo Group Limited [Lenovo ThinkSystem SD650 V3, Lenovo ThinkSystem SR670 V2]
Atos SE [BullSequana XH3000, BullSequana XH2000]
NEC Corporation [SX-Aurora TSUBASA, NEC Vector Engine]
Dell Technologies [Dell EMC PowerEdge XE8545, Dell EMC PowerSwitch Z9332F]
Microsoft [Microsoft Azure NDv5, Microsoft Azure HPC Cache]
Amazon Web Services (AWS) [AWS Graviton3, AWS Nitro System]
Sugon [Sugon TC8600, Sugon I620-G30]
Google [Google TPU v4, Google Cloud HPC VM]
Alibaba Cloud [Alibaba Cloud ECS Bare Metal Instance, Alibaba Cloud HPC Cluster]
Market Analysis The exascale computing landscape is characterized by high-stakes R&D, global governmental collaborations, and fierce private sector competition. With countries like the U.S., China, and members of the EU launching national initiatives, the market is shaped by a mix of geopolitical strategy and cutting-edge technology. Key players are focusing on developing energy-efficient architectures, innovative software stacks, and seamless integration with artificial intelligence and machine learning platforms. Hardware giants are partnering with universities, startups, and defense organizations to accelerate deployments and overcome system-level challenges such as cooling, parallelism, and power consumption.
Market Trends
Surge in demand for high-performance computing in AI and deep learning
Integration of exascale systems with cloud and edge computing ecosystems
Government funding and national strategic investments on the rise
Development of heterogeneous computing systems (CPUs, GPUs, accelerators)
Emergence of quantum-ready hybrid systems alongside exascale architecture
Adoption across healthcare, aerospace, energy, and climate research sectors
Market Scope
Supercomputing for Scientific Discovery: Empowering real-time modeling and simulations at unprecedented speeds
Defense and Intelligence Advancements: Enhancing cybersecurity, encryption, and strategic simulations
Precision Healthcare Applications: Supporting drug discovery, genomics, and predictive diagnostics
Sustainable Energy Innovations: Enabling complex energy grid management and fusion research
Smart Cities and Infrastructure: Driving intelligent urban planning, disaster management, and IoT integration
As global industries shift toward data-driven decision-making, the market scope of exascale computing is expanding dramatically. Its capacity to manage and interpret massive datasets in real-time is making it essential for competitive advantage in a rapidly digitalizing world.
Market Forecast The trajectory of the exascale computing market points toward rapid scalability and broader accessibility. With increasing collaborations between public and private sectors, we can expect a new wave of deployments that bridge research and industry. The market is moving from proof-of-concept to full-scale operationalization, setting the stage for widespread adoption across diversified verticals. Upcoming innovations in chip design, power efficiency, and software ecosystems will further accelerate this trend, creating a fertile ground for startups and enterprise adoption alike.
Access Complete Report: https://www.snsinsider.com/reports/exascale-computing-market-6035
Conclusion Exascale computing is no longer a vision of the future—it is the powerhouse of today’s digital evolution. As industries align with the pace of computational innovation, those embracing exascale capabilities will lead the next wave of transformation. With its profound impact on science, security, and commerce, the exascale computing market is not just growing—it is redefining the very nature of progress. Businesses, researchers, and nations prepared to ride this wave will find themselves at the forefront of a smarter, faster, and more resilient future.
About Us:
SNS Insider is one of the leading market research and consulting agencies that dominates the market research industry globally. Our company's aim is to give clients the knowledge they require in order to function in changing circumstances. In order to give you current, accurate market data, consumer insights, and opinions so that you can make decisions with confidence, we employ a variety of techniques, including surveys, video talks, and focus groups around the world.
Contact Us:
Jagney Dave - Vice President of Client Engagement
Phone: +1-315 636 4242 (US) | +44- 20 3290 5010 (UK)
0 notes
Text
AlphaEvolve Coding Agent using LLM Algorithmic Innovation

AlphaEvolve
Large language models drive AlphaEvolve, a powerful coding agent that discovers and optimises difficult algorithms. It solves both complex and simple mathematical and computational issues.
AlphaEvolve combines automated assessors' rigour with LLMs' creativity. This combination lets it validate solutions and impartially assess their quality and correctness. AlphaEvolve uses evolution to refine its best ideas. It coordinates an autonomous pipeline that queries LLMs and calculates to develop algorithms for user-specified goals. An evolutionary method improves automated evaluation metrics scores by building programs.
Human users define the goal, set assessment requirements, and provide an initial solution or code skeleton. The user must provide a way, usually a function, to automatically evaluate produced solutions by mapping them to scalar metrics to be maximised. AlphaEvolve lets users annotate code blocks in a codebase that the system will build. As a skeleton, the remaining code lets you evaluate the developed parts. Though simple, the initial program must be complete.
AlphaEvolve can evolve a search algorithm, the solution, or a function that creates the solution. These methods may help depending on the situation.
AlphaEvolve's key components are:
AlphaEvolve uses cutting-edge LLMs like Gemini 2.0 Flash and Gemini 2.0 Pro. Gemini Pro offers deep and insightful suggestions, while Gemini Flash's efficiency maximises the exploration of many topics. This ensemble technique balances throughput and solution quality. The major job of LLMs is to assess present solutions and recommend improvements. AlphaEvolve's performance is improved with powerful LLMs despite being model-agnostic. LLMs either generate whole code blocks for brief or completely changed code or diff-style code adjustments for focused updates.
Prompt Sample:
This section pulls programs from the Program database to build LLM prompts. Equations, code samples, relevant literature, human-written directions, stochastic formatting, and displayed evaluation results can enhance prompts. Another method is meta-prompt evolution, where the LLM suggests prompts.
Pool of Evaluators
This runs and evaluates proposed programs using user-provided automatic evaluation metrics. These measures assess solution quality objectively. AlphaEvolve may evaluate answers on progressively complicated scenarios in cascades to quickly eliminate less promising examples. It also provides LLM-generated feedback on desirable features that measurements cannot measure. Parallel evaluation speeds up the process. AlphaEvolve optimises multiple metrics. AlphaEvolve can only solve problems with machine-grade solutions, but its automated assessment prevents LLM hallucinations.
The program database stores created solutions and examination results. It uses an evolutionary algorithm inspired by island models and MAP-elites to manage the pool of solutions and choose models for future generations to balance exploration and exploitation.
Distributed Pipeline:
AlphaEvolve is an asynchronous computing pipeline developed in Python using asyncio. This pipeline with a controller, LLM samplers, and assessment nodes is tailored for throughput to produce and evaluate more ideas within a budget.
AlphaEvolve has excelled in several fields:
It improved hardware, data centres, and AI training across Google's computing ecosystem.
AlphaEvolve recovers 0.7% of Google's worldwide computer resources using its Borg cluster management system heuristic. This in-production solution's performance and human-readable code improve interpretability, debuggability, predictability, and deployment.
It suggested recreating a critical arithmetic circuit in Google's Tensor Processing Units (TPUs) in Verilog, removing unnecessary bits, and putting it into a future TPU. AlphaEvolve can aid with hardware design by suggesting improvements to popular hardware languages.
It sped up a fundamental kernel in Gemini's architecture by 23% and reduced training time by 1% by finding better ways to partition massive matrix multiplication operations, increasing AI performance and research. Thus, kernel optimisation engineering time was considerably reduced. This is the first time Gemini optimised its training technique with AlphaEvolve.
AlphaEvolve optimises low-level GPU operations to speed up Transformer FlashAttention kernel implementation by 32.5%. It can optimise compiler Intermediate Representations (IRs), indicating promise for incorporating AlphaEvolve into the compiler workflow or adding these optimisations to current compilers.
AlphaEvolve developed breakthrough gradient-based optimisation processes that led to novel matrix multiplication algorithms in mathematics and algorithm discovery. It enhanced Strassen's 1969 approach by multiplying 4x4 complex-valued matrices with 48 scalar multiplications. AlphaEvolve matched or outperformed best solutions for many matrix multiplication methods.
When applied to over 50 open mathematics problems, AlphaEvolve enhanced best-known solutions in 20% and rediscovered state-of-the-art solutions in 75%. It improved the kissing number problem by finding a configuration that set a new lower bound in 11 dimensions. Additionally, it improved bounds on packing difficulties, Erdős's minimum overlap problem, uncertainty principles, and autocorrelation inequalities. These results were often achieved by AlphaEvolve using problem-specific heuristic search strategies.
AlphaEvolve outperforms FunSearch due to its capacity to evolve across codebases, support for many metrics, and use of frontier LLMs with rich context. It differs from evolutionary programming by automating evolution operator creation via LLMs. It improves artificial intelligence mathematics and science by superoptimizing code.
One limitation of AlphaEvolve is that it requires automated evaluation problems. Manual experimentation is not among its capabilities. LLM evaluation is possible but not the major focus.
AlphaEvolve should improve as LLMs code better. Google is exploring a wider access program and an Early Access Program for academics. AlphaEvolve's broad scope suggests game-changing uses in business, sustainability, medical development, and material research. Future phases include reducing AlphaEvolve's performance to base LLMs and maybe integrating natural-language feedback approaches.
#AlphaEvolve#googleAlphaEvolve#codingagent#AlphaEvolveCodingAgent#googleCodingAgent#largelanguagemodels#technology#technologynews#technews#news#govindhtech
0 notes
Text
Best Open-Source AI Frameworks for Developers in 2025
Artificial Intelligence (AI) is transforming industries, and open-source frameworks are at the heart of this revolution. For developers, choosing the right AI tools can make the difference between a successful project and a stalled experiment. In 2025, several powerful open-source frameworks stand out, each with unique strengths for different AI applications—from deep learning and natural language processing (NLP) to scalable deployment and edge AI.
Here’s a curated list of the best open-source AI frameworks developers should know in 2025, along with their key features, use cases, and why they matter.
1. TensorFlow – The Industry Standard for Scalable AI
Developed by Google, TensorFlow remains one of the most widely used AI frameworks. It excels in building and deploying production-grade machine learning models, particularly for deep learning and neural networks.
Why TensorFlow?
Flexible Deployment: Runs on CPUs, GPUs, and TPUs, with support for mobile (TensorFlow Lite) and web (TensorFlow.js).
Production-Ready: Used by major companies for large-scale AI applications.
Strong Ecosystem: Extensive libraries (Keras, TFX) and a large developer community.
Best for: Enterprises, researchers, and developers needing scalable, end-to-end AI solutions.
2. PyTorch – The Researcher’s Favorite
Meta’s PyTorch has gained massive popularity for its user-friendly design and dynamic computation graph, making it ideal for rapid prototyping and academic research.
Why PyTorch?
Pythonic & Intuitive: Easier debugging and experimentation compared to static graph frameworks.
Dominates Research: Preferred for cutting-edge AI papers and NLP models.
TorchScript for Deployment: Converts models for optimized production use.
Best for: AI researchers, startups, and developers focused on fast experimentation and NLP.
3. Hugging Face Transformers – The NLP Powerhouse
Hugging Face has revolutionized natural language processing (NLP) by offering pre-trained models like GPT, BERT, and T5 that can be fine-tuned with minimal code.
Why Hugging Face?
Huge Model Library: Thousands of ready-to-use NLP models.
Easy Integration: Works seamlessly with PyTorch and TensorFlow.
Community-Driven: Open-source contributions accelerate AI advancements.
Best for: Developers building chatbots, translation tools, and text-generation apps.
4. JAX – The Next-Gen AI Research Tool
Developed by Google Research, JAX is gaining traction for high-performance numerical computing and machine learning research.
Why JAX?
Blazing Fast: Optimized for GPU/TPU acceleration.
Auto-Differentiation: Simplifies gradient-based ML algorithms.
Composable Functions: Enables advanced research in AI and scientific computing.
Best for: Researchers and developers working on cutting-edge AI algorithms and scientific ML.
5. Apache MXNet – Scalable AI for the Cloud
Backed by Amazon Web Services (AWS), MXNet is designed for efficient, distributed AI training and deployment.
Why MXNet?
Multi-Language Support: Python, R, Scala, and more.
Optimized for AWS: Deep integration with Amazon SageMaker.
Lightweight & Fast: Ideal for cloud and edge AI.
Best for: Companies using AWS for scalable AI model deployment.
6. ONNX – The Universal AI Model Format
The Open Neural Network Exchange (ONNX) allows AI models to be converted between frameworks (e.g., PyTorch to TensorFlow), ensuring flexibility.
Why ONNX?
Framework Interoperability: Avoid vendor lock-in by switching between tools.
Edge AI Optimization: Runs efficiently on mobile and IoT devices.
Best for: Developers who need cross-platform AI compatibility.
Which AI Framework Should You Choose?
The best framework depends on your project’s needs:
For production-scale AI → TensorFlow
For research & fast prototyping → PyTorch
For NLP → Hugging Face Transformers
For high-performance computing → JAX
For AWS cloud AI → Apache MXNet
For cross-framework compatibility → ONNX
Open-source AI tools are making advanced machine learning accessible to everyone. Whether you're a startup, enterprise, or researcher, leveraging the right framework can accelerate innovation.
#artificial intelligence#machine learning#deep learning#technology#tech#web developers#techinnovation#web#ai
0 notes
Text
Artificial Intelligence Market Economic Impact and Global Trade Dynamics to 2033
Artificial Intelligence (AI) has moved from a futuristic concept to a foundational pillar of modern business, economy, and society. As AI technologies mature, they are driving transformative change across industries — from healthcare and manufacturing to retail, finance, and entertainment. The global AI market is expected to expand exponentially in the coming years, fueled by advancements in machine learning (ML), natural language processing (NLP), computer vision, robotics, and edge AI.
With the rise of data-driven decision-making and automation, AI is no longer a “nice to have” but a mission-critical tool for organizations looking to maintain competitiveness and efficiency. As of 2024, the global AI market was valued at approximately USD 207 billion and is projected to reach over USD 1.84 trillion by 2032, growing at a CAGR of 31.2% during the forecast period.
Download a Free Sample Report:-https://tinyurl.com/52sdj3fb
Understanding Artificial Intelligence
Artificial Intelligence refers to the simulation of human intelligence in machines and systems programmed to think, learn, and solve problems autonomously. AI can be categorized into:
Narrow AI: Specialized for specific tasks (e.g., voice assistants, spam filters).
General AI: Human-like problem-solving and reasoning (still in research stages).
Super AI: Hypothetical AI surpassing human intelligence (conceptual).
Today’s business world predominantly leverages narrow AI and increasingly sophisticated machine learning models to automate workflows, personalize services, and uncover insights from vast datasets.
Key Market Drivers
1. Data Explosion
With the growth of the Internet of Things (IoT), social media, and eCommerce, the global data footprint is expanding exponentially. AI algorithms, particularly deep learning systems, thrive on big data — using it to recognize patterns, make predictions, and automate complex decision-making processes.
Industries like healthcare, retail, and finance are tapping into AI-powered analytics to:
Predict customer behavior.
Detect fraud.
Optimize operations.
Enable personalized marketing.
2. Advancements in Computing Power
The evolution of cloud computing and Graphics Processing Units (GPUs) has removed major computational barriers for AI development. Specialized AI chips from companies like NVIDIA, Intel, and Google (TPUs) allow faster model training, real-time inference, and efficient processing of unstructured data — particularly for image recognition, NLP, and autonomous systems.
3. Enterprise Automation Boom
AI-driven automation is reshaping industries, especially in:
Manufacturing: predictive maintenance, smart robotics.
Finance: algorithmic trading, fraud detection.
Retail: dynamic pricing, inventory optimization.
Customer Service: AI chatbots, virtual assistants.
This trend allows companies to cut costs, reduce human error, and accelerate time-to-market.
4. Rising Adoption of AI in Healthcare
The healthcare sector is one of AI’s fastest-growing application areas, including:
Medical image analysis.
AI-powered diagnostics.
Personalized treatment recommendations.
Drug discovery acceleration.
AI models like Google's DeepMind have demonstrated diagnostic accuracy that rivals — and sometimes exceeds — that of human doctors, especially in radiology and ophthalmology.
5. Government and Corporate Investments
Global investment in AI is surging, driven by public and private sector enthusiasm.
The U.S., China, the EU, and India have all announced multi-billion-dollar AI research and development initiatives.
Big Tech companies (Google, Microsoft, OpenAI, Meta, Amazon) are investing heavily in large-scale AI systems and foundation models, including generative AI.
Market Challenges
Despite rapid growth, the AI market faces several roadblocks:
Ethical and Bias Concerns: AI systems can perpetuate societal biases if trained on flawed datasets.
Regulatory Uncertainty: Emerging legislation, including the EU AI Act, will require developers and enterprises to rethink AI compliance.
High Implementation Costs: Advanced AI solutions often require significant capital outlay for computing infrastructure, skilled personnel, and data preparation.
Data Privacy and Security: Concerns over user data exploitation and AI-powered cyberattacks are creating regulatory and ethical dilemmas.
Industry Trends Shaping the AI Market
1. Generative AI Revolution
Generative AI models like OpenAI's GPT, Google's Gemini, and Meta's Llama are redefining content creation, customer service, software development, and even scientific research.
Key applications include:
Automated writing and design.
Code generation.
Text-to-image/video models.
Drug and material discovery.
By 2032, generative AI is expected to become a standard tool in business workflows, from marketing to product engineering.
2. Edge AI Deployment
Edge AI processes data locally, on devices such as smartphones, autonomous vehicles, and IoT sensors, rather than sending it to cloud servers. This enables:
Reduced latency.
Enhanced data privacy.
Offline AI capability.
Industries like automotive, healthcare devices, and industrial IoT are especially benefitting from edge AI, a trend expected to accelerate as 5G networks mature globally.
3. Explainable AI (XAI)
As AI systems take on more critical roles — such as credit scoring, healthcare diagnostics, and autonomous navigation — there is growing demand for transparency and interpretability.
Explainable AI enables stakeholders to:
Understand how AI models make decisions.
Build trust in AI-assisted systems.
Ensure ethical and fair use.
XAI is likely to become a legal requirement in regulated industries by the end of the decade.
4. AI-as-a-Service (AIaaS)
Cloud-based AI platforms provided by tech giants (AWS, Google Cloud, Microsoft Azure) allow businesses to integrate AI capabilities without building proprietary solutions.
Popular offerings include:
NLP APIs.
Vision recognition tools.
Predictive analytics models.
Machine Learning Operations (MLOps) platforms.
AIaaS is expected to democratize access to advanced AI systems, especially for small and medium enterprises.
Regional Insights
North America
North America remains a global leader in AI research, development, and commercialization. The presence of major tech companies and top universities has fostered a thriving AI ecosystem. The U.S. is particularly dominant in AI patents and venture capital investments.
Europe
The European Union has adopted a cautious but structured approach to AI growth, balancing innovation with regulation. The EU AI Act aims to establish clear ethical guidelines while promoting the adoption of AI across healthcare, automotive, and financial services.
Asia-Pacific
The region is expected to experience the highest growth rate in AI adoption through 2032, led by China, Japan, South Korea, and India. China’s government aims to make the country the global AI leader by 2030, investing heavily in autonomous vehicles, surveillance systems, and smart manufacturing.
Competitive Landscape
The AI industry is highly dynamic and fragmented, with competition between startups, tech giants, and niche AI specialists.
Leading Players:
Google (Alphabet)
Microsoft
OpenAI
IBM
Amazon Web Services (AWS)
NVIDIA
Baidu
Meta Platforms
Salesforce
Oracle
Partnerships, open-source collaboration, and mergers & acquisitions are shaping the competitive dynamics as companies race to build more efficient AI models and domain-specific solutions.
Future Outlook: 2025 to 2032
Looking ahead, AI is expected to evolve from a technical advantage to a foundational capability embedded in nearly every digital product and service. Key developments will include:
Artificial General Intelligence (AGI) research breakthroughs.
Deeper integration of AI in human-centric industries (education, law, creative arts).
AI-driven cybersecurity systems that adapt in real time.
Full-scale automation of industries, from logistics to pharmaceuticals.
Ethical AI governance, open AI ecosystems, and responsible innovation will emerge as defining pillars of the AI market in the next decade.
Conclusion
The Artificial Intelligence market stands on the brink of unprecedented expansion. Fueled by a convergence of data abundance, computational power, cloud accessibility, and a growing reliance on automation, AI is reshaping industries, societies, and economies.
From autonomous vehicles and personalized medicine to financial forecasting and creative content generation, AI is no longer the future — it is the present. And as we move toward 2032, it will increasingly define how businesses innovate, compete, and thrive in an interconnected world.
Read Full Report:-https://www.uniprismmarketresearch.com/verticals/information-communication-technology/artificial-intelligence
0 notes
Text
Harnessing the Power of AI: How Businesses Can Thrive in the Age of Acceleration
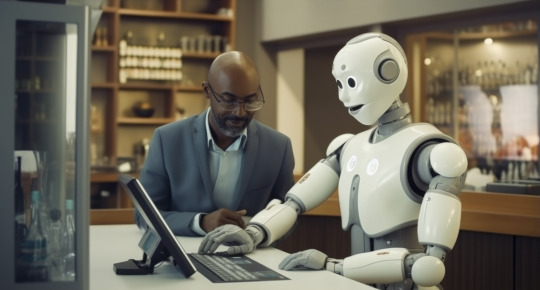
Introduction
AI adoption has surged by 270% since 2015, reshaping industries in ways once thought impossible. The Houston Chronicle reported this shift, pointing out how fast AI moves from idea to reality. Over the past ten years, AI has grown from a research topic to a key part of business strategy. It automates simple tasks and digs deep into data for insights. Yet many companies struggle to use it well. This article gives you a clear, step-by-step guide to make AI work for your business. It’s for executives, entrepreneurs, and professionals ready to act.
Why should you care? Three reasons stand out. First, companies using AI gain an edge in speed, new ideas, and customer happiness. Second, AI tools are now within reach for all, not just big players. Third, AI changes more than profits—it affects jobs, ethics, and rules you must follow.
By the end, you’ll know AI’s past, present, and future. You’ll see real examples of wins and losses. Plus, you’ll learn how 9 Figure Media helps brands lead with AI and get noticed.
Historical Background and Context
1. Early Foundations (1950s–1970s)
AI started in the 1950s with big thinkers asking: Can machines think? Alan Turing kicked things off in 1950 with his paper on machine intelligence. He created the Turing Test to check if machines could act human. In 1956, John McCarthy ran the Dartmouth Workshop, naming the field “Artificial Intelligence.” Early work built systems that followed rules, like the Logic Theorist, which proved math theorems.
Key dates:
1950: Turing’s paper sets the stage.
1956: Dartmouth makes AI official.
1966: ELIZA, a basic chatbot, talks to people.
2. AI Winters and Renewed Optimism (1970s–1990s)
Excitement faded fast. Computers lacked power, and hopes ran too high. Money dried up in the 1970s and 1980s, causing “AI winters.” But these slow years brought progress. Judea Pearl built Bayesian networks in the 1980s to handle uncertainty. By the 1990s, machine learning took off with tools like decision trees, focusing on data over strict rules.
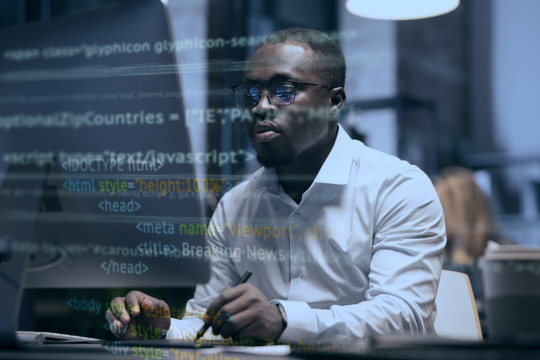
3. Big Data, Deep Learning, and Commercialization (2000s–2010s)
The 2000s changed everything. Data poured in from social media, sensors, and online shopping. New tech, like GPUs, powered deep neural networks. Big wins followed:
2011: IBM Watson beats humans on Jeopardy!
2012: AlexNet masters image recognition.
2016: AlphaGo outsmarts a Go champion.
Businesses jumped in. Streaming services recommended shows. Banks spotted fraud. Factories predicted machine breakdowns.
4. The Era of Generative AI and Democratization (2020s–Present)
Now, AI creates content—text, images, even music. Tools like GPT models write like humans. Cloud services and simple platforms let anyone use AI, no coding needed. Examples:
Health+ magazine boosted engagement 40% with personalized content.
A clothing brand cut overstock 25% with trend forecasting, as Women’s Wear Daily noted.
Part 1: The Breakneck Speed of AI Development
1. Why AI Is Accelerating
Advances in Computing Power
Old rules like Moore’s Law slow down, but new tools step up. GPUs and TPUs handle AI tasks fast. NVIDIA says GPU shipments for AI jumped 80% in 2022. Quantum computing, still early, promises even bigger leaps.
Explosion of Data and Algorithmic Innovation
Data grows daily—175 zettabytes by 2025, says IDC. New algorithms learn from it without much help. Transformers, born in 2017, process long chunks of data, powering language and image tools.
Global Investment Surge
Money flows into AI. The U.S. gave $1.5 billion to AI research in 2020. Private cash hit $93.5 billion for startups in 2021, per CB Insights. Big firms like Amazon and Microsoft buy startups and build AI labs.

2. Key Trends Redefining Business
Hyper-Automation: Beyond RPA
Basic automation follows rules. Hyper-automation adds AI to tackle messy data and decisions. A logistics company cut invoice errors 90% and halved processing time with it.
Democratization: AI for Everyone
Simple platforms like DataRobot let non-tech staff build models. Women’s Wear Daily shows fashion brands using these to predict demand and tweak marketing.
Real-Time Intelligence: The New Norm
Old reports can’t keep up. AI adjusts pricing and analytics on the fly. A travel agency raised revenue 12% with real-time pricing.
What’s next? Part 2 looks at AI’s impact—gains, risks, and must-dos.
Part 2: The Dual Impact of AI on Business
AI offers big wins but punishes delay. Here’s the breakdown.
1. Opportunities
a. Efficiency and Cost Savings
AI simplifies tough jobs. A retail chain in the Houston Chronicle cut stockouts 45% and saved 20% on inventory costs with forecasting. Health Men’s magazine sped up editing 60%, lifting ad sales. Andrew Ng, ex-Google Brain leader, says AI builds lean companies.
b. Enhanced Customer Experience
AI tailors everything. Chatbots answer fast. Netflix ties 75% of watch time to recommendations. Online stores see orders rise 10-15% with smart suggestions.
c. Innovation and New Revenue Streams
AI opens doors. A SaaS firm, with 9 Figure Media’s help, turned analytics into a subscription, growing revenue 25% in six months. Smart products feed data back, keeping customers hooked.
2. Risks of Inaction
a. Disruption by Agile Competitors
Blockbuster ignored streaming and collapsed. Today, AI startups outpace slow movers. Act late, and you lose.
b. Talent Gaps and Cultural Resistance
MIT Sloan says 58% of leaders lack AI skills in-house. Without training, teams fall behind.
c. Ethical, Legal, and Regulatory Pitfalls
AI can mess up—bias or privacy slips hurt brands. GDPR fines in Europe top €1.1 billion. Get ethics and rules right early.
Part 3: Taking Control—Strategies to Future-Proof Your Business
1. Build an AI-Ready Culture
Upskill and Reskill
Train everyone. A goods company taught 5,000 workers data basics online.
Human-AI Collaboration
Let AI crunch numbers while you plan. 9 Figure Media’s team drafts PR with AI, then edits, boosting output 40%.
2. Adopt a Strategic Roadmap
Audit Workflows
Check every process. Find spots for AI—like speeding up invoices or sales leads.
Vendor vs. In-House
Use vendors for speed, build your own for control.
3. Prioritize Ethics and Governance
Ethics Frameworks
Track data and models clearly to avoid bias.
Regulatory Readiness
Follow laws like the EU AI Act with regular checks.

4. Stay Agile and Experimental
Trend Monitoring
Read Women’s Wear Daily for retail, Houston Chronicle for tech, or Health+ for health AI.
Pilot and Iterate
Test small. A logistics firm saved 12% on fuel in 90 days, then scaled.
Part 4: Case Studies
Success Story: Inventory Optimization in Retail
Background
Sunridge Retail Group, a 50-year-old Midwest chain with 200 stores, faced demand swings. Manual orders left shelves empty or overfull, costing $5 million yearly.
Challenge
Quarterly spreadsheets missed trends, losing sales and piling up stock.
Solution and Implementation
Partnered with an AI vendor for a forecasting tool using sales, weather, and social data.
Built a cloud system for real-time updates.
Trained the model weekly with feedback.
9 Figure Media ran a campaign to get staff onboard.
Results
Stockouts dropped 45%.
Saved $2 million in inventory costs.
Sales rose 15% in one season.
Paid off in nine months, with 85% return.
CIO Maria Lopez says, “AI made us proactive. Customers get what they want.” 9 Figure Media landed stories in the Houston Chronicle and Women’s Wear Daily, boosting Sunridge’s rep.
Cautionary Tale: Logistics Firm Left Behind
Background
Midland Logistics, a 40-year freight firm with 500 trucks, stuck to manual routing and upkeep.
Challenge
Fuel costs and driver shortages hit hard. Manual plans ignored traffic and weather.
Missed Opportunity
Rival SwiftHaul used AI routing, cutting mileage 12% and fuel 8%. They won clients with speed and price.
Outcome
Midland lost 18% market share in three years.
Margins fell 5 points, income dropped 12%.
A merger saved them after leadership changed.
Ex-CEO James Carter admits, “We moved too slow. It cost us.”
Lessons
Test AI early.
Push your team to adapt.
Work with partners to catch up.
Call to Action
AI drives success today. Companies that act now win with speed, happy customers, and new ideas. Wait, and you fade. Start your AI path: map key uses, train your team, set rules, and team up with 9 Figure Media to share your wins in places like the Houston Chronicle.
Checklist: 5 Steps to Start Your AI Journey Today
Assess your AI readiness across teams.
Start a training program for AI basics.
Pick 2-3 projects with big payoffs.
Set up ethics and rule systems.
Work with AI and PR experts like 9 Figure Media to tell your story.
References and Further Reading
Brynjolfsson, E., & McAfee, A. (2014). The Second Machine Age. W.W. Norton.
McKinsey & Company. (2023). The State of AI in 2023.
IDC. (2021). Global Datasphere Forecast.
National AI Initiative Act of 2020, U.S. Congress.
Houston Chronicle, AI adoption case studies.
Women’s Wear Daily, AI in fashion forecasting.
Health+ Magazine, AI content personalization report.
0 notes
Text
AI & Data Center Chips Market to Skyrocket to $97.8B by 2034
AI & Data Center Chips Market is booming, fueled by the rising need for powerful, efficient computing to support AI-driven innovations. This market focuses on semiconductors like GPUs, TPUs, ASICs, and FPGAs — critical for enhancing machine learning, data analytics, and cloud computing. GPUs lead the charge, powering deep learning, while CPUs remain essential for broader data center operations.
To Request Sample Report : https://www.globalinsightservices.com/request-sample/?id=GIS33028 &utm_source=SnehaPatil&utm_medium=Article
North America dominates the scene with cutting-edge tech and investment, while Asia-Pacific follows closely, driven by rapid digital growth. The U.S. and China are setting the pace with their robust tech ecosystems and AI-forward policies.
Key players such as NVIDIA, Intel, and AMD shape the market — NVIDIA with unmatched GPUs, Intel with powerful processors, and AMD with aggressive pricing. Market segments include training vs. inference chips, 7nm to 3nm tech nodes, and applications ranging from computer vision to robotics.
Despite challenges like supply chain issues and regulations like GDPR and U.S. export controls, the future looks bright. Edge computing, high-performance systems, and smarter AI chips are set to revolutionize industries.
#ai #datacenter #semiconductors #gpuchips #tpuchips #asics #ml #deeplearning #cloudcomputing #edgecomputing #hpc #techtrends #futuretech #chipdesign #aidevelopment #intelligencechips #bigdata #digitaltransformation #aiinfrastructure #smartech #nvidia #intel #amd #computervision #robotics #naturalanguageprocessing #itindustry #cloudservices #aitech #aihardware #7nm #5nm #3nm #chipmarket #aiinnovation
0 notes
Text
An Inside Look at Manufacturing Materials with RapidMade’s Micah Chaban
Introduction
Micah Chaban is the Founder and VP of Sales at RapidMade, a company that has been revolutionizing manufacturing with cutting-edge 3D printing, thermoforming, and machining solutions. With a background in advanced manufacturing and a commitment to customer-focused innovation, Micah has helped businesses across industries optimize their production processes with the right materials.
In this interview, Micah provides insights into how manufacturers can select the best materials for their needs, the latest advancements in 3D printing, and how RapidMade is helping companies navigate modern manufacturing challenges.
What makes material selection so critical in manufacturing?
Material selection determines a product’s durability, weight, flexibility, and performance—it’s one of the first decisions that needs to be made in any manufacturing process. If you choose the wrong material, you could end up with a part that’s too brittle, too heavy, or unable to withstand heat, chemicals, or mechanical stress.
At RapidMade, we work with clients to balance material properties with cost and manufacturability. You can have the strongest material in the world, but if it’s too expensive or difficult to produce at scale, it may not be practical. We always look for the right combination of strength, weight, cost, and environmental resistance for each application.
How has 3D printing changed material capabilities in manufacturing?
3D printing has completely transformed the way we think about materials and part production. Traditional manufacturing methods often require expensive tooling, long lead times, and design constraints. With 3D printing, we can create complex geometries, lightweight structures, and customized components without costly molds or machining setups.
One of the biggest advancements has been the development of stronger and more diverse materials. In the past, 3D printing was mostly used for prototyping, but today, we’re using materials like Nylon PA-12, carbon-filled composites, Ultem, and metal alloys to create end-use, production-grade parts. These materials allow manufacturers to replace traditionally machined or injection-molded parts with 3D printed alternatives that are just as strong—if not stronger—but significantly lighter and more cost-effective.
What are the key material options available for 3D printing?
At RapidMade, we work with four main 3D printing technologies, each with its own material strengths.
For Multi Jet Fusion (MJF), we offer Nylon PA-12, which is lightweight, chemical-resistant, and durable, making it great for industrial and consumer applications. We also use TPU (Thermoplastic Polyurethane) for flexible, rubber-like parts that need high wear resistance.
With Stereolithography (SLA), we have high-resolution resins that produce ultra-smooth parts. We use flame-retardant SLA resins for aerospace and automotive applications and elastomers for seals, grips, and impact-resistant parts.
For Fused Deposition Modeling (FDM), we work with high-performance thermoplastics like ABS, Polycarbonate, and Ultem 9085, which are commonly used in aerospace, medical, and industrial applications due to their heat resistance and mechanical strength.
Finally, we offer Metal 3D Printing with materials like 316L Stainless Steel and 17-4 PH Stainless Steel, which are ideal for high-strength, corrosion-resistant parts used in medical, aerospace, and industrial tooling.
How does thermoforming compare to 3D printing when it comes to material options?
Thermoforming is a great alternative for medium-to-large production runs of plastic parts. Unlike 3D printing, which is typically better for small-batch production and intricate geometries, thermoforming is ideal for scaling up production while keeping costs low.
The material selection is different too. With thermoforming, we use durable, lightweight plastics like HIPS (High-Impact Polystyrene), ABS, PETG, and HDPE for packaging, automotive panels, and protective covers. For high-performance applications, we offer Polycarbonate, Kydex, and even thermoformable carbon fiber, which provide superior impact resistance, flame retardancy, and lightweight strength.
If you need large, affordable plastic parts with strength and flexibility, thermoforming is the way to go. If you need highly complex, customized, or low-volume parts, 3D printing is the better option.
Why is machining still an essential manufacturing method despite advancements in 3D printing?
Machining will always be essential because some parts require extreme precision, high-strength materials, or specific surface finishes that 3D printing or thermoforming can’t achieve. It’s the best method for low-to-medium production runs of highly engineered components that need tight tolerances.
We machine a wide range of metals and plastics, including Aluminum, Stainless Steel, Carbon Steel, and Copper for structural and aerospace components. For non-metal applications, we machine Delrin, Nylon, PEEK, and PTFE (Teflon), which are great for low-friction, high-wear applications in medical and industrial fields.
If you’re making structural parts, load-bearing components, or medical implants that need precise dimensions and wear resistance, machining is often the best choice.
What are the biggest mistakes companies make when selecting materials for manufacturing?
One of the biggest mistakes is choosing a material based solely on cost without considering performance and manufacturability. A cheaper material might work initially, but if it doesn’t hold up in real-world conditions, you’ll end up with product failures, higher replacement costs, and lost production time.
Another mistake is not considering the full production process. Some materials are great for prototyping but can’t scale efficiently. Others might be strong but too brittle for certain applications. That’s why we always ask our customers:
What environment will the part be used in?
What mechanical stresses will it experience?
What’s the expected production volume?
By answering these questions upfront, we can recommend the right material for both prototyping and full-scale production.
What trends do you see shaping the future of manufacturing materials?
We’re seeing a lot of exciting developments, especially in composite materials and sustainable manufacturing.
Carbon-filled and fiber-reinforced materials are growing in popularity because they provide high strength with reduced weight, making them ideal for aerospace, automotive, and industrial applications. We’re also seeing biodegradable and recycled materials gain traction as companies focus more on sustainability and reducing plastic waste.
On the technology side, 3D printing continues to advance, with new materials being developed for higher heat resistance, improved flexibility, and even conductive properties for electronics applications.
Overall, the future of manufacturing is about better materials, faster production, and smarter design choices—and that’s exactly what we focus on at RapidMade.
How can businesses get expert advice on material selection and manufacturing?
We know material selection can be overwhelming, which is why our team works closely with clients to help them choose the best material for their application. Whether you’re in aerospace, medical, industrial manufacturing, or consumer products, we can guide you through the best 3D printing, thermoforming, or machining solutions to meet your needs.
If you need a 3D printing service or expert guidance on materials and manufacturing, contact us at [email protected] or visit rapidmade.com to learn more about how we can help optimize your production process.
0 notes
Text
Hardware Manufacturer: The Backbone of Modern Technology
In today's fast-paced digital world, the role of a Hardware Manufacturer cannot be overstated. From smartphones to servers, hardware manufacturers are the unsung heroes behind the devices and systems that power our daily lives. This article delves into the importance of hardware manufacturers, their impact on technology, and how they are shaping the future of innovation.
What is a Hardware Manufacturer?
A Hardware Manufacturer is a company that designs, develops, and produces physical components used in electronic devices and systems. These components range from microchips and circuit boards to entire devices like laptops, smartphones, and servers. Hardware manufacturers play a critical role in the tech ecosystem, providing the essential building blocks that enable software to function.
Key Responsibilities of a Hardware Manufacturer
Research and Development (R&D): Hardware manufacturers invest heavily in R&D to create cutting-edge components that meet the ever-growing demands of technology.
Production and Assembly: They oversee the mass production of hardware components, ensuring quality and efficiency.
Quality Control: Rigorous testing is conducted to ensure that the hardware meets industry standards and performs reliably.
Innovation: Hardware manufacturers are at the forefront of technological advancements, driving innovations like AI chips, 5G modems, and quantum computing hardware.
The Importance of Hardware Manufacturers in the Tech Ecosystem
Hardware manufacturers are the backbone of the technology industry. Without them, the software and applications we rely on daily would have no platform to run on. Here’s why they are indispensable:
1. Enabling Technological Advancements
From artificial intelligence to the Internet of Things (IoT), hardware manufacturers provide the physical infrastructure needed to support these technologies. For instance, AI-powered devices require specialized chips designed and produced by hardware manufacturers.
2. Driving Economic Growth
The hardware manufacturing industry is a significant contributor to the global economy. It creates jobs, fosters innovation, and supports related industries like software development and telecommunications.
3. Enhancing User Experience
High-quality hardware ensures smooth and efficient performance, enhancing the overall user experience. Whether it’s a faster processor or a more durable smartphone, hardware manufacturers play a key role in improving device functionality.
Challenges Faced by Hardware Manufacturers
While hardware manufacturers are crucial to the tech industry, they face several challenges:
1. Supply Chain Disruptions
Global events like the COVID-19 pandemic have highlighted the vulnerabilities in the supply chain. Hardware manufacturers often rely on components from multiple countries, making them susceptible to delays and shortages.
2. Rapid Technological Changes
The tech industry evolves at a breakneck pace. Hardware manufacturers must constantly innovate to keep up with new trends and consumer demands.
3. Environmental Concerns
The production of hardware components can have a significant environmental impact. Manufacturers are under increasing pressure to adopt sustainable practices and reduce their carbon footprint.
How Hardware Manufacturers are Shaping the Future
Despite these challenges, hardware manufacturers are driving some of the most exciting developments in technology. Here are a few areas where they are making a significant impact:
1. Artificial Intelligence and Machine Learning
AI and ML require specialized hardware like GPUs and TPUs. Hardware manufacturers are developing advanced chips that can handle the complex computations needed for these technologies.
2. 5G Technology
The rollout of 5G networks relies heavily on hardware manufacturers. They produce the infrastructure and devices needed to support faster and more reliable connectivity.
3. Quantum Computing
Quantum computing is poised to revolutionize industries like healthcare, finance, and logistics. Hardware manufacturers are at the forefront of developing the quantum processors and systems that will make this possible.
4. Sustainable Hardware
As environmental concerns grow, hardware manufacturers are focusing on creating eco-friendly products. This includes using recyclable materials, reducing energy consumption, and designing products with longer lifespans.
Top Hardware Manufacturers to Watch
Several hardware manufacturers are leading the charge in innovation and sustainability. Here are a few notable names:
1. Intel
Intel is a global leader in semiconductor manufacturing, producing processors and chips that power a wide range of devices.
2. NVIDIA
Known for its GPUs, NVIDIA is a key player in the AI and gaming industries. Its hardware is essential for tasks like deep learning and real-time rendering.
3. TSMC (Taiwan Semiconductor Manufacturing Company)
TSMC is the world’s largest dedicated semiconductor foundry, producing chips for companies like Apple, AMD, and Qualcomm.
4. Samsung Electronics
Samsung is a major player in the hardware manufacturing space, producing everything from smartphones to memory chips.
5. AMD
AMD is a leading manufacturer of CPUs and GPUs, known for its high-performance processors and competitive pricing.
The Future of Hardware Manufacturing
The future of hardware manufacturing is bright, with several trends set to shape the industry:
1. Increased Automation
Automation is transforming the manufacturing process, making it faster, more efficient, and less prone to errors.
2. Focus on Sustainability
As consumers and regulators demand more eco-friendly products, hardware manufacturers are adopting greener practices.
3. Integration of AI
AI is not just a product of hardware manufacturing; it’s also a tool that manufacturers are using to optimize production and improve quality control.
4. Customization and Personalization
With advancements in manufacturing technology, hardware manufacturers can offer more customized and personalized products to meet specific consumer needs.
Conclusion
Hardware manufacturers are the unsung heroes of the tech industry, providing the essential components that power our digital world. From enabling cutting-edge technologies like AI and 5G to driving economic growth and innovation, their impact is profound. As the industry continues to evolve, hardware manufacturers will play a pivotal role in shaping the future of technology. By addressing challenges like supply chain disruptions and environmental concerns, they can continue to thrive and lead the way in innovation.
Whether you’re a tech enthusiast or a business owner, understanding the importance of hardware manufacturers can help you appreciate the complexity and ingenuity behind the devices we use every day. As we look to the future, one thing is clear: hardware manufacturers will remain at the heart of technological progress.
1 note
·
View note
Text
Price: [price_with_discount] (as of [price_update_date] - Details) [ad_1] Product Description Quick installation This back cover can be installed in a minimal time simply by just applying it to your mobile with no tool required. Built-in top quality This cover is crafted from high-quality TPU material for ultimate protection and long-lasting usage. Elegant dexterity All the cuts and holes are precise and done with machines that give it an embellishing finish. About Fashionury A well-known brand in the mobile industry. Fashionury is a design-focused organization that effectively puts unique and contemporary designs from across the world in the hands of consumers. We design items that are both long-lasting and attractive. Compatibility - Designed for Nothing Phone 1 Reliable Protection - This Desginer cover provide full 360* protection to the mobile, As, It covers from the four sides of your phone. This cover helps to protect your phone from Accidental Drops, Bumps and Scretches. Installation - Simply has to unbox the cover and has to apply directly, No Tool is required. Completely Scratch Resistant, Protective Cutting - Perfect cutouts allow easy access to all buttons, camera control and all other controls. Material -Designer, Durable TPU material, feel comfortable with unique desgines. [ad_2]
0 notes
Text
Ironwood AI Chip: Google’s 7th-gen Tensor Processing Unit

Ironwood, Google's seventh-generation Tensor Processing Unit (TPU), is the first AI accelerator designed for inference and the most scalable and performant.
Google's most difficult AI training and serving workloads have used TPUs for over 10 years, and Cloud customers can too. Ironwood is the most powerful, competent, and energy-efficient TPU. It is also built for large-scale inferential AI models.
Ironwood is a major milestone in AI and its framework. AI models that produce insights and interpret themselves are replacing responsive models that provide real-time information for human interpretation. In the “age of inference,” AI agents will proactively produce and retrieve data to collaboratively deliver answers and insights, not just facts.
Ironwood can manage the huge computational and communication needs of next-generation generative AI. It can support 9,216 liquid-cooled chips linked by 10 MW of revolutionary Inter-Chip Interconnect (ICI) networking. It is one of several unique features of the Google Cloud AI Hypercomputer architecture, which optimises software and hardware for complicated AI tasks. By using Google's Pathways software stack, Ironwood AI Chip lets developers easily and consistently use tens of thousands of Ironwood TPUs.
Ironwood powers the inference age
Ironwood AI Chip handles the complex communication and computation needs of "thinking models," including complex reasoning tasks, Mixture of Experts (MoEs), and Large Language Models. These models require efficient memory access and massive parallel computing. Ironwood is designed to manipulate massive tensors with little data flow and latency. Frontier thinking models demand more processing than a single chip can manage. Google developed Ironwood TPUs with a high-bandwidth, low-latency ICI network for TPU pod-scale synchronous, coordinated communication.
Ironwood comes in 256 and 9,216 chip variants for Google Cloud customers based on AI workloads.
El Capitan, the world's largest supercomputer, delivers 1.7 Exaflops per pod when scaled to 9,216 processors per pod, or 42.5 Exaflops in total. Ironwood can handle 24 times that. Ironwood AI Chip delivers massive parallel computing capacity for the hardest AI tasks, such as ultra-dense LLM or MoE models with thinking skills for training and inference. Each chip is outstanding with 4,614 TFLOPs peak computing. AI technology has advanced greatly. Ironwood's memory and network design ensures data availability for optimal performance at this massive scale.
Ironwood also contains an upgraded SparseCore, an accelerator for ultra-large embeddings common in advanced ranking and recommendation workloads. Ironwood's increased SparseCore support speeds up scientific, financial, and AI workloads.
Google DeepMind's Pathways machine learning software enables distributed processing across several TPU devices. Google Cloud's Pathways make it easy to construct hundreds of thousands of Ironwood AI Chips to swiftly test AI computation limits.
Key Ironwood characteristics
Google Cloud, which integrates AI computing into Gmail, Search, and other services for billions of users everyday, is the only hyperscaler with over 10 years of experience enabling cutting-edge research. Ironwood AI Chip capabilities are based on this experience. Notable traits include:
AI applications are more cost-effective due to performance advancements and power efficiency. Ironwood has double the performance per watt of Trillium, a sixth-generation TPU introduced last year. The offer more capacity per watt for client workloads when power availability limits AI capabilities. Advanced liquid cooling methods and optimised chip design can retain up to double the performance of traditional air cooling under continual, heavy AI workloads. Compared to the 2018 Cloud TPU, Ironwood AI Chip is 30 times more power efficient.
High Bandwidth Memory capacity increases significantly. Ironwood can analyse bigger models and information with 192 GB per chip, six times more than Trillium, reducing data transfers and improving speed.
HBM bandwidth was 4.5 times Trillium and reached 7.2 TBps per chip. For modern AI's memory-intensive operations, this high bandwidth ensures fast data access.
Improved Inter-Chip Interconnect bandwidth. This is now 1.2 Tbps bidirectional, 1.5x faster than Trillium, enabling faster chip-to-chip communication and scaled distributed training and inference.
Ironwood meets tomorrow's AI needs
In the era of inference, Ironwood AI Chip's processing power, memory capacity, ICI networking advances, and dependability are revolutionary. With these advances and a nearly twofold boost in power efficiency, the most demanding clients can now train and serve workloads with the greatest performance and lowest latency while meeting exponential computing demand. Modern models like Gemini 2.5 and the Nobel Prize-winning AlphaFold employ TPUs. When Ironwood launches later this year, we're enthusiastic to see what AI advancements developers and Google Cloud customers will create.
#technology#technews#govindhtech#news#technologynews#Ironwood AI Chip#Ironwood AI#Ironwood#google Ironwood#Inter-Chip Interconnect#Tensor Processing Unit#Ironwood TPUs
0 notes
Text
Top 5 Trends in the Computer Components Industry for 2024
The computer components industry is constantly evolving, driven by technological advances, changing consumer needs, and shifting market dynamics. As we move into 2024, several key trends are emerging that will shape the future of this industry. From innovations in artificial intelligence (AI) to sustainability efforts, here’s a look at the top 5 trends that will dominate the computer components market this year.
1. AI-Powered Hardware and Specialized Chips
Artificial intelligence (AI) is no longer just a buzzword — it’s becoming an integral part of modern computing. In 2024, we’re seeing more specialized hardware designed to optimize AI performance, including AI-accelerating chips and coprocessors. These components are built to handle the heavy workloads required for AI algorithms, machine learning (ML), and deep learning tasks.
Companies are increasingly investing in AI-specific processors, such as NVIDIA’s A100 Tensor Core or Google’s Tensor Processing Units (TPUs), which offer higher performance for AI workloads compared to traditional processors. Additionally, AI-enhanced GPUs are becoming essential for industries like gaming, data centers, and autonomous vehicles. For businesses in the computer components wholesale industry, this presents an opportunity to cater to the growing demand for hardware optimized for AI-driven applications.
2. Sustainability and Eco-Friendly Components
Sustainability is no longer just a trend — it’s a necessity in the tech world. In 2024, consumers and businesses alike are placing increasing emphasis on eco-friendly computing solutions. The demand for green hardware is surging, driven by growing concerns about e-waste, energy consumption, and the carbon footprint of electronic products.
Manufacturers are responding by designing more energy-efficient components, such as low-power CPUs and energy-efficient memory chips, as well as incorporating recyclable materials in their designs. Companies like Intel, AMD, and others are taking steps to reduce their environmental impact by committing to carbon-neutral goals and offering more sustainable products. For wholesalers and IT partners, this shift towards sustainability is an opportunity to offer clients products that align with their environmental goals.
3. Gaming Hardware and High-Performance Components
The gaming industry continues to be a driving force behind innovation in computer components. In 2024, the demand for high-performance hardware — especially graphics cards (GPUs), processors (CPUs), and memory — will remain strong, fueled by the rise of 4K gaming, virtual reality (VR), and augmented reality (AR) experiences.
Ray tracing technology and high-refresh-rate monitors are becoming standard features in gaming setups, pushing the limits of what computer components need to deliver. Manufacturers are introducing even more powerful GPUs, with the latest models from NVIDIA’s RTX 4000 series and AMD’s RDNA 3 architecture offering increased performance for cutting-edge gaming experiences. As a wholesaler, staying ahead of these gaming hardware trends can position you as a key supplier for both individual gamers and gaming businesses.
4. Next-Gen Storage Solutions: SSDs and Beyond
In 2024, the demand for solid-state drives (SSDs) is expected to continue its upward trajectory as more consumers and businesses shift away from traditional hard drives (HDDs). SSDs offer faster read/write speeds, higher durability, and greater energy efficiency, making them ideal for everything from gaming to enterprise applications.
Additionally, we are seeing the rise of even faster storage solutions like PCIe Gen 4 and Gen 5 SSDs, which are capable of lightning-fast data transfer speeds. NVMe SSDs are becoming standard in high-performance systems, and new technologies like 3D NAND are pushing the limits of storage capacity and efficiency. Wholesalers should be prepared to meet this growing demand by keeping up with the latest storage innovations.
5. The Rise of Modular and Customizable PCs
Customization has become a key driver of consumer and business demand in the computer components market. In 2024, we’re seeing more users and businesses opting for modular PCs — systems that allow users to swap out and upgrade individual components easily. This trend is especially prevalent in the gaming and DIY enthusiast markets.
Modular PC designs enable users to customize their systems to meet specific needs, whether it’s for gaming, professional content creation, or computational tasks. Companies like NZXT and Corsair are leading the charge with customizable PC cases, cooling solutions, and easily interchangeable components. As a wholesaler, you can tap into this trend by offering a wide range of modular components, from CPU coolers to GPU mounts, to help customers build their ideal systems.
Conclusion
The computer components industry in 2024 is poised for exciting advancements, driven by AI, sustainability, gaming, storage innovations, and customization. For wholesalers like VSTL — Your IT Partners, staying informed and adaptable to these trends is crucial to meeting the demands of an evolving market. Whether you’re sourcing the latest high-performance components, offering eco-friendly options, or supporting the rise of gaming and DIY PCs, these trends present opportunities to stay ahead of the competition and provide valuable solutions to your customers.
Want to Buy IT Components in Bulk from VSTL?
At VSTL, your trusted IT partner, we offer a wide range of high-quality computer components at wholesale prices, perfect for businesses looking to buy in bulk. Whether you need CPUs, GPUs, motherboards, memory, storage solutions, or accessories, we provide competitive pricing and reliable delivery for all your bulk purchasing needs. With our commitment to excellent customer service, fast shipping, and top-tier product selection, you can streamline your supply chain and ensure your business stays ahead in the fast-paced IT industry. Contact us today to learn how VSTL can support your bulk purchasing needs!
0 notes
Text
From Chips to Insights: How AMD is Shaping the Future of Artificial Intelligence
Introduction
In an age where technological advancements are reshaping industries and redefining the boundaries of what’s possible, few companies have made a mark quite like Advanced Micro Devices (AMD). Renowned primarily for its semiconductor technology, AMD has transitioned from being a mere chip manufacturer to a pioneering force in the realm of artificial intelligence (AI). This article delves into the various dimensions of AMD's contributions, exploring how their innovations in hardware and software are setting new benchmarks amd for AI capabilities.
Through robust engineering, strategic partnerships, and groundbreaking research initiatives, AMD is not just riding the wave of AI; it’s actively shaping its future. Buckle up as we explore this transformative journey - from chips to insights.
From Chips to Insights: How AMD is Shaping the Future of Artificial Intelligence
AMD's evolution is a remarkable tale. Initially focused on producing microprocessors and graphics cards, the company has now pivoted towards harnessing AI technologies to enhance performance across various sectors. But how did this transition happen?
The Genesis: Understanding AMD’s Technological Roots
AMD started its journey as a chip manufacturer but quickly recognized the rising tide of AI. The need for faster processing capabilities became evident with increased data generation across industries. With that realization came a shift in focus towards developing versatile architectures that support machine learning algorithms essential for AI applications.
AMD's Product Portfolio: A Closer Look
One cannot understand how AMD shapes AI without diving into its product offerings:
Ryzen Processors: Known for their multi-threading capabilities that allow parallel processing, crucial for training AI models efficiently. Radeon GPUs: Graphics cards designed not just for gaming but also optimized for deep learning tasks. EPYC Server Processors: Tailored for high-performance computing environments where large datasets demand immense processing power.
Each product plays a pivotal role in enhancing computational performance, thus accelerating the pace at which AI can be developed and deployed.

youtube
Driving Innovation through Research and Development
AMD's commitment to R&D underpins its success in shaping AI technologies. Investments in cutting-edge research facilities enable them to innovate continuously. For instance, the development of AMD Cpu ROCm (Radeon Open Compute) platform provides an open-source framework aimed at facilitating machine learning workloads, thereby democratizing access to powerful computing resources.
The Role of Hardware in Artificial Intelligence Understanding Hardware Acceleration
What exactly is hardware acceleration? It refers to using specialized hardware components—like GPUs or TPUs—to perform certain tasks more efficiently than traditional CPUs alone could manage. In AI contexts, this means faster training times and enhanced model performance.
AMD GPUs and Their Impact on Machine Learning
Graphics Processing Units (GPUs) have become indispensable in machine learning due to their ability to perform multiple cal
0 notes
Text
The Evolution and Growth of TPU Film in India: A Comprehensive Analysis
Introduction: Understanding the TPU Film Revolution
The Indian manufacturing sector has witnessed remarkable growth in recent years, with specialized materials like Thermoplastic Polyurethane (TPU) film emerging as a crucial component across various industries. As TPU film manufacturers in India continue to expand their operations, the country is positioning itself as a significant player in the global TPU market. This comprehensive analysis explores the current landscape, challenges, and opportunities within India's TPU film manufacturing sector.
The Rise of TPU Film Manufacturing in India
India's journey in TPU film production began modestly but has grown exponentially over the past decade. With increasing domestic demand and export opportunities, numerous manufacturers have established state-of-the-art facilities across industrial hubs in Gujarat, Maharashtra, and Tamil Nadu. These manufacturers have invested heavily in research and development, leading to innovative products that meet international standards while maintaining cost-effectiveness.
Understanding Thermoplastic in India
The best Thermoplastic polyurethane in India has evolved from being primarily import-dependent to having a robust domestic manufacturing base. The versatility of TPU as a material has driven its adoption across multiple sectors, from automotive to electronics. Indian manufacturers have mastered the art of producing different grades of TPU, catering to varied industrial requirements while maintaining high-quality standards.
Applications and Market Segments
Industrial Applications
The industrial sector remains the largest consumer of TPU films, utilizing them for:
Machine part protection
Conveyor belt systems
Industrial packaging
Anti-corrosion coatings
Consumer Electronics
The booming electronics industry has created substantial demand for TPU protective film, particularly in:
Smartphone screen protectors
Laptop covers
Tablet accessories
Wearable device protection
Automotive Sector
Modern vehicles incorporate TPU films in various applications:
Paint protection films
Interior surface protection
Undercarriage protection
Dashboard and console protection
Manufacturing Excellence and Technology
Advanced Production Techniques
Indian manufacturers have adopted cutting-edge technologies, including:
Precision extrusion systems
Advanced quality control mechanisms
Automated production lines
Clean room facilities for specialized products
Quality Control and Standards
The industry maintains strict quality parameters through:
ISO certifications
International testing standards
Regular quality audits
Advanced testing laboratories
Market Dynamics and Growth Factors
Economic Drivers
Several factors contribute to the sector's growth:
Increasing industrial automation
Growing electronics manufacturing
Rising automotive production
Export opportunities
Regulatory Environment
The government has supported the industry through:
Make in India initiatives
Industrial corridors development
Export promotion schemes
Quality standard implementation
Innovation and Research Development
Product Development
Indian TPU film manufacturers focus on:
Custom formulations
Enhanced durability
Improved clarity
Environmental sustainability
Sustainable Manufacturing
Sustainability initiatives include:
Reduced energy consumption
Waste reduction programs
Recycling initiatives
Eco-friendly formulations
Supply Chain and Distribution
Raw Material Sourcing
Manufacturers have developed robust supply chains through:
Local supplier development
International partnerships
Quality raw material sourcing
Strategic inventory management
Distribution Networks
Efficient distribution is achieved via:
Pan-India presence
Strategic warehousing
Just-in-time delivery
Digital tracking systems
Future Prospects and Challenges
Growth Opportunities
The sector shows promising growth potential in:
Electric vehicle components
Smart device protection
Medical device protection
Sustainable packaging
Industry Challenges
Manufacturers must address:
Raw material price fluctuations
Technology upgradation costs
International competition
Quality consistency
Environmental Considerations
Sustainable Practices
The industry is adopting:
Energy-efficient production
Water conservation
Waste reduction
Green technology integration
Regulatory Compliance
Manufacturers ensure:
Environmental clearances
Pollution control measures
Waste management systems
Safety protocols
Investment and Financial Aspects
Capital Requirements
The industry requires investments in:
Modern machinery
Research facilities
Quality control systems
Skilled workforce development
Return on Investment
Investors can expect:
Growing market share
Export opportunities
Technology valorization
Sustainable growth
Human Resource Development
Skill Development
Companies focus on:
Technical training programs
Quality control expertise
Research capabilities
Management skills
Employment Generation
The sector contributes through:
Direct employment
Indirect job creation
Skill enhancement
Career development
Market Competition and Positioning
Competitive Advantage
Indian manufacturers leverage:
Cost-effective production
Quality standards
Technical expertise
Customer service
Market Differentiation
Companies focus on:
Product specialization
Custom solutions
Value-added services
Technical support
Customer Service and Support
Technical Assistance
Manufacturers provide:
Application guidance
Problem-solving support
Product selection assistance
Installation support
After-Sales Service
Services include:
Product warranties
Technical support
Replacement policies
Customer feedback systems
Industry Collaborations and Partnerships
Research Institutions
Partnerships exist with:
Technical institutes
Research laboratories
Testing facilities
Innovation centers
International Collaborations
Companies maintain:
Technology transfers
Joint ventures
Export partnerships
Knowledge exchange
Quality Assurance and Testing
Testing Protocols
Manufacturers implement:
Material testing
Performance testing
Durability testing
Environmental testing
Certification Standards
The industry maintains:
ISO certifications
Industry standards
Environmental compliance
Safety certifications
Market Expansion Strategies
Domestic Growth
Companies focus on:
Regional expansion
Market penetration
Product diversification
Customer base expansion
Export Markets
Opportunities exist in:
Southeast Asia
Middle East
Africa
South America
Conclusion
The TPU film manufacturing sector in India represents a dynamic and growing industry with significant potential for further expansion. As manufacturers continue to invest in technology, quality, and sustainability, the sector is well-positioned to meet both domestic and international demand. The industry's commitment to innovation, quality, and customer service ensures its continued growth and success in the global market.
Frequently Asked Questions (FAQs)
What makes TPU film different from other protective films?
TPU film offers superior elasticity, durability, and scratch resistance compared to other materials. It has excellent optical clarity, self-healing properties for minor scratches, and maintains its protective properties even under extreme conditions. Unlike other films, TPU can be easily applied without bubbles and doesn't yellow over time.
How long does TPU protective film typically last?
The lifespan of TPU film varies depending on usage conditions and quality, but high-quality TPU protective films can last between 3-5 years under normal conditions. Industrial-grade TPU films used in heavy-duty applications may have even longer lifespans when properly maintained and installed.
Is TPU film environmentally friendly?
Modern TPU films are increasingly being manufactured with environmental considerations in mind. Many TPU film manufacturers in India now produce recyclable variants and use eco-friendly production processes. While traditional TPU is not biodegradable, newer formulations are being developed with enhanced environmental properties.
What thickness of TPU film is ideal for electronic device protection?
For electronic devices, TPU film thickness typically ranges from 0.1mm to 0.3mm. The ideal thickness depends on the specific application: screen protectors usually use 0.1-0.15mm film for better touch sensitivity, while back covers might use 0.2-0.3mm for enhanced protection. Custom thicknesses are available for specific requirements.
0 notes
Text
How Apple’s AI is Years Behind Competitors: A Deep Dive
In the rapidly evolving world of artificial intelligence (AI), some tech giants lead the charge while others struggle to keep up. While Apple is widely regarded for its innovation in hardware and design, it is lagging behind in one crucial area—AI. Companies like Google, Microsoft, and OpenAI have surged ahead, leaving Apple grappling with a future increasingly defined by artificial intelligence. In this article, we'll explore the reasons Apple’s AI strategy is years behind its competitors and what that could mean for the future of the tech giant.
1. Siri's Stagnation
Apple was once a pioneer in AI-driven voice assistants with the release of Siri in 2011. Initially seen as a breakthrough, Siri has since failed to keep pace with rivals like Amazon Alexa, Google Assistant, and even newer systems like ChatGPT from OpenAI. While Alexa and Google Assistant have become household names known for their deep integrations, better conversational capabilities, and broader functionality, Siri remains comparatively rigid and lacks the same level of contextual understanding and adaptability.
Key Problems with Siri:
Limited conversational depth: Siri often fails to engage in multi-turn conversations or handle complex queries.
Less integration with third-party apps: While Google Assistant can interact seamlessly with thousands of third-party services, Siri is still limited in scope.
Slow learning curve: Siri's ability to improve based on user interactions seems minimal compared to the fast-learning AI models seen in other assistants.
2. Lack of AI-Focused Hardware and Infrastructure
Apple has always excelled in creating beautifully designed and highly functional hardware, but its AI capabilities are not well-supported by its hardware ecosystem. Google's Tensor Processing Units (TPUs) and Nvidia’s GPUs, for example, are pushing AI computations forward at an unprecedented pace. Microsoft’s cloud infrastructure, built through Azure, supports AI services that cater to large-scale enterprise needs.
In contrast, Apple’s hardware is not as well-suited for cutting-edge AI development. While the company has made strides with its in-house chips like the A-series and M-series processors, these are more geared towards general computing and efficiency rather than AI-specific tasks. Apple lacks the kind of AI-focused infrastructure seen in competitors, putting it behind in areas like machine learning model training and large-scale AI deployment.
3. Lack of Open AI Development
OpenAI, Google, and Microsoft are creating a significant impact by democratizing access to AI technology. For example, OpenAI’s GPT models are open to developers and businesses via API, enabling others to build on top of their technology. Google’s AI research is also openly available, providing valuable contributions to the broader scientific community.
Apple’s approach, in contrast, is much more closed. The company has always prioritized privacy and security, which is commendable, but this philosophy has also led to a restrictive AI development environment. Apple doesn’t offer the same level of open tools, frameworks, or APIs for AI development, slowing innovation and limiting the broader tech ecosystem's ability to build on its AI technologies.
4. Apple’s Privacy-First Approach is a Double-Edged Sword
Apple's commitment to user privacy is one of its defining principles. This focus on privacy makes Apple's AI solutions, such as Siri, more cautious in terms of data collection and usage compared to its competitors. However, this also limits the company's ability to use data to train advanced AI models. Competitors like Google have access to enormous datasets, allowing them to develop AI systems that can learn from billions of interactions and provide personalized experiences at scale.
For instance, Google Assistant uses data from search queries, emails, and even location to provide highly tailored responses, while Siri’s functionality remains relatively basic. Apple's privacy-first approach, while important, puts constraints on its ability to innovate quickly in the AI space, where data is essential for improving performance and capabilities.
5. Delayed AI Integration Across Products
Another major factor is Apple’s sluggish integration of AI into its core products and services. Companies like Google and Microsoft are embedding AI into nearly every product, from search engines and web browsers to enterprise-level cloud services. Microsoft, for example, has incorporated AI into its Office Suite (e.g., Excel and Word) and is leveraging OpenAI’s GPT models across its entire ecosystem.
Apple, on the other hand, has been slow to integrate AI meaningfully beyond a few features in Photos, Siri, and iOS predictive text. While its products benefit from machine learning in terms of performance, battery life, and camera features, Apple is not innovating at the same scale when it comes to leveraging AI across its ecosystem.
6. Underwhelming AI Acquisitions
While Apple has made numerous AI-related acquisitions over the years, it hasn't translated them into groundbreaking consumer-facing technologies. Companies like Google have used acquisitions to rapidly advance their AI capabilities, but Apple’s acquisitions—such as Turi (a machine learning company) and Xnor.ai (edge-based AI)—haven't resulted in significant improvements in its core products. Meanwhile, competitors like Microsoft have made strategic acquisitions like OpenAI, giving them an enormous advantage in large language models and generative AI.
7. Competitors Are Moving Faster
The world of AI moves at breakneck speed, and Apple has not matched the urgency of its competitors. OpenAI's iterative advancements with GPT models, Google’s continuous improvements in areas like search and cloud AI, and Microsoft’s aggressive AI-driven strategies in enterprise software are setting the pace. Apple, meanwhile, continues to focus on refining its user experience and hardware design, which, while valuable, doesn't place them at the forefront of the AI revolution.
The Road Ahead: Can Apple Catch Up?
Despite its slow progress in AI, Apple still has considerable resources and brand loyalty to leverage. The company's strength lies in its ability to create seamless hardware-software experiences, and there’s potential for Apple to use AI in innovative ways within this ecosystem. For example, integrating AI-driven health features in its wearables, or making Siri more contextually aware and intelligent, could give Apple a unique edge.
However, to catch up, Apple will need to significantly ramp up its AI research and development, consider opening up its AI platforms to developers, and potentially ease some of its privacy constraints in a responsible manner. Without a bold move, Apple risks becoming an AI follower rather than a leader in the next wave of technological innovation.
In conclusion, Apple has built its empire on revolutionary design, seamless user experience, and premium hardware, but as AI becomes the cornerstone of future technology, its reluctance or inability to lead in AI innovation puts it in a precarious position. While the company is far from out of the game, it must shift gears if it hopes to keep pace in the AI arms race.
Contact Us
0 notes