#web automation using robot framework
Explore tagged Tumblr posts
Text
Best Practices for Designing a Test Automation Framework
Designing a robust test automation framework is essential for scalable, maintainable, and efficient testing. A well-structured framework helps teams standardize test processes, accelerate execution, and improve code reusability. Here are key best practices to follow:
Define a Clear Architecture
Choose a layered structure that separates test scripts, utilities, and test data. This modularity improves maintainability and enables easy updates.
Select the Right Tools and Tech Stack
Choose tools that align with your application, team skillsets, and CI/CD goals—like Selenium, TestNG, Cypress, or Playwright. Integrate with version control and build tools for automation framework continuity.
Use Data-Driven and Keyword-Driven Approaches
Implement reusable test logic that supports parameterization. This reduces redundancy and allows flexibility in running tests with various datasets.
Enable Logging, Reporting, and Exception Handling
Build in detailed logs and custom reports for quick debugging. Include robust error handling to prevent script failures from breaking the entire suite.
Ensure Scalability and Maintainability
Design the framework to scale with your application. Follow coding standards, comment code clearly, and regularly refactor for performance.
A well-designed framework is the foundation for long-term test automation success.
#AI test automation#tcoe testing#test management tools#qa test management tools#automation tools for testing#performance testing tools#open source testing#web automation using robot framework#test automation strategy#testing coe framework
0 notes
Text
Thanks for the thoughtful reply. I see things a bit differently. allow me to share what I hope to be a thoughtful reply as well:
In terms of plagiarism, I think there are two different issues at play: firstly, training on data one doesn't own, and secondly, reproducing that data and presenting it as original data.
I agree that these are both issues but I don't think they're of equal concern or frequency. In particular, I see "plagiarism" as representing the second issue more than the first. Reproducing other people's works is not ok. That is plagiarism. That has occasionally happened with AI and must be sorted out. No questions there. However, that represents a fraction of a fraction of results. It is a very small and niche issue which is already being addressed.
That said, the first problem - using unowned data - is more nebulous, legally and ethically speaking. On one hand, AI is doing basically the same thing that humans do, which is learn and imitate from others. On the other hand, AI can do this with a level of detail and speed which humans can't. Then there's also issues with the data. For instance, if google uses youtube to train their AI, they probably have some legal right to that as a result of hosting the video. Whenever people use "free" websites, there are usually "costs" which we agree to. (Is that fair? idk. personally, it's ok with me. I think it's a fair trade.) That said, I also expect legal frameworks to develop which make it easier for people to opt-in or opt-out or get compensation as these systems grow. Lastly, it's not clear to me that it's bad for AI to learn from these sources in general - again, much like how humans do. I only really see a problem when it starts to produce actual plagiarism, as discussed above.
Also, in terms of data, I agree with some of your points. I do want to point out, though, that data isn't a finite resource. Just the opposite. We put more and more data on the web each year. in 2010, it got around 2 zetabytes; in 2015 it was 15 zetabytes, in 2020, 64 zetabytes, in 2024, we're expected to have put online nearly 150 zetabytes. And with the growth of things like smartphones, video apps (youtube, tiktok, etc), augmented reality glasses, autonomous robots, self-driving cars, and people engaging with their AIs - these companies are going to get ever growing amounts of rich data, about the world, environments, movement, conversation, and more.
"And that gets to the part of your closer that I wanted to talk about. With "AI" as it is, we are not going to get to a post-labor world like you want. Replacing artists and writers and other creatives will instead create a post-art world... But in such a world, humans aren't just sitting on their asses doing nothing - a lot of people will be creating, not because they have to, but because they want to. Art should be the last job replaced in the process of creating such a world, but the people in charge are trying to make it the first, because their ideal world is nowhere near in line with yours."
To be clear, I'm not interested in replacing artists more or less than any other profession bc I see all professions as valuable. I want to see *all* jobs get automated as much as is possible to get us all to a post-labor society. And yes, it is my hope and expectation that everyone will pursue their hobbies and interests once we no longer have to work. I also know that there's a lot of public focus on AI-Art since a lot of the semi-decent AI products right now are art generators. however, that's only bc art has more wiggle room in its results. But AI is coming for ALL jobs. And I think that's good.
That said, I agree that the rich see AI as a way to get even richer and not necessarily to benefit society. However, I also think that massive unemployment will steer the country (and all countries) toward post-labor systems, such as increasingly shortened work-weeks, UBI, and other changes. I am concerned about how difficult that transition period will be. But that's largely on us and how we vote. (literally one of the reasons I told people not to vote for trump. he dgaf about anyone.)
AI is not a bad technology. It is a major boon for the world. The fight is with capitalism.
'People are panicking about AI tools the same way they did when the calculator was invented, stop worrying' cannot stress enough the calculator did not forcibly pervade every aspect of our lives, has such a low error rate it's a statistical anomaly when it does happen, isn't built on mass plagiarism, and does not obliterate the fucking environment when you use it. Be so fucking serious right now
107K notes
·
View notes
Text
Digital Transformation Market – Will Cloud and AI Redefine Competitive Advantage by 2032
Digital Transformation Market was valued at USD 895.7 Billion in 2023 and is expected to reach USD 6877.6 Billion by 2032 and grow at a CAGR of 25.44% from 2024-2032.
Digital Transformation Market is reshaping global industries as organizations accelerate the adoption of advanced technologies to remain competitive. Cloud computing, AI, IoT, automation, and big data are no longer optional—they're foundational. Companies across sectors are rethinking operations, improving customer experience, and building agile infrastructure to drive long-term success.
U.S.: Enterprises are rapidly embracing digital-first models to lead in agility, scalability, and efficiency
Digital Transformation Market continues to thrive as enterprises prioritize innovation and resilience. With increased investment in digital tools, especially post-pandemic, the market has evolved into a strategic priority. Businesses are now focusing on seamless integration, data-driven decision-making, and enhancing productivity through smarter digital ecosystems.
Get Sample Copy of This Report: https://www.snsinsider.com/sample-request/2834
Market Keyplayers:
Microsoft - Microsoft Azure
IBM - IBM Watson
Salesforce - Salesforce Customer 360
Oracle - Oracle Cloud Infrastructure
SAP - SAP S/4HANA
Google - Google Cloud Platform
Amazon Web Services (AWS) - AWS Lambda
Cisco - Cisco Meraki
Accenture - myConcerto
Deloitte - Deloitte Digital
Infosys - Infosys Digital Services
Capgemini - Capgemini Cloud Services
Wipro - Wipro HOLMES
HCL Technologies - HCL Digital Transformation Services
Adobe - Adobe Experience Cloud
TCS (Tata Consultancy Services) - TCS BaNCS
ServiceNow - ServiceNow ITSM
Atos - Atos Digital Transformation Solutions
Zebra Technologies - Zebra's SmartVision
Pega - Pega Customer Decision Hub
Market Analysis
The digital transformation journey is being shaped by rising customer expectations, the demand for real-time insights, and the need to modernize legacy systems. In both the U.S. and Europe, industries including finance, healthcare, manufacturing, and retail are leveraging digital tools to streamline operations, ensure compliance, and unlock new growth avenues. Strategic partnerships between tech providers and enterprises are further fueling adoption.
Market Trends
Surge in cloud-native application development
Increased investment in AI-powered analytics
Adoption of remote and hybrid work tech stacks
Growth of edge computing for faster data processing
Rise in cybersecurity platforms integrated with digital infrastructure
Expansion of RPA (Robotic Process Automation) to automate workflows
Use of digital twins in manufacturing and infrastructure
Market Scope
The Digital Transformation Market is expanding at an unprecedented pace, touching nearly every industry and process. Organizations are not only adopting technology but embedding it into their core strategies.
End-to-end digital integration across business units
Scalable SaaS platforms supporting cross-functional teams
Industry-specific transformation frameworks
Real-time customer experience management tools
AI and machine learning models enhancing personalization
Smart infrastructure for cities and enterprises
Forecast Outlook
The market outlook for digital transformation is one of aggressive innovation and cross-industry convergence. With continuous advancement in AI, automation, and cloud computing, enterprises will increasingly leverage digital technologies to boost agility and customer-centricity. U.S. and European firms are expected to lead in deployment, setting benchmarks for global digital maturity. Competitive advantage will hinge on speed of adoption, data utilization, and transformation strategy execution.
Access Complete Report: https://www.snsinsider.com/reports/digital-transformation-market-2834
Conclusion
Digital transformation is no longer about future-proofing—it's about leading the present. In a market where agility defines market share, businesses that integrate digital into their DNA will dominate. As the U.S. sets benchmarks in enterprise-scale deployment and Europe emphasizes secure, compliant digital growth, the stage is set for an era of smarter, faster, and more connected organizations.
About Us:
SNS Insider is one of the leading market research and consulting agencies that dominates the market research industry globally. Our company's aim is to give clients the knowledge they require in order to function in changing circumstances. In order to give you current, accurate market data, consumer insights, and opinions so that you can make decisions with confidence, we employ a variety of techniques, including surveys, video talks, and focus groups around the world.
Related Reports:
U.S.A embraces cutting-edge innovations to revolutionize the Digital Farming Market
U.S.A sees rising investment in the Distribution Automation Market to boost energy efficiency and reliability
Contact Us:
Jagney Dave - Vice President of Client Engagement
Phone: +1-315 636 4242 (US) | +44- 20 3290 5010 (UK)
Mail us: [email protected]
0 notes
Text
Why Corporate Training Must Embrace Emerging Technologies in 2025
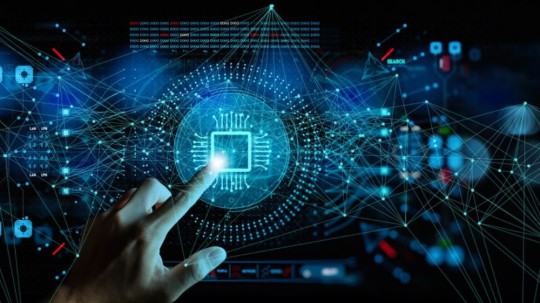
In 2025, the pace of digital transformation is no longer just rapid—it’s relentless. Companies that want to stay competitive must not only adopt new technologies but also ensure their workforce is ready to use them effectively. This is where Emerging Technology Courses play a pivotal role in corporate training programs.
The New Reality: Technology Is the Business
From artificial intelligence and machine learning to blockchain, cloud computing, and data science, modern enterprises are built on emerging technologies. The success of these implementations depends not just on the tools themselves, but on the people using them. Upskilling employees with practical, hands-on training ensures smoother transitions, fewer bottlenecks, and faster innovation.
Key Benefits of Adopting Emerging Technology Courses
1. Enhanced Workforce Readiness
Employees trained in AI, blockchain, cloud platforms, or IoT can contribute more strategically to business goals. Emerging Technology Courses prepare them to solve real-world problems using next-gen tools.
2. Stronger Innovation Culture
A workforce familiar with emerging technologies is more likely to experiment, innovate, and improve processes. This mindset shift is vital in a future-forward organization.
3. Greater Efficiency and Automation
Courses in Robotic Process Automation (RPA), Hyper Automation, and advanced analytics enable teams to automate routine tasks, freeing up valuable time for higher-order thinking.
4. Improved Security and Compliance
Cybersecurity is a major concern in the digital age. Training employees through dedicated programs not only builds awareness but also ensures compliance with evolving regulatory frameworks.
5. Talent Retention and Attraction
Top talent today seeks employers who invest in their growth. Offering Emerging Technology Courses enhances your brand as a progressive employer and improves employee retention.
Why 2025 Is the Turning Point
Technologies like Web 3.0, Generative AI, and quantum computing are no longer experimental—they’re entering the mainstream. Waiting any longer to equip your workforce could mean falling behind.
Pragati Software, through its Emerging Technologies Group, offers industry-aligned, expert-led courses that are tailored to specific organizational needs. Their programs combine theoretical grounding with hands-on labs to ensure employees not only understand the technology but can apply it immediately in their roles.
Final Thoughts
The future of work is here—and it’s tech-driven. Companies that prioritize continuous learning through Emerging Technology Courses will be the ones to lead markets, attract top talent, and build resilient digital cultures.
If your organization hasn’t yet integrated such courses into your corporate training strategy, 2025 is the year to start.
0 notes
Text
With the emergence of Node.js, building highly robust and intuitive websites in JavaScript has become simple and cost-effective than ever. The innovative and feature-rich framework has brought radical changes in web application development, replacing conventional web sockets with advanced push innovation. Furthermore, applications built on Node.js are lightweight and fast loading irrespective of a high concentration of information. The framework also helps to automate the tasks, which would otherwise be extremely cumbersome. This article lists the useful tools that I have learned from good node.js books and my development experience on various projects. Here are the top Node.js tools for effective web application development. Top Tools for Node.js Developers Stylus It is a highly robust and revolutionary CSS language that is power-packed with smart features and particularly built for the Node.js framework. Every logical operation is expressive and very easy to use which makes it a top Node.js tool for developers. The syntax is very flexible and minimal as compared to other tools. Express.js It is one of the most important tools for Node.js and is most effective for creating a host of mobile and web applications, and APIs. It offers a gamut of features like simplified multiple routing, template engines, database integration and others to power popular applications and websites. Nightwatch.js It is a user-friendly end-to-end Node.js based testing solution that is best for browser-based apps and websites. It harnesses Selenium WebDriver API to perform a range of assertions and commands on the DOM element. Hapi.js It is a powerful Node.js framework that is used to build application program interfaces (APIs) and software applications. It is a highly robust plug-in system and has numerous features like implement caching, configuration-based functionality, input validation, error handling, logging, etc. It enables developers to focus on writing reusable application logic rather than spending time building infrastructure. Socket.io The cross-browser web socket is most effective in building real-time apps across all mobile devices and browsers. It is one of the fastest and most reliable real-time engines. The advantages of this library are streamlined API, better debugging, scalability, high integration, CDN deliveries, and binary support. Cylon.js This powerful JavaScript framework is the ideal choice for physical computing, robotics, and the Internet of Things. It makes it remarkably easy to send commands to robots and devices. Math.js It is a vast math library for JavaScript and Node.js and is extremely powerful and easy to use. It provides an integrated solution to work with big numbers, numbers, complex numbers, matrices, and units. It is easily extensible and supports chained operations. Jade It is one of the most popular template engines and resources for Node.js. It is typically built for server-side templating in Node.js, but can also be used in other environments. Jade is designed only to create XML-like documents. Mojito The JavaScript framework is based on Yahoo! Cocktails and is a mobile development platform and MVC framework that offer a range of features like easy data fetching, integrated unit testing, local development tools, and a library for localization and internationalization. Sails.js It is a powerful Node.js framework and comprises of all features needed to create anything from a simple chat client to a large-scale enterprise web application. It works on the Express framework to handle sockets and HTTP requests. It is a front-end agnostic framework that gives you the flexibility to choose the right technology to build front-end. Meteor With this effective JavaScript app platform, you can seamlessly build applications that are faster and easy to use than you ever thought before. Everything works seamlessly on this Node.js tool, right from database access to server business logic to rendering to the client. Eskimo
Eskimo makes it simpler to build Node.js apps, APIs and online stores more rapidly than ever. It can be used for literally everything – referral systems, coming soon pages, CDN hosted assets and others. Simple commands and options are bundled with the CLI, making an app or web development easier and fast. Pomelo Pomelo is a fast, highly scalable and distributed game server framework for Node.js that comes with numerous tools and libraries, and provides Open Source game demo in HTML5. It offers a scalable architecture for a game, easy to use framework and API, all bundled into one to improve performance. It can help to extend severs and handle response/request, RPC, and broadcast with minimal codes. Broccoli.js It serves as the asset pipeline to build highly robust and ambitious applications on Node.js. Broccoli.js is super fast, highly flexible and needs less coding to build your assets. In fact, Broccoli is just JavaScript! Koa.js The new web framework for Node.js is designed to build smaller yet more robust and expressive web applications and APIs. It offers an entire suite of methods and tools to make writing servers faster and enjoyable. Vows.js Vows.js is a behavior-driven development framework for Node.js that is designed to test asynchronous code. This framework prioritizes clarity, speed of execution and user experience. PencilBlue It is an Open Source content management system for Node.js that is completely scalable and fully responsive. It is easy to use, extendable and is the best option for everything and not just blogs. Set It is an unobtrusive, dry template engine for Node.js that renders 100% valid HTML5 templates. It is visual, and therefore, designing in your favorite visual editor becomes simple. You can even use mock data in templates. NodeOS It is the first operating system that is powered by npm and is developed on top of Linux Kernel with Node.js run-time. It uses npm as its core package manager, and is open and easy to contribute to. Node JS Logging Tool Analyzing the distributed server logs can be really cumbersome on node.js servers. This is a logging tool that can aggregate and help analyze the logs on node servers. Taunus Taunus is a micro-isomorphic MVC engine for Node.js that is focused on delivering an enhanced experience to the end-users. It uses server-side rendering to deliver content to the end-users. Conclusion Despite its amazing features and key functionalities, Node.js is a new framework that needs the above-mentioned tools to build highly robust and interactive web and mobile applications. These tools help the developers harness the full potential of Node.js, designing and implementing beautiful applications. Furthermore, the tools make coding and writing application logic-less complex and simple. The user-friendly and intuitive Node.js tools also help in error handling, input validation, logging, and other functionalities, making application development seamless than before. Article Updates Article Updated on Dec 2019: Minor fixes and link updated. Mehul Rajput is a CEO of Mindinventory, a prominent web development and mobile app development company specialized in Node.JS Development. Delivering best solutions to its local and global clients it leads to better business all around.
0 notes
Text
Best Programming Languages for Automation Testing Beginners
Stepping into the world of automation testing can feel overwhelming at first—especially when it comes to choosing the right programming language. The good news? You don’t need to be a software developer to learn automation testing. What you do need is a solid grasp of one programming language that aligns well with testing tools and frameworks.
If you're planning to enroll in Automation Testing Classes in Pune, you’ll likely start by learning one of these beginner-friendly languages. Let’s explore the best options to begin your journey in test automation.
1. Java – The Most Popular Choice
Java is one of the most widely used languages in automation testing, especially with Selenium WebDriver. Its strong community support, abundance of learning resources, and wide adoption by companies make it a top choice for beginners.
Why Java?
Seamless integration with Selenium, TestNG, Appium, and other tools
Strong object-oriented structure, which helps in building reusable frameworks
Tons of tutorials and documentation available for self-study
If you're attending structured Automation Testing Classes in Pune, chances are you'll be introduced to Java early in the course.
2. Python – Simple and Readable
Python is becoming increasingly popular among new testers due to its simple syntax and clean code structure. It’s beginner-friendly and versatile, making it ideal for those who are intimidated by traditional programming languages.
Why Python?
Shorter learning curve for non-coders
Compatible with testing tools like PyTest, Selenium, and Robot Framework
Growing use in API and AI-based testing
Python is an excellent starting point if you're looking to transition from manual to automation testing without getting bogged down by complex code.
3. JavaScript – For Web-Focused Testers
If your focus is web testing or front-end automation, JavaScript is a strong contender. Modern tools like Cypress and Playwright use JavaScript or TypeScript and offer powerful features for end-to-end testing.
Why JavaScript?
Great for full-stack testers or those working in JavaScript-heavy environments
Tools like Cypress and Playwright are quick, modern, and developer-friendly
Ideal for testers who work closely with frontend development teams
Enrolling in Automation Testing Classes in Pune that include modern web automation tools will often expose you to JavaScript-based frameworks.
4. C# – A Strong Option for .NET Environments
C# is widely used in organizations that rely on Microsoft technologies. Paired with Selenium and NUnit, it provides robust support for automation in Windows-based systems.
Why C#?
Well-suited for testers working in .NET development environments
Clean syntax and strong performance
Easy integration with Visual Studio and Azure DevOps
While not as commonly taught in beginner courses as Java or Python, C# is worth considering if you're targeting .NET companies.
Final Thoughts: Choose One and Go Deep
It’s easy to get distracted by all the options, but remember: you don’t need to learn every language. Start with one that aligns with your course or career goals and go deep. Java and Python are often recommended for beginners due to their simplicity, popularity, and wide tool compatibility.
If you're still unsure, joining a structured program like the Automation Testing Classes in Pune offered by trusted institutes can guide your choice based on current industry demand and job market trends.
About Skillio
Skillio (formerly Testing Shastra) is Pune’s trusted name in software testing education. Known for its job-focused curriculum, Skillio trains students in top automation tools and programming languages, helping them build real-world skills from day one. Whether you’re starting fresh or upskilling, Skillio’s expert-led Automation Testing Classes in Pune are designed to get you job-ready fast.
To get more information about such IT courses from Skillio,
Contact details —
Website: https://helloskillio.com/
Address: 504, Ganeesham E, Pimple Saudagar, Pune.
Email: [email protected]
Get directions
0 notes
Text
Tech Industry Highlights from Kinghelm (May 5 – May 9, 2025)
1. Humanoid Robots Poised to Address Labor Shortages
Date: May 5, 2025
What’s Happening: Companies like Tesla, Agility Robotics, and Apptronik are advancing humanoid robot technologies aimed at mitigating labor shortages, particularly in physically demanding sectors.
Why It Matters: The humanoid robot market is projected to grow from $20 billion in 2030 to $1.2 trillion by 2040, potentially revolutionizing industries such as logistics, warehousing, and elder care.
Source: Investor's Business Daily
2. OpenAI Introduces Model Spec Framework for AI Behavior
Date: May 9, 2025
What’s Happening: OpenAI released its Model Spec framework, establishing guidelines to ensure AI tools like GPT-4 operate responsibly, adhering to laws and social norms.
Why It Matters: This initiative aims to enhance the safety and reliability of AI systems, fostering greater trust and ethical standards in AI deployment.
Source: Blue Tech Wave Media
3. Google DeepMind Unveils AlphaFold 3 for Molecular Modeling
Date: May 9, 2025
What’s Happening: DeepMind announced AlphaFold 3, an AI model capable of predicting structures of DNA, RNA, and other molecules, extending beyond protein folding.
Why It Matters: This advancement could accelerate drug discovery and deepen our understanding of biological processes, impacting healthcare and biotechnology research.
Source: Blue Tech Wave Media
4. Apple Launches iPad Pro with AI-Focused M4 Chip
Date: May 8, 2025
What’s Happening: Apple introduced a new iPad Pro equipped with the M4 chip, designed to enhance AI capabilities and performance.
Why It Matters: This move positions the iPad Pro as a powerful tool for AI development and applications, signaling Apple's commitment to advancing AI integration in its devices.
Source: Blue Tech Wave Media
5. Meta Expands AI Tools for Advertising
Date: May 8, 2025
What’s Happening: Meta unveiled new AI-driven features for its advertising platforms, enabling automated content creation and customization for ad campaigns.
Why It Matters: These tools aim to streamline the ad creation process, allowing businesses to produce tailored content more efficiently and potentially increasing ad effectiveness.
Source: Blue Tech Wave Media
6. Opera's AI Assistant 'Aria' Now Summarizes Web Pages on Android
Date: May 6, 2025
What’s Happening: Opera's in-browser AI assistant, Aria, gained the ability to summarize text-based web pages on Android devices, enhancing user experience.
Why It Matters: This feature allows users to quickly grasp the content of articles and documents, improving information accessibility and efficiency in browsing.
Source: TechCrunch
About Kinghelm
Kinghelm is a leading provider of high-quality electronic components, including RoHS-compliant antennas, wires, plug-ins, switches, and connectors. With over 17 years of experience, the company serves industries including automotive, telecommunications, industrial automation, medical devices, and consumer electronics. Kinghelm is known for its durable, reliable components that meet international standards and are used in applications ranging from renewable energy to IoT devices.
0 notes
Text
Top 10 Skills You’ll Master In A 2-Year PGDM Programme In 2025

In today’s rapidly evolving business environment, employers are looking for professionals who combine domain expertise with tech fluency, strategic thinking, and adaptability. A 2 Year PGDM Programme in 2025 is no longer just about traditional business theory—it’s about mastering multidimensional skills that align with modern industry needs.
Here are the top 10 skills you’ll gain through a 2 Year PGDM Programme in 2025:
1. Strategic Thinking & Decision Making
Learn how to evaluate complex business environments using frameworks like SWOT, PESTLE, and Porter’s Five Forces.
Develop the ability to make data-backed, long-term decisions in high-pressure scenarios.
Practical case studies from global companies strengthen strategic insight.
2. Data Analytics & Business Intelligence
Become proficient in data analysis tools such as Excel, Power BI, and Tableau.
Understand predictive analytics, data visualization, and data-driven strategy formulation.
Learn how to translate raw data into actionable business insights.
3. Digital Marketing & SEO Mastery
Stay ahead of the curve with hands-on training in digital campaigns, influencer marketing, and paid media.
Master SEO strategies, web analytics, and content optimization using tools like Google Analytics, SEMrush, and HubSpot.
Develop ROI-focused digital marketing plans tailored for modern consumers.
4. Leadership & People Management
Learn contemporary leadership models like transformational and servant leadership.
Develop soft skills such as conflict resolution, emotional intelligence, and team building.
Participate in live leadership labs and simulations to enhance people management capabilities.
5. Financial Literacy & Tech-Driven Finance
Build strong financial modeling and budgeting skills.
Learn the use of financial software like SAP, Tally, and QuickBooks.
Understand the influence of fintech and blockchain on financial decision-making.
6. Innovation & Design Thinking
Understand and apply design thinking to solve real-world business problems.
Participate in innovation bootcamps and product prototyping exercises.
Learn to foster a culture of creativity and experimentation within business teams.
7. Sustainability & ESG Awareness
Gain insights into Environmental, Social, and Governance (ESG) frameworks.
Learn how sustainability initiatives impact business value and investor confidence.
Explore global case studies on responsible business practices.
8. AI & Automation Readiness
Get introduced to the basics of artificial intelligence, machine learning, and robotic process automation.
Understand how AI tools are transforming HR, marketing, finance, and supply chain operations.
Learn how to integrate automation into business workflows ethically and efficiently.
9. Global Business Etiquette & Cross-Cultural Communication
Build communication skills to operate effectively in international markets.
Learn about cultural intelligence, global negotiation styles, and virtual collaboration.
Participate in exchange programmes or virtual global immersion experiences.
10. Entrepreneurial Mindset & Start-Up Readiness
Develop the skills needed to build and scale your own venture.
Learn business planning, fundraising, pitching, and product-market fit strategies.
Interact with start-up mentors, incubators, and real founders during the programme.
In Summary
The 2-Year PGDM Programme in 2025 goes beyond conventional management education. It’s designed to prepare students for the challenges of a tech-centric, sustainability-conscious, and globally integrated world. Whether you're aiming to become a high-impact manager, an agile entrepreneur, or a future-ready corporate leader, these top 10 skills will position you for long-term success.
When choosing a PGDM programme, look for one that offers industry collaborations, experiential learning, and a future-focused curriculum. Because the right skills, taught in the right way, can be the biggest differentiator in your career journey.
0 notes
Text
Exploring the Performance Landscape of ASX Technology Stocks
Highlights
Focus on companies listed in the technology sector of the Australian Securities Exchange
Overview of recent market themes influencing ASX Technology Stocks
Examines prominent sectors including software, AI, digital services, and cloud computing
The technology sector on the Australian Securities Exchange (ASX) represents a diverse range of enterprises involved in digital innovation, software development, cloud infrastructure, cybersecurity, and artificial intelligence. ASX Technology Stocks often align with global advancements in tech, adapting and expanding in areas like machine learning, digital transformation, and enterprise solutions.
Digital Platforms and Software Development Trends
Many ASX-listed companies in the technology segment are engaged in enterprise software and service delivery through cloud-based platforms. These entities are contributing to the increasing adoption of software-as-a-service (SaaS) frameworks. Businesses in this domain focus on functionality that enhances workflow automation, customer relationship management, and enterprise-level integration systems. ASX Technology Stocks related to software development often highlight scalable service models that cater to small and large-scale clients across various industries. These firms typically emphasize innovation in product development cycles and agile methodologies.
Cybersecurity and Data Protection Solutions
Cybersecurity remains a core segment within the ASX Technology Stocks universe. Several firms are providing network security, threat detection, and privacy protection tools, addressing rising concerns around data breaches and digital asset security. These entities serve sectors such as finance, healthcare, and education, where secure data handling is critical. The emphasis is on real-time monitoring tools and systems that prevent unauthorized access, ensuring compliance with evolving regulatory frameworks.
Artificial Intelligence and Automation
Companies working with artificial intelligence and automation technologies represent a growing niche within ASX Technology Stocks. These firms typically develop platforms for machine learning, natural language processing, and robotic process automation. The implementation of AI is evident in areas like customer service automation, intelligent document processing, and predictive maintenance in industrial settings. Automation tools are also driving efficiencies in logistics, manufacturing, and financial operations, often with an emphasis on scalability and cost-effectiveness.
Cloud Computing and Infrastructure Development
Cloud computing infrastructure providers are central to the ASX Technology Stocks list. These businesses deliver services such as remote storage, virtual server provisioning, and hybrid cloud solutions. The focus is on enabling digital transformation for both public and private sector clients. Key areas of development include data center expansion, container orchestration, and secure cloud-based collaboration platforms. Companies in this space emphasize high availability and service continuity, which are crucial for operational resilience.
E-commerce and Digital Payment Technologies
Several firms within ASX Technology Stocks are operating in the digital commerce and payments space. These businesses build systems for online transaction processing, digital wallets, and integrated checkout solutions. Their platforms are used by retailers and service providers to support seamless, secure transactions across web and mobile channels. Some companies also specialize in customer identity verification, fraud prevention, and mobile point-of-sale technologies, aligning with increasing digital engagement across industries.
Call to Action
Explore the evolving world of ASX Technology Stocks to stay informed about developments in cybersecurity, AI, cloud infrastructure, and enterprise software. Stay connected with sector-specific news and updates to monitor how technology trends are shaping various industries.
0 notes
Text
Leveraging AI in Robotic Test Automation for Smarter Testing Processes
Artificial Intelligence (AI) is revolutionizing robotic test automation by enabling smarter, more efficient testing processes. Here’s how AI enhances test automation:
Intelligent Test Case Generation
AI algorithms analyze application behaviour and user interactions to identify critical test scenarios. This eliminates guesswork and ensures comprehensive coverage with minimal manual intervention.
Self-Healing Automation Scripts
AI-powered automation can detect and adapt to changes in application UI or workflows, ensuring scripts remain functional even after updates. This reduces maintenance efforts and downtime.
Predictive Analytics
AI tools analyze historical data to predict potential failure points and prioritize high-risk areas for testing. This targeted approach optimizes resource allocation and improves efficiency.
Enhanced Accuracy and Efficiency
By automating repetitive and complex tasks with precision, AI eliminates human errors, accelerates execution, and improves the reliability of test outcomes.
Visual and Cognitive Testing
AI enables visual recognition and natural language processing for testing complex UI elements and voice/text-based interfaces, making it ideal for modern applications.
Continuous Learning
AI systems continuously learn from test data, improving decision-making and test case relevance over time.
Integrating AI into robotic test automation empowers QA teams to deliver smarter, faster, and more reliable testing, ensuring high-quality software in dynamic development environments.
#robotic test automation solution#robotic automation solution#robot framework web automation#mainframe automation using robot framework#robotics process automation testing#web automation using robot framework#warehouse automation robots
0 notes
Text
A Comprehensive Guide to the Top 7 Python Testing Frameworks

In today’s fast-paced development landscape, delivering high-quality, bug-free software is a non-negotiable requirement. Whether you're developing a web app, data pipeline, or AI solution, one thing remains constant—testing is essential. And when it comes to testing in Python, developers are spoiled for choice.
Python has long been celebrated for its simplicity and versatility, making it the backbone of many industries—from web development to AI. If you're serious about reliability and continuous delivery, it’s time to explore the top Python testing frameworks dominating 2025’s development practices.
Let’s dive into the top 7 Python testing frameworks and see how they stack up in real-world development environments.
1. Pytest – The Developer Favorite
Pytest is arguably the most popular testing framework in the Python ecosystem. It’s simple, powerful, and incredibly flexible.
Key Features:
Supports unit testing, functional testing, and API testing
Fixtures for complex setup
Plugins like pytest-django, pytest-cov, and more
Ideal for both beginners and seasoned developers, Pytest is often the top choice when you hire Python developers to build robust web or software applications.
2. Unittest (Built-in) – Python’s Native Test Framework
Inspired by Java’s JUnit, Unittest is Python’s standard testing library. While it's not as flashy or feature-rich as Pytest, it's perfect for developers who prefer sticking to built-in modules.
Key Features:
Test discovery
Test fixtures (setUp, tearDown)
Supports test automation in CI/CD environments
For teams new to testing, this is often the starting point before moving to more advanced frameworks.
3. Behave – Behavior-Driven Development (BDD)
Behave enables Behavior-Driven Development, allowing teams to write human-readable tests in the "Given-When-Then" format.
Key Features:
Great for cross-functional collaboration
Gherkin syntax support
Ideal for user journey or acceptance testing
Startups and enterprises alike choose Behave when they hire dedicated Python developers to build user-centric applications with business logic validation at every step.
4. Nose2 – Successor to Nose
While the original Nose is no longer actively maintained, Nose2 is here to pick up the torch. It's compatible with unittest and offers more plugins and improved extensibility.
Key Features:
Automatic test discovery
Plugins for test coverage, parallel testing, and more
Supports legacy Nose tests
Nose2 is perfect for teams transitioning from older testing ecosystems or managing large-scale test suites.
5. Robot Framework – For Acceptance Testing
Robot Framework is a keyword-driven testing tool perfect for acceptance testing and robotic process automation.
Key Features:
Supports Selenium, API testing, database testing
Human-readable syntax
Integrates with Python libraries
It's widely used in enterprise environments and often seen in projects managed by a mature Python development company.
6. Testify – Scalable Testing for Large Codebases
Testify is a modern, feature-rich alternative to unittest and Nose, designed with scalability and readability in mind.
Key Features:
Class-based test organization
Built-in assertion methods
Clean API for large-scale development
For companies scaling their operations, Testify offers a neat balance of power and readability. It’s a good option for teams using Python for modern software development.
7. Tox – Testing Across Environments
Tox isn’t a test runner in itself but a tool that automates testing in different Python environments. It’s indispensable for Python library authors or those managing multiple versions.
Key Features:
Test automation for different Python versions
Dependency management
Seamless CI/CD integration
Tox is especially useful when paired with other frameworks like Pytest or Unittest, ensuring your code is compatible across all Python environments.
How to Choose the Right Framework?
Choosing the right Python testing framework depends on:
Project size and complexity
Team skill level
Framework support and community
Integration with CI/CD tools and third-party services
If your business is investing in Python, the smart move is to hire Python developers who are proficient in one or more of these frameworks and can align with your development goals.
Why Testing Frameworks Matter in Modern Development
With the growing demand for faster delivery and fewer bugs, adopting structured testing processes has become standard practice. Testing ensures stability, increases confidence in releases, and accelerates development cycles.
Modern frameworks also enable:
Continuous Integration/Delivery (CI/CD) pipelines
Test-driven development (TDD)
Behavior-driven development (BDD)
Cross-platform compatibility checks
The developers you choose must align with these practices—an experienced Python development company will already have these workflows baked into their development culture.
Closing Thoughts
In 2025, the role of Python in shaping digital products continues to grow—from web platforms and enterprise solutions to AI-driven software. To keep up with this momentum, testing must be at the heart of every project.
Whether you're enhancing your development pipeline, scaling your startup, or modernizing enterprise systems, these frameworks will guide your way. But tools are only as good as the hands that wield them.
Make the right choice—hire dedicated Python developers who understand the importance of quality and know how to integrate these tools effectively.
For those beginning their journey, here’s a solid starting point: our Guide to Python for Web Development and Python: The Top Frameworks & Best Practices blog series cover everything you need to build stable, scalable applications.
Need help with your next project? Tuvoc Technologies offers expert Python development services tailored for today’s software landscape. Let’s build something exceptional—together.
#Python#PythonProgramming#PythonDev#Coding#SoftwareDevelopment#PythonTesting#TestAutomation#UnitTesting#SoftwareTesting#QAEngineer#PyTest#Unittest#TDD (Test-Driven Development)#DevTips#CodeQuality
0 notes
Text
Explore the top Python courses in Ahmedabad with prices and reviews
Python is a general-purpose programming language that focuses on readability, versatility, and extensive tools/libraries, and it is easy to learn. It is a choice of programming language for various applications such as data science, web development, and AI. Due to these advantages and easy-to-learn features that can be applied across industries such as data analytics, web development, finance, and programming, it is a good skill to learn. Python Courses in Ahmedabad offer students different types of courses and modes of learning. Let’s discuss the different courses available for Python.
Python Full-stack course with fundamentals
A full-stack course will typically cover front-end and back-end development training. The course focuses on learning to use versatile tools like Django and Flash. How to create, manage, and manipulate databases, both SQL and No SQL, and use front-end technologies like HTML, CSS, and JavaScript, and a full understanding of the framework. Highsky IT Solutions, Ahmedabad, offers complete full-stack courses and is one of the best institutes with experienced and skilled educators.
These courses provide comprehensive training in the use of Python languages according to industry standards and real-life project experience.
These courses are suitable for fresh graduates as well as professionals and are highly rated among the students due to their curriculum.
Beginner-friendly basic Python course
This course is designed for those who have little to no programming knowledge and covers the fundamentals of programming, including how to write programs, data types, variables, and control structures. These courses do not have extensive industry training opportunities, are focused mainly on theoretical knowledge of writing programs, and avoid complex mathematics, making them suitable for a wide audience. You can easily find these courses by searching for Python Programming Classes near me.
These courses are affordable and available in different price ranges and are rated moderately among the students.
Specialized Python courses
Specialized Python courses offer working knowledge of Python practices focused on special fields and their requirements, such as data science, web development, and AI. These courses are designed to cater to the needs of specific fields with hands-on projects, industry-relevant skills, and interactive learning. Other specialized areas for Python programming are game development, automation, and the robotics industry.
They are relatively pricey and are offered by a limited number of educators but are highly rated for skill development in the industry.
Advanced Python courses
These advanced courses are quite similar to the specialized courses, except that they are not industry-specific and cater to different fields. These courses have training and real-life project-handling training that are relevant to various fields and not specific to a single field. These courses provide knowledge of automation, web development, machine learning, AI, and others within a single course.
These courses are similar in price range to the specialized courses and are generally rated higher in every aspect. Python programming language, due to its versatile and wide applications, is one of the most preferred programming languages across industries. It is very easy to learn, which makes it the choice of programming language for some major global companies such as IBM, Spotify, Google, etc. Many training institutes offer different courses, such as the Python Certification Course in Ahmedabad, for new and experienced candidates. If you are looking to find an affordable yet comprehensive course in Python, you may visit Highskyit Solutions for more details.
#linux certification ahmedabad#red hat certification ahmedabad#linux online courses in ahmedabad#data science training ahmedabad#rhce rhcsa training ahmedabad#aws security training ahmedabad#docker training ahmedabad#red hat training ahmedabad#microsoft azure cloud certification#python courses in ahmedabad
0 notes
Text
Artificial Intelligence Market Size, Share, Analysis, Forecast, and Growth Trends to 2032: Cloud AI and Edge Computing to Drive Moment
The Artificial Intelligence Market was valued at USD 178.6 Billion in 2023 and is expected to reach USD 2465.8 Billion by 2032, growing at a CAGR of 33.89% from 2024-2032.
Artificial Intelligence Market is advancing at a remarkable pace, transforming industries with automation, predictive analytics, and intelligent decision-making. From finance and healthcare to retail and manufacturing, AI applications are reshaping business models across the globe, with the USA and Europe leading the charge through significant investments and rapid tech adoption.
Analyze key drivers shaping the AIoT market across the United States
Artificial Intelligence Market continues to fuel innovation and productivity as organizations turn to AI for operational efficiency and customer personalization. With scalable cloud solutions and widespread access to machine learning tools, companies are building smarter, data-driven ecosystems that redefine competitive advantage.
Get Sample Copy of This Report: https://www.snsinsider.com/sample-request/2801
Market Keyplayers:
Google (Alphabet Inc.) - Google AI
IBM - IBM Watson
Microsoft - Azure AI
Amazon Web Services (AWS) - AWS Deep Learning AMIs
NVIDIA Corporation - NVIDIA DGX Systems
Intel Corporation - Intel Nervana
Baidu, Inc. - Baidu AI
Salesforce - Salesforce Einstein
Apple Inc. - Siri
Tencent - Tencent AI Lab
SAP - SAP Leonardo
Adobe Inc. - Adobe Sensei
OpenAI - GPT-3
Market Analysis
The Artificial Intelligence Market is being driven by a surge in enterprise digital transformation, increased computing power, and the availability of vast data sets. AI technologies, including natural language processing, computer vision, and deep learning, are unlocking new capabilities in automation, risk detection, and human-machine collaboration.
The USA leads global AI development with strong tech infrastructure and investment, while Europe balances innovation with a regulatory-first approach, emphasizing ethical AI and data privacy.
Market Trends
Growth of generative AI in content creation, coding, and design
Integration of AI with IoT, robotics, and edge computing
Increased adoption of AI chatbots and virtual assistants in customer service
Expansion of AI-powered cybersecurity tools for threat detection
Surge in AI for healthcare diagnostics and drug discovery
Use of AI algorithms for hyper-personalized marketing
Democratization of AI tools through no-code and low-code platforms
Market Scope
The scope of the Artificial Intelligence Market is expanding rapidly as AI moves from experimental labs to core business functions. Companies are embedding AI into everything from supply chains to customer experiences, setting new benchmarks for speed and intelligence.
Predictive maintenance in manufacturing
AI-enhanced financial forecasting and fraud detection
Smart assistants for business operations
AI in autonomous systems (vehicles, drones)
Talent acquisition powered by AI screening tools
Real-time language translation and transcription
AI-aided legal research and contract analysis
Forecast Outlook
The Artificial Intelligence Market is poised for exponential growth as businesses, governments, and consumers embrace intelligent systems that learn, adapt, and evolve. With advancements in multimodal models and AI-as-a-service platforms, the path ahead is one of deep integration and rapid deployment. The market’s future will be defined by ethical frameworks, regulatory alignment, and the race to build trusted, scalable solutions. USA and Europe remain pivotal arenas, shaping global standards and accelerating AI maturity across sectors.
Access Complete Report: https://www.snsinsider.com/reports/artificial-intelligence-market-2801
Conclusion
The Artificial Intelligence Market is not just a technological revolution—it’s a transformation of how the world works, learns, and innovates. In an era defined by speed, data, and intelligence, AI stands at the center of progress.
Related Reports:
How AI is Transforming Call Centers Across the US: Market Analysis and Forecast
Analyze key drivers shaping the AIoT market across the United States
About Us:
SNS Insider is one of the leading market research and consulting agencies that dominates the market research industry globally. Our company's aim is to give clients the knowledge they require in order to function in changing circumstances. In order to give you current, accurate market data, consumer insights, and opinions so that you can make decisions with confidence, we employ a variety of techniques, including surveys, video talks, and focus groups around the world.
Contact Us:
Jagney Dave - Vice President of Client Engagement
Phone: +1-315 636 4242 (US) | +44- 20 3290 5010 (UK)
#Artificial Intelligence Market#Artificial Intelligence Market Scope#Artificial Intelligence Market Trends
0 notes
Text
Learning AI? These Programming Languages Are Perfect for Beginners

Introduction
Selecting the right AI programming languages can significantly impact the efficiency, scalability, and performance of AI models. Whether you're a beginner or an experienced developer, understanding the strengths of different AI-focused languages will help you make informed decisions.
Artificial Intelligence (AI) is revolutionizing industries, driving automation, and transforming businesses worldwide. From chatbots to self-driving cars, AI-powered applications are becoming an essential part of our daily lives. But before you can dive into AI development, you need to understand which programming languages are best suited for AI projects.
In this blog, we'll explore the top AI programming languages, their applications, and why they are ideal for AI development. We’ll also discuss how working with a Custom AI Development Company can streamline your AI projects.
Why Choosing the Right AI Programming Language Matters
Different AI projects require different programming languages based on complexity, efficiency, and scalability. Choosing the right AI programming languages ensures:
Faster AI model training and deployment
Better integration with AI libraries and frameworks
Efficient memory and data processing
Scalability for large-scale AI applications
Top AI Programming Languages for Beginners
1. Python – The King of AI Development
Python is the most popular programming language for AI development due to its simplicity, vast libraries, and strong community support. Frameworks like TensorFlow, PyTorch, and Scikit-learn make AI development seamless.
Why Python for AI?
Easy to learn and implement
Extensive libraries for machine learning and deep learning
Strong community support
If you're starting with AI, hiring experts from a Custom AI Development Company can help you implement AI solutions efficiently.
2. R – Best for Data Analysis & Statistics
R is widely used in AI for statistical computing and data visualization. It’s ideal for AI applications in finance, healthcare, and research.
Why R for AI?
Advanced data analysis capabilities
Great for AI-driven data visualization
Used in statistical modeling and predictive analytics
If you're looking to Hire AI Developers, consider professionals with expertise in R for AI-powered data solutions.
3. Java – Great for AI-Based Web Applications
Java is a powerful programming language used in AI for building large-scale applications, chatbots, and AI-driven enterprise software.
Why Java for AI?
Scalability and cross-platform compatibility
Works well with AI libraries like Deeplearning4j
High security for AI applications
Java is often recommended for businesses looking for AI App Development solutions that require robust backend support.
4. C++ – Best for High-Performance AI Applications
C++ is known for its speed and efficiency, making it ideal for AI projects requiring complex computations, such as robotics and computer vision.
Why C++ for AI?
Faster execution speed
Direct hardware interaction
Used in real-time AI applications
To optimize AI project costs, use an AI cost calculator to estimate development expenses and choose the most cost-effective approach.
5. JavaScript – AI on the Web
JavaScript is increasingly being used in AI, especially for web-based AI applications and interactive machine learning models.
Why JavaScript for AI?
Works well with TensorFlow.js for AI-powered web apps
Ideal for AI chatbots and virtual assistants
Real-time AI processing on web browsers
A trusted AI Development Company can help integrate AI models into JavaScript-based applications for seamless user experiences.
6. Swift – AI for iOS Applications
Swift is gaining popularity in AI development, particularly for creating AI-powered applications on Apple’s ecosystem.
Why Swift for AI?
Optimized for iOS and macOS applications
Works well with Apple’s Core ML framework
Ideal for AI-driven mobile apps
AI-based iOS apps are growing, making Swift a valuable choice for AI enthusiasts looking to develop intelligent iPhone applications.
7. Julia – The Rising Star in AI
Julia is a relatively new programming language designed for high-performance numerical computing, making it an excellent choice for AI applications.
Why Julia for AI?
High execution speed
Best for large-scale AI and machine learning models
Easy integration with Python and C++
Julia is becoming a preferred language for AI researchers working on deep learning and high-speed computational AI tasks.
How to Get Started with AI Programming?
If you're new to AI, follow these steps to begin your journey:
Choose the Right AI Programming Language – Pick a language that suits your AI project goals.
Learn AI Libraries and Frameworks – Explore TensorFlow, PyTorch, Scikit-learn, and OpenAI’s tools.
Work on AI Projects – Start with small projects like chatbots, image recognition, or sentiment analysis.
Join AI Communities – Engage with AI developers on platforms like GitHub, Stack Overflow, and Kaggle.
Consider Professional Help – Partnering with an AI Development Company can fast-track AI implementation and ensure project success.
Final Thoughts
Choosing the right AI programming languages depends on your project’s requirements and expertise. Whether you opt for Python, R, Java, C++, JavaScript, Swift, or Julia, each language has unique advantages that make AI development efficient and scalable.
If you’re looking to implement AI solutions, consider partnering with a Custom AI Development Company or Hire AI Developers to bring your AI vision to life.
Ready to start your AI journey? Explore the best AI programming languages and take the first step toward AI innovation!
0 notes
Text
Automated Testing in the Cloud: Ensuring Quality at Speed
In the fast-paced world of software development, ensuring application quality without compromising release speed is critical. Automated testing in the cloud plays a vital role in achieving this by streamlining testing processes, improving accuracy, and accelerating feedback loops.
By integrating automated testing into cloud environments, organizations can deliver high-performing applications faster while reducing manual effort and human error. This blog explores the key benefits, strategies, and tools for implementing cloud-based automated testing to achieve quality at speed.
Why Automated Testing is Essential in the Cloud
Traditional testing methods often struggle to keep up with modern cloud environments due to:
❌ Frequent Code Changes: Continuous updates increase testing demands. ❌ Scalability Challenges: Testing across multiple environments is complex. ❌ Resource Constraints: Manual testing slows down release cycles.
By leveraging cloud-based automated testing, teams can test faster, identify issues earlier, and ensure applications run reliably in dynamic cloud environments.
Key Benefits of Automated Testing in the Cloud
✅ Faster Release Cycles: Automated tests quickly validate code changes. ✅ Improved Accuracy: Automated scripts reduce the risk of human error. ✅ Scalable Testing Environments: Easily scale tests across multiple devices, browsers, and platforms. ✅ Cost Efficiency: Pay only for resources used during testing. ✅ Enhanced Collaboration: Cloud-based platforms enable global teams to collaborate seamlessly.
Types of Automated Tests for Cloud Applications
🔹 1. Unit Testing
Validates individual components or functions to ensure they perform as expected. ✅ Ideal for testing logic, algorithms, and isolated code units. ✅ Provides fast feedback to developers.
🔧 Tools: JUnit, NUnit, PyTest
🔹 2. Integration Testing
Ensures multiple components or services work together correctly. ✅ Ideal for testing APIs, database interactions, and microservices. ✅ Detects issues in service-to-service communication.
🔧 Tools: Postman, REST Assured, Karate
🔹 3. Functional Testing
Validates application features against defined business requirements. ✅ Ensures UI elements, workflows, and key features behave as expected. ✅ Ideal for cloud-based SaaS platforms and web applications.
🔧 Tools: Selenium, Cypress, Playwright
🔹 4. Performance Testing
Simulates user traffic to measure application speed, scalability, and stability. ✅ Identifies performance bottlenecks and capacity limits. ✅ Ensures applications remain responsive under load.
🔧 Tools: JMeter, k6, Gatling
🔹 5. Security Testing
Ensures cloud applications are resilient to security threats. ✅ Identifies vulnerabilities like SQL injection, XSS, and data breaches. ✅ Ensures compliance with security standards.
🔧 Tools: OWASP ZAP, Burp Suite, Astra
🔹 6. Regression Testing
Verifies that new code changes don’t break existing functionality. ✅ Critical for continuous integration pipelines. ✅ Ensures stability in frequent cloud deployments.
🔧 Tools: TestNG, Selenium, Robot Framework
Best Practices for Implementing Automated Cloud Testing
🔹 1. Integrate Testing into CI/CD Pipelines
Embedding automated tests directly into your CI/CD pipeline ensures continuous validation of new code.
✅ Trigger automated tests on every code commit or merge. ✅ Use parallel testing to run multiple tests simultaneously for faster results. ✅ Implement fail-fast strategies to identify issues early.
🔧 Tools: Jenkins, GitLab CI/CD, Azure DevOps
🔹 2. Use Scalable Test Environments
Leverage cloud platforms to create dynamic and scalable test environments.
✅ Automatically spin up cloud-based test servers as needed. ✅ Simulate real-world user environments across browsers, devices, and networks. ✅ Scale test environments based on project size or workload.
🔧 Tools: AWS Device Farm, BrowserStack, Sauce Labs
🔹 3. Implement Test Data Management
Effective data management ensures test accuracy and consistency.
✅ Use data masking and anonymization for sensitive data. ✅ Generate synthetic data to test various scenarios. ✅ Manage dynamic test data across environments.
🔧 Tools: TDM, Datprof, GenRocket
🔹 4. Automate Infrastructure Setup with IaC
Infrastructure as Code (IaC) ensures test environments are consistent and reproducible.
✅ Use IaC tools to define testing infrastructure as code. ✅ Automate environment setup to eliminate manual provisioning errors. ✅ Easily create, update, or destroy test environments as needed.
🔧 Tools: Terraform, AWS CloudFormation, Azure Resource Manager
🔹 5. Leverage Service Virtualization for Reliable Testing
Service virtualization enables testing of application components even when dependent services are unavailable.
✅ Simulate APIs, databases, and third-party integrations. ✅ Test applications in isolated environments without dependencies.
🔧 Tools: WireMock, Mountebank, Hoverfly
🔹 6. Monitor Test Performance and Results
Monitoring provides insights into test coverage, success rates, and performance bottlenecks.
✅ Use dashboards to track key metrics. ✅ Set alerts for test failures or performance anomalies. ✅ Continuously analyze test trends for process improvement.
🔧 Tools: Grafana, Datadog, TestRail
Salzen Cloud’s Approach to Automated Cloud Testing
At Salzen Cloud, we help businesses adopt scalable and effective automated testing frameworks. Our solutions include:
✔️ Implementing comprehensive CI/CD pipelines with integrated testing. ✔️ Designing scalable test environments to support complex cloud infrastructures. ✔️ Leveraging advanced tools to ensure application performance, security, and reliability.
Conclusion
Automated testing in the cloud accelerates development cycles while ensuring software quality. By combining scalable test environments, CI/CD integration, and comprehensive test coverage, businesses can achieve faster releases and improved reliability.
With Salzen Cloud, you can implement cutting-edge testing strategies to deliver high-performing cloud applications with confidence.
Need guidance on building a robust cloud testing strategy? Let's connect! 🚀
0 notes
Text
Master Your Python Interview with These Essential Q&A Tips Python continues to dominate the tech industry, powering applications in fields ranging from web development to machine learning. Its simplicity and versatility make it a favorite among developers and employers alike. For candidates preparing for Python interviews, understanding the commonly asked questions and how to answer them effectively can be the key to landing your dream job. This guide covers essential Python interview questions and answers, categorized for beginners, intermediates, and experts. Let’s dive in! 1. Basic Python Interview Questions Q1: What is Python, and what are its key features? Answer: Python is a high-level, interpreted programming language known for its readability and simplicity. Key features include: Easy syntax, similar to English. Dynamically typed (no need to declare variable types). Extensive standard libraries. Cross-platform compatibility. Supports multiple paradigms: object-oriented, procedural, and functional. Q2: What are Python’s data types? Answer: Python offers the following built-in data types: Numeric: int, float, complex Sequence: list, tuple, range Text: str Set: set, frozenset Mapping: dict Boolean: bool Binary: bytes, bytearray, memoryview Q3: Explain Python’s Global Interpreter Lock (GIL). Answer: The Global Interpreter Lock (GIL) is a mutex that protects access to Python objects, preventing multiple native threads from executing Python bytecode simultaneously. This ensures thread safety but can limit multithreading performance in CPU-bound tasks. Q4: What are Python’s popular frameworks? Answer: Some popular Python frameworks include: Web Development: Django, Flask, FastAPI Data Science: TensorFlow, PyTorch, Pandas Automation: Selenium, Robot Framework 2. Intermediate Python Interview Questions Q5: What is the difference between shallow and deep copying? Answer: Shallow Copy: Creates a new object but inserts references to the original objects within it. Use copy.copy(). Deep Copy: Creates a new object and recursively copies all objects within it. Use copy.deepcopy(). Q6: What are Python decorators? Answer: Decorators are functions that modify the behavior of another function or method. They are applied using the @decorator_name syntax and are commonly used for: Logging Authentication Performance measurement Access control Example: def decorator(func): def wrapper(): print("Before function execution") func() print("After function execution") return wrapper @decorator def say_hello(): print("Hello!") say_hello() Q7: How is memory managed in Python? Answer: Python uses automatic memory management through: Reference Counting: Tracks the number of references to an object. Garbage Collection: Reclaims memory when objects are no longer in use. Memory Pools: Allocates memory blocks to improve efficiency. 3. Advanced Python Interview Questions Q8: Explain Python’s metaclasses. Answer: Metaclasses define how classes behave. They control class creation and are specified using the metaclass keyword in class definitions. Metaclasses are commonly used to: Enforce coding standards. Add methods or attributes dynamically. Perform validation during class creation. Q9: What are Python’s comprehensions? Answer: Comprehensions provide a concise way to create sequences. Types include: List Comprehension: [x for x in range(10)] Set Comprehension: x for x in range(10) Dictionary Comprehension: x: x**2 for x in range(10) Generator Expression: (x for x in range(10)) Q10: How can you optimize Python code? Answer: Use built-in functions and libraries. Apply list comprehensions instead of loops. Use generators for large datasets. Leverage caching with functools.lru_cache. Profile code using cProfile and optimize hotspots.
Python Coding Challenges for Interviews Challenge 1: Reverse a String Write a function to reverse a string without using built-in functions. def reverse_string(s): result = "" for char in s: result = char + result return result Challenge 2: FizzBuzz Problem Print numbers from 1 to 100. For multiples of 3, print “Fizz”; for multiples of 5, print “Buzz”; for multiples of both, print “FizzBuzz”. for i in range(1, 101): if i % 3 == 0 and i % 5 == 0: print("FizzBuzz") elif i % 3 == 0: print("Fizz") elif i % 5 == 0: print("Buzz") else: print(i) Challenge 3: Find Duplicates in a List Write a function to find duplicate elements in a list. def find_duplicates(lst): seen = set() duplicates = set() for item in lst: if item in seen: duplicates.add(item) else: seen.add(item) return list(duplicates) Conclusion Preparing for a Python interview requires a mix of theoretical knowledge and hands-on practice. The questions above cover a wide range of topics, ensuring you are well-equipped for technical discussions. Remember, employers value problem-solving skills and clear communication as much as technical proficiency. Practice consistently, and you’ll be ready to ace that Python interview!
0 notes