#Cluster sampling considerations
Explore tagged Tumblr posts
Text
Cluster Sampling: Types, Advantages, Limitations, and Examples
Explore the various types, advantages, limitations, and real-world examples of cluster sampling in our informative blog. Learn how this sampling method can help researchers gather data efficiently and effectively for insightful analysis.
#Cluster sampling#Sampling techniques#Cluster sampling definition#Cluster sampling steps#Types of cluster sampling#Advantages of cluster sampling#Limitations of cluster sampling#Cluster sampling comparison#Cluster sampling examples#Cluster sampling applications#Cluster sampling process#Cluster sampling methodology#Cluster sampling in research#Cluster sampling in surveys#Cluster sampling in statistics#Cluster sampling design#Cluster sampling procedure#Cluster sampling considerations#Cluster sampling analysis#Cluster sampling benefits#Cluster sampling challenges#Cluster sampling vs other methods#Cluster sampling vs stratified sampling#Cluster sampling vs random sampling#Cluster sampling vs systematic sampling#Cluster sampling vs convenience sampling#Cluster sampling vs multistage sampling#Cluster sampling vs quota sampling#Cluster sampling vs snowball sampling#Cluster sampling steps explained
0 notes
Text
¶ … statistics have on shaping healthcare policy and guiding evidence-based practice, it is critical that researchers understand how to present the results of their studies. It is also critical that healthcare workers develop strong skills in statistical literacy, so that the results of studies are not misconstrued. Not all research results are generalizable to a population outside of the sample. Even the most carefully constructed research designs need to be critically analyzed. Similarly, care must be taken when communicating statistical results to a general audience. The American Statistical Association (1999) outlines eight main areas of ethical concern. Those areas of concern include the following: • Professionalism • Responsibilities to employers or funders • Responsibilities in testimony or publications • Responsibilities to research subjects • Responsibilities to research team colleagues • Responsibilities to other statisticians • Responsibilities regarding allegations of misconduct • Responsibilities of employers or clients to the integrity of research In the healthcare setting, each of these ethical duties is relevant, but it is the latter that may be most relevant to daily work for practitioners who read, gather, disseminate, discuss, and interpret research findings and often implement those findings into evidence-based practice. It is therefore critical that statisticians be aware of the impact their work has on public health. Statisticians are supportive of creating the "evidence-based society" and evidence-based organizational culture in healthcare (p. 5). However, statisticians are also in the unique position of having to offer warnings to healthcare practitioners, administrators, and pharmacists eager to deliver new products and services to the patient population. Statisticians deal with uncertainties and probabilities, whereas non-statisticians, even within the medical science arena, seek clear-cut, black-and-white answers. When it comes to actual research methods and design, the role of statisticians is more immediately apparent. For example, statisticians have an ethical responsibility to honestly and objectively interpret raw data, regardless of the substantive content of a research hypothesis. A statistician with access to participant personal information has an ethical responsibility to preserve and safeguard privacy and confidentiality. The issues all researchers face when conducting experiments including informed consent, remain salient. Ascribing to statistical ethics generally promotes integrity, validity, and reliability in medical research overall. The ethics of professionalism, responsibilities to employers, and the ethical responsibilities to research team colleagues require that statisticians and researchers ascribe to best practices in research design. Statisticians need to disclose the precise methods of data analysis used, including the software they use, and what data that needed to be excluded from the study and why. Research design should reflect the best interests of providing accurate results, not necessarily according to the desires and preferences of funders. Specific types of research designs and methodologies present unique ethical considerations. For example, cluster randomized trials have become increasingly common in healthcare and particularly in public health research. Cluster randomized trials help determine clustered effects of an intervention such as a vaccine. The ethic of responsibility to research participants, as outlined by the ASA (1999) includes issues related to informed consent. Informed consent is different in cluster randomized trials versus other research designs. There are two basic types of cluster randomized trials: cluster-cluster, where the entire cluster is being measured, and individual cluster trials, where the individual participants are being measured. The type of design will vary depending on the research question and hypotheses. Informed consent protocol may differ depending on which of these cluster randomized trial methods is used. Of course, this simplistic classification ignores the plethora of other types of cluster randomized trials but general rules of thumb for informed consent create common sense guidelines for statisticians. Regardless of type of cluster randomized trial, the primary areas of ethical concern include units of randomization, units of intervention, and units of observation ("Medical Ethics and Statistics," n.d.). Units of randomization imply different types or levels of consent. Individual consent alone may not be enough. Consent might need to be gleaned from community organizations and other group stakeholders like families or professional organizations. Care must be taken when presuming a leader of any organization speaks on behalf of the entire group or community, as this is not always the case. However, the use of proxies or gatekeepers will be inevitable in cluster randomized trials due to the complex nature of their design and the method of intervention administration. Consent must also be acquired at all levels of the trial, such as when an intervention is first administered and then when data is being collected. When cluster randomized trials are carried out in developing countries with problematic political institutions and inadequate legal or institutional safeguards to protect individuals, statisticians have a greater responsibility to ensure social justice. This is why consent must be gathered at multiple levels and at multiple stages in an intervention, as well as consulting with local officials ("Medical Ethics and Statistics," n.d.). Opting out of a trial presents its own unique ethical considerations, because a researcher cannot persuade a person to participate without appearing coercive. Medical statistics ethical considerations extend throughout the world, raising important social justice and human rights concerns (Aynsley-Green, et al., 2012). When a funding organization seeks confirmation for a treatment intervention, it risks systematically excluding large numbers of people from receiving a potentially beneficial intervention. Alternatively, informed consent can be distorted as when potential participants overestimate instead of underestimate the risks of participation in a trial and lack access to appropriate information. Statisticians operating under the guidance of professional organizational codes of ethics like that of the ASA (1999) will understand their primary obligations are to their professional standards, to their colleagues, and their employers. Cluster randomized trials sometimes threaten to undermine ethical responsibilities to research participants, as when an intervention is an airborne element affecting an entire community, or when a treatment intervention is available and desired but not being offered to non-participants. The latter scenario is politically problematic, given the potential for a treatment intervention to help people with terminal illnesses. Although the ASA (1999) does not mention social justice as an ethical responsibility of statisticians, social justice ethics are implied when well-endowed countries possessing rich medical services resources conduct experimental medicine trials on communities in developing countries. The recipient communities will frequently have large portions of the population suffering from diseases like HIV / AIDS, who do not wish to receive placebos in the trials. Yet without a control group, the research designs might suffer, causing an ethical conflict between the social justice responsibility to research participants and the responsibility to the professional duties of statisticians. Utilitarian excuses for the use of placebo controls need to be mitigated by commitments to redistributive justice ("Medical Ethics and Statistics," n.d.). Also, statisticians have a duty to promote ethical standards and professionalism in research; they are not singularly responsible for the root causes of social injustice. It is not right to sacrifice the integrity of research in the interests of appeasing public outcry. In addition to the issues raise when performing cluster trials in developing countries, statisticians focusing on medical ethics can encourage professional responsibility in other areas. Participants need to be informed about all the ramifications of research, and all stakeholders need to be informed of the social justice ramifications of their interventions. Yet the integrity of statisticians and the medical research community cannot be compromised. Therefore, communications is one of the most important areas of ethical conduct for a statistician. The interpretation of research results needs to be fully honest, disclosing all uncertainties and refraining from drawing improper conclusions. Pressure on the part of paying clients and organizations with a stake in the results should not influence statistical analysis or interpretations of results (Gelman, 2014). Communication of results beyond the actual study also becomes the ethical responsibility of the statistician, because oversight ensures the results are not exaggerated or misrepresented. Acceptable risk and other levels of uncertainty need to be conveyed to the healthcare community, which can then relay appropriate information to patients. Similarly, the media involved in translating studies into language laypeople can understand may use hyperbole or oversimplify results. Statistical literacy should be taught more widely at the university level in order to promote medical ethics. References American Statistical Association (1999). Ethical guidelines for statistical practice. Aynsley-Green, A, et al. (2012). Medical, statistical, ethical and human rights considerations in the assessment of age in children and young people subject to immigration control. British Medical Bulletin 102(1): 17-42. Gelman, A. (2014). Ethics and statistics. Retrieved online: http://www.stat.columbia.edu/~gelman/presentations/ethicstalk_2014_handout.pdf "Medical Ethics and Statistics," (n.d.). Retrieved online: http://www.wiley.com/legacy/products/subject/reference/cam001-.pdf Read the full article
0 notes
Text
Research findings, clinical knowledge, knowledge resulting from basic science as well as opinion from experts are all regarded as “evidence” (Drake and Goldman 32). Practices that are based on research findings, however, have high chances of resulting in outcomes that match the desires of patients across different settings as well as geographic locations. Research Problem The challenge for evidence-based practice is caused by the pressure from healthcare facilities due to containment of cost, larger availability of information, and greater sway of consumers regarding care and treatment options. This kind of practice demands some changes in students’ education, more research that is practice-relevant, and a working relationship between researchers and clinicians (Drake and Goldman 38). Evidence-based form of practice also brings an opportunity for nursing care to be more effective, more individualized, dynamic, and streamlined, and opportunities to maximize clinical judgment effects. When there is reliance on evidence in defining best practices but not for supporting practices that exist, then nursing care is said to be keeping pace with recent technological changes and benefits from the developments of new knowledge (Drake and Goldman 49). Although many young professionals have embraced this new approach, it has come with its challenges. Several research studies have indicated that the perception of nurses towards EBP is positive and they regard it as useful to better care for patients. This research will critically analyze the barriers to full acceptance of EBP. Research Design This will be a descriptive research design. Qualitative research does not, by definition, aim to precisely estimate population parameters or test hypotheses. However, most qualitative projects do attempt to. This design was identified as the most convenient and ensured that the data obtained gave answers to the research questions. The descriptive design also offers the opportunity for a logical structure of the inquiry into the problem of the study. According to Walsh descriptive surveys are good at providing information and explanations for research questions (45). Methods of Data Collection In this research, survey questionnaires were used as a means of collecting data. The questionnaire was formulated by a team that consisted of nurses and professionals from the information technology department at the university. Also, a great deal of consultation was given to research survey instruments that have been used by previous researchers on the same subject. For this matter, the questionnaires that were previously issued tackling data-seeking behavior and information needs of nurses and other medical practitioners were also given considerable weight in review. A review of a draft copy of the questionnaire was conducted to ensure the contents were valid (Walsh 34). This was performed by a group of experts consisting of nurse researchers, lecturers of information studies, registered nurses, and nursing managers. A few changes were made to that effect. Sampling Technique This research covered a population size consisting of all nurses in the state. However, a sample will be selected and the presumption made that the sample will reflect the features of the entire population. In this research, a cluster sampling technique was used. Under this method, several clusters were made and questionnaires were issued randomly. Read the full article
0 notes
Text
CONCLUSION
We have taken for the subjects of the foregoing moral essays, twelve samples of married couples, carefully selected from a large stock on hand, open to the inspection of all comers. These samples are intended for the benefit of the rising generation of both sexes, and, for their more easy and pleasant information, have been separately ticketed and labelled in the manner they have seen.
We have purposely excluded from consideration the couple in which the lady reigns paramount and supreme, holding such cases to be of a very unnatural kind, and like hideous births and other monstrous deformities, only to be discreetly and sparingly exhibited.
And here our self-imposed task would have ended, but that to those young ladies and gentlemen who are yet revolving singly round the church, awaiting the advent of that time when the mysterious laws of attraction shall draw them towards it in couples, we are desirous of addressing a few last words.
Before marriage and afterwards, let them learn to centre all their hopes of real and lasting happiness in their own fireside; let them cherish the faith that in home, and all the English virtues which the love of home engenders, lies the only true source of domestic felicity; let them believe that round the household gods, contentment and tranquillity cluster in their gentlest and most graceful forms; and that many weary hunters of happiness through the noisy world, have learnt this truth too late, and found a cheerful spirit and a quiet mind only at home at last.
How much may depend on the education of daughters and the conduct of mothers; how much of the brightest part of our old national character may be perpetuated by their wisdom or frittered away by their folly—how much of it may have been lost already, and how much more in danger of vanishing every day—are questions too weighty for discussion here, but well deserving a little serious consideration from all young couples nevertheless.
To that one young couple on whose bright destiny the thoughts of nations are fixed, may the youth of England look, and not in vain, for an example. From that one young couple, blessed and favoured as they are, may they learn that even the glare and glitter of a court, the splendour of a palace, and the pomp and glory of a throne, yield in their power of conferring happiness, to domestic worth and virtue. From that one young couple may they learn that the crown of a great empire, costly and jewelled though it be, gives place in the estimation of a Queen to the plain gold ring that links her woman’s nature to that of tens of thousands of her humble subjects, and guards in her woman’s heart one secret store of tenderness, whose proudest boast shall be that it knows no Royalty save Nature’s own, and no pride of birth but being the child of heaven!
So shall the highest young couple in the land for once hear the truth, when men throw up their caps, and cry with loving shouts—
God bless them.
_____
Title | Previous Chapter | Next Chapter |
0 notes
Text
Common Pitfalls in Machine Learning and How to Avoid Them

Selecting and training algorithms is a key step in building machine learning models.
Here’s a brief overview of the process:
Selecting the Right Algorithm The choice of algorithm depends on the type of problem you’re solving (e.g., classification, regression, clustering, etc.), the size and quality of your data, and the computational resources available.
Common algorithm choices include:
For Classification: Logistic Regression Decision Trees Random Forests Support Vector Machines (SVM) k-Nearest Neighbors (k-NN) Neural
Networks For Regression: Linear Regression Decision Trees Random Forests Support Vector Regression (SVR) Neural Networks For Clustering:
k-Means DBSCAN Hierarchical Clustering For Dimensionality Reduction: Principal Component Analysis (PCA) t-Distributed Stochastic Neighbor Embedding (t-SNE)
Considerations when selecting an algorithm:
Size of data:
Some algorithms scale better with large datasets (e.g., Random Forests, Gradient Boosting).
Interpretability:
If understanding the model is important, simpler models (like Logistic Regression or Decision Trees) might be preferred.
Performance:
Test different algorithms and use cross-validation to compare performance (accuracy, precision, recall, etc.).
2. Training the Algorithm After selecting an appropriate algorithm, you need to train it on your dataset.
Here’s how you can train an algorithm:
Preprocess the data:
Clean the data (handle missing values, outliers, etc.). Normalize/scale the features (especially important for algorithms like SVM or k-NN).
Encode categorical variables if necessary (e.g., using one-hot encoding).
Split the data:
Divide the data into training and test sets (typically 80–20 or 70–30 split).
Train the model:
Fit the model to the training data using the chosen algorithm and its hyperparameters. Optimize the hyperparameters using techniques like Grid Search or Random Search.
Evaluate the model: Use the test data to evaluate the model’s performance using metrics like accuracy, precision, recall, F1 score (for classification), mean squared error (for regression), etc.
Perform cross-validation to get a more reliable performance estimate.
3. Model Tuning and Hyperparameter Optimization Hyperparameter tuning: Many algorithms come with hyperparameters that affect their performance (e.g., the depth of a decision tree, learning rate for gradient descent).
You can use methods like: Grid Search:
Try all possible combinations of hyperparameters within a given range.
Random Search:
Randomly sample hyperparameters from a range, which is often more efficient for large search spaces.
Cross-validation:
Use k-fold cross-validation to get a better understanding of how the model generalizes to unseen data.
4. Model Evaluation and Fine-tuning Once you have trained the model, fine-tune it by adjusting hyperparameters or using advanced techniques like regularization to avoid overfitting.
If the model isn’t performing well, try:
Selecting different features.
Trying more advanced models (e.g., ensemble methods like Random Forest or Gradient Boosting).
Gathering more data if possible.
By iterating through these steps and refining the model based on evaluation, you can build a robust machine learning model for your problem.
WEBSITE: https://www.ficusoft.in/data-science-course-in-chennai/
0 notes
Text
Sample Collection Methods: A Comprehensive Guide.

1. Introduction
In research and data analysis, the accuracy and reliability of results often hinge on the quality of the sample collected. Without proper sample collection methods, even the most sophisticated analyses can lead to misleading conclusions. Understanding various sample collection techniques is essential for researchers across disciplines.
2. What is Sample Collection?
Sample collection is the process of gathering data or specimens from a subset of a population to draw meaningful conclusions about the whole. Whether it involves surveying a group of people, collecting soil samples, or selecting data points from a dataset, the purpose is to represent the larger population accurately.
3. Why is Proper Sample Collection Important?
The way a sample is collected can significantly influence the validity, accuracy, and generalizability of the research findings. A well-collected sample minimizes bias, ensures representativeness, and increases confidence in the results. In contrast, poorly collected samples can lead to incorrect interpretations, wasted resources, and flawed decisions.
4. Types of Sample Collection Methods
There are several methods of sample collection, each suitable for different research objectives and populations.
4.1. Probability Sampling Methods
Probability sampling ensures every member of the population has an equal chance of being selected, which reduces bias.
Simple Random Sampling: In this method, every individual in the population has an equal chance of being selected, often using random number generators or lotteries.
Stratified Sampling: The population is divided into distinct subgroups (strata), and samples are randomly selected from each stratum to ensure representation.
5. Factors to Consider When Choosing a Sample Collection Method
Selecting the right sample collection method depends on factors such as the research objective, target population, and available resources.
Research Goals: The purpose of the research often dictates the type of sample needed. For example, clinical trials require rigorous randomization.
Population Size and Diversity: Larger and more diverse populations may need stratified or cluster sampling to ensure representation.
Time and Budget Constraints: Convenience sampling may be used when resources are limited, but it can compromise representativeness.
Ethical Considerations: Ensuring voluntary participation and protecting participants' rights are critical in sample collection.
6. Challenges in Sample Collection
While sample collection is crucial, it comes with its own set of challenges that researchers need to address to maintain data integrity.
Sampling Bias: This occurs when certain groups are overrepresented or underrepresented in the sample.
Non-Response Issues: Participants’ unwillingness or inability to respond can skew results.
Access to the Target Population: Reaching specific populations can be difficult due to logistical, cultural, or ethical barriers.
7. Tips for Effective Sample Collection
To ensure the quality and reliability of your data, consider these best practices for effective sample collection:
Clearly Define the Population: Identify the characteristics and scope of the population you wish to study.
Use Appropriate Sampling Techniques: Choose methods that align with your research objectives and constraints.
Pilot-Test Your Sampling Strategy: Test your approach on a small scale to identify potential issues.
Monitor and Evaluate the Process: Continuously assess the sampling process to ensure it remains on track.
8. Examples of Sample Collection in Practice
Sample collection methods are widely used across various fields, from healthcare to market research.
Example 1: Clinical Trials in Medicine: Randomized controlled trials often rely on stratified sampling to ensure diverse representation of age, gender, and medical conditions.
Example 2: Customer Surveys in Marketing: Businesses frequently use convenience sampling to gather quick feedback from customers.
Example 3: Environmental Sampling for Pollution Studies: Researchers may use systematic sampling to measure pollutant levels across specific geographic areas.
9. Conclusion
Sample collection is a cornerstone of successful research, as it lays the foundation for accurate and meaningful insights. By understanding and carefully selecting appropriate methods, researchers can minimize bias, overcome challenges, and produce reliable results that drive informed decision-making.
Get more information here ...
https://clinfinite.com/SampleCollectionMethods63.php
0 notes
Text
Running a K-Means Cluster Analysis
What is a k-means cluster analysis
K-means cluster analysis is an algorithm that groups similar objects into groups called clusters. The endpoint of cluster analysis is a set of clusters, where each cluster is distinct from each other cluster, and the objects within each cluster are broadly similar to
Cluster analysis is a set of data reduction techniques which are designed to group similar observations in a dataset, such that observations in the same group are as similar to each other as possible, and similarly, observations in different groups are as different to each other as possible. Compared to other data reduction techniques like factor analysis (FA) and principal components analysis (PCA), which aim to group by similarities across variables (columns) of a dataset, cluster analysis aims to group observations by similarities across rows.
Description
K-means is one method of cluster analysis that groups observations by minimizing Euclidean distances between them. Euclidean distances are analagous to measuring the hypotenuse of a triangle, where the differences between two observations on two variables (x and y) are plugged into the Pythagorean equation to solve for the shortest distance between the two points (length of the hypotenuse). Euclidean distances can be extended to n-dimensions with any number n, and the distances refer to numerical differences on any measured continuous variable, not just spatial or geometric distances. This definition of Euclidean distance, therefore, requires that all variables used to determine clustering using k-means must be continuous
Procedure
In order to perform k-means clustering, the algorithm randomly assigns k initial centers (k specified by the user), either by randomly choosing points in the “Euclidean space” defined by all n variables, or by sampling k points of all available observations to serve as initial centers. It then iteratively assigns each observation to the nearest center. Next, it calculates the new center for each cluster as the centroid mean of the clustering variables for each cluster’s new set of observations. K-means re-iterates this process, assigning observations to the nearest center (some observations will change cluster). This process repeats until a new iteration no longer re-assigns any observations to a new cluster. At this point, the algorithm is considered to have converged, and the final cluster assignments constitute the clustering solution.
There are several k-means algorithms available. The standard algorithm is the Hartigan-Wong algorithm, which aims to minimize the Euclidean distances of all points with their nearest cluster centers, by minimizing within-cluster sum of squared errors (SSE).
Software
-means is implemented in many statistical software programs:In R, in the cluster package, use the function: k-means(x, centers, iter.max=10, nstart=1). The data object on which to perform clustering is declared in x. The number of clusters k is specified by the user in centers=#. k-means() will repeat with different initial centroids (sampled randomly from the entire dataset) nstart=# times and choose the best run (smallest SSE). iter.max=# sets a maximum number of iterations allowed (default is 10) per run.In STATA, use the command: cluster kmeans [varlist], k(#) [options]. Use [varlist] to declare the clustering variables, k(#) to declare k. There are other options to specify similarity measures instead of Euclidean distances.In SAS, use the command: PROC FASTCLUS maxclusters=k; var [varlist]. This requires specifying k and the clustering variables in [varlist].In SPSS, use the function: Analyze -> Classify -> K-Means Cluster. Additional help files are available online.
Considerations
means clustering requires all variables to be continuous. Other methods that do not require all variables to be continuous, including some heirarchical clustering methods, have different assumptions and are discussed in the resources list below. K-means clustering also requires a priori specification of the number of clusters, k. Though this can be done empirically with the data (using a screeplot to graph within-group SSE against each cluster solution), the decision should be driven by theory, and improper choices can lead to erroneous clusters. See Peeples’ online R walkthrough R script for K-means cluster analysis below for examples of choosing cluster solutions.
The choice of clustering variables is also of particular importance. Generally, cluster analysis methods require the assumption that the variables chosen to determine clusters are a comprehensive representation of the underlying construct of interest that groups similar observations. While variable choice remains a debated topic, the consensus in the field recommends clustering on as many variables as possible, as long as the set fits this description, and the variables that do not describe much of the variance in Euclidean distances between observations will contribute less to cluster assignment. Sensitivity analyses are recommended using different cluster solutions and sets of clustering variables to determine robustness of the clustering algorithm.K-means by default aims to minimize within-group sum of squared error as measured by Euclidean distances, but this is not always justified when data assumptions are not met. Consult textbooks and online guides in resources section below, especially Robinson’s R-blog: K-means clustering is not a free lunch for examples of the issues encountered with k-means clustering when assumptions are violated.Lastly, cluster analysis methods are similar to other data reduction techniques in that they are largely exploratory tools, thus results should be interpreted with caution. Many techniques exist for validating results from cluster analysis, including internally with cross-validation or bootstrapping, validating on conceptual groups theorized a priori or with expert opinion, or external validation with separate datasets. A common application of cluster analysis is as a tool for predicting cluster membership on future observations using existing data, but it does not describe why the observations are grouped that way. As such, cluster analysis is often used in conjunction with factor analysis, where cluster analysis is used to describe how observations are similar, and factor analysis is used to describe why observations are similar. Ultimately, validity of cluster analysis results should be determined by theory and by utility of cluster descriptions.
0 notes
Text
Comprehensive Breakdown of a Data Science Curriculum: What to Expect from Start to Finish
Comprehensive Breakdown of a Data Science Curriculum: What to Expect from Start to Finish
A Data Science course typically covers a broad range of topics, combining elements from statistics, computer science, and domain-specific knowledge. Here’s a breakdown of what you can expect from a comprehensive Data Science curriculum:
1. Introduction to Data Science
Overview of Data Science: Understanding what Data Science is and its significance.
Applications of Data Science: Real-world examples and case studies.
2. Mathematics and Statistics
Linear Algebra: Vectors, matrices, eigenvalues, and eigenvectors.
Calculus: Derivatives and integrals, partial derivatives, gradient descent.
Probability and Statistics: Probability distributions, hypothesis testing, statistical inference, sampling, and data distributions.
3. Programming for Data Science
Python/R: Basics and advanced concepts of programming using Python or R.
Libraries and Tools: NumPy, pandas, Matplotlib, seaborn for Python; dplyr, ggplot2 for R.
Data Manipulation and Cleaning: Techniques for preprocessing, cleaning, and transforming data.
4. Data Visualization
Principles of Data Visualization: Best practices, visualization types.
Tools and Libraries: Tableau, Power BI, and libraries like Matplotlib, seaborn, Plotly.
5. Data Wrangling
Data Collection: Web scraping, APIs.
Data Cleaning: Handling missing data, data types, normalization.
6. Exploratory Data Analysis (EDA)
Descriptive Statistics: Mean, median, mode, standard deviation.
Data Exploration: Identifying patterns, anomalies, and visual exploration.
7. Machine Learning
Supervised Learning: Linear regression, logistic regression, decision trees, random forests, support vector machines.
Unsupervised Learning: K-means clustering, hierarchical clustering, PCA (Principal Component Analysis).
Model Evaluation: Cross-validation, bias-variance tradeoff, ROC/AUC.
8. Deep Learning
Neural Networks: Basics of neural networks, activation functions.
Deep Learning Frameworks: TensorFlow, Keras, PyTorch.
Applications: Image recognition, natural language processing.
9. Big Data Technologies
Introduction to Big Data: Concepts and tools.
Hadoop and Spark: Ecosystem, HDFS, MapReduce, PySpark.
10. Data Engineering
ETL Processes: Extract, Transform, Load.
Data Pipelines: Building and maintaining data pipelines.
11. Database Management
SQL and NoSQL: Database design, querying, and management.
Relational Databases: MySQL, PostgreSQL.
NoSQL Databases: MongoDB, Cassandra.
12. Capstone Project
Project Work: Applying the concepts learned to real-world data sets.
Presentation: Communicating findings effectively.
13. Ethics and Governance
Data Privacy: GDPR, data anonymization.
Ethical Considerations: Bias in data, ethical AI practices.
14. Soft Skills and Career Preparation
Communication Skills: Presenting data findings.
Team Collaboration: Working in data science teams.
Job Preparation: Resume building, interview preparation.
Optional Specializations
Natural Language Processing (NLP)
Computer Vision
Reinforcement Learning
Time Series Analysis
Tools and Software Commonly Used:
Programming Languages: Python, R
Data Visualization Tools: Tableau, Power BI
Big Data Tools: Hadoop, Spark
Databases: MySQL, PostgreSQL, MongoDB, Cassandra
Machine Learning Libraries: Scikit-learn, TensorFlow, Keras, PyTorch
Data Analysis Libraries: NumPy, pandas, Matplotlib, seaborn
Conclusion
A Data Science course aims to equip students with the skills needed to collect, analyze, and interpret large volumes of data, and to communicate insights effectively. The curriculum is designed to be comprehensive, covering both theoretical concepts and practical applications, often culminating in a capstone project that showcases a student’s ability to apply what they've learned.
Acquire Skills and Secure a Job with best package in a reputed company in Ahmedabad with the Best Data Science Course Available
Or contact US at 1802122121 all Us 18002122121
Call Us 18002122121
Call Us 18002122121
Call Us 18002122121
Call Us 18002122121
0 notes
Text
Regulatory Considerations in the DNA and Gene Cloning Services Market
The DNA and Gene Cloning Services Market is experiencing significant growth driven by the increasing demand for custom DNA constructs, genetically engineered organisms, and gene editing services in various fields, including biotechnology, pharmaceuticals, agriculture, and research. Gene cloning, the process of replicating and amplifying specific DNA sequences, plays a crucial role in molecular biology research, drug discovery, and biomanufacturing.
Get a free Sample: https://www.marketdigits.com/request/sample/3785
One of the primary drivers of market growth is the expanding applications of gene cloning and genetic engineering in biopharmaceutical production. Recombinant DNA technology allows for the production of therapeutic proteins, antibodies, vaccines, and gene therapies, offering potential treatments for a wide range of diseases, including cancer, genetic disorders, and infectious diseases. As a result, pharmaceutical companies and biotech firms are increasingly outsourcing gene cloning services to specialized providers to expedite their drug development pipelines and reduce time-to-market.
Furthermore, advancements in gene editing technologies, such as CRISPR-Cas9, TALENs, and zinc finger nucleases, have revolutionized the field of genetic engineering, enabling precise modifications of DNA sequences in various organisms. Gene editing services, including gene knockout, knock-in, and point mutation, are in high demand for applications such as functional genomics, cell line engineering, and agricultural biotechnology. Additionally, the emergence of synthetic biology and genome editing platforms has created new opportunities for engineering custom DNA constructs and genetic circuits for biomanufacturing and biotechnology applications.
The DNA and Gene Cloning Services Market is Valued USD 3.02 billion in 2024 and projected to reach USD 7.84 billion by 2030, growing at a CAGR of CAGR of 14.6% During the Forecast period of 2024-2032.
The DNA and gene cloning services market is characterized by the presence of specialized providers offering a wide range of services, including gene synthesis, gene cloning, gene editing, plasmid preparation, and DNA sequencing. Major players in the market include Thermo Fisher Scientific Inc., GenScript Biotech Corporation, Eurofins Scientific SE, Integrated DNA Technologies, Inc. (IDT), and OriGene Technologies, Inc., among others. These companies provide end-to-end solutions for DNA manipulation, from design and synthesis to cloning and validation, catering to the diverse needs of academic research labs, biotech startups, and pharmaceutical companies worldwide.
Major vendors in the global DNA and Gene Cloning Services Market are Aragen Life Sciences, Bio-Techne, Charles River Laboratories, Curia, Eurofins, GenScript, Integrated DNA Technologies, MedGenome, Sino Biological, Syngene, Twist Bioscience and Others
Emerging trends in the DNA and gene cloning services market include the development of high-throughput cloning platforms, automation technologies, and cloud-based bioinformatics tools for DNA design and analysis. These advancements enable researchers to streamline the gene cloning process, reduce experimental variability, and accelerate scientific discovery. Additionally, there is growing interest in gene synthesis and DNA assembly methods that enable the construction of large, complex DNA sequences, such as gene clusters, pathways, and synthetic genomes, for applications in synthetic biology and metabolic engineering.
Looking ahead, the DNA and gene cloning services market is poised for continued growth driven by advancements in genomics, gene editing, and biomanufacturing technologies. As the demand for custom DNA constructs and genetically engineered organisms continues to rise, specialized service providers will play a critical role in supporting research, drug development, and biotechnology innovation. Collaborations between industry stakeholders, academic institutions, and regulatory agencies will be essential in addressing technical challenges, ensuring quality standards, and facilitating the translation of genetic engineering advancements into real-world applications.
0 notes
Text
Find Best Sanitary Pad Raw Material Near Me
Introduction: When it comes to manufacturing sanitary pads, sourcing high-quality raw materials is essential for ensuring the effectiveness and comfort of the end product. Finding the best sanitary pad raw materials near you can help streamline your manufacturing process and ensure the quality of your products. Here are some tips for finding the best sanitary pad raw materials near you:
Local Suppliers: Look for local suppliers of sanitary pad raw materials. Local suppliers can offer more competitive prices and faster delivery times, helping you reduce costs and improve efficiency.
Trade Shows and Exhibitions: Attend trade shows and exhibitions related to the hygiene products industry. These events often feature suppliers of sanitary pad raw materials, giving you the opportunity to meet them in person and discuss your requirements.
Online Directories: Use online directories to find suppliers of sanitary pad raw materials near you. These directories allow you to search for suppliers based on location, making it easier to find suppliers in your area.
Industry Associations: Join industry associations related to the hygiene products industry. These associations often have resources and networks that can help you find suppliers of sanitary pad raw materials near you.
Local Manufacturing Hubs: Visit local manufacturing hubs or industrial estates. These areas often have clusters of suppliers and manufacturers, making it easier to find suppliers of sanitary pad raw materials near you.
Supplier Visits: Arrange visits to potential suppliers' facilities. This will allow you to assess their manufacturing processes, quality control measures, and capabilities firsthand.
Quality Control: Ensure that the supplier maintains strict quality control measures. Request samples of their raw materials for testing before making a purchase.
Customer Reviews: Look for customer reviews and testimonials online. This can give you insight into the reputation and reliability of potential suppliers.
Conclusion: Finding the best sanitary pad raw materials near you requires thorough research and consideration. By following these tips, you can find reliable suppliers that offer high-quality raw materials to meet your manufacturing needs.
Factory Address:
Gata No. 34, Mauza Khadwai, Tehsil Kirawali, Runkata, Agra, Uttar Pradesh, India-282007 📩 [email protected]
Contact: 8800775462
0 notes
Text
Health Lifestyle Part A: Quantitative Article by Burdette, Needham, Hill, and Taylor Background Lifestyles have a correlation effect on our health, and we must define health not from a singular perspective but from a society base to collect much data, which shows health trends and behavior in the community. The researchers recognize that little research has been done to determine the lifestyle behavior on our overall health care and that the impact can be huge if no quantitative and qualitative research is done. The researchers have set out a few types of research carried out on related topics. They identify the studies done on an association of holding multiple social roles and health outcomes. However, the authors have objectively laid out the problem statement, which is, how acquiring multiple roles in early adulthood influence health behavior. Literature review The researchers have used several past projects carried out by different others. The topics in the literature review are problems related to the topic the researchers were handling. Some questions have touched on population and general lifestyle behaviors and their effects on health. “Health and lifestyles," and "Human Health Development" are the key topics the researchers discussed. Other topics include: "Diabetes Prevention Program," "Diabetes Prevention," “Health Behaviors in Adolescents” and “Lifestyle Health Behavior in Adults." The topics had limitations and had not addressed the particular concern that the statement was raising. Methodology A quantitative approach was the preferred method for the authors. Data obtained from Add Health, an institution that primarily collects adolescence data from schools, families and other learning institutions. Sampled data was already available, and quantitative analysis was used to define a critical process of calculation. The measurement was also used as the subtype to calculate the variables. Self-rating was part of the health analysis used to obtain data. Data analysis Data were evaluated using a Latent Class Analysis (LCA), this involved model latent healthy lifestyle from observed health behavior indicators. LCA is similar to other reduction data analysis techniques. LCA uses the variable with a multinomial distribution to establish clusters of individuals based on observed indicators Secondly, predicting healthy lifestyle based on self-health measuring, and role occupancy during the transition to early adulthood was another suitable method (Burdette, Needham, Hill, Taylor, 2017).Latent class analysis was also used as both a dependent and independent variable in the data analyses. Conclusion The results gave a significant approach to lifestyle health. The researchers suggested the importance of modeling overall health instead of focusing on an individual health behavior. Secondly, the result demonstrated how holding many social lifestyles influence health behavior. The conclusion makes sense because the results explain a correlation between essential roles and health behavior, which is already shown in primary health care (Burdette et al., 2017). The researchers have also concluded that adolescence lifestyle has a significant influence on self-rated health. Researcher’s Conclusion Analysis The investigator's conclusion emphasizes on the significance of concentrating on overall healthy lifestyles rather than individual approach to health behavior. The literature review showed that there was little research carried out on the purpose statement but data collected had demonstrated that overall approach to social lifestyle gave particular health behavior (Burdette et al., 2017). The quantitative method was the best approach to use for this type of research because it gives both numerical and descriptive data as supported by the results. Protection and Consideration The research does not plainly admit to getting parental consent from adolescence bracket who are below 18 years, and this is a breach of ethical standards in research and research formulation. Even though the study was voluntary for students, were they informed? Ethical standards, especially when getting data from children below eighteen years is a serious violation. Strengths and Limitations The authors used a broad sample base, which enhances the accuracy of the results. Strength in the research is the sample size. In one year, a record of 15,000 young adults, 18000 parents and 143 school officials were interviewed (Burdette et al., 2017). The large sample size represents a significant data with variables that can give an overall picture of the lifestyle behavior on the health of young adults. Conversely, a young group may not have the right perspective about their health and may give false information. For example, the interviews conducted at home in the presence of their parents may have a lot of errors; telling the interviewer that you drink and your parents are sitting across is the unlikely occurrence. Read the full article
0 notes
Text
Selecting and training algorithms is a key step in building machine learning models

Selecting and training algorithms is a key step in building machine learning models. Here’s a brief overview of the process:
Selecting the Right Algorithm The choice of algorithm depends on the type of problem you’re solving (e.g., classification, regression, clustering, etc.), the size and quality of your data, and the computational resources available.
Common algorithm choices include:
For Classification: Logistic Regression Decision Trees Random Forests Support Vector Machines (SVM) k-Nearest Neighbors (k-NN) Neural Networks For Regression:
Linear Regression Decision Trees Random Forests Support Vector Regression (SVR) Neural Networks For Clustering:
k-Means DBSCAN Hierarchical Clustering For Dimensionality Reduction: Principal Component Analysis (PCA) t-Distributed Stochastic Neighbor Embedding (t-SNE) Considerations when selecting an algorithm:
Size of data: Some algorithms scale better with large datasets (e.g., Random Forests, Gradient Boosting).
Interpretability: If understanding the model is important, simpler models (like Logistic Regression or Decision Trees) might be preferred.
Performance:
Test different algorithms and use cross-validation to compare performance (accuracy, precision, recall, etc.).
2. Training the Algorithm After selecting an appropriate algorithm, you need to train it on your dataset.
Here’s how you can train an algorithm:
Preprocess the data: Clean the data (handle missing values, outliers, etc.). Normalize/scale the features (especially important for algorithms like SVM or k-NN). Encode categorical variables if necessary (e.g., using one-hot encoding).
Split the data:
Divide the data into training and test sets (typically 80–20 or 70–30 split). Train the model: Fit the model to the training data using the chosen algorithm and its hyperparameters.
Optimize the hyperparameters using techniques like Grid Search or Random Search.
Evaluate the model:
Use the test data to evaluate the model’s performance using metrics like accuracy, precision, recall, F1 score (for classification), mean squared error (for regression), etc. Perform cross-validation to get a more reliable performance estimate.
3. Model Tuning and Hyperparameter
Optimization Hyperparameter tuning:
Many algorithms come with hyperparameters that affect their performance (e.g., the depth of a decision tree, learning rate for gradient descent).
You can use methods like:
Grid Search:
Try all possible combinations of hyperparameters within a given range.
Random Search:
Randomly sample hyperparameters from a range, which is often more efficient for large search spaces.
Cross-validation:
Use k-fold cross-validation to get a better understanding of how the model generalizes to unseen data.
4. Model Evaluation and Fine-tuning Once you have trained the model, fine-tune it by adjusting hyperparameters or using advanced techniques like regularization to avoid overfitting.
If the model isn’t performing well, try: Selecting different features. Trying more advanced models (e.g., ensemble methods like Random Forest or Gradient Boosting).
Gathering more data if possible.
By iterating through these steps and refining the model based on evaluation, you can build a robust machine learning model for your problem.
WEBSITE: https://www.ficusoft.in/deep-learning-training-in-chennai/
0 notes
Link
When mix and matching wood tones in furniture and decor, there are several key considerations to keep in mind to ensure a harmonious and visually pleasing result. Here are some tips:
Undertones: Pay attention to the undertones of each wood tone you're working with. Some may have warm undertones (such as red or yellow), while others may have cool undertones (like gray or blue). Try to pair wood tones with similar undertones for a cohesive look.
Contrast: While matching wood tones can create a cohesive look, incorporating contrasting tones can add interest and depth to your space. Experiment with pairing light and dark wood tones or mixing warm and cool tones for visual contrast.
Balance: Aim for balance in your space by distributing different wood tones evenly throughout the room. Avoid clustering all of one type of wood in a single area, as this can create an imbalance and make the space feel disjointed.
Texture: Consider the texture of the wood in addition to its color. Mixing different textures, such as smooth finishes with rough or distressed finishes, can add dimension and visual interest to your space.
Use a Unifying Element: Introduce a unifying element, such as a rug, piece of artwork, or accent color, to tie together different wood tones in the room. This can help create a cohesive overall look and prevent the space from feeling too eclectic.
Sample Swatches: Before committing to a mix of wood tones, gather sample swatches or small pieces of wood to see how they look together in your space. This can help you visualize the final result and make any necessary adjustments before purchasing furniture or decor.
Consider the Style: Different wood tones may complement certain design styles better than others. For example, warm, rich wood tones often pair well with traditional or rustic styles, while cooler, lighter tones may suit modern or Scandinavian-inspired spaces.
Experiment: Don't be afraid to experiment with different combinations of wood tones until you find the look that feels right for your space. Trust your instincts and go with what you love.
By keeping these considerations in mind, you can mix and match wood tones effectively to create a cohesive, visually appealing space that reflects your personal style.
0 notes
Text
Installing Hadoop
Installing Apache Hadoop involves steps and considerations to ensure a successful setup. Here’s a general guide on how to install Hadoop:
Prerequisites
Java Installation: Hadoop requires Java to be installed on your system. You can check your Java version by running the JavaJava version on your terminal.
SSH: Hadoop requires SSH access to manage its nodes. It would help if you had SSH installed and configured on your system.
Installation Steps
Download Hadoop: Go to the Apache Hadoop official website and download the latest stable release.
Extract Files: Extract the downloaded tar file to a directory of your choice, typically under /usr/local/Hadoop.
Configuration: Configure Hadoop by editing the following files:
hadoop-env.sh: Set the Java Home variable.
core-site.xml: Set the Hadoop temporary directory and the default file system name.
hdfs-site.xml: Configure settings for HDFS, like replication factor and block size.
mapred-site.xml: Configure settings for MapReduce.
yarn-site.xml: Configure settings for YARN.
Setup SSH: If not already done, generate SSH keys and enable password-less SSH access to your local machine.
Format the Namenode: Before starting Hadoop for the first time, format the HDFS filesystem via hdfs namenode -format.
Starting Hadoop: Start the Hadoop daemons — the NameNode, DataNode, ResourceManager, and NodeManager. This can be done using the start. Sh and start-yarn.sh scripts.
Verification: After starting Hadoop, you can use the JPS command to check if the Hadoop daemons are running. You should see processes like NameNode, DataNode, ResourceManager, and NodeManager.
Web Interfaces: Hadoop provides web UIs for HDFS and YARN, which can be accessed to monitor the cluster’s status and jobs.
Post-Installation
Testing the Installation: Run some basic Hadoop commands or a sample MapReduce job to ensure everything works correctly.
Cluster Setup: If you’re setting up a multi-node cluster, you must replicate the configuration process on each node and modify settings for proper network communication.
Firewall Settings: Ensure that any firewalls on your system are configured to allow traffic on the necessary ports used by Hadoop.
Regular Maintenance: Regularly check and maintain your Hadoop cluster for optimal performance.
Remember, each step might require specific commands or configurations based on your operating system and the version of Hadoop you’re installing. Also, always check the official Hadoop documentation for accurate and detailed instructions.
For bulk email communication regarding this process, ensure the email is clear, concise, and contains all the necessary links and instructions. Avoid using overly complex language or large attachments to reduce the risk of being marked as spam. Using a trusted email service provider and following best practices for bulk email sending is also beneficial.
Hadoop Training Demo Day 1 Video:
youtube
You can find more information about Hadoop Training in this Hadoop Docs Link
Conclusion:
Unogeeks is the №1 IT Training Institute for Hadoop Training. Anyone Disagree? Please drop in a comment
You can check out our other latest blogs on Hadoop Training here — Hadoop Blogs
Please check out our Best In Class Hadoop Training Details here — Hadoop Training
S.W.ORG
— — — — — — — — — — — -
For Training inquiries:
Call/Whatsapp: +91 73960 33555
Mail us at: [email protected]
Our Website ➜ https://unogeeks.com
Follow us:
Instagram: https://www.instagram.com/unogeeks
Facebook: https://www.facebook.com/UnogeeksSoftwareTrainingInstitute
Twitter: https://twitter.com/unogeeks
#unogeeks #training #ittraining #unogeekstraining
0 notes
Text
Automotive Human Machine Interface (HMI) Market Size, Trends, Share, Report 2024-2032
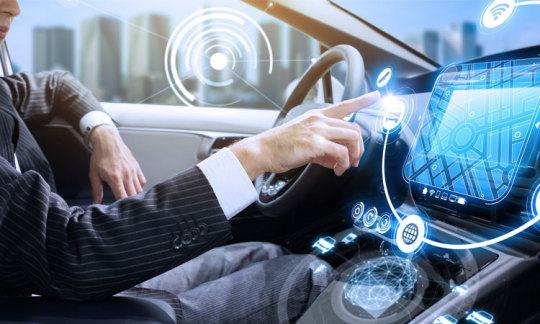
IMARC Group, a leading market research company, has recently releases report titled “Automotive HMI Market: Global Industry Trends, Share, Size, Growth, Opportunity and Forecast 2024-2032” The global automotive HMI market size reached US$ 21.6 Billion in 2023. Looking forward, IMARC Group expects the market to reach US$ 48.3 Billion by 2032, exhibiting a growth rate (CAGR) of 9.08% during 2024-2032.
Request For Sample Copy of Report: https://www.imarcgroup.com/automotive-hmi-market/requestsample
Factors Affecting the Growth of the Automotive HMI Industry:
Advancements in Technology:
Technological advancements are leading to the development of more responsive and intuitive touchscreen displays in vehicles. High-resolution screens with capacitive touch capabilities provide a smartphone-like experience for users, making interactions with the human-machine interface (HMI) more user-friendly. Advanced voice recognition systems powered by artificial intelligence (AI) and natural language processing (NLP) are becoming integral to modern HMIs. Drivers can control various functions and access information through voice commands, enhancing safety and convenience.
Safety Regulations:
Safety regulations impose limits on the visual and manual distractions that in-vehicle systems can present to drivers. This is driving the development of HMIs with interfaces designed to minimize distractions, such as simplified menus and voice-controlled features. Many safety regulations encourage the use of hands-free and voice control systems for tasks like making phone calls, sending texts, and accessing navigation. As a result, automakers are investing in advanced voice recognition technology and integrating it into their HMIs to ensure compliance with these regulations.
Connected and Autonomous Vehicles:
Connected and autonomous vehicles generate vast amounts of data related to navigation, sensor inputs, and vehicle status. HMIs must effectively display this complex information to the driver in a comprehensible and user-friendly manner. Advanced graphics and intuitive layouts are essential to convey this data effectively. Autonomous vehicles rely on a multitude of sensors, such as light detection and ranging (LiDAR), radar, and cameras. The HMI provides real-time feedback from these sensors to keep the driver informed about the surroundings of vehicles and the status of autonomous driving features.
Leading Companies Operating in the Global Industry:
Alps Electric Co. Ltd.
Capgemini Engineering (Capgemini SE)
Continental Aktiengesellschaft
DENSO Corporation
EAO AG
Faurecia Clarion Electronics Co. Ltd. (Faurecia SE)
Harman International Industries (Samsung Electronics Co. Ltd.)
Luxoft (DXC Technology)
Marelli Holdings Co. Ltd.
Panasonic Holdings Corporation
Robert Bosch GmbH (Robert Bosch Stiftung GmbH)
Valeo and Visteon Corporation
Automotive HMI Market Report Segmentation:
By Product:
Instrument Cluster
Central Display
Head-Up Display
Others
Instrument cluster represented the largest segment as it is an essential component of every vehicle.
By Access Type:
Standard
Multimodal
Multimodal accounted for the largest market share due to its utilization in combining touchscreens, voice recognition, and physical controls.
By Technology:
Visual Interface
Acoustic
Mechanical
Others
Visual interface exhibits a clear dominance in the market on account of its effectiveness in conveying information to drivers and passengers.
By Vehicle Type:
Passenger Cars
Commercial Vehicles
Passenger cars holds the biggest market share as they have a considerably larger market volume compared to other vehicle types.
Regional Insights:
North America (United States, Canada)
Asia Pacific (China, Japan, India, South Korea, Australia, Indonesia, Others)
Europe (Germany, France, United Kingdom, Italy, Spain, Russia, Others)
Latin America (Brazil, Mexico, Others)
Middle East and Africa
Asia Pacific enjoys the leading position in the automotive HMI market due to the thriving automotive industry.
Global Automotive HMI Market Trends:
Automotive HMIs are increasingly integrating advanced technologies, such as augmented reality (AR), gesture recognition, and artificial intelligence (AI), to enhance user experiences and provide more intuitive control options. Voice recognition systems are becoming more sophisticated, allowing drivers and passengers to interact with the HMI using natural language commands, contributing to safer and hands-free operation.
Multimodal HMIs that combine touchscreens, voice control, and physical buttons are gaining traction, offering users flexibility to interact with vehicle systems.
Other Key Points Covered in the Report:
COVID-19 Impact
Porters Five Forces Analysis
Value Chain Analysis
Strategic Recommendations
About Us
IMARC Group is a leading market research company that offers management strategy and market research worldwide. We partner with clients in all sectors and regions to identify their highest-value opportunities, address their most critical challenges, and transform their businesses.
IMARC Group’s information products include major market, scientific, economic and technological developments for business leaders in pharmaceutical, industrial, and high technology organizations. Market forecasts and industry analysis for biotechnology, advanced materials, pharmaceuticals, food and beverage, travel and tourism, nanotechnology and novel processing methods are at the top of the company’s expertise.
Contact US
IMARC Group
134 N 4th St. Brooklyn, NY 11249, USA
USA: +1-631-791-1145 | Asia: +91-120-433-0800
Email: [email protected]
Follow us on Twitter: @imarcglobal
LinkedIn: https://www.linkedin.com/company/imarc-group/mycompany/
0 notes
Text
CRISPR Frontier: Charting the Course in Gene Editing Technologies
The CRISPR gene editing market is experiencing unprecedented growth and innovation, poised to revolutionize the field of biotechnology and healthcare. CRISPR, short for Clustered Regularly Interspaced Short Palindromic Repeats, represents a groundbreaking technology that enables precise modifications to the DNA of living organisms. Its versatility and ease of use have unlocked new possibilities for addressing a wide range of applications, from basic research and drug discovery to agricultural biotechnology and therapeutic interventions.
Get a free Sample: https://www.marketdigits.com/request/sample/3890
One of the primary drivers of growth in the CRISPR gene editing market is its potential for precision medicine. CRISPR-based therapies hold promise for treating a variety of genetic disorders, including inherited diseases such as cystic fibrosis, sickle cell anemia, and Duchenne muscular dystrophy. By correcting or modifying disease-causing mutations at the DNA level, CRISPR offers the potential to provide long-term, curative treatments for patients who previously had few or no therapeutic options.
In 2024, the CRISPR Gene Editing Market is valued at USD 1.8 billion and is anticipated to reach USD 9.2 billion by 2030, with a projected compound annual growth rate (CAGR) of 22.6% during the forecast period from 2024 to 2032.
Moreover, the CRISPR gene editing market is expanding rapidly beyond traditional therapeutic applications into areas such as agriculture, biotechnology, and industrial applications. In agriculture, CRISPR-based technologies are being used to develop crops with improved yield, nutritional content, and resistance to pests and diseases, offering solutions to global food security challenges. In biotechnology, CRISPR is revolutionizing drug discovery and development by enabling precise modifications to cellular pathways and drug targets, leading to the identification of novel therapeutics for a wide range of diseases.
Major vendors in the global CRISPR Gene Editing Market are Agilent Technologies, Inc., Beam Therapeutics., Caribou Biosciences, Inc., CRISPR Therapeutics., Editas Medicine, eGenesis, GenScript, Integrated DNA Technologies, Inc., Intellia Therapeutics.Inc, Lonza Group, Ltd, Merck KGaA, OriGene Technologies, Inc., PerkinElmer Inc., Poseida Therapeutics, Inc., Precision BioSciences, Synthego, Thermo, Fisher Scientific, Inc, ToolGen, Inc., VERVE THERAPEUTICS, INC., and Others.
Additionally, the CRISPR gene editing market is fueled by ongoing advancements in technology and research, as well as increasing investment from both public and private sectors. CRISPR-based tools and platforms are continually being refined and optimized, allowing researchers to perform gene editing experiments with greater efficiency, accuracy, and scalability. Moreover, the development of novel CRISPR-based systems, such as base editing and prime editing, expands the toolkit of gene editing techniques and further enhances the capabilities of this transformative technology.
Despite the immense promise of CRISPR gene editing, challenges remain, including ethical considerations, off-target effects, and regulatory hurdles. The ethical implications of manipulating the human genome raise complex questions about safety, equity, and consent, necessitating careful consideration and oversight. Additionally, off-target effects, where CRISPR inadvertently modifies unintended regions of the genome, pose potential risks to patient safety and the integrity of genetic information. Addressing these challenges requires ongoing research, collaboration, and dialogue among stakeholders to ensure the responsible and ethical use of CRISPR technology.
In conclusion, the CRISPR gene editing market represents a paradigm shift in biotechnology and healthcare, with far-reaching implications for human health, agriculture, and industrial applications. By harnessing the power of CRISPR to precisely modify the genetic code, we have the potential to revolutionize the treatment of genetic diseases, enhance crop productivity, and develop innovative therapeutics for a wide range of conditions. As research advances and the technology matures, the future holds promise for CRISPR to transform the way we understand and manipulate the building blocks of life.
0 notes