#GPU-based cloud computing
Explore tagged Tumblr posts
Text
Unveiling the Future of AI: Why Sharon AI is the Game-Changer You Need to Know
Artificial Intelligence (AI) is no longer just a buzzword; it’s the backbone of innovation in industries ranging from healthcare to finance. As businesses look to scale and innovate, leveraging advanced AI services has become crucial. Enter Sharon AI, a cutting-edge platform that’s reshaping how organizations harness AI’s potential. If you haven’t heard of Sharon AI yet, it’s time to dive in.
Why AI is Essential in Today’s World
The adoption of artificial intelligence has skyrocketed over the past decade. From chatbots to complex data analytics, AI is driving efficiency, accuracy, and innovation. Businesses that leverage AI are not just keeping up; they’re leading their industries. However, one challenge remains: finding scalable, high-performance computing solutions tailored to AI.
That’s where Sharon AI steps in. With its GPU-based computing infrastructure, the platform offers solutions that are not only powerful but also sustainable, addressing the growing need for eco-friendly tech.
What Sets Sharon AI Apart?
Sharon AI specializes in providing advanced compute infrastructure for high-performance computing (HPC) and AI applications. Here’s why Sharon AI stands out:
Scalability: Whether you’re a startup or a global enterprise, Sharon AI offers flexible solutions to match your needs.
Sustainability: Their commitment to building net-zero energy data centers, like the 250 MW facility in Texas, highlights a dedication to green technology.
State-of-the-Art GPUs: Incorporating NVIDIA H100 GPUs ensures top-tier performance for AI and HPC workloads.
Reliability: Operating from U.S.-based data centers, Sharon AI guarantees secure and efficient service delivery.
Services Offered by Sharon AI
Sharon AI’s offerings are designed to empower businesses in their AI journey. Key services include:
GPU Cloud Computing: Scalable GPU resources tailored for AI and HPC applications.
Sustainable Data Centers: Energy-efficient facilities ensuring low carbon footprints.
Custom AI Solutions: Tailored services to meet industry-specific needs.
24/7 Support: Expert assistance to ensure seamless operations.
Why Businesses Are Turning to Sharon AI
Businesses today face growing demands for data-driven decision-making, predictive analytics, and real-time processing. Traditional computing infrastructure often falls short, making Sharon AI’s advanced solutions a must-have for enterprises looking to stay ahead.
For instance, industries like healthcare benefit from Sharon AI’s ability to process massive datasets quickly and accurately, while financial institutions use their solutions to enhance fraud detection and predictive modeling.
The Growing Demand for AI Services
Searches related to AI solutions, HPC platforms, and sustainable computing are increasing as businesses seek reliable providers. By offering innovative solutions, Sharon AI is positioned as a leader in this space.If you’re searching for providers or services such as GPU cloud computing, NVIDIA GPU solutions, or AI infrastructure services, Sharon AI is a name you’ll frequently encounter. Their offerings are designed to cater to the rising demand for efficient and sustainable AI computing solutions.
0 notes
Text
Tech Breakdown: What Is a SuperNIC? Get the Inside Scoop!
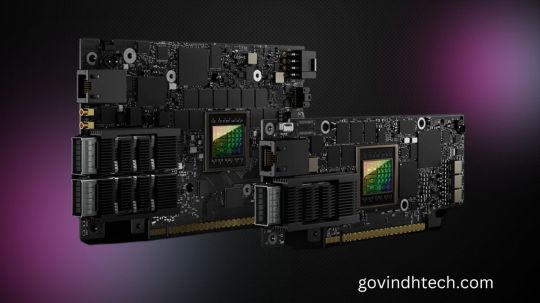
The most recent development in the rapidly evolving digital realm is generative AI. A relatively new phrase, SuperNIC, is one of the revolutionary inventions that makes it feasible.
Describe a SuperNIC
On order to accelerate hyperscale AI workloads on Ethernet-based clouds, a new family of network accelerators called SuperNIC was created. With remote direct memory access (RDMA) over converged Ethernet (RoCE) technology, it offers extremely rapid network connectivity for GPU-to-GPU communication, with throughputs of up to 400Gb/s.
SuperNICs incorporate the following special qualities:
Ensuring that data packets are received and processed in the same sequence as they were originally delivered through high-speed packet reordering. This keeps the data flow’s sequential integrity intact.
In order to regulate and prevent congestion in AI networks, advanced congestion management uses network-aware algorithms and real-time telemetry data.
In AI cloud data centers, programmable computation on the input/output (I/O) channel facilitates network architecture adaptation and extension.
Low-profile, power-efficient architecture that effectively handles AI workloads under power-constrained budgets.
Optimization for full-stack AI, encompassing system software, communication libraries, application frameworks, networking, computing, and storage.
Recently, NVIDIA revealed the first SuperNIC in the world designed specifically for AI computing, built on the BlueField-3 networking architecture. It is a component of the NVIDIA Spectrum-X platform, which allows for smooth integration with the Ethernet switch system Spectrum-4.
The NVIDIA Spectrum-4 switch system and BlueField-3 SuperNIC work together to provide an accelerated computing fabric that is optimized for AI applications. Spectrum-X outperforms conventional Ethernet settings by continuously delivering high levels of network efficiency.
Yael Shenhav, vice president of DPU and NIC products at NVIDIA, stated, “In a world where AI is driving the next wave of technological innovation, the BlueField-3 SuperNIC is a vital cog in the machinery.” “SuperNICs are essential components for enabling the future of AI computing because they guarantee that your AI workloads are executed with efficiency and speed.”
The Changing Environment of Networking and AI
Large language models and generative AI are causing a seismic change in the area of artificial intelligence. These potent technologies have opened up new avenues and made it possible for computers to perform new functions.
GPU-accelerated computing plays a critical role in the development of AI by processing massive amounts of data, training huge AI models, and enabling real-time inference. While this increased computing capacity has created opportunities, Ethernet cloud networks have also been put to the test.
The internet’s foundational technology, traditional Ethernet, was designed to link loosely connected applications and provide wide compatibility. The complex computational requirements of contemporary AI workloads, which include quickly transferring large amounts of data, closely linked parallel processing, and unusual communication patterns all of which call for optimal network connectivity were not intended for it.
Basic network interface cards (NICs) were created with interoperability, universal data transfer, and general-purpose computing in mind. They were never intended to handle the special difficulties brought on by the high processing demands of AI applications.
The necessary characteristics and capabilities for effective data transmission, low latency, and the predictable performance required for AI activities are absent from standard NICs. In contrast, SuperNICs are designed specifically for contemporary AI workloads.
Benefits of SuperNICs in AI Computing Environments
Data processing units (DPUs) are capable of high throughput, low latency network connectivity, and many other sophisticated characteristics. DPUs have become more and more common in the field of cloud computing since its launch in 2020, mostly because of their ability to separate, speed up, and offload computation from data center hardware.
SuperNICs and DPUs both have many characteristics and functions in common, however SuperNICs are specially designed to speed up networks for artificial intelligence.
The performance of distributed AI training and inference communication flows is highly dependent on the availability of network capacity. Known for their elegant designs, SuperNICs scale better than DPUs and may provide an astounding 400Gb/s of network bandwidth per GPU.
When GPUs and SuperNICs are matched 1:1 in a system, AI workload efficiency may be greatly increased, resulting in higher productivity and better business outcomes.
SuperNICs are only intended to speed up networking for cloud computing with artificial intelligence. As a result, it uses less processing power than a DPU, which needs a lot of processing power to offload programs from a host CPU.
Less power usage results from the decreased computation needs, which is especially important in systems with up to eight SuperNICs.
One of the SuperNIC’s other unique selling points is its specialized AI networking capabilities. It provides optimal congestion control, adaptive routing, and out-of-order packet handling when tightly connected with an AI-optimized NVIDIA Spectrum-4 switch. Ethernet AI cloud settings are accelerated by these cutting-edge technologies.
Transforming cloud computing with AI
The NVIDIA BlueField-3 SuperNIC is essential for AI-ready infrastructure because of its many advantages.
Maximum efficiency for AI workloads: The BlueField-3 SuperNIC is perfect for AI workloads since it was designed specifically for network-intensive, massively parallel computing. It guarantees bottleneck-free, efficient operation of AI activities.
Performance that is consistent and predictable: The BlueField-3 SuperNIC makes sure that each job and tenant in multi-tenant data centers, where many jobs are executed concurrently, is isolated, predictable, and unaffected by other network operations.
Secure multi-tenant cloud infrastructure: Data centers that handle sensitive data place a high premium on security. High security levels are maintained by the BlueField-3 SuperNIC, allowing different tenants to cohabit with separate data and processing.
Broad network infrastructure: The BlueField-3 SuperNIC is very versatile and can be easily adjusted to meet a wide range of different network infrastructure requirements.
Wide compatibility with server manufacturers: The BlueField-3 SuperNIC integrates easily with the majority of enterprise-class servers without using an excessive amount of power in data centers.
#Describe a SuperNIC#On order to accelerate hyperscale AI workloads on Ethernet-based clouds#a new family of network accelerators called SuperNIC was created. With remote direct memory access (RDMA) over converged Ethernet (RoCE) te#it offers extremely rapid network connectivity for GPU-to-GPU communication#with throughputs of up to 400Gb/s.#SuperNICs incorporate the following special qualities:#Ensuring that data packets are received and processed in the same sequence as they were originally delivered through high-speed packet reor#In order to regulate and prevent congestion in AI networks#advanced congestion management uses network-aware algorithms and real-time telemetry data.#In AI cloud data centers#programmable computation on the input/output (I/O) channel facilitates network architecture adaptation and extension.#Low-profile#power-efficient architecture that effectively handles AI workloads under power-constrained budgets.#Optimization for full-stack AI#encompassing system software#communication libraries#application frameworks#networking#computing#and storage.#Recently#NVIDIA revealed the first SuperNIC in the world designed specifically for AI computing#built on the BlueField-3 networking architecture. It is a component of the NVIDIA Spectrum-X platform#which allows for smooth integration with the Ethernet switch system Spectrum-4.#The NVIDIA Spectrum-4 switch system and BlueField-3 SuperNIC work together to provide an accelerated computing fabric that is optimized for#Yael Shenhav#vice president of DPU and NIC products at NVIDIA#stated#“In a world where AI is driving the next wave of technological innovation#the BlueField-3 SuperNIC is a vital cog in the machinery.” “SuperNICs are essential components for enabling the future of AI computing beca
1 note
·
View note
Text
World's Most Powerful Business Leaders: Insights from Visionaries Across the Globe
In the fast-evolving world of business and innovation, visionary leadership has become the cornerstone of driving global progress. Recently, Fortune magazine recognized the world's most powerful business leaders, acknowledging their transformative influence on industries, economies, and societies.
Among these extraordinary figures, Elon Musk emerged as the most powerful business leader, symbolizing the future of technological and entrepreneurial excellence.
Elon Musk: The Game-Changer
Elon Musk, the CEO of Tesla, SpaceX, and X (formerly Twitter), has redefined innovation with his futuristic endeavors. From pioneering electric vehicles at Tesla to envisioning Mars colonization with SpaceX, Musk's revolutionary ideas continue to shape industries. Recognized as the most powerful business leader by Fortune, his ventures stand as a testament to what relentless ambition and innovation can achieve. Digital Fraud and Cybercrime: India Blocks 59,000 WhatsApp Accounts and 6.7 Lakh SIM Cards Also Read This....
Musk's influence extends beyond his corporate achievements. As a driver of artificial intelligence and space exploration, he inspires the next generation of leaders to push boundaries. His leadership exemplifies the power of daring to dream big and executing with precision.

Mukesh Ambani: The Indian Powerhouse
Mukesh Ambani, the chairman of Reliance Industries, represents the epitome of Indian business success. Ranked among the top 15 most powerful business leaders globally, Ambani has spearheaded transformative projects in telecommunications, retail, and energy, reshaping India's economic landscape. His relentless focus on innovation, particularly with Reliance Jio, has revolutionized the digital ecosystem in India.
Under his leadership, Reliance Industries has expanded its global footprint, setting new benchmarks in business growth and sustainability. Ambani’s vision reflects the critical role of emerging economies in shaping the global business narrative.

Defining Powerful Leadership
The criteria for identifying powerful business leaders are multifaceted. According to Fortune, leaders were evaluated based on six key metrics:
Business Scale: The size and impact of their ventures on a global level.
Innovation: Their ability to pioneer advancements that redefine industries.
Influence: How effectively they inspire others and create a lasting impact.
Trajectory: The journey of their career and the milestones achieved.
Business Health: Metrics like profitability, liquidity, and operational efficiency.
Global Impact: Their contribution to society and how their leadership addresses global challenges.
Elon Musk and Mukesh Ambani exemplify these qualities, demonstrating how strategic vision and innovative execution can create monumental change.

Other Global Icons in Leadership
The list of the world's most powerful business leaders features numerous iconic personalities, each excelling in their respective domains:
Satya Nadella (Microsoft): A transformative leader who has repositioned Microsoft as a cloud-computing leader, emphasizing customer-centric innovation.
Sundar Pichai (Alphabet/Google): A driving force behind Google’s expansion into artificial intelligence, cloud computing, and global digital services.
Jensen Huang (NVIDIA): The architect of the AI revolution, whose GPUs have become indispensable in AI-driven industries.
Tim Cook (Apple): Building on Steve Jobs' legacy, Cook has solidified Apple as a leader in innovation and user-centric design.
These leaders have shown that their influence isn’t confined to financial success alone; it extends to creating a better future for the world.
Leadership in Action: Driving Innovation and Progress
One common thread unites these leaders—their ability to drive innovation. For example:
Mary Barra (General Motors) is transforming the auto industry with her push toward electric vehicles, ensuring a sustainable future.
Sam Altman (OpenAI) leads advancements in artificial intelligence, shaping ethical AI practices with groundbreaking models like ChatGPT.
These visionaries have proven that impactful leadership is about staying ahead of trends, embracing challenges, and delivering solutions that inspire change.
The Indian Connection: Rising Global Influence
Apart from Mukesh Ambani, Indian-origin leaders such as Sundar Pichai and Satya Nadella have earned global recognition. Their ability to bridge cultural boundaries and lead multinational corporations demonstrates the increasing prominence of Indian talent on the world stage.
Conclusion
From technological advancements to economic transformation, these powerful business leaders are shaping the future of our world. Elon Musk and Mukesh Ambani stand at the forefront, representing the limitless potential of visionary leadership. As industries continue to evolve, their impact serves as a beacon for aspiring leaders worldwide.
This era of leadership emphasizes not only achieving success but also leveraging it to create meaningful change. In the words of Elon Musk: "When something is important enough, you do it even if the odds are not in your favor." Rajkot Job Update
#elon musk#mukesh ambani#x platform#spacex#tesla#satya nadella#sundar pichai#jensen huang#rajkot#our rajkot#Rajkot Job#Rajkot Job Vacancy#job vacancy#it jobs
8 notes
·
View notes
Text
wait can i talk my shit for a sec part of the reason that the new generation is so tech illiterate is because of those shitty ass chromebooks because they're cloud-based and you can't really open up a terminal or like a proper settings or anything and while I know not every school has the budget for bulkier thick client computers and that stuff is restricted as a security thing but a worrying amount of people don't even know about basic components (cpu, ram, gpu, etc) and I feel like we need to fund better education on these types of things and I know some schools have pilot programs (army jrotc cyber for example) but this needs to be a more widespread thing!!!! you don't even need to go that much in-depth just like "ok kids this is a terminal" even make it a game like a little flash game or something!!!! I'm tired of people seeing a command line and/or inspect element and immediately go "omg they're hacking!!!!!!1!111!!!!" and that's lowk the media's fault with the "hacker" character in the 90s and 00s "I'm into the mainframe!" and that along with the tech industry boom really made compsci and cysec seem really inaccessible which it really isn't at the basic level! but alas, the things I've suggested aren't in place right now so go out and educate yourself! I don't have any resources to do that (so much for practicing what I preach) but if anyone has any that'd be really cool. the rise of GUI and cloud-based systems isn't completely at fault but it is still a part of the problem
via mosaic twitter
3 notes
·
View notes
Text
Bitcoin Mining
The Evolution of Bitcoin Mining: From Solo Mining to Cloud-Based Solutions
Introduction
Bitcoin mining has come a long way since its early days when individuals could mine BTC using personal computers. Over the years, advancements in technology and increasing network difficulty have led to the rise of more sophisticated mining methods. Today, cloud mining solutions like NebuMine are revolutionizing cryptocurrency mining by making it more accessible and efficient. This article explores the journey of Bitcoin mining, from solo efforts to large-scale cloud mining operations.

The Early Days of Bitcoin Mining
In the beginning, Bitcoin mining was simple. Miners could use regular CPUs to solve cryptographic puzzles and validate transactions. However, as more participants joined the network, mining difficulty increased, leading to the adoption of more powerful GPUs.
As BTC mining grew, miners began forming mining pools to combine computing power and share rewards. This shift marked the transition from individual mining to more collective efforts in cryptocurrency mining.
The Rise of ASIC Mining
The introduction of Application-Specific Integrated Circuits (ASICs) in Bitcoin mining changed the game completely. These highly specialized machines offered unmatched efficiency, significantly increasing mining power while consuming less energy than GPUs.
However, ASICs also made mining more competitive, pushing small-scale miners out of the market. This led to the rise of large mining farms, further centralizing BTC mining operations.
The Shift to Cloud Mining
As the mining landscape became more challenging, cloud mining emerged as a viable alternative. Instead of investing in expensive hardware, users could rent mining power from platforms like NebuMine, enabling them to participate in Bitcoin mining without technical expertise or maintenance costs.
Cloud mining offers several advantages:
Accessibility: Users can start crypto mining without purchasing expensive equipment.
Scalability: Miners can adjust their computing power based on market conditions.
Convenience: No need for hardware setup, electricity costs, or cooling management.
With platforms like NebuMine, cloud mining has become a practical way for individuals and businesses to engage in BTC mining and Ethereum mining without the hassle of traditional setups.
Ethereum Mining and the Future of Crypto Mining
While Bitcoin mining has dominated the industry, Ethereum mining has also played a crucial role in the crypto space. With Ethereum’s shift to Proof-of-Stake (PoS), many miners have sought alternatives, further driving interest in cloud mining services.
Cryptocurrency mining continues to evolve, with new innovations such as AI-driven mining optimization and decentralized mining pools shaping the future. Platforms like NebuMine are at the forefront of this transformation, making cloud mining more accessible, efficient, and sustainable.
Conclusion
The evolution of Bitcoin mining highlights the industry's rapid advancements, from solo mining to industrial-scale operations and now cloud mining. As technology continues to advance, cloud mining solutions like NebuMine are paving the way for the future of cryptocurrency mining, making it easier for users to participate in BTC mining and Ethereum mining without technical barriers.
Check out our website to get more information about Cryptocurrency mining!
#Bitcoin mining#Cloud mining#Crypto mining#BTC mining#Ethereum mining#Cryptocurrency mining#SoundCloud
2 notes
·
View notes
Text
Efficient GPU Management for AI Startups: Exploring the Best Strategies
The rise of AI-driven innovation has made GPUs essential for startups and small businesses. However, efficiently managing GPU resources remains a challenge, particularly with limited budgets, fluctuating workloads, and the need for cutting-edge hardware for R&D and deployment.
Understanding the GPU Challenge for Startups
AI workloads—especially large-scale training and inference—require high-performance GPUs like NVIDIA A100 and H100. While these GPUs deliver exceptional computing power, they also present unique challenges:
High Costs – Premium GPUs are expensive, whether rented via the cloud or purchased outright.
Availability Issues – In-demand GPUs may be limited on cloud platforms, delaying time-sensitive projects.
Dynamic Needs – Startups often experience fluctuating GPU demands, from intensive R&D phases to stable inference workloads.
To optimize costs, performance, and flexibility, startups must carefully evaluate their options. This article explores key GPU management strategies, including cloud services, physical ownership, rentals, and hybrid infrastructures—highlighting their pros, cons, and best use cases.
1. Cloud GPU Services
Cloud GPU services from AWS, Google Cloud, and Azure offer on-demand access to GPUs with flexible pricing models such as pay-as-you-go and reserved instances.
✅ Pros:
✔ Scalability – Easily scale resources up or down based on demand. ✔ No Upfront Costs – Avoid capital expenditures and pay only for usage. ✔ Access to Advanced GPUs – Frequent updates include the latest models like NVIDIA A100 and H100. ✔ Managed Infrastructure – No need for maintenance, cooling, or power management. ✔ Global Reach – Deploy workloads in multiple regions with ease.
❌ Cons:
✖ High Long-Term Costs – Usage-based billing can become expensive for continuous workloads. ✖ Availability Constraints – Popular GPUs may be out of stock during peak demand. ✖ Data Transfer Costs – Moving large datasets in and out of the cloud can be costly. ✖ Vendor Lock-in – Dependency on a single provider limits flexibility.
🔹 Best Use Cases:
Early-stage startups with fluctuating GPU needs.
Short-term R&D projects and proof-of-concept testing.
Workloads requiring rapid scaling or multi-region deployment.
2. Owning Physical GPU Servers
Owning physical GPU servers means purchasing GPUs and supporting hardware, either on-premises or collocated in a data center.
✅ Pros:
✔ Lower Long-Term Costs – Once purchased, ongoing costs are limited to power, maintenance, and hosting fees. ✔ Full Control – Customize hardware configurations and ensure access to specific GPUs. ✔ Resale Value – GPUs retain significant resale value (Sell GPUs), allowing you to recover investment costs when upgrading. ✔ Purchasing Flexibility – Buy GPUs at competitive prices, including through refurbished hardware vendors. ✔ Predictable Expenses – Fixed hardware costs eliminate unpredictable cloud billing. ✔ Guaranteed Availability – Avoid cloud shortages and ensure access to required GPUs.
❌ Cons:
✖ High Upfront Costs – Buying high-performance GPUs like NVIDIA A100 or H100 requires a significant investment. ✖ Complex Maintenance – Managing hardware failures and upgrades requires technical expertise. ✖ Limited Scalability – Expanding capacity requires additional hardware purchases.
🔹 Best Use Cases:
Startups with stable, predictable workloads that need dedicated resources.
Companies conducting large-scale AI training or handling sensitive data.
Organizations seeking long-term cost savings and reduced dependency on cloud providers.
3. Renting Physical GPU Servers
Renting physical GPU servers provides access to high-performance hardware without the need for direct ownership. These servers are often hosted in data centers and offered by third-party providers.
✅ Pros:
✔ Lower Upfront Costs – Avoid large capital investments and opt for periodic rental fees. ✔ Bare-Metal Performance – Gain full access to physical GPUs without virtualization overhead. ✔ Flexibility – Upgrade or switch GPU models more easily compared to ownership. ✔ No Depreciation Risks – Avoid concerns over GPU obsolescence.
❌ Cons:
✖ Rental Premiums – Long-term rental fees can exceed the cost of purchasing hardware. ✖ Operational Complexity – Requires coordination with data center providers for management. ✖ Availability Constraints – Supply shortages may affect access to cutting-edge GPUs.
🔹 Best Use Cases:
Mid-stage startups needing temporary GPU access for specific projects.
Companies transitioning away from cloud dependency but not ready for full ownership.
Organizations with fluctuating GPU workloads looking for cost-effective solutions.
4. Hybrid Infrastructure
Hybrid infrastructure combines owned or rented GPUs with cloud GPU services, ensuring cost efficiency, scalability, and reliable performance.
What is a Hybrid GPU Infrastructure?
A hybrid model integrates: 1️⃣ Owned or Rented GPUs – Dedicated resources for R&D and long-term workloads. 2️⃣ Cloud GPU Services – Scalable, on-demand resources for overflow, production, and deployment.
How Hybrid Infrastructure Benefits Startups
✅ Ensures Control in R&D – Dedicated hardware guarantees access to required GPUs. ✅ Leverages Cloud for Production – Use cloud resources for global scaling and short-term spikes. ✅ Optimizes Costs – Aligns workloads with the most cost-effective resource. ✅ Reduces Risk – Minimizes reliance on a single provider, preventing vendor lock-in.
Expanded Hybrid Workflow for AI Startups
1️⃣ R&D Stage: Use physical GPUs for experimentation and colocate them in data centers. 2️⃣ Model Stabilization: Transition workloads to the cloud for flexible testing. 3️⃣ Deployment & Production: Reserve cloud instances for stable inference and global scaling. 4️⃣ Overflow Management: Use a hybrid approach to scale workloads efficiently.
Conclusion
Efficient GPU resource management is crucial for AI startups balancing innovation with cost efficiency.
Cloud GPUs offer flexibility but become expensive for long-term use.
Owning GPUs provides control and cost savings but requires infrastructure management.
Renting GPUs is a middle-ground solution, offering flexibility without ownership risks.
Hybrid infrastructure combines the best of both, enabling startups to scale cost-effectively.
Platforms like BuySellRam.com help startups optimize their hardware investments by providing cost-effective solutions for buying and selling GPUs, ensuring they stay competitive in the evolving AI landscape.
The original article is here: How to manage GPU resource?
#GPU Management#High Performance Computing#cloud computing#ai hardware#technology#Nvidia#AI Startups#AMD#it management#data center#ai technology#computer
2 notes
·
View notes
Text
AI & Data Centers vs Water + Energy
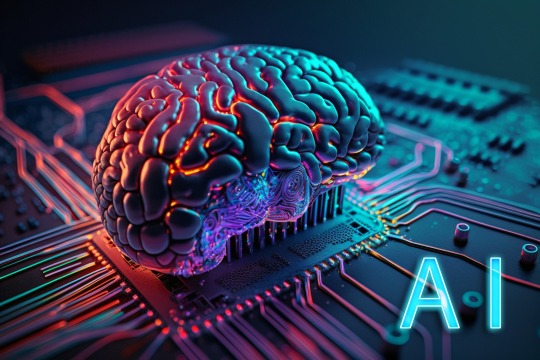
We all know that AI has issues, including energy and water consumption. But these fields are still young and lots of research is looking into making them more efficient. Remember, most technologies tend to suck when they first come out.
Deploying high-performance, energy-efficient AI
"You give up that kind of amazing general purpose use like when you're using ChatGPT-4 and you can ask it everything from 17th century Italian poetry to quantum mechanics, if you narrow your range, these smaller models can give you equivalent or better kind of capability, but at a tiny fraction of the energy consumption," says Ball."...
"I think liquid cooling is probably one of the most important low hanging fruit opportunities... So if you move a data center to a fully liquid cooled solution, this is an opportunity of around 30% of energy consumption, which is sort of a wow number.... There's more upfront costs, but actually it saves money in the long run... One of the other benefits of liquid cooling is we get out of the business of evaporating water for cooling...
The other opportunity you mentioned was density and bringing higher and higher density of computing has been the trend for decades. That is effectively what Moore's Law has been pushing us forward... [i.e. chips rate of improvement is faster than their energy need growths. This means each year chips are capable of doing more calculations with less energy. - RCS] ... So the energy savings there is substantial, not just because those chips are very, very efficient, but because the amount of networking equipment and ancillary things around those systems is a lot less because you're using those resources more efficiently with those very high dense components"
New tools are available to help reduce the energy that AI models devour
"The trade-off for capping power is increasing task time — GPUs will take about 3 percent longer to complete a task, an increase Gadepally says is "barely noticeable" considering that models are often trained over days or even months... Side benefits have arisen, too. Since putting power constraints in place, the GPUs on LLSC supercomputers have been running about 30 degrees Fahrenheit cooler and at a more consistent temperature, reducing stress on the cooling system. Running the hardware cooler can potentially also increase reliability and service lifetime. They can now consider delaying the purchase of new hardware — reducing the center's "embodied carbon," or the emissions created through the manufacturing of equipment — until the efficiencies gained by using new hardware offset this aspect of the carbon footprint. They're also finding ways to cut down on cooling needs by strategically scheduling jobs to run at night and during the winter months."
AI just got 100-fold more energy efficient
Northwestern University engineers have developed a new nanoelectronic device that can perform accurate machine-learning classification tasks in the most energy-efficient manner yet. Using 100-fold less energy than current technologies...
“Today, most sensors collect data and then send it to the cloud, where the analysis occurs on energy-hungry servers before the results are finally sent back to the user,” said Northwestern’s Mark C. Hersam, the study’s senior author. “This approach is incredibly expensive, consumes significant energy and adds a time delay...
For current silicon-based technologies to categorize data from large sets like ECGs, it takes more than 100 transistors — each requiring its own energy to run. But Northwestern’s nanoelectronic device can perform the same machine-learning classification with just two devices. By reducing the number of devices, the researchers drastically reduced power consumption and developed a much smaller device that can be integrated into a standard wearable gadget."
Researchers develop state-of-the-art device to make artificial intelligence more energy efficient
""This work is the first experimental demonstration of CRAM, where the data can be processed entirely within the memory array without the need to leave the grid where a computer stores information,"...
According to the new paper's authors, a CRAM-based machine learning inference accelerator is estimated to achieve an improvement on the order of 1,000. Another example showed an energy savings of 2,500 and 1,700 times compared to traditional methods"
5 notes
·
View notes
Text
Update: Patience Fans Winning Again (Import Poll Decisions Too!)
So I've got a version working with all the features for a solid beta, but... only on my computer.
It should work when I set it up with all the cloud nonsense it needs, but it costs money, I don't wanna spend money right now, and I can't really make you pay for a product I'm not sure will work right.
So here's the deal: I'm starting a paid internship soon, so once I have that income I'll be willing to spend money on this. Problem: it's a National Parks internship so I won't have internet or the computer that I make LyingBard with until I get back... in 3-6 months.
But! I also want to ask you an important question...
I've been making LyingBard as a Cloud-Based app. It allows you to use it from anywhere through my website, but it also means I incur the cost of finding GPUs to run this with while you have a perfectly good GPU sitting in your computer doing nothing (also cloud apps are a pain in the ass).
I could also make it a Desktop App, but it would be about 1GB (blame PyTorch), it would only run on NVIDIA GPUs, and you'd have to set up your own discord bots and stuff (although I can try and streamline that for you).
Another big problem is money. Cloud-Based stuff costs money to run and becomes basically a job even if it doesn't pay enough. I'm considering a career in the National Parks Service so I won't be available most of the time if something goes wrong and stuff can go VERY wrong with a paid cloud app (think leaked passwords, compromised payment processor (Stripe) account, that sort of thing).
A Desktop App, however, can be left alone without spontaneously deleting itself or attacking you, but I would certainly have to focus on a career unless you donated a whole heck of a lot.
So DECIDE TIME! After carefully (or carelessly, idc) considering these options. WHICH DO YOU CHOOSE?!
Unfortunately, LyingBard isn't releasing until I get back regardless. Even the desktop app is just a bit too much work for me to get done before I leave.
#fubai#lyingbard#ai tts#ai#lyrebird ai#rtvs#please vote in the poll this is important ok?#also reply with your thoughts I want to hear it even if you don't think you fully understand#also ask questions too
6 notes
·
View notes
Text
How can you optimize the performance of machine learning models in the cloud?
Optimizing machine learning models in the cloud involves several strategies to enhance performance and efficiency. Here’s a detailed approach:
Choose the Right Cloud Services:
Managed ML Services:
Use managed services like AWS SageMaker, Google AI Platform, or Azure Machine Learning, which offer built-in tools for training, tuning, and deploying models.
Auto-scaling:
Enable auto-scaling features to adjust resources based on demand, which helps manage costs and performance.
Optimize Data Handling:
Data Storage:
Use scalable cloud storage solutions like Amazon S3, Google Cloud Storage, or Azure Blob Storage for storing large datasets efficiently.
Data Pipeline:
Implement efficient data pipelines with tools like Apache Kafka or AWS Glue to manage and process large volumes of data.
Select Appropriate Computational Resources:
Instance Types:
Choose the right instance types based on your model’s requirements. For example, use GPU or TPU instances for deep learning tasks to accelerate training.
Spot Instances:
Utilize spot instances or preemptible VMs to reduce costs for non-time-sensitive tasks.
Optimize Model Training:
Hyperparameter Tuning:
Use cloud-based hyperparameter tuning services to automate the search for optimal model parameters. Services like Google Cloud AI Platform’s HyperTune or AWS SageMaker’s Automatic Model Tuning can help.
Distributed Training:
Distribute model training across multiple instances or nodes to speed up the process. Frameworks like TensorFlow and PyTorch support distributed training and can take advantage of cloud resources.
Monitoring and Logging:
Monitoring Tools:
Implement monitoring tools to track performance metrics and resource usage. AWS CloudWatch, Google Cloud Monitoring, and Azure Monitor offer real-time insights.
Logging:
Maintain detailed logs for debugging and performance analysis, using tools like AWS CloudTrail or Google Cloud Logging.
Model Deployment:
Serverless Deployment:
Use serverless options to simplify scaling and reduce infrastructure management. Services like AWS Lambda or Google Cloud Functions can handle inference tasks without managing servers.
Model Optimization:
Optimize models by compressing them or using model distillation techniques to reduce inference time and improve latency.
Cost Management:
Cost Analysis:
Regularly analyze and optimize cloud costs to avoid overspending. Tools like AWS Cost Explorer, Google Cloud’s Cost Management, and Azure Cost Management can help monitor and manage expenses.
By carefully selecting cloud services, optimizing data handling and training processes, and monitoring performance, you can efficiently manage and improve machine learning models in the cloud.
2 notes
·
View notes
Text
A3 Ultra VMs With NVIDIA H200 GPUs Pre-launch This Month
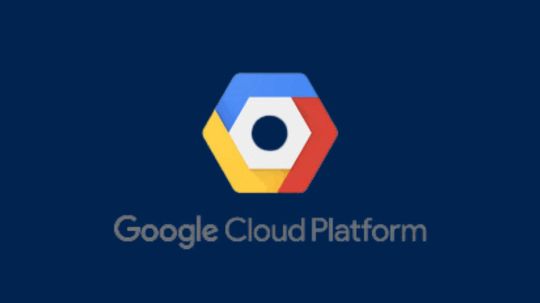
Strong infrastructure advancements for your future that prioritizes AI
To increase customer performance, usability, and cost-effectiveness, Google Cloud implemented improvements throughout the AI Hypercomputer stack this year. Google Cloud at the App Dev & Infrastructure Summit:
Trillium, Google’s sixth-generation TPU, is currently available for preview.
Next month, A3 Ultra VMs with NVIDIA H200 Tensor Core GPUs will be available for preview.
Google’s new, highly scalable clustering system, Hypercompute Cluster, will be accessible beginning with A3 Ultra VMs.
Based on Axion, Google’s proprietary Arm processors, C4A virtual machines (VMs) are now widely accessible
AI workload-focused additions to Titanium, Google Cloud’s host offload capability, and Jupiter, its data center network.
Google Cloud’s AI/ML-focused block storage service, Hyperdisk ML, is widely accessible.
Trillium A new era of TPU performance
Trillium A new era of TPU performance is being ushered in by TPUs, which power Google’s most sophisticated models like Gemini, well-known Google services like Maps, Photos, and Search, as well as scientific innovations like AlphaFold 2, which was just awarded a Nobel Prize! We are happy to inform that Google Cloud users can now preview Trillium, our sixth-generation TPU.
Taking advantage of NVIDIA Accelerated Computing to broaden perspectives
By fusing the best of Google Cloud’s data center, infrastructure, and software skills with the NVIDIA AI platform which is exemplified by A3 and A3 Mega VMs powered by NVIDIA H100 Tensor Core GPUs it also keeps investing in its partnership and capabilities with NVIDIA.
Google Cloud announced that the new A3 Ultra VMs featuring NVIDIA H200 Tensor Core GPUs will be available on Google Cloud starting next month.
Compared to earlier versions, A3 Ultra VMs offer a notable performance improvement. Their foundation is NVIDIA ConnectX-7 network interface cards (NICs) and servers equipped with new Titanium ML network adapter, which is tailored to provide a safe, high-performance cloud experience for AI workloads. A3 Ultra VMs provide non-blocking 3.2 Tbps of GPU-to-GPU traffic using RDMA over Converged Ethernet (RoCE) when paired with our datacenter-wide 4-way rail-aligned network.
In contrast to A3 Mega, A3 Ultra provides:
With the support of Google’s Jupiter data center network and Google Cloud’s Titanium ML network adapter, double the GPU-to-GPU networking bandwidth
With almost twice the memory capacity and 1.4 times the memory bandwidth, LLM inferencing performance can increase by up to 2 times.
Capacity to expand to tens of thousands of GPUs in a dense cluster with performance optimization for heavy workloads in HPC and AI.
Google Kubernetes Engine (GKE), which offers an open, portable, extensible, and highly scalable platform for large-scale training and AI workloads, will also offer A3 Ultra VMs.
Hypercompute Cluster: Simplify and expand clusters of AI accelerators
It’s not just about individual accelerators or virtual machines, though; when dealing with AI and HPC workloads, you have to deploy, maintain, and optimize a huge number of AI accelerators along with the networking and storage that go along with them. This may be difficult and time-consuming. For this reason, Google Cloud is introducing Hypercompute Cluster, which simplifies the provisioning of workloads and infrastructure as well as the continuous operations of AI supercomputers with tens of thousands of accelerators.
Fundamentally, Hypercompute Cluster integrates the most advanced AI infrastructure technologies from Google Cloud, enabling you to install and operate several accelerators as a single, seamless unit. You can run your most demanding AI and HPC workloads with confidence thanks to Hypercompute Cluster’s exceptional performance and resilience, which includes features like targeted workload placement, dense resource co-location with ultra-low latency networking, and sophisticated maintenance controls to reduce workload disruptions.
For dependable and repeatable deployments, you can use pre-configured and validated templates to build up a Hypercompute Cluster with just one API call. This include containerized software with orchestration (e.g., GKE, Slurm), framework and reference implementations (e.g., JAX, PyTorch, MaxText), and well-known open models like Gemma2 and Llama3. As part of the AI Hypercomputer architecture, each pre-configured template is available and has been verified for effectiveness and performance, allowing you to concentrate on business innovation.
A3 Ultra VMs will be the first Hypercompute Cluster to be made available next month.
An early look at the NVIDIA GB200 NVL72
Google Cloud is also awaiting the developments made possible by NVIDIA GB200 NVL72 GPUs, and we’ll be providing more information about this fascinating improvement soon. Here is a preview of the racks Google constructing in the meantime to deliver the NVIDIA Blackwell platform’s performance advantages to Google Cloud’s cutting-edge, environmentally friendly data centers in the early months of next year.
Redefining CPU efficiency and performance with Google Axion Processors
CPUs are a cost-effective solution for a variety of general-purpose workloads, and they are frequently utilized in combination with AI workloads to produce complicated applications, even if TPUs and GPUs are superior at specialized jobs. Google Axion Processors, its first specially made Arm-based CPUs for the data center, at Google Cloud Next ’24. Customers using Google Cloud may now benefit from C4A virtual machines, the first Axion-based VM series, which offer up to 10% better price-performance compared to the newest Arm-based instances offered by other top cloud providers.
Additionally, compared to comparable current-generation x86-based instances, C4A offers up to 60% more energy efficiency and up to 65% better price performance for general-purpose workloads such as media processing, AI inferencing applications, web and app servers, containerized microservices, open-source databases, in-memory caches, and data analytics engines.
Titanium and Jupiter Network: Making AI possible at the speed of light
Titanium, the offload technology system that supports Google’s infrastructure, has been improved to accommodate workloads related to artificial intelligence. Titanium provides greater compute and memory resources for your applications by lowering the host’s processing overhead through a combination of on-host and off-host offloads. Furthermore, although Titanium’s fundamental features can be applied to AI infrastructure, the accelerator-to-accelerator performance needs of AI workloads are distinct.
Google has released a new Titanium ML network adapter to address these demands, which incorporates and expands upon NVIDIA ConnectX-7 NICs to provide further support for virtualization, traffic encryption, and VPCs. The system offers best-in-class security and infrastructure management along with non-blocking 3.2 Tbps of GPU-to-GPU traffic across RoCE when combined with its data center’s 4-way rail-aligned network.
Google’s Jupiter optical circuit switching network fabric and its updated data center network significantly expand Titanium’s capabilities. With native 400 Gb/s link rates and a total bisection bandwidth of 13.1 Pb/s (a practical bandwidth metric that reflects how one half of the network can connect to the other), Jupiter could handle a video conversation for every person on Earth at the same time. In order to meet the increasing demands of AI computation, this enormous scale is essential.
Hyperdisk ML is widely accessible
For computing resources to continue to be effectively utilized, system-level performance maximized, and economical, high-performance storage is essential. Google launched its AI-powered block storage solution, Hyperdisk ML, in April 2024. Now widely accessible, it adds dedicated storage for AI and HPC workloads to the networking and computing advancements.
Hyperdisk ML efficiently speeds up data load times. It drives up to 11.9x faster model load time for inference workloads and up to 4.3x quicker training time for training workloads.
With 1.2 TB/s of aggregate throughput per volume, you may attach 2500 instances to the same volume. This is more than 100 times more than what big block storage competitors are giving.
Reduced accelerator idle time and increased cost efficiency are the results of shorter data load times.
Multi-zone volumes are now automatically created for your data by GKE. In addition to quicker model loading with Hyperdisk ML, this enables you to run across zones for more computing flexibility (such as lowering Spot preemption).
Developing AI’s future
Google Cloud enables companies and researchers to push the limits of AI innovation with these developments in AI infrastructure. It anticipates that this strong foundation will give rise to revolutionary new AI applications.
Read more on Govindhtech.com
#A3UltraVMs#NVIDIAH200#AI#Trillium#HypercomputeCluster#GoogleAxionProcessors#Titanium#News#Technews#Technology#Technologynews#Technologytrends#Govindhtech
2 notes
·
View notes
Text
Okay but like, I literally just bought a refurbed business computer that's been upgraded to today's standards (it originally came out in 2012/2013).
Works soooooooo much better than any new laptop I've ever had, and still has room for further upgrades. Like, I only bought the lower-end refurb that the seller had available. DVD rw drive. Integrated graphics. Intel i5 core processor (I forget the specifics on that) and 8gb ram with 500gb HDD. But I can further upgrade it by converting to SSD using an adapter and have a few tb of internal storage and it handles up to 32 GB ram.
And that's before I get around to things like sound cards and GPUs. That's just the refurb stock model I've got.
The laptop I have from 2017 can handle only up to 16gb ram. And has 500gb SSD but the motherboard can't really handle more than that. Basic DVD drive, but it is cd rw just not DVD rw. My husband's 2020 laptop? 8gb ram but it's soldered in and can't be upgraded with 500gb SSD that also can't be upgraded. No DVD/CD drive.
Took a look at Walmart a few days ago, most affordable laptops are basically chromebooks with windows 11 on em. 250gb internal storage but with a Microsoft subscription you can have a TB of cloud storage! Also, 4gb ram, with the midrange models having gasp! 8gb ram and still only 250ssd internal storage! By the way the "affordable" price range at my local Walmart starts at $350ish. Not a single model available from any brand has a DVD or CD drive. For that you've got to drop nearly $1000 for the desktop tower alone. And that's for base models with 8-16gb and 500gb-1tb internal storage. Monitor, keyboard, mouse, gpu not included. But hey you get rgb decorative lighting I guess.
And this is just at my local Walmart in a rural-ish town where the only other place to buy a computer is Staples. And even then, they don't have nearly as much as Walmart.
The only reason I bought my refurb desktop from a discount seller was because my laptops are too old and costly to repair at the moment and I needed SOMETHING to be able to start streaming again and playing Minecraft with my husband. And I only paid $98 for it with tax. Free shipping. It came with a free keyboard and mouse and surprise internal speakers that didn't originally come on that particular model. It was cheap, better than any of the laptops I have, still fully upgradeable, and while it doesn't have fancy HDMI connector due simply to the fact the motherboard just doesn't come with one, I can remedy that with an adapter for under $20 if I choose.
So yeah, with the damn near unusability of newer model laptops in the market and the near unaffordability of even the bottom end models yeah, I can completely see a return to desktops. Especially with the ease of availability of upgraded refurbs on the market nowadays as many businesses are forced to upgrade to less capable machines with newer software that requires always online cloud computing in order to still function as a business. So their older perfectly serviceable machines are being sold off and or salvaged and resold to regular folks.
i give it scant years before desktop computers come back fashion a la record players and people talk about bringing back computer rooms, not taking your computer around the house, "intentional" computing, "it's really important to take the time out and just sit with your computer and really absorb the action of computing" it will be no different from the romance and nostalgia surrounding the notion of throwing on a vinyl
502 notes
·
View notes
Text
Notebook Market Emerging Trends Reflecting Technological Evolution and Consumer Demand
The notebook market is witnessing rapid change fueled by the convergence of new technologies, changing work environments, and increasing demand for portable computing devices. As remote work, e-learning, and digital collaboration become mainstream, manufacturers are developing innovative notebook models with advanced features, improved performance, and enhanced user experience. The following emerging trends are reshaping the notebook industry and setting the stage for future growth.

1. Rise of Ultra-Thin and Lightweight Notebooks
Consumers increasingly prefer sleek, lightweight devices that combine portability with high performance. Manufacturers are focusing on reducing the form factor without compromising on processing power. The demand for ultrabooks and slim notebooks has surged, driven by professionals, students, and travelers who require high mobility. These devices often feature premium materials such as aluminum chassis and edge-to-edge displays, enhancing both aesthetics and durability.
2. Integration of AI and Machine Learning Features
The integration of AI and machine learning into notebooks is becoming a key differentiator. AI-enhanced notebooks provide capabilities such as intelligent battery management, adaptive performance optimization, and voice assistant integration. Features like real-time language translation, facial recognition, and automated security protocols are becoming standard in premium notebooks, improving efficiency and personalization for users.
3. Growing Popularity of Gaming Notebooks
Gaming notebooks are no longer niche products catering only to hardcore gamers. The rising popularity of e-sports, immersive gaming experiences, and high-performance mobile computing has led to a surge in demand for gaming laptops. These notebooks are equipped with advanced GPUs, high-refresh-rate displays, customizable keyboards, and efficient cooling systems. Manufacturers are increasingly offering thinner and more portable gaming laptops without sacrificing performance, making them suitable for work and play.
4. 5G-Enabled and Always-Connected Notebooks
Connectivity is a major focus area in the notebook market. With the rollout of 5G networks, manufacturers are launching notebooks with integrated 5G modems, enabling ultra-fast internet access and seamless cloud-based workflows. These always-connected notebooks cater to remote workers, digital nomads, and professionals who require constant connectivity, regardless of location. This trend aligns with the growing demand for reliable mobile computing solutions.
5. Sustainability and Eco-Friendly Notebook Design
Environmental consciousness is influencing purchasing decisions, prompting notebook manufacturers to adopt sustainable practices. Companies are introducing notebooks made with recycled materials, energy-efficient components, and modular designs to reduce e-waste. Additionally, brands are focusing on extending product lifecycles through easy repairability and upgrade options. Eco-friendly packaging and carbon-neutral production processes are becoming integral to notebook manufacturing strategies.
6. Hybrid Work Culture Driving Demand for Versatile Notebooks
The shift to hybrid work models is reshaping consumer preferences. Employees seek versatile notebooks that support productivity both at home and in the office. As a result, 2-in-1 convertibles, detachable keyboards, and touchscreen notebooks are gaining popularity. These devices offer flexibility, allowing users to switch between laptop and tablet modes depending on their workflow needs. Collaboration features such as high-quality webcams, noise-canceling microphones, and enhanced connectivity options are now essential in modern notebooks.
7. Enhanced Security Features for Data Protection
With the increasing reliance on mobile devices for work and personal use, data security has become paramount. Notebook manufacturers are embedding advanced security features, including biometric authentication, hardware-based encryption, and privacy screens. The emphasis on safeguarding sensitive data is particularly strong in enterprise-grade notebooks, as organizations prioritize cybersecurity to mitigate risks associated with remote work.
8. Demand for High-Performance Notebooks in Education Sector
The education sector continues to drive significant demand for affordable, durable, and high-performance notebooks. With e-learning becoming a permanent part of education systems, students and educators require devices that support video conferencing, online collaboration, and digital content creation. Chromebooks and entry-level notebooks designed for educational purposes are increasingly in demand, especially in emerging markets.
Conclusion
The notebook market is evolving rapidly, driven by advancements in technology, changing consumer lifestyles, and the global shift towards digital ecosystems. Trends such as AI integration, 5G connectivity, ultra-portable designs, and sustainable production practices are shaping the future of notebooks. Manufacturers that adapt to these trends and prioritize innovation, user experience, and environmental responsibility are poised to thrive in this dynamic market.
As competition intensifies and consumer expectations evolve, stakeholders across the notebook industry must stay agile and leverage these emerging trends to meet growing demand and unlock new opportunities.
0 notes
Text
Transform Your Career with a Cutting-Edge Artificial Intelligence Course in Gurgaon
The rise of Artificial Intelligence (AI) has completely transformed the global job market, opening doors to exciting and futuristic career paths. From smart assistants like Siri and Alexa to advanced medical diagnostics, AI is powering innovations that were once only possible in science fiction. If you’re looking to future-proof your career, enrolling in an Artificial Intelligence course is the perfect first step.
For individuals living in Delhi NCR, there’s no better place to learn than Gurgaon. Known as India’s corporate and tech capital, the city offers some of the most dynamic and hands-on Artificial Intelligence course in Gurgaon, tailored for both freshers and experienced professionals.
What Makes an Artificial Intelligence Course Valuable?
A comprehensive Artificial Intelligence course provides deep insights into various technologies and concepts such as:
Machine Learning Algorithms
Neural Networks
Natural Language Processing (NLP)
Computer Vision
Reinforcement Learning
AI for Data Analytics
Python and TensorFlow Programming
Such a course not only builds theoretical knowledge but also provides practical exposure through projects, assignments, and industry case studies.
Why Opt for an Artificial Intelligence Course in Gurgaon?
Gurgaon is more than just a city—it’s a tech powerhouse. Here’s why pursuing an Artificial Intelligence course in Gurgaon gives you an advantage:
1. Location Advantage
With offices of Google, Microsoft, Accenture, and many AI startups located in Gurgaon, students get better networking and employment opportunities.
2. Industry Collaboration
Many training institutes in Gurgaon have partnerships with tech companies, offering industry-led workshops, hackathons, and internships.
3. High-Quality Institutes
Several top training institutes and edtech platforms offer AI courses in Gurgaon, complete with updated syllabi, live mentoring, and certification from recognized bodies.
4. Placement Support
One of the biggest benefits is post-course support. Most AI courses in Gurgaon include resume development, mock interviews, and placement drives.
5. Advanced Infrastructure
Training centers are equipped with labs, AI tools, GPUs, and cloud-based platforms—giving learners real-world experience.
Who Can Join an AI Course?
An Artificial Intelligence course is suitable for:
Engineering & Computer Science Students
Software Developers & IT Professionals
Data Analysts & Business Intelligence Experts
Marketing Professionals using AI for customer insights
Entrepreneurs who want to automate and innovate using AI
Anyone interested in future technologies
Whether you're switching careers or just getting started, AI has room for all.
Benefits of Learning Artificial Intelligence
Here are some reasons why an Artificial Intelligence course can change your career:
High Salary Potential: AI professionals are among the highest-paid in the tech industry.
Growing Demand: According to reports, the AI market in India is expected to reach $7.8 billion by 2025.
Global Opportunities: AI jobs are in demand in countries like the USA, Canada, Germany, and the UK.
Innovation: Be part of cutting-edge solutions in healthcare, fintech, autonomous vehicles, and smart cities.
Where to Enroll?
Several reputed institutes in Gurgaon offer AI training with certification. Look for features like:
Project-based learning
1:1 mentor support
Interview preparation
Internship and placement tie-ups
Globally recognized certifications (Google, IBM, Microsoft, etc.)
Final Thoughts
Artificial Intelligence is not just the future—it is the present. The earlier you adopt this technology and build expertise, the greater your advantage in the competitive job market. If you’re located in or near Delhi NCR, enrolling in an Artificial Intelligence course in Gurgaon gives you the perfect combination of location, training, and opportunity.
Get ready to lead the future. Join a top-rated Artificial Intelligence course today and turn your passion for tech into a successful career.
0 notes
Text
Data Center Accelerator Market Set to Transform AI Infrastructure Landscape by 2031
The global data center accelerator market is poised for exponential growth, projected to rise from USD 14.4 Bn in 2022 to a staggering USD 89.8 Bn by 2031, advancing at a CAGR of 22.5% during the forecast period from 2023 to 2031. Rapid adoption of Artificial Intelligence (AI), Machine Learning (ML), and High-Performance Computing (HPC) is the primary catalyst driving this expansion.
Market Overview: Data center accelerators are specialized hardware components that improve computing performance by efficiently handling intensive workloads. These include Graphics Processing Units (GPUs), Tensor Processing Units (TPUs), Field Programmable Gate Arrays (FPGAs), and Application-Specific Integrated Circuits (ASICs), which complement CPUs by expediting data processing.
Accelerators enable data centers to process massive datasets more efficiently, reduce reliance on servers, and optimize costs a significant advantage in a data-driven world.
Market Drivers & Trends
Rising Demand for High-performance Computing (HPC): The proliferation of data-intensive applications across industries such as healthcare, autonomous driving, financial modeling, and weather forecasting is fueling demand for robust computing resources.
Boom in AI and ML Technologies: The computational requirements of AI and ML are driving the need for accelerators that can handle parallel operations and manage extensive datasets efficiently.
Cloud Computing Expansion: Major players like AWS, Azure, and Google Cloud are investing in infrastructure that leverages accelerators to deliver faster AI-as-a-service platforms.
Latest Market Trends
GPU Dominance: GPUs continue to dominate the market, especially in AI training and inference workloads, due to their capability to handle parallel computations.
Custom Chip Development: Tech giants are increasingly developing custom chips (e.g., Meta’s MTIA and Google's TPUs) tailored to their specific AI processing needs.
Energy Efficiency Focus: Companies are prioritizing the design of accelerators that deliver high computational power with reduced energy consumption, aligning with green data center initiatives.
Key Players and Industry Leaders
Prominent companies shaping the data center accelerator landscape include:
NVIDIA Corporation – A global leader in GPUs powering AI, gaming, and cloud computing.
Intel Corporation – Investing heavily in FPGA and ASIC-based accelerators.
Advanced Micro Devices (AMD) – Recently expanded its EPYC CPU lineup for data centers.
Meta Inc. – Introduced Meta Training and Inference Accelerator (MTIA) chips for internal AI applications.
Google (Alphabet Inc.) – Continues deploying TPUs across its cloud platforms.
Other notable players include Huawei Technologies, Cisco Systems, Dell Inc., Fujitsu, Enflame Technology, Graphcore, and SambaNova Systems.
Recent Developments
March 2023 – NVIDIA introduced a comprehensive Data Center Platform strategy at GTC 2023 to address diverse computational requirements.
June 2023 – AMD launched new EPYC CPUs designed to complement GPU-powered accelerator frameworks.
2023 – Meta Inc. revealed the MTIA chip to improve performance for internal AI workloads.
2023 – Intel announced a four-year roadmap for data center innovation focused on Infrastructure Processing Units (IPUs).
Gain an understanding of key findings from our Report in this sample - https://www.transparencymarketresearch.com/sample/sample.php?flag=S&rep_id=82760
Market Opportunities
Edge Data Center Integration: As computing shifts closer to the edge, opportunities arise for compact and energy-efficient accelerators in edge data centers for real-time analytics and decision-making.
AI in Healthcare and Automotive: As AI adoption grows in precision medicine and autonomous vehicles, demand for accelerators tuned for domain-specific processing will soar.
Emerging Markets: Rising digitization in emerging economies presents substantial opportunities for data center expansion and accelerator deployment.
Future Outlook
With AI, ML, and analytics forming the foundation of next-generation applications, the demand for enhanced computational capabilities will continue to climb. By 2031, the data center accelerator market will likely transform into a foundational element of global IT infrastructure.
Analysts anticipate increasing collaboration between hardware manufacturers and AI software developers to optimize performance across the board. As digital transformation accelerates, companies investing in custom accelerator architectures will gain significant competitive advantages.
Market Segmentation
By Type:
Central Processing Unit (CPU)
Graphics Processing Unit (GPU)
Application-Specific Integrated Circuit (ASIC)
Field-Programmable Gate Array (FPGA)
Others
By Application:
Advanced Data Analytics
AI/ML Training and Inference
Computing
Security and Encryption
Network Functions
Others
Regional Insights
Asia Pacific dominates the global market due to explosive digital content consumption and rapid infrastructure development in countries such as China, India, Japan, and South Korea.
North America holds a significant share due to the presence of major cloud providers, AI startups, and heavy investment in advanced infrastructure. The U.S. remains a critical hub for data center deployment and innovation.
Europe is steadily adopting AI and cloud computing technologies, contributing to increased demand for accelerators in enterprise data centers.
Why Buy This Report?
Comprehensive insights into market drivers, restraints, trends, and opportunities
In-depth analysis of the competitive landscape
Region-wise segmentation with revenue forecasts
Includes strategic developments and key product innovations
Covers historical data from 2017 and forecast till 2031
Delivered in convenient PDF and Excel formats
Frequently Asked Questions (FAQs)
1. What was the size of the global data center accelerator market in 2022? The market was valued at US$ 14.4 Bn in 2022.
2. What is the projected market value by 2031? It is projected to reach US$ 89.8 Bn by the end of 2031.
3. What is the key factor driving market growth? The surge in demand for AI/ML processing and high-performance computing is the major driver.
4. Which region holds the largest market share? Asia Pacific is expected to dominate the global data center accelerator market from 2023 to 2031.
5. Who are the leading companies in the market? Top players include NVIDIA, Intel, AMD, Meta, Google, Huawei, Dell, and Cisco.
6. What type of accelerator dominates the market? GPUs currently dominate the market due to their parallel processing efficiency and widespread adoption in AI/ML applications.
7. What applications are fueling growth? Applications like AI/ML training, advanced analytics, and network security are major contributors to the market's growth.
Explore Latest Research Reports by Transparency Market Research: Tactile Switches Market: https://www.transparencymarketresearch.com/tactile-switches-market.html
GaN Epitaxial Wafers Market: https://www.transparencymarketresearch.com/gan-epitaxial-wafers-market.html
Silicon Carbide MOSFETs Market: https://www.transparencymarketresearch.com/silicon-carbide-mosfets-market.html
Chip Metal Oxide Varistor (MOV) Market: https://www.transparencymarketresearch.com/chip-metal-oxide-varistor-mov-market.html
About Transparency Market Research Transparency Market Research, a global market research company registered at Wilmington, Delaware, United States, provides custom research and consulting services. Our exclusive blend of quantitative forecasting and trends analysis provides forward-looking insights for thousands of decision makers. Our experienced team of Analysts, Researchers, and Consultants use proprietary data sources and various tools & techniques to gather and analyses information. Our data repository is continuously updated and revised by a team of research experts, so that it always reflects the latest trends and information. With a broad research and analysis capability, Transparency Market Research employs rigorous primary and secondary research techniques in developing distinctive data sets and research material for business reports. Contact: Transparency Market Research Inc. CORPORATE HEADQUARTER DOWNTOWN, 1000 N. West Street, Suite 1200, Wilmington, Delaware 19801 USA Tel: +1-518-618-1030 USA - Canada Toll Free: 866-552-3453 Website: https://www.transparencymarketresearch.com Email: [email protected] of Form
Bottom of Form
0 notes
Text
Drive Results with These 7 Steps for Data for AI Success
Artificial Intelligence (AI) is transforming industries—from predictive analytics in finance to personalized healthcare and smart manufacturing. But despite the hype and investment, many organizations struggle to realize tangible value from their AI initiatives. Why? Because they overlook the foundational requirement: high-quality, actionable data for AI.

AI is only as powerful as the data that fuels it. Poor data quality, silos, and lack of governance can severely hamper outcomes. To maximize returns and drive innovation, businesses must adopt a structured approach to unlocking the full value of their data for AI.
Here are 7 essential steps to make that happen.
Step 1: Establish a Data Strategy Aligned to AI Goals
The journey to meaningful AI outcomes begins with a clear strategy. Before building models or investing in platforms, define your AI objectives and align them with business goals. Do you want to improve customer experience? Reduce operational costs? Optimize supply chains?
Once goals are defined, identify what data for AI is required—structured, unstructured, real-time, historical—and where it currently resides. A comprehensive data strategy should include:
Use case prioritization
ROI expectations
Data sourcing and ownership
Key performance indicators (KPIs)
This ensures that all AI efforts are purpose-driven and data-backed.
Step 2: Break Down Data Silos Across the Organization
Siloed data is the enemy of AI. In many enterprises, critical data for AI is scattered across departments, legacy systems, and external platforms. These silos limit visibility, reduce model accuracy, and delay project timelines.
A centralized or federated data architecture is essential. This can be achieved through:
Data lakes or data fabric architectures
APIs for seamless system integration
Cloud-based platforms for unified access
Enabling open and secure data sharing across business units is the foundation of AI success.
Step 3: Ensure Data Quality, Consistency, and Completeness
AI thrives on clean, reliable, and well-labeled data. Dirty data—full of duplicates, errors, or missing values—leads to inaccurate predictions and flawed insights. Organizations must invest in robust data quality management practices.
Key aspects of quality data for AI include:
Accuracy: Correctness of data values
Completeness: No missing or empty fields
Consistency: Standardized formats across sources
Timeliness: Up-to-date and relevant
Implement automated tools for profiling, cleansing, and enriching data to maintain integrity at scale.
Step 4: Govern Data with Security and Compliance in Mind
As data for AI becomes more valuable, it also becomes more vulnerable. Privacy regulations such as GDPR and CCPA impose strict rules on how data is collected, stored, and processed. Governance is not just a legal necessity—it builds trust and ensures ethical AI.
Best practices for governance include:
Data classification and tagging
Role-based access control (RBAC)
Audit trails and lineage tracking
Anonymization or pseudonymization of sensitive data
By embedding governance early in the AI pipeline, organizations can scale responsibly and securely.
Step 5: Build Scalable Infrastructure to Support AI Workloads
Collecting data for AI is only one part of the equation. Organizations must also ensure their infrastructure can handle the scale, speed, and complexity of AI workloads.
This includes:
Scalable storage solutions (cloud-native, hybrid, or on-prem)
High-performance computing resources (GPUs/TPUs)
Data streaming and real-time processing frameworks
AI-ready data pipelines for continuous integration and delivery
Investing in flexible, future-proof infrastructure ensures that data isn’t a bottleneck but a catalyst for AI innovation.
Step 6: Use Metadata and Cataloging to Make Data Discoverable
With growing volumes of data for AI, discoverability becomes a major challenge. Teams often waste time searching for datasets that already exist, or worse, recreate them. Metadata management and data cataloging solve this problem.
A modern data catalog allows users to:
Search and find relevant datasets
Understand data lineage and usage
Collaborate through annotations and documentation
Evaluate data quality and sensitivity
By making data for AI discoverable, reusable, and transparent, businesses accelerate time-to-insight and reduce duplication.
Step 7: Foster a Culture of Data Literacy and Collaboration
Ultimately, unlocking the value of data for AI is not just about tools or technology—it’s about people. Organizations must create a data-driven culture where employees understand the importance of data and actively participate in its lifecycle.
Key steps to build such a culture include:
Training programs for non-technical teams on AI and data fundamentals
Cross-functional collaboration between data scientists, engineers, and business leaders
Incentivizing data sharing and reuse
Encouraging experimentation with small-scale AI pilots
When everyone—from C-suite to frontline workers—values data for AI, adoption increases and innovation flourishes.
Conclusion: A Roadmap to Smarter AI Outcomes
AI isn’t magic. It’s a disciplined, strategic capability that relies on well-governed, high-quality data for AI. By following these seven steps—strategy, integration, quality, governance, infrastructure, discoverability, and culture—organizations can unlock the true potential of their data assets.
In a competitive digital economy, your ability to harness the power of data for AI could determine the future of your business. Don’t leave that future to chance—invest in your data, and AI will follow.
Read Full Article : https://businessinfopro.com/7-steps-to-unlocking-the-value-of-data-for-ai/
About Us: Businessinfopro is a trusted platform delivering insightful, up-to-date content on business innovation, digital transformation, and enterprise technology trends. We empower decision-makers, professionals, and industry leaders with expertly curated articles, strategic analyses, and real-world success stories across sectors. From marketing and operations to AI, cloud, and automation, our mission is to decode complexity and spotlight opportunities driving modern business growth. At Businessinfopro, we go beyond news—we provide perspective, helping businesses stay agile, informed, and competitive in a rapidly evolving digital landscape. Whether you're a startup or a Fortune 500 company, our insights are designed to fuel smarter strategies and meaningful outcomes.
0 notes
Link
0 notes