#SAP to BigQuery
Explore tagged Tumblr posts
Text
Replication of SAP Applications to Google BigQuery
In this post, we will go into the various facets of replicating applications from SAP to BigQuery through SAP Data Services.
Presently, users can integrate data such as Google BigQuery with business data that exists in the SAP Data Warehouse Cloud of the SAP Business Technology Platform. This is done with the help of hyper-scaler storage. What is important here is that data is queried through virtual tables directly with specific tools.

It is also possible to replicate data from SAP to BigQuery and assess all SAP data in one place.
To start the process of replicating data from SAP to BigQuery, ensure that the database is on SAP HANA or any other platform supported by SAP. This SAP to BigQuery activity is typically used to merge data in SAP systems with that of BigQuery.
After the replication process is completed, this data is used for getting deep business insights from Machine Learning (ML) for petabyte scale analytics. The SAP to BigQuery replication process is not complex and SAP system administrators with knowledge of configuring SAP Basis, SAP DS, and the Google Cloud can easily do it.
Here is the replication process of SAP to BigQuery.
Update all data in the source system SAP applications
The SAP LT Replication Server replicates all changes made to the data. These are then stored in the Operational Data Queue.
A subscriber of the Operational Delta Queue, SAP DS, continually tracks changes to the data at pre-determined intervals.
The data from the delta queue is extracted by SAP DS and then processed and formatted to match the structure that is supported by BigQuery.
Finally, data is loaded from SAP to BigQuery.
0 notes
Text
AWS vs. Google Cloud : Quel Cloud Choisir pour Votre Entreprise en 2025?
L’adoption du cloud computing est désormais incontournable pour les entreprises souhaitant innover, scaler et optimiser leurs coûts. Parmi les leaders du marché, Amazon Web Services (AWS) et Google Cloud se démarquent. Mais comment choisir entre ces deux géants ? Cet article compare leurs forces, faiblesses, et cas d’usage pour vous aider à prendre une décision éclairée.
1. AWS : Le Pionnier du Cloud
Lancé en 2006, AWS domine le marché avec 32% de parts de marché (source : Synergy Group, 2023). Sa principale force réside dans son écosystème complet et sa maturité.
Points forts :
Portefeuille de services étendu : Plus de 200 services, incluant des solutions pour le calcul (EC2), le stockage (S3), les bases de données (RDS, DynamoDB), l’IA/ML (SageMaker), et l’IoT.
Globalisation : Présence dans 32 régions géographiques, idéal pour les entreprises ayant besoin de latence ultra-faible.
Enterprise-ready : Outils de gouvernance (AWS Organizations), conformité (HIPAA, GDPR), et une communauté de partenaires immense (ex : Salesforce, SAP).
Hybride et edge computing : Services comme AWS Outposts pour intégrer le cloud dans les data centers locaux.
Cas d’usage privilégiés :
Startups en forte croissance (ex : Netflix, Airbnb).
Projets nécessitant une personnalisation poussée.
Entreprises cherchant une plateforme « tout-en-un ».
2. Google Cloud : L’Expert en Data et IA
Google Cloud, bien que plus récent (2011), mise sur l’innovation technologique et son expertise en big data et machine learning. Avec environ 11% de parts de marché, il séduit par sa simplicité et ses tarifs compétitifs.
Points forts :
Data Analytics et AI/ML : Des outils comme BigQuery (analyse de données en temps réel) et Vertex AI (plateforme de ML unifiée) sont des références.
Kubernetes natif : Google a créé Kubernetes, et Google Kubernetes Engine (GKE) reste la solution la plus aboutie pour orchestrer des conteneurs.
Tarification transparente : Modèle de facturation à la seconde et remises automatiques (sustained use discounts).
Durabilité : Google Cloud vise le « zéro émission nette » d’ici 2030, un atour pour les entreprises éco-responsables.
Cas d’usage privilégiés :
Projets data-driven (ex : Spotify pour l’analyse d’utilisateurs).
Environnements cloud-native et conteneurisés.
Entreprises cherchant à intégrer de l’IA générative (ex : outils basés sur Gemini).
3. Comparatif Clé : AWS vs. Google Cloud
CritèreAWSGoogle CloudCalculEC2 (flexibilité maximale)Compute Engine (simplicité)StockageS3 (leader du marché)Cloud Storage (performant)Bases de donnéesAurora, DynamoDBFirestore, BigtableAI/MLSageMaker (outils variés)Vertex AI + intégration TensorFlowTarificationComplexe (mais réserve d’instances)Plus prévisible et flexibleSupport clientPayant (plans à partir de $29/mois)Support inclus à partir de $300/mois
4. Quel Cloud Choisir ?
Optez pour AWS si :
Vous avez besoin d’un catalogue de services exhaustif.
Votre architecture est complexe ou nécessite une hybridation.
La conformité et la sécurité sont prioritaires (secteurs régulés).
Préférez Google Cloud si :
Vos projets tournent autour de la data, de l’IA ou des conteneurs.
Vous cherchez une tarification simple et des innovations récentes.
La durabilité et l’open source sont des critères clés.
5. Tendances 2024 : IA Générative et Serverless
Les deux plateformes investissent massivement dans l’IA générative :
AWS propose Bedrock (accès à des modèles comme Claude d’Anthropic).
Google Cloud mise sur Duet AI (assistant codéveloppeur) et Gemini.
Côté serverless, AWS Lambda et Google Cloud Functions restent compétitifs, mais Google se distingue avec Cloud Run (conteneurs serverless).
Conclusion
AWS et Google Cloud répondent à des besoins différents. AWS est le choix « safe » pour une infrastructure complète, tandis que Google Cloud brille dans les projets innovants axés data et IA. Pour trancher, évaluez vos priorités : coûts, expertise technique, et roadmap à long terme.
Et vous, quelle plateforme utilisez-vous ? Partagez votre expérience en commentaire !
0 notes
Text
IoT Analytics Market Analysis: Size, Share, Scope, Forecast Trends & Industry Report 2032
The IoT Analytics Market was valued at USD 26.90 billion in 2023 and is expected to reach USD 180.36 billion by 2032, growing at a CAGR of 23.60% from 2024-2032.
The Internet of Things (IoT) Analytics Market is witnessing exponential growth as organizations worldwide increasingly rely on connected devices and real-time data to drive decision-making. As the number of IoT-enabled devices surges across sectors like manufacturing, healthcare, retail, automotive, and smart cities, the demand for analytics solutions capable of processing massive data streams is at an all-time high. These analytics not only help in gaining actionable insights but also support predictive maintenance, enhance customer experiences, and optimize operational efficiencies.
IoT Analytics Market Size, Share, Scope, Analysis, Forecast, Growth, and Industry Report 2032 suggests that advancements in cloud computing, edge analytics, and AI integration are pushing the boundaries of what’s possible in IoT ecosystems. The ability to process and analyze data at the edge, rather than waiting for it to travel to centralized data centers, is allowing businesses to act in near real-time. This acceleration in data-driven intelligence is expected to reshape entire industries by improving responsiveness and reducing operational lags.
Get Sample Copy of This Report: https://www.snsinsider.com/sample-request/5493
Market Keyplayers:
Accenture (myConcerto, Accenture Intelligent Platform Services)
Aeris (Aeris IoT Platform, Aeris Mobility Suite)
Amazon Web Services, Inc. (AWS IoT Core, AWS IoT Analytics)
Cisco Systems, Inc. (Cisco IoT Control Center, Cisco Kinetic)
Dell Inc. (Dell Edge Gateway, Dell Technologies IoT Solutions)
Hewlett Packard Enterprise Development LP (HPE IoT Platform, HPE Aruba Networks)
Google (Google Cloud IoT, Google Cloud BigQuery)
OpenText Web (OpenText IoT Platform, OpenText AI & IoT)
Microsoft (Azure IoT Suite, Microsoft Power BI)
Oracle (Oracle IoT Cloud, Oracle Analytics Cloud)
PTC (ThingWorx, Vuforia)
Salesforce, Inc. (Salesforce IoT Cloud, Salesforce Einstein Analytics)
SAP SE (SAP Leonardo IoT, SAP HANA Cloud)
SAS Institute Inc. (SAS IoT Analytics, SAS Visual Analytics)
Software AG (Cumulocity IoT, webMethods)
Teradata (Teradata Vantage, Teradata IntelliCloud)
IBM (IBM Watson IoT, IBM Maximo)
Siemens (MindSphere, Siemens IoT 2040 Gateway)
Intel (Intel IoT Platform, Intel Analytics Zoo)
Honeywell (Honeywell IoT Platform, Honeywell Forge)
Bosch (Bosch IoT Suite, Bosch Connected Industry)
Trends Shaping the IoT Analytics Market
The evolution of the IoT analytics market is marked by key trends that highlight the sector’s transition from basic connectivity to intelligent automation and predictive capabilities. One of the most significant trends is the growing integration of Artificial Intelligence (AI) and Machine Learning (ML) into analytics platforms. These technologies enable smarter data interpretation, anomaly detection, and more accurate forecasting across various IoT environments.
Another major trend is the shift toward edge analytics, where data processing is performed closer to the source. This reduces latency and bandwidth usage, making it ideal for industries where time-sensitive data is critical—such as healthcare (real-time patient monitoring) and industrial automation (machine health monitoring). Additionally, multi-cloud and hybrid infrastructure adoption is growing as companies seek flexibility, scalability, and resilience in how they handle vast IoT data streams.
Enquiry of This Report: https://www.snsinsider.com/enquiry/5493
Market Segmentation:
By Type
Descriptive Analytics
Diagnostic Analytics
Predictive Analytics
Prescriptive Analytics
By Component
Solution
Services
By Organization Size
Small & Medium Enterprises
Large Enterprises
By Deployment
On-Premises
Cloud
By Application
Energy Management
Predictive Maintenance
Asset Management
Inventory Management
Remote Monitoring
Others
By End Use
Manufacturing
Energy & Utilities
Retail & E-commerce
Healthcare & Life Sciences
Transportation & Logistics
IT & Telecom
Market Analysis
The IoT analytics market is expected to grow significantly, driven by the proliferation of connected devices and the need for real-time data insights. According to recent forecasts, the market is projected to reach multibillion-dollar valuations by 2032, growing at a robust CAGR. Key factors contributing to this growth include the increasing use of smart sensors, 5G deployment, and a shift toward Industry 4.0 practices across manufacturing and logistics sectors.
Enterprises are rapidly adopting IoT analytics to streamline operations, reduce costs, and create new revenue streams. In sectors such as smart agriculture, analytics platforms help monitor crop health and optimize water usage. In retail, real-time customer behavior data is used to enhance shopping experiences and inventory management. Governments and municipalities are also leveraging IoT analytics for smart city applications like traffic management and energy efficiency.
Future Prospects
Looking ahead, the IoT analytics market holds vast potential as the digital transformation of industries accelerates. Innovations such as digital twins—virtual replicas of physical assets that use real-time data—will become more prevalent, enabling deeper analytics and simulation-driven decision-making. The combination of 5G, IoT, and AI will unlock new use cases in autonomous vehicles, remote healthcare, and industrial robotics, where instantaneous insights are essential.
The market is also expected to see increased regulatory focus and data governance, particularly in sectors handling sensitive information. Ensuring data privacy and security while maintaining analytics performance will be a key priority. As a result, vendors are investing in secure-by-design platforms and enhancing their compliance features to align with global data protection standards.
Moreover, the democratization of analytics tools—making advanced analytics accessible to non-technical users—is expected to grow. This shift will empower frontline workers and decision-makers with real-time dashboards and actionable insights, reducing reliance on centralized data science teams. Open-source platforms and API-driven ecosystems will also support faster integration and interoperability across various IoT frameworks.
Access Complete Report: https://www.snsinsider.com/reports/iot-analytics-market-5493
Conclusion
The IoT analytics market is positioned as a cornerstone of the digital future, with its role expanding from simple monitoring to predictive and prescriptive intelligence. As the volume, variety, and velocity of IoT data continue to increase, so does the need for scalable, secure, and intelligent analytics platforms. Companies that leverage these capabilities will gain a significant competitive edge, transforming how they operate, interact with customers, and drive innovation.
About Us:
SNS Insider is one of the leading market research and consulting agencies that dominates the market research industry globally. Our company's aim is to give clients the knowledge they require in order to function in changing circumstances. In order to give you current, accurate market data, consumer insights, and opinions so that you can make decisions with confidence, we employ a variety of techniques, including surveys, video talks, and focus groups around the world.
Contact Us:
Jagney Dave - Vice President of Client Engagement
Phone: +1-315 636 4242 (US) | +44- 20 3290 5010 (UK)
0 notes
Text
Innovations in Data Orchestration: How Azure Data Factory is Adapting
Introduction
As businesses generate and process vast amounts of data, the need for efficient data orchestration has never been greater. Data orchestration involves automating, scheduling, and managing data workflows across multiple sources, including on-premises, cloud, and third-party services.
Azure Data Factory (ADF) has been a leader in ETL (Extract, Transform, Load) and data movement, and it continues to evolve with new innovations to enhance scalability, automation, security, and AI-driven optimizations.
In this blog, we will explore how Azure Data Factory is adapting to modern data orchestration challenges and the latest features that make it more powerful than ever.
1. The Evolution of Data Orchestration
🚀 Traditional Challenges
Manual data integration between multiple sources
Scalability issues in handling large data volumes
Latency in data movement for real-time analytics
Security concerns in hybrid and multi-cloud setups
🔥 The New Age of Orchestration
With advancements in cloud computing, AI, and automation, modern data orchestration solutions like ADF now provide: ✅ Serverless architecture for scalability ✅ AI-powered optimizations for faster data pipelines ✅ Real-time and event-driven data processing ✅ Hybrid and multi-cloud connectivity
2. Key Innovations in Azure Data Factory
✅ 1. Metadata-Driven Pipelines for Dynamic Workflows
ADF now supports metadata-driven data pipelines, allowing organizations to:
Automate data pipeline execution based on dynamic configurations
Reduce redundancy by using parameterized pipelines
Improve reusability and maintenance of workflows
✅ 2. AI-Powered Performance Optimization
Microsoft has introduced AI-powered recommendations in ADF to:
Suggest best data pipeline configurations
Automatically optimize execution performance
Detect bottlenecks and improve parallelism
✅ 3. Low-Code and No-Code Data Transformations
Mapping Data Flows provide a visual drag-and-drop interface
Wrangling Data Flows allow users to clean data using Power Query
Built-in connectors eliminate the need for custom scripting
✅ 4. Real-Time & Event-Driven Processing
ADF now integrates with Event Grid, Azure Functions, and Streaming Analytics, enabling:
Real-time data movement from IoT devices and logs
Trigger-based workflows for automated data processing
Streaming data ingestion into Azure Synapse, Data Lake, or Cosmos DB
✅ 5. Hybrid and Multi-Cloud Data Integration
ADF now provides:
Expanded connector support (AWS S3, Google BigQuery, SAP, Databricks)
Enhanced Self-Hosted Integration Runtime for secure on-prem connectivity
Cross-cloud data movement with Azure, AWS, and Google Cloud
✅ 6. Enhanced Security & Compliance Features
Private Link support for secure data transfers
Azure Key Vault integration for credential management
Role-based access control (RBAC) for governance
✅ 7. Auto-Scaling & Cost Optimization Features
Auto-scaling compute resources based on workload
Cost analysis tools for optimizing pipeline execution
Pay-per-use model to reduce costs for infrequent workloads
3. Use Cases of Azure Data Factory in Modern Data Orchestration
🔹 1. Real-Time Analytics with Azure Synapse
Ingesting IoT and log data into Azure Synapse
Using event-based triggers for automated pipeline execution
🔹 2. Automating Data Pipelines for AI & ML
Integrating ADF with Azure Machine Learning
Scheduling ML model retraining with fresh data
🔹 3. Data Governance & Compliance in Financial Services
Secure movement of sensitive data with encryption
Using ADF with Azure Purview for data lineage tracking
🔹 4. Hybrid Cloud Data Synchronization
Moving data from on-prem SAP, SQL Server, and Oracle to Azure Data Lake
Synchronizing multi-cloud data between AWS S3 and Azure Blob Storage
4. Best Practices for Using Azure Data Factory in Data Orchestration
✅ Leverage Metadata-Driven Pipelines for dynamic execution ✅ Enable Auto-Scaling for better cost and performance efficiency ✅ Use Event-Driven Processing for real-time workflows ✅ Monitor & Optimize Pipelines using Azure Monitor & Log Analytics ✅ Secure Data Transfers with Private Endpoints & Key Vault
5. Conclusion
Azure Data Factory continues to evolve with innovations in AI, automation, real-time processing, and hybrid cloud support. By adopting these modern orchestration capabilities, businesses can:
Reduce manual efforts in data integration
Improve data pipeline performance and reliability
Enable real-time insights and decision-making
As data volumes grow and cloud adoption increases, Azure Data Factory’s future-ready approach ensures that enterprises stay ahead in the data-driven world.
WEBSITE: https://www.ficusoft.in/azure-data-factory-training-in-chennai/
0 notes
Text
SAP Analytics Cloud: Transforming Business Intelligence and Data Analytics
In today's fast-paced digital world, businesses need innovative tools to effectively manage and analyze vast amounts of data. SAP Analytics Cloud (SAC) is one such platform that integrates data analytics, visualization, and business intelligence (BI) to help organizations of all sizes make data-driven decisions.
What is SAP Analytics Cloud?
SAP Analytics Cloud is an all-in-one, cloud-based business intelligence platform that brings together multiple capabilities, such as data visualization, data analysis, planning, and predictive analytics. It allows businesses to leverage data insights through intuitive tools, empowering users to make informed decisions.
This powerful software provides integration with various data sources, from on-premise systems to cloud-based solutions, helping businesses create a unified view of their data.
Key Features of SAP Analytics Cloud
Data Connectivity and Integration SAP Analytics Cloud seamlessly integrates with numerous data sources such as SAP HANA, SAP BW, Google BigQuery, and Microsoft Excel, among others. Its ability to consolidate data from different systems allows businesses to create a comprehensive data view and make accurate, actionable decisions.
Business Intelligence One of the standout features of SAP Analytics Cloud is its business intelligence (BI) capabilities. With SAC, users can build dynamic dashboards, reports, and visualizations to better understand business performance. These BI features empower organizations to track their key performance indicators (KPIs) and monitor business health in real-time.
Planning and Forecasting SAP Analytics Cloud also excels in planning and forecasting. Whether it’s budgeting, financial planning, or resource allocation, SAC provides robust planning capabilities. The platform's predictive analytics help businesses anticipate future trends, allowing them to plan more effectively.
Predictive Analytics Through predictive analytics, SAP Analytics Cloud goes beyond just reporting. By using machine learning algorithms, SAC identifies trends and makes future predictions. This allows businesses to stay ahead of market changes, forecast customer demands, and improve overall strategic decision-making.
Collaboration SAP Analytics Cloud promotes collaboration with features that allow team members to share dashboards, provide feedback, and discuss insights in real-time. This built-in collaboration framework ensures that teams can align on data-driven decisions and work towards shared business objectives.
How SAP Analytics Cloud Improves Business Performance
The true value of SAP Analytics Cloud lies in its ability to enhance decision-making processes. By integrating business intelligence, predictive analytics, and planning tools, SAC provides businesses with the ability to:
Make Data-Driven Decisions: With real-time access to consolidated data, businesses can base decisions on up-to-date information, rather than relying on outdated reports.
Increase Efficiency: SAC’s user-friendly interface and automated features help reduce the time required for data analysis, allowing employees to focus on more important tasks.
Enhance Forecasting: With its powerful predictive features, SAC enables businesses to make more accurate forecasts, helping them prepare for the future.
Boost Collaboration: By fostering collaboration across teams, SAC ensures that everyone in an organization works towards common goals, using the same data and insights.
Benefits of Using SAP Analytics Cloud
Cost-Effectiveness As a cloud-based solution, SAP Analytics Cloud eliminates the need for large investments in hardware and infrastructure. Businesses pay for only the resources they use, making it a cost-effective option for companies of all sizes.
Scalability SAP Analytics Cloud is scalable, allowing it to grow with your business. Whether you're a small organization or a large enterprise, SAC can meet your increasing data needs and analytics requirements.
Real-Time Insights One of SAC's most valuable features is its ability to provide real-time insights. With instant access to data, businesses can react quickly to changes in the market or customer behavior.
User-Friendly Interface Even those without a technical background can easily use SAP Analytics Cloud. The platform’s intuitive interface ensures that everyone—from business analysts to executives—can access and interpret data without requiring specialized knowledge.
SAP Analytics Cloud vs. Other Analytics Tools
While there are many analytics platforms available, SAP Analytics Cloud stands out due to its comprehensive features. Unlike traditional business intelligence tools, SAC integrates data visualization, predictive analytics, and planning into one unified platform. This holistic approach allows businesses to address all their data-related needs with one tool.
Furthermore, its cloud-based model offers flexibility and scalability, making it ideal for businesses of all sizes. If your organization already uses SAP's ERP solutions, SAC provides seamless integration, making it an even more valuable tool.
Conclusion
SAP Analytics Cloud is a game-changing tool for businesses looking to leverage data analytics, forecasting, and collaborative decision-making. By providing business intelligence, predictive analytics, and planning tools in one platform, SAC helps organizations make smarter, faster, and more informed decisions. Whether you're a small business or a large enterprise, the scalability and flexibility of SAP Analytics Cloud can drive your organization’s growth and success, making it an invaluable asset in the modern data-driven landscape.
0 notes
Text
Best Informatica Cloud Training in India | Informatica IICS
Cloud Data Integration (CDI) in Informatica IICS
Introduction
Cloud Data Integration (CDI) in Informatica Intelligent Cloud Services (IICS) is a powerful solution that helps organizations efficiently manage, process, and transform data across hybrid and multi-cloud environments. CDI plays a crucial role in modern ETL (Extract, Transform, Load) and ELT (Extract, Load, Transform) operations, enabling businesses to achieve high-performance data processing with minimal complexity. In today’s data-driven world, businesses need seamless integration between various data sources, applications, and cloud platforms. Informatica Training Online
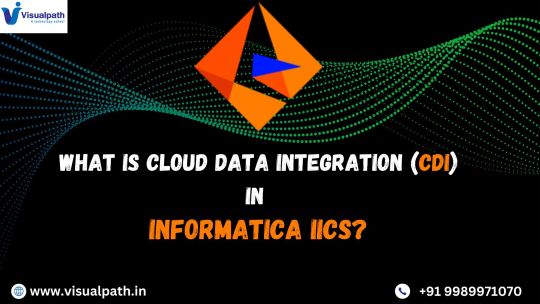
What is Cloud Data Integration (CDI)?
Cloud Data Integration (CDI) is a Software-as-a-Service (SaaS) solution within Informatica IICS that allows users to integrate, transform, and move data across cloud and on-premises systems. CDI provides a low-code/no-code interface, making it accessible for both technical and non-technical users to build complex data pipelines without extensive programming knowledge.
Key Features of CDI in Informatica IICS
Cloud-Native Architecture
CDI is designed to run natively on the cloud, offering scalability, flexibility, and reliability across various cloud platforms like AWS, Azure, and Google Cloud.
Prebuilt Connectors
It provides out-of-the-box connectors for SaaS applications, databases, data warehouses, and enterprise applications such as Salesforce, SAP, Snowflake, and Microsoft Azure.
ETL and ELT Capabilities
Supports ETL for structured data transformation before loading and ELT for transforming data after loading into cloud storage or data warehouses.
Data Quality and Governance
Ensures high data accuracy and compliance with built-in data cleansing, validation, and profiling features. Informatica IICS Training
High Performance and Scalability
CDI optimizes data processing with parallel execution, pushdown optimization, and serverless computing to enhance performance.
AI-Powered Automation
Integrated Informatica CLAIRE, an AI-driven metadata intelligence engine, automates data mapping, lineage tracking, and error detection.
Benefits of Using CDI in Informatica IICS
1. Faster Time to Insights
CDI enables businesses to integrate and analyze data quickly, helping data analysts and business teams make informed decisions in real-time.
2. Cost-Effective Data Integration
With its serverless architecture, businesses can eliminate on-premise infrastructure costs, reducing Total Cost of Ownership (TCO) while ensuring high availability and security.
3. Seamless Hybrid and Multi-Cloud Integration
CDI supports hybrid and multi-cloud environments, ensuring smooth data flow between on-premises systems and various cloud providers without performance issues. Informatica Cloud Training
4. No-Code/Low-Code Development
Organizations can build and deploy data pipelines using a drag-and-drop interface, reducing dependency on specialized developers and improving productivity.
5. Enhanced Security and Compliance
Informatica ensures data encryption, role-based access control (RBAC), and compliance with GDPR, CCPA, and HIPAA standards, ensuring data integrity and security.
Use Cases of CDI in Informatica IICS
1. Cloud Data Warehousing
Companies migrating to cloud-based data warehouses like Snowflake, Amazon Redshift, or Google BigQuery can use CDI for seamless data movement and transformation.
2. Real-Time Data Integration
CDI supports real-time data streaming, enabling enterprises to process data from IoT devices, social media, and APIs in real-time.
3. SaaS Application Integration
Businesses using applications like Salesforce, Workday, and SAP can integrate and synchronize data across platforms to maintain data consistency. IICS Online Training
4. Big Data and AI/ML Workloads
CDI helps enterprises prepare clean and structured datasets for AI/ML model training by automating data ingestion and transformation.
Conclusion
Cloud Data Integration (CDI) in Informatica IICS is a game-changer for enterprises looking to modernize their data integration strategies. CDI empowers businesses to achieve seamless data connectivity across multiple platforms with its cloud-native architecture, advanced automation, AI-powered data transformation, and high scalability. Whether you’re migrating data to the cloud, integrating SaaS applications, or building real-time analytics pipelines, Informatica CDI offers a robust and efficient solution to streamline your data workflows.
For organizations seeking to accelerate digital transformation, adopting Informatics’ Cloud Data Integration (CDI) solution is a strategic step toward achieving agility, cost efficiency, and data-driven innovation.
For More Information about Informatica Cloud Online Training
Contact Call/WhatsApp: +91 7032290546
Visit: https://www.visualpath.in/informatica-cloud-training-in-hyderabad.html
#Informatica Training in Hyderabad#IICS Training in Hyderabad#IICS Online Training#Informatica Cloud Training#Informatica Cloud Online Training#Informatica IICS Training#Informatica Training Online#Informatica Cloud Training in Chennai#Informatica Cloud Training In Bangalore#Best Informatica Cloud Training in India#Informatica Cloud Training Institute#Informatica Cloud Training in Ameerpet
0 notes
Text
Best Software for Predictive Analytics in 2025

As we approach 2025, businesses are increasingly relying on predictive analytics software to make smarter, data-driven decisions. These tools analyze vast amounts of data to predict future trends, helping organizations stay ahead of the curve. With data creation expected to reach 180 zettabytes by 2025, leveraging predictive analytics has never been more crucial.
Here are the top 5 predictive analytics software of 2025:
Google Cloud BigQuery: Known for its serverless architecture, BigQuery allows businesses to analyze massive datasets quickly, with machine learning integrations for predictive insights. Its real-time analytics and geospatial capabilities make it ideal for businesses needing fast, scalable predictions.
Amazon QuickSight: This AWS-powered tool uses machine learning and natural language processing to forecast trends and identify anomalies. QuickSight’s auto-scaling architecture ensures it performs well even with millions of queries, making it ideal for companies in the AWS ecosystem.
Adobe Analytics: A leader in marketing analytics, Adobe Analytics uses AI-powered insights to predict customer behaviors and trends. It excels in cross-channel tracking and real-time data visualization, offering businesses a 360-degree view of customer journeys.
Tableau: Known for its interactive dashboards, Tableau integrates with Salesforce Einstein Discovery to provide predictive analytics. Its ability to handle complex datasets from diverse sources makes it a top choice for data-driven decision-making.
SAP Analytics Cloud: Combining predictive analytics with business intelligence, SAP Analytics Cloud offers AI-driven forecasting and smart insights. It allows seamless integration with other SAP applications, providing a unified workflow for enterprise-level planning.
These tools empower businesses to harness their data, uncover insights, and make informed decisions, ensuring they are prepared for the future.
0 notes
Text
What are the alternatives for SAP HANA?
SAP HANA is a leading in-memory database platform, but several alternatives cater to different organizational needs. Popular options include Oracle Database, known for its scalability and robust features; Microsoft SQL Server, offering integration with Microsoft tools; IBM Db2, recognized for AI-driven analytics; Amazon Aurora, a cloud-based solution with high availability; and Google BigQuery, designed for big data analytics.
If you're looking to upgrade your SAP skills, Anubhav Online Training is highly recommended.
Anubhav Oberoy, a globally renowned corporate trainer, offers comprehensive SAP courses suitable for both beginners and professionals. His sessions are tailored for practical, industry-relevant knowledge and are available online to accommodate global participants. Check out his upcoming batches at Anubhav Trainings. Whether you're pursuing a career in SAP or seeking to enhance your expertise, Anubhav's training programs provide exceptional guidance and real-world insights.
#free online sap training#sap online training#sap abap training#sap hana training#sap ui5 and fiori training#best corporate training#best sap corporate training#sap corporate training#online sap corporate training
0 notes
Text
Overview Of The ABAP SDK For Google Cloud And Use cases
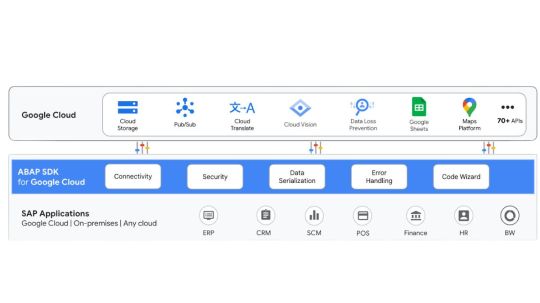
ABAP SDK for Google Cloud
With the ABAP SDK for Google Cloud, SAP developers may leverage Google Cloud‘s capabilities in their preferred ABAP programming language. The SDK is offered as an ABAP class collection of client libraries. ABAP developers can access and utilize Google Cloud APIs by using these classes.
ABAP developers can concentrate on creating the business logic because the SDK handles the laborious tasks of developing connectivity, security, data serialization, and error handling right out of the box. A code wizard is also included in the SDK to help you get started with boilerplate code quickly. This shortens the time to business value and significantly reduces the amount of code that developers must write.
Use cases
With Google Cloud‘s ABAP SDK, you can create useful business apps. Typical use cases include:
Transform insights into actions in real time
Use generative AI in your SAP apps to extract actionable insights from large amounts of unstructured and structured business data to help you make better business decisions.
Automate and streamline SAP business procedures
Create extensions that use Document AI, Address Validation, Cloud Translation AI, and Cloud Storage to automate business activities like posting sales orders.
Smooth systems and integration of data
Exchange business process data with external systems by utilizing event-driven architecture in conjunction with Pub/Sub and BigQuery.
Safe SAP apps and systems
To safely store, retrieve, and send sensitive data, use Cloud Key Management Service’s Secret Manager.
These are but a handful of common application cases in business. With support for over 70 Google Cloud APIs, the ABAP SDK for Google Cloud extends the Google Cloud’s capabilities to the ABAP platform, giving you countless chances to revolutionize your company.
ABAP SDK for Google Cloud editions
The two variants of the ABAP SDK for Google Cloud give developers the ability to utilize the SDK for ABAP programs in the cloud, on-premises, on Google Cloud, on any other cloud, and on S/4HANA Cloud Private Edition and S/4HANA Cloud Public Edition.
SAP BTP edition: for usage with other cloud ABAP applications, including S/4HANA Cloud Private Edition and S/4HANA Cloud Public Edition. On-premises or any cloud edition: S/4HANA, ECC, and S/4HANA Cloud Private Edition can be used on-premises or in any cloud edition.
The two ABAP SDK editions for Google Cloud are depicted in the following diagram along with installation locations. No matter the edition, the SDK offers connection with more than 70 Google Cloud APIs, enabling you to create creative solutions for a variety of SAP business operations.
SAP BTP edition
In the SAP BTP, ABAP environment, you install the ABAP SDK for Google Cloud‘s SAP BTP edition.
With this edition, you can use SAP’s side-by-side extension suggestion to create extensions and connectors.
See What’s new with the SAP BTP edition of the ABAP SDK for Google Cloud for information on updates and improvements to the SAP BTP edition.
On-premises or any cloud edition
The ABAP SDK for Google Cloud can be installed on-premises or on any cloud instance on your SAP host system running Compute Engine, RISE with S/4HANA Cloud Private edition, or any cloud virtual machine.
With this version, you may create integrations and in-app extensions right within your SAP application.
For smooth communication with Google Cloud’s Vertex AI platform, the on-premises or cloud edition of the ABAP SDK for Google Cloud, starting with version 1.8, provides a specialized tool called Vertex AI SDK for ABAP. Vertex AI SDK for ABAP Overview provides details about the Vertex AI SDK for ABAP.
See What’s new with the on-premises or any cloud edition of ABAP SDK for Google Cloud for information on updates and improvements to the on-premises or any cloud edition of the technology.
Reference architectures
With the aid of the reference architectures, investigate the ABAP SDK for Google Cloud and learn how the SDK may innovate your SAP application environment. For more sophisticated AI and machine learning features, you may utilize the SDK to integrate with Vertex AI and other Google Cloud services like BigQuery, Pub/Sub, Cloud Storage, and many more.
Google Cloud community
On Cloud Forums, you can talk with other users about the ABAP SDK for Google Cloud.
Local resources
You might investigate the following community resources to help you make the most of the ABAP SDK for Google Cloud:
Cloud Storage as content repository
By establishing a connection between your SAP system and Cloud Storage, it enables you to store attachments and archive previous SAP data. This open-source solution implements the SAP Content Server Interface and was created with the ABAP SDK for Google Cloud. It can be set up to archive data files and save and retrieve PDF documents using a SAP GUI panel.
OpenAPI Generator for ABAP SDK for Google Cloud
By producing ABAP classes that are compatible with the ABAP SDK for Google Cloud, the OpenAPI Generator for ABAP SDK for Google Cloud enables you to include your private or custom APIs hosted on Google Cloud into your SAP applications.
Read more on Govindhtech.com
#ABAP#SDK#ABAPSDK#CloudStorage#S/4HANA#APIs#SAPBTP#VertexAISDK#News#Technews#Technology#Technologynews#Technologytrends#govindhtech
0 notes
Text
SAP Analytics Cloud
SAP Analytics Cloud (SAC), part of the SAP BTP platform, is a cloud-based enterprise analytics solution that integrates Business Intelligence (BI), planning, and predictive analytics into a unified workflow. SAC offers a comprehensive view of organizational data by connecting with various sources, such as SAP BW, SAP S/4HANA, Google BigQuery, SQL databases, and open formats like CSV and JSON. It supports both live and data acquisition connections, delivering real-time insights and advanced analytics, including predictive modeling and machine learning.
The platform includes over 100 pre-built SAP business content packages to speed up implementation and supports collaborative financial planning and budgeting with real-time data and simulations. SAC features robust security with role-based access control, encryption, and GDPR compliance.
Recent enhancements include unified story and application artifacts, improved running total calculations, and optimized planning workflows. Upcoming updates will integrate with SAP Data Sphere and introduce advanced custom widgets. SAC’s design facilitates seamless integration with the SAP ecosystem, promoting self-service analytics and operational efficiency.
0 notes
Text
SNOWFLAKE BIGQUERY
Snowflake vs. BigQuery: Choosing the Right Cloud Data Warehouse
The cloud data warehouse market is booming and for good reasons. Modern cloud data warehouses offer scalability, performance, and ease of management that traditional on-premises solutions can’t match. Two titans in this space are Snowflake and Google BigQuery. Let’s break down their strengths, weaknesses, and ideal use cases.
Architectural Foundations
Snowflake employs a hybrid architecture with separate storage and compute layers, which allows for independent resource scaling. Snowflake uses “virtual warehouses,” which are clusters of compute nodes, to handle query execution.
BigQuery: Leverages a serverless architecture, meaning users don’t need to worry about managing the computing infrastructure. BigQuery automatically allocates resources behind the scenes, simplifying the user experience.
Performance
Snowflake and BigQuery deliver exceptional performance for complex analytical queries on massive datasets. However, there are nuances:
Snowflake: Potentially offers better fine-tuning. Users can select different virtual warehouse sizes for specific workloads and change them on the fly.
BigQuery: Generally shines in ad-hoc analysis due to its serverless nature and ease of getting started.
Data Types and Functionality
Snowflake: Provides firm support for semi-structured data (JSON, Avro, Parquet, XML), offering flexibility when dealing with data from various sources.
BigQuery: Excels with structured data and has native capabilities for geospatial analysis.
Pricing Models
Snowflake: Primarily usage-based with per-second billing for virtual warehouses. Offers both on-demand and pre-purchased capacity options.
BigQuery provides a usage-based model where you pay for the data processed. It also offers flat-rate pricing options for predictable workloads.
Use Cases
Snowflake
Environments with fluctuating workloads or unpredictable query patterns.
Workloads heavily rely on semi-structured data.
Organizations desiring fine control over compute scaling.
BigQuery
Ad-hoc analysis and rapid exploration of large datasets
Companies integrated with the Google Cloud Platform (GCP) ecosystem.
Workloads requiring geospatial analysis capabilities.
Beyond the Basics
Security: Both platforms offer robust security features, such as data encryption, role-based access control, and support for various compliance standards.
Multi-Cloud Support: Snowflake is available across the top cloud platforms (AWS, Azure, GCP), while BigQuery is native to GCP.
Ecosystem: Snowflake and BigQuery boast well-developed communities, integrations, and a wide range of third-party tools.
Making the Decision
There’s no clear-cut “winner” between Snowflake and BigQuery. The best choice depends on your organization’s specific needs:
Assess your current and future data volume and complexity.
Consider how the pricing models align with your budget and usage patterns.
Evaluate your technical team’s comfort level with managing infrastructure ( Snowflake) vs. a more fully managed solution (BigQuery).
Factor in any existing investments in specific cloud platforms or ecosystems.
Remember: The beauty of the cloud is that you can often experiment with Snowflake and BigQuery. Consider proofs of concept or use free trial periods to test them in real-world scenarios with your data.
youtube
You can find more information about Snowflake in this Snowflake
Conclusion:
Unogeeks is the No.1 IT Training Institute for SAP Training. Anyone Disagree? Please drop in a comment
You can check out our other latest blogs on Snowflake here – Snowflake Blogs
You can check out our Best In Class Snowflake Details here – Snowflake Training
Follow & Connect with us:
———————————-
For Training inquiries:
Call/Whatsapp: +91 73960 33555
Mail us at: [email protected]
Our Website ➜ https://unogeeks.com
Follow us:
Instagram: https://www.instagram.com/unogeeks
Facebook: https://www.facebook.com/UnogeeksSoftwareTrainingInstitute
Twitter: https://twitter.com/unogeeks
0 notes
Text
SNOWFLAKE GCP
Snowflake on GCP: Powering Up Your Data Analytics
Snowflake, the revolutionary cloud data platform, seamlessly integrates with Google Cloud Platform (GCP), offering businesses a powerful combination of data warehousing, analytics, and data-driven insights. If you’re exploring cloud data solutions, Snowflake on GCP provides an extraordinary opportunity to streamline your operations and enhance decision-making.
Why Snowflake on GCP?
Here’s why this duo is a compelling choice for modern data architecture:
Performance and Scalability: GCP’s global infrastructure, known for its speed and reach, provides an ideal foundation for Snowflake’s unique multi-cluster, shared-data architecture. This means you can experience lightning-fast query performance even when dealing with massive datasets or complex workloads.
Separation of Storage and Compute: Snowflake’s architecture decouples storage and compute resources. You can scale each independently, optimizing costs and ensuring flexibility to meet changing demands. If you need more computational power for complex analysis, scale up your virtual warehouses without worrying about adding storage.
Ease of Use: Snowflake is a fully managed service that takes care of infrastructure setup, maintenance, and upgrades. This frees up your team to focus on data analysis and strategy rather than administrative tasks.
Pay-Per-Use Model: Snowflake and GCP offer pay-per-use pricing, ensuring you only pay for the resources you consume. This promotes cost control and makes budgeting predictable.
Native Integration with GCP Services: Effortlessly connect Snowflake with GCP’s powerful tools like BigQuery, Google Cloud Storage, Looker, and more. This integration unlocks advanced analytics and machine learning capabilities, enabling you to extract the maximum value from your data.
Critical Use Cases for Snowflake on GCP
Data Warehousing and Analytics: Snowflake’s scalability and performance make it ideal as a modern data warehouse. Effortlessly centralize your data from various sources, structure it, and use it for comprehensive reporting and business intelligence.
Data Lake Enablement: Snowflake’s ability to query data directly from cloud storage, like Google Cloud Storage, turns your storage into a flexible, cost-effective data lake. Analyze raw, semi-structured, and structured data without complex ETL processes.
Data Science and Machine Learning: Accelerate data preparation for your data science and machine learning initiatives. With Snowflake accessing data in GCP, data scientists, and ML engineers spend less time on data wrangling and more time building models.
Getting Started
Setting up Snowflake on GCP is a straightforward process within the Snowflake interface. It involves:
Creating a Snowflake Account: If you haven’t already, sign up for a Snowflake account.
Selecting Google Cloud Platform: During account creation, choose GCP as your preferred cloud platform.
Configuring Integrations: Set up secure integrations between Snowflake and other GCP services you want to use (e.g., Google Cloud Storage for a data lake).
Let’s Wrap Up
The combination of Snowflake and GCP empowers organizations to build robust, agile, and cost-effective data ecosystems. If you want to modernize your data infrastructure, enhance analytical performance, and gain transformative insights, Snowflake on GCP is an alliance worth exploring.
youtube
You can find more information about Snowflake in this Snowflake
Conclusion:
Unogeeks is the No.1 IT Training Institute for SAP Training. Anyone Disagree? Please drop in a comment
You can check out our other latest blogs on Snowflake here – Snowflake Blogs
You can check out our Best In Class Snowflake Details here – Snowflake Training
Follow & Connect with us:
———————————-
For Training inquiries:
Call/Whatsapp: +91 73960 33555
Mail us at: [email protected]
Our Website ➜ https://unogeeks.com
Follow us:
Instagram: https://www.instagram.com/unogeeks
Facebook: https://www.facebook.com/UnogeeksSoftwareTrainingInstitute
Twitter: https://twitter.com/unogeeks
0 notes
Text
Big Data and Analytics in GCC Market: Size, Share, Scope, Analysis, Forecast, Growth and Industry Report 2032 – Retail and E-commerce Trends
Big Data and Analytics are transforming the operational frameworks of Global Capability Centers (GCCs) across the globe. As businesses increasingly recognize the pivotal role of data in driving strategic initiatives, Global Capability Centers are evolving into centers of excellence for data-driven decision-making. According to research 76% of Global Capability Centers identified data as a critical area for future growth,
Big Data and Analytics in GCC Market is experiencing rapid growth due to the region’s digital transformation initiatives. Governments and enterprises are leveraging data to drive innovation, optimize services, and improve decision-making. As a result, demand for data-driven strategies is surging across sectors.
Big Data and Analytics in GCC Market continues to evolve with the rising adoption of AI, cloud computing, and IoT technologies. From smart cities to healthcare and finance, businesses in the Gulf Cooperation Council (GCC) are embracing analytics to remain competitive, improve operational efficiency, and enhance customer experiences.
Get Sample Copy of This Report: https://www.snsinsider.com/sample-request/4716
Market Keyplayers:
IBM Corporation (IBM Watson, IBM Cloud Pak for Data)
Microsoft Corporation (Microsoft Azure, Power BI)
Oracle Corporation (Oracle Analytics Cloud, Oracle Big Data Service)
SAP SE (SAP HANA, SAP BusinessObjects)
SAS Institute Inc. (SAS Viya, SAS Data Management)
Google LLC (Google Cloud Platform, BigQuery)
Amazon Web Services (AWS) (Amazon Redshift, Amazon EMR)
Tableau Software (Tableau Desktop, Tableau Online)
Teradata Corporation (Teradata Vantage, Teradata Cloud)
Cloudera, Inc. (Cloudera Data Platform, Cloudera Machine Learning)
Snowflake Inc. (Snowflake Cloud Data Platform)
MicroStrategy Incorporated (MicroStrategy Analytics)
Qlik Technologies (Qlik Sense, QlikView)
Palantir Technologies (Palantir Foundry, Palantir Gotham)
TIBCO Software Inc. (TIBCO Spotfire, TIBCO Data Science)
Domo, Inc. (Domo Business Cloud)
Sisense Inc. (Sisense for Cloud Data Teams, Sisense Fusion)
Alteryx, Inc. (Alteryx Designer, Alteryx Connect)
Zoho Corporation (Zoho Analytics, Zoho DataPrep)
ThoughtSpot Inc. (ThoughtSpot Search & AI-Driven Analytics)
Trends Shaping the Market
Government-Led Digital Initiatives: National visions such as Saudi Arabia’s Vision 2030 and the UAE’s Smart Government strategy are fueling the adoption of big data solutions across public and private sectors.
Growth in Smart City Projects: Cities like Riyadh, Dubai, and Doha are integrating big data analytics into infrastructure development, transportation, and citizen services to enhance urban living.
Increased Investment in Cloud and AI: Cloud-based analytics platforms and AI-powered tools are gaining traction, enabling scalable and real-time insights.
Sector-Wide Adoption: Industries including oil & gas, healthcare, finance, and retail are increasingly utilizing analytics for predictive insights, risk management, and personalization.
Enquiry of This Report: https://www.snsinsider.com/enquiry/4716
Market Segmentation:
By Type
Shared Service Centers
Innovation Centers
Delivery Centers
By Industry Vertical
Banking and Financial Services
Healthcare
Retail
Manufacturing
Telecommunications
By Functionality
Descriptive Analytics
Predictive Analytics
Prescriptive Analytics
Real-time Analytics
By Technology Type
Data Management
Analytics Tools
Artificial Intelligence & Machine Learning
By End-User
Large Enterprises
Small and Medium Enterprises (SMEs)
Market Analysis
Accelerated Digital Transformation: Organizations across the GCC are shifting to digital-first operations, creating vast amounts of data that require robust analytics solutions.
Public and Private Sector Collaboration: Joint efforts between governments and tech firms are fostering innovation, resulting in smart platforms for public services, energy, and education.
Data-Driven Decision Making: Businesses are leveraging data to improve ROI, streamline operations, and personalize offerings—especially in e-commerce, banking, and telecommunications.
Cybersecurity and Data Privacy Awareness: With the increase in data generation, there’s a growing emphasis on securing data through advanced governance and compliance frameworks.
Future Prospects
The Big Data and Analytics in GCC Market is expected to witness exponential growth over the next five years. With increasing internet penetration, 5G rollout, and continued focus on digital infrastructure, data-driven technologies will become even more central to economic and social development in the region.
Talent Development and Upskilling: Governments are investing in training programs and digital literacy to prepare a workforce capable of managing and interpreting big data.
Emerging Startups and Innovation Hubs: The GCC is witnessing a rise in homegrown analytics startups and incubators that are driving localized solutions tailored to regional needs.
AI Integration: The convergence of AI with big data will unlock new insights and automate complex tasks in sectors such as logistics, healthcare diagnostics, and financial modeling.
Regulatory Frameworks: Future success will depend on the creation of robust regulatory policies ensuring data privacy, cross-border data flows, and ethical AI usage.
Access Complete Report: https://www.snsinsider.com/reports/big-data-and-analytics-in-gcc-market-4716
Conclusion
The Big Data and Analytics in GCC Market stands at the forefront of digital transformation. With strong government backing, sector-wide adoption, and a growing tech ecosystem, the region is well-positioned to become a data-driven powerhouse. As the market matures, the focus will shift from data collection to intelligent utilization—empowering smarter decisions, better services, and sustainable growth across the GCC.
About Us:
SNS Insider is one of the leading market research and consulting agencies that dominates the market research industry globally. Our company's aim is to give clients the knowledge they require in order to function in changing circumstances. In order to give you current, accurate market data, consumer insights, and opinions so that you can make decisions with confidence, we employ a variety of techniques, including surveys, video talks, and focus groups around the world.
Contact Us:
Jagney Dave - Vice President of Client Engagement
Phone: +1-315 636 4242 (US) | +44- 20 3290 5010 (UK)
0 notes
Text
Exploring the Role of Azure Data Factory in Hybrid Cloud Data Integration
Introduction
In today’s digital landscape, organizations increasingly rely on hybrid cloud environments to manage their data. A hybrid cloud setup combines on-premises data sources, private clouds, and public cloud platforms like Azure, AWS, or Google Cloud. Managing and integrating data across these diverse environments can be complex.
This is where Azure Data Factory (ADF) plays a crucial role. ADF is a cloud-based data integration service that enables seamless movement, transformation, and orchestration of data across hybrid cloud environments.
In this blog, we’ll explore how Azure Data Factory simplifies hybrid cloud data integration, key use cases, and best practices for implementation.
1. What is Hybrid Cloud Data Integration?
Hybrid cloud data integration is the process of connecting, transforming, and synchronizing data between: ✅ On-premises data sources (e.g., SQL Server, Oracle, SAP) ✅ Cloud storage (e.g., Azure Blob Storage, Amazon S3) ✅ Databases and data warehouses (e.g., Azure SQL Database, Snowflake, BigQuery) ✅ Software-as-a-Service (SaaS) applications (e.g., Salesforce, Dynamics 365)
The goal is to create a unified data pipeline that enables real-time analytics, reporting, and AI-driven insights while ensuring data security and compliance.
2. Why Use Azure Data Factory for Hybrid Cloud Integration?
Azure Data Factory (ADF) provides a scalable, serverless solution for integrating data across hybrid environments. Some key benefits include:
✅ 1. Seamless Hybrid Connectivity
ADF supports over 90+ data connectors, including on-prem, cloud, and SaaS sources.
It enables secure data movement using Self-Hosted Integration Runtime to access on-premises data sources.
✅ 2. ETL & ELT Capabilities
ADF allows you to design Extract, Transform, and Load (ETL) or Extract, Load, and Transform (ELT) pipelines.
Supports Azure Data Lake, Synapse Analytics, and Power BI for analytics.
✅ 3. Scalability & Performance
Being serverless, ADF automatically scales resources based on data workload.
It supports parallel data processing for better performance.
✅ 4. Low-Code & Code-Based Options
ADF provides a visual pipeline designer for easy drag-and-drop development.
It also supports custom transformations using Azure Functions, Databricks, and SQL scripts.
✅ 5. Security & Compliance
Uses Azure Key Vault for secure credential management.
Supports private endpoints, network security, and role-based access control (RBAC).
Complies with GDPR, HIPAA, and ISO security standards.
3. Key Components of Azure Data Factory for Hybrid Cloud Integration
1️⃣ Linked Services
Acts as a connection between ADF and data sources (e.g., SQL Server, Blob Storage, SFTP).
2️⃣ Integration Runtimes (IR)
Azure-Hosted IR: For cloud data movement.
Self-Hosted IR: For on-premises to cloud integration.
SSIS-IR: To run SQL Server Integration Services (SSIS) packages in ADF.
3️⃣ Data Flows
Mapping Data Flow: No-code transformation engine.
Wrangling Data Flow: Excel-like Power Query transformation.
4️⃣ Pipelines
Orchestrate complex workflows using different activities like copy, transformation, and execution.
5️⃣ Triggers
Automate pipeline execution using schedule-based, event-based, or tumbling window triggers.
4. Common Use Cases of Azure Data Factory in Hybrid Cloud
🔹 1. Migrating On-Premises Data to Azure
Extracts data from SQL Server, Oracle, SAP, and moves it to Azure SQL, Synapse Analytics.
🔹 2. Real-Time Data Synchronization
Syncs on-prem ERP, CRM, or legacy databases with cloud applications.
🔹 3. ETL for Cloud Data Warehousing
Moves structured and unstructured data to Azure Synapse, Snowflake for analytics.
🔹 4. IoT and Big Data Integration
Collects IoT sensor data, processes it in Azure Data Lake, and visualizes it in Power BI.
🔹 5. Multi-Cloud Data Movement
Transfers data between AWS S3, Google BigQuery, and Azure Blob Storage.
5. Best Practices for Hybrid Cloud Integration Using ADF
✅ Use Self-Hosted IR for Secure On-Premises Data Access ✅ Optimize Pipeline Performance using partitioning and parallel execution ✅ Monitor Pipelines using Azure Monitor and Log Analytics ✅ Secure Data Transfers with Private Endpoints & Key Vault ✅ Automate Data Workflows with Triggers & Parameterized Pipelines
6. Conclusion
Azure Data Factory plays a critical role in hybrid cloud data integration by providing secure, scalable, and automated data pipelines. Whether you are migrating on-premises data, synchronizing real-time data, or integrating multi-cloud environments, ADF simplifies complex ETL processes with low-code and serverless capabilities.
By leveraging ADF’s integration runtimes, automation, and security features, organizations can build a resilient, high-performance hybrid cloud data ecosystem.
WEBSITE: https://www.ficusoft.in/azure-data-factory-training-in-chennai/
0 notes
Text
Data warehouse cloud solutions, such as Amazon Redshift, Google BigQuery, and Snowflake, have transformed the way organizations handle data.
SAP DataSphere offers several features and capabilities to ensure data quality and integrity. Here are some key aspects of DWC that contribute to maintaining data quality:
Data Modeling: DataSphere allows users to create a logical data model that defines the structure, relationships, and business rules of the data. By establishing a standardized model, data integrity can be enforced consistently across different data sources and transformations.
Data Integration: DataSphere provides connectors and integration capabilities to pull data from various sources, such as databases, cloud applications, and on-premises systems. This integration process can include data cleansing, data transformation, and data enrichment steps to ensure that the data being loaded into DataSphere is accurate, complete, and consistent.
Data Profiling: DataSphere includes data profiling functionality that enables users to analyze the quality of data before it is loaded into the system. Data profiling helps identify data anomalies, such as missing values, duplicates, or inconsistencies, allowing users to take corrective actions before the data is used for reporting or analysis.
Data Validation: DataSphere allows users to define validation rules to ensure the accuracy and integrity of data. These rules can be set up to validate data against specific criteria, such as data types, ranges, or reference tables. Data that doesn't meet the defined validation rules can be flagged or rejected, preventing the propagation of inaccurate information.
Call on +91-84484 54549
Mail on [email protected]
Website: http://Anubhavtrainings.com
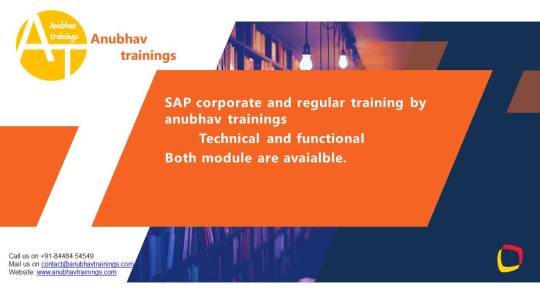
0 notes
Text
How Does CAI Differ from CDI in Informatica Cloud?
Informatica Cloud is a powerful platform that offers various integration services to help businesses manage and process data efficiently. Two of its core components—Cloud Application Integration (CAI) and Cloud Data Integration (CDI)—serve distinct but complementary purposes. While both are essential for a seamless data ecosystem, they address different integration needs. This article explores the key differences between CAI and CDI, their use cases, and how they contribute to a robust data management strategy. Informatica Training Online
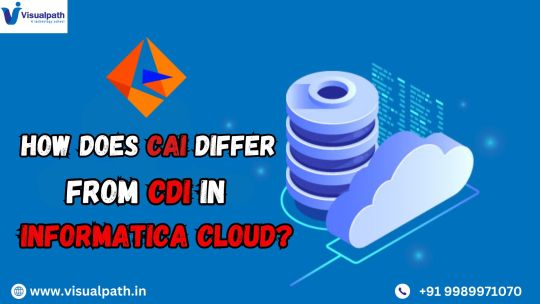
What is Cloud Application Integration (CAI)?
Cloud Application Integration (CAI) is designed to enable real-time, event-driven integration between applications. It facilitates communication between different enterprise applications, APIs, and services, ensuring seamless workflow automation and business process orchestration. CAI primarily focuses on low-latency and API-driven integration to connect diverse applications across cloud and on-premises environments.
Key Features of CAI: Informatica IICS Training
Real-Time Data Processing: Enables instant data exchange between systems without batch processing delays.
API Management: Supports REST and SOAP-based web services to facilitate API-based interactions.
Event-Driven Architecture: Triggers workflows based on system events, such as new data entries or user actions.
Process Automation: Helps in automating business processes through orchestration of multiple applications.
Low-Code Development: Provides a drag-and-drop interface to design and deploy integrations without extensive coding.
Common Use Cases of CAI:
Synchronizing customer data between CRM (Salesforce) and ERP (SAP).
Automating order processing between e-commerce platforms and inventory management systems.
Enabling chatbots and digital assistants to interact with backend databases in real time.
Creating API gateways for seamless communication between cloud and on-premises applications.
What is Cloud Data Integration (CDI)?
Cloud Data Integration (CDI), on the other hand, is focused on batch-oriented and ETL-based data integration. It enables organizations to extract, transform, and load (ETL) large volumes of data from various sources into a centralized system such as a data warehouse, data lake, or business intelligence platform.
Key Features of CDI: Informatica Cloud Training
Batch Data Processing: Handles large datasets and processes them in scheduled batches.
ETL & ELT Capabilities: Transforms and loads data efficiently using Extract-Transform-Load (ETL) or Extract-Load-Transform (ELT) approaches.
Data Quality and Governance: Ensures data integrity, cleansing, and validation before loading into the target system.
Connectivity with Multiple Data Sources: Integrates with relational databases, cloud storage, big data platforms, and enterprise applications.
Scalability and Performance Optimization: Designed to handle large-scale data operations efficiently.
Common Use Cases of CDI:
Migrating legacy data from on-premises databases to cloud-based data warehouses (e.g., Snowflake, AWS Redshift, Google BigQuery).
Consolidating customer records from multiple sources for analytics and reporting.
Performing scheduled data synchronization between transactional databases and data lakes.
Extracting insights by integrating data from IoT devices into a centralized repository.
CAI vs. CDI: Key Differences
CAI is primarily designed for real-time application connectivity and event-driven workflows, making it suitable for businesses that require instant data exchange. It focuses on API-driven interactions and process automation, ensuring seamless communication between enterprise applications. On the other hand, CDI is focused on batch-oriented data movement and transformation, enabling organizations to manage large-scale data processing efficiently.
While CAI is ideal for integrating cloud applications, automating workflows, and enabling real-time decision-making, CDI is better suited for ETL/ELT operations, data warehousing, and analytics. The choice between CAI and CDI depends on whether a business needs instant data transactions or structured data transformations for reporting and analysis. IICS Online Training
Which One Should You Use?
Use CAI when your primary need is real-time application connectivity, process automation, and API-based data exchange.
Use CDI when you require batch processing, large-scale data movement, and structured data transformation for analytics.
Use both if your organization needs a hybrid approach, where real-time data interactions (CAI) are combined with large-scale data transformations (CDI).
Conclusion
Both CAI and CDI play crucial roles in modern cloud-based integration strategies. While CAI enables seamless real-time application interactions, CDI ensures efficient data transformation and movement for analytics and reporting. Understanding their differences and choosing the right tool based on business needs can significantly improve data agility, process automation, and decision-making capabilities within an organization.
For More Information about Informatica Cloud Online Training
Contact Call/WhatsApp: +91-9989971070
Visit: https://www.visualpath.in/informatica-cloud-training-in-hyderabad.html
Visit Blog: https://visualpathblogs.com/category/informatica-cloud/
WhatsApp: https://www.whatsapp.com/catalog/919989971070/
#Informatica Training in Hyderabad#IICS Training in Hyderabad#IICS Online Training#Informatica Cloud Training#Informatica Cloud Online Training#Informatica IICS Training#Informatica Training Online#Informatica Cloud Training in Chennai#Informatica Cloud Training In Bangalore#Best Informatica Cloud Training in India#Informatica Cloud Training Institute#Informatica Cloud Training in Ameerpet
0 notes