#Self-supervised Learning Market Forecast
Explore tagged Tumblr posts
Text
#Self-supervised Learning Market#Self-supervised Learning Market Analysis#Self-supervised Learning Market Scope#Self-supervised Learning Market Growth#Self-supervised Learning Market Forecast
0 notes
Text
AI Sales Agents

In the ever-evolving landscape of technology, artificial intelligence is no longer a futuristic concept—it’s a present-day force reshaping how professionals work, communicate, and innovate. One of the most transformative developments in this field is the rise of AI agents—intelligent systems designed to operate autonomously, collaborate with humans, and complete complex tasks across industries. These agents are becoming indispensable tools for professionals, offering efficiency, accuracy, and innovation at scale.
For those looking to leverage this emerging technology in their careers, educational credentials such as the Certified Agentic AI Expert™, Certified Agentic AI Developer™, AI Course, Gen AI Course, ChatGPT Course, and Blockchain Certification are paving the way for deep understanding and practical application.
What Are AI Agents?
AI agents are software programs equipped with the ability to perceive their environment, interpret goals, plan actions, execute tasks, and learn from outcomes—all with minimal human supervision. Unlike traditional AI systems that rely on specific commands or narrow tasks, AI agents can operate in dynamic environments, solve problems proactively, and make decisions in real time.
Whether it's managing emails, summarizing documents, scheduling meetings, analyzing financial reports, or writing code, AI agents can serve as digital assistants that not only perform routine tasks but also adapt and improve over time.
Why Professionals Are Turning to AI Agents
Professionals across industries—whether in finance, marketing, law, healthcare, or tech—are increasingly embracing AI agents for several reasons:
Time-Saving Automation: AI agents handle repetitive and administrative tasks, freeing up time for strategic thinking.
Data-Driven Decision Making: Agents can analyze massive datasets, extract insights, and present actionable recommendations in seconds.
Scalability: Unlike human teams that scale with headcount, AI agents scale with code—enabling one individual to do the work of many.
24/7 Availability: AI agents don’t sleep, take breaks, or need vacation—making them ideal for global businesses that operate across time zones.
Core Capabilities of AI Agents
Natural Language Understanding:
Many agents are powered by large language models (LLMs), like OpenAI’s GPT or Google’s Gemini, allowing them to understand and generate human language fluently. This makes them ideal for tasks like drafting emails, responding to queries, and summarizing long-form content.
Multi-Step Reasoning:
Unlike basic chatbots, modern AI agents can reason through multi-step problems. For example, a legal assistant agent could review a contract, identify risk clauses, cross-reference regulatory guidelines, and draft revisions.
Tool Integration:
AI agents can be programmed to use tools like web browsers, spreadsheets, APIs, and databases. This allows them to go beyond static responses and perform actions—booking appointments, sending reports, or pulling data from external systems.
Memory and Personalization:
Some agents are equipped with memory modules that allow them to remember user preferences, prior interactions, and contextual details. This enables more personalized and efficient workflows over time.
Self-Improvement:
Through feedback and training loops, AI agents can refine their behavior, improve accuracy, and adapt to changing environments—similar to how humans learn from experience.
Real-World Applications by Role
Marketers use AI agents to generate social media content, analyze campaign performance, and automate customer segmentation.
Lawyers deploy agents to review case files, extract legal precedents, and draft initial versions of legal documents.
Doctors and medical researchers benefit from AI agents that summarize medical journals, flag anomalies in patient records, or suggest treatment options.
Financial analysts rely on AI agents to monitor markets, forecast trends, and create dynamic reports for clients.
Software developers can delegate bug tracking, documentation, and even code writing to intelligent agents.
Building and Managing AI Agents: Training & Credentials
To work effectively with AI agents—or build your own—it’s essential to acquire the right training. Here are some key credentials that provide the necessary skills:
Certified Agentic AI Expert™:
Ideal for business leaders, strategists, and consultants, this certification focuses on understanding agentic systems, ethical frameworks, AI governance, and implementation strategies across sectors.
Certified Agentic AI Developer™:
Geared toward developers and technical professionals, this program offers hands-on training in building autonomous AI systems. It includes working with Python, LLMs, APIs, and frameworks like LangChain to develop production-ready agents.
AI Course (Fundamentals to Advanced):
A general-purpose course for beginners or professionals, covering everything from machine learning basics to advanced neural networks and deployment strategies.
Gen AI Course (Generative AI):
Specializes in using AI to create content, images, text, and code. Professionals in creative, marketing, and media fields benefit enormously from these skills.
ChatGPT Course:
Focused on prompt engineering, fine-tuning, and deploying GPT-based systems for customer support, knowledge management, and task automation.
Blockchain Certification:
As AI agents increasingly interact with decentralized platforms, a blockchain certification helps professionals understand how to integrate agents with smart contracts, decentralized identity systems, and secure data layers.
Challenges and Considerations
While AI agents offer substantial benefits, they also come with challenges:
Ethical Use: It’s vital to ensure that AI agents act within legal and ethical boundaries—especially in sensitive industries like healthcare or finance.
Security: Since agents often access sensitive data, robust cybersecurity and access controls are a must.
Bias and Fairness: Developers must be vigilant about bias in training data and ensure fairness in decision-making processes.
Oversight: Professionals should treat AI agents as collaborators, not replacements. Human oversight is crucial for quality control and strategic alignment.
Final Thoughts
AI agents are transforming the professional world—not by replacing humans, but by augmenting their capabilities. As organizations look for faster, smarter, and more adaptive ways to operate, the demand for intelligent agents—and those who can build and manage them—is surging.
Whether you're a beginner exploring an AI course, enhancing your creative edge through a Gen AI course, diving deep with a ChatGPT course, or specializing through a Certified Agentic AI Expert™, Certified Agentic AI Developer™, or Blockchain Certification, there’s never been a better time to integrate AI agents into your professional journey.
The workplace of the future will be built not just by humans, but by teams of humans and AI agents working side by side. And the professionals who master this collaboration will lead the way.
0 notes
Text
Unlocking the Future of Finance with AI Crypto Price Prediction
Cryptocurrency markets have long been known for their volatility and unpredictability. Investors and traders alike are constantly seeking tools that can give them an edge. Enter AI crypto price prediction—an emerging technology that uses artificial intelligence to forecast price movements in digital assets. As AI continues to reshape industries, it's proving to be a game-changer in the world of crypto trading.

In this article, we’ll dive into how AI is applied to predict crypto prices, the technology behind it, its current limitations, and what the future may hold.
Why Predicting Crypto Prices Is So Challenging
Before we explore how AI helps with crypto predictions, it’s important to understand why forecasting prices in this market is particularly difficult. Cryptocurrency markets are influenced by a broad spectrum of factors:
Extreme volatility caused by speculative trading
Lack of regulatory uniformity
Global news and social media sentiment
Technical issues like network congestion or hard forks
Whale movements and low liquidity in certain tokens
Unlike traditional assets, cryptocurrencies don’t have earnings reports, dividends, or other financial indicators that help in valuation. This is where machine learning and AI step in—to fill the gap and analyze patterns humans can’t easily detect.
What Is AI Crypto Price Prediction?
AI crypto price prediction involves using artificial intelligence models, such as neural networks and deep learning algorithms, to analyze historical and real-time data and make forecasts about future price movements. These systems are built to learn from complex datasets and improve their performance over time.
Rather than relying on simple indicators like RSI or moving averages, AI models consider a variety of signals:
Historical price and volume data
Blockchain metrics like hash rate and wallet activity
Market sentiment from social media and news headlines
Macroeconomic indicators
Technical indicators, integrated into more advanced frameworks
Some platforms even incorporate natural language processing (NLP) to understand public mood based on tweets, Reddit threads, and news stories.
How AI Models Work
Most AI models used for predicting crypto prices fall into a few categories:
1. Supervised Learning
These models are trained using labeled datasets where the expected output (like price at time t+1) is known. They learn to predict future values based on input features like price trends, volume, and sentiment scores.
2. Unsupervised Learning
These models cluster data or detect anomalies without a predefined target. Useful for detecting outliers or significant market shifts.
3. Reinforcement Learning
A more experimental but powerful approach where an AI "agent" learns how to make profitable trades by interacting with a simulated market environment.
Tools and Platforms Using AI for Crypto
Several fintech startups and crypto analytics firms are already deploying AI crypto price prediction tools. Here are a few examples:
Santiment: Offers behavior analytics and on-chain signals driven by AI.
IntoTheBlock: Provides AI-based analysis of crypto assets including holders, transactions, and volatility.
Fetch.ai: A decentralized AI network that enables autonomous agents for trading and data sharing.
HaasOnline: Offers customizable AI bots for crypto trading.
These platforms aim to give users an analytical edge—highlighting when markets are likely to move, in which direction, and with what momentum.
Pros of AI in Crypto Trading
There are several benefits of using AI for predicting crypto prices:
Speed & Efficiency: AI models can process millions of data points in seconds, reacting faster than human traders.
Reduced Emotional Bias: AI doesn’t suffer from fear, greed, or FOMO. It sticks to data.
Scalable Analysis: AI can monitor hundreds of assets across multiple time frames simultaneously.
Self-Improving Systems: Many AI models are designed to learn from new data and improve over time.
These strengths make AI an increasingly popular tool for traders looking for reliable insights in an unpredictable market.
Pitfalls and Limitations
Despite the promise, AI crypto price prediction isn't flawless. Some of the biggest challenges include:
Overfitting: AI models trained too closely on past data might not perform well in real-world conditions.
Garbage In, Garbage Out: If the input data is poor or biased, the prediction will be too.
Black Box Nature: Many deep learning models offer little transparency, making it difficult to understand why a prediction was made.
Market Disruptions: Unexpected events—like a regulatory crackdown or exchange hack—can instantly make predictions invalid.
AI should be viewed as a support tool rather than a magic wand. It works best when combined with solid risk management and trading experience.
The Future of AI in Crypto Markets
The future of AI in the crypto space is bright and multifaceted. As blockchain and AI converge, we’re likely to see:
Decentralized AI protocols that offer prediction services on-chain
Smart contracts using AI to trigger actions based on price predictions
Hybrid AI-human investment teams, where analysts collaborate with intelligent models
Personalized trading bots tailored to individual risk profiles and goals
Regulations may also evolve to ensure transparency and accountability for AI-driven decisions, especially in financial markets.
Final Thoughts
AI is changing the way we understand and interact with crypto markets. By offering fast, data-driven insights, AI crypto price prediction tools are helping traders and investors make better-informed decisions. While they’re not perfect, their capabilities are improving rapidly.
As with any investment tool, it's important to do your own research, understand the limitations of the technology, and avoid over-relying on predictions. But one thing is clear: AI is no longer just a buzzword—it’s a vital part of the future of crypto trading.
1 note
·
View note
Text
Data Mining Techniques: Unlocking Insights from Big Data

Introduction
Data mining is a crucial process in extracting meaningful patterns and insights from large datasets. Businesses, researchers, and organizations use data mining techniques to make informed decisions, detect trends, and enhance operational efficiency. This blog explores key data mining techniques and their real-world applications.
1. Classification
Definition: Classification is a supervised learning technique used to categorize data into predefined classes or labels.
Common Algorithms:
Decision Trees
Random Forest
Support Vector Machines (SVM)
Naïve Bayes
Example: Email filtering systems use classification to distinguish between spam and legitimate emails.
2. Clustering
Definition: Clustering is an unsupervised learning technique that groups similar data points together based on shared characteristics.
Common Algorithms:
K-Means Clustering
Hierarchical Clustering
DBSCAN (Density-Based Spatial Clustering)
Example: Customer segmentation in marketing to identify different consumer groups based on buying behavior.
3. Association Rule Mining
Definition: This technique identifies relationships between variables in large datasets, often used for market basket analysis.
Common Algorithms:
Apriori Algorithm
FP-Growth (Frequent Pattern Growth)
Example: Retail stores use association rules to discover product purchase patterns, such as "Customers who buy bread often buy butter."
4. Regression Analysis
Definition: Regression is a statistical technique used to predict numerical values based on historical data.
Common Algorithms:
Linear Regression
Logistic Regression
Polynomial Regression
Example: Predicting house prices based on location, size, and other attributes.
5. Anomaly Detection
Definition: Anomaly detection identifies unusual patterns or outliers that do not conform to expected behavior.
Common Algorithms:
Isolation Forest
Local Outlier Factor (LOF)
One-Class SVM
Example: Fraud detection in banking by identifying suspicious transactions.
6. Neural Networks and Deep Learning
Definition: Advanced techniques that simulate human brain functions to analyze complex patterns in large datasets.
Common Models:
Convolutional Neural Networks (CNN)
Recurrent Neural Networks (RNN)
Artificial Neural Networks (ANN)
Example: Image recognition systems in self-driving cars and medical diagnostics.
Applications of Data Mining
Healthcare: Disease prediction and patient risk assessment.
Finance: Credit scoring and fraud detection.
Retail: Personalized recommendations and sales forecasting.
Social Media: Sentiment analysis and trend prediction.
Conclusion
Data mining techniques are essential for uncovering hidden patterns and making data-driven decisions. As businesses continue to generate massive amounts of data, leveraging these techniques can provide valuable insights, improve efficiency, and drive innovation.
Which data mining techniques have you used? Share your experiences in the comments!
0 notes
Text
AI Agent Development: Unlocking the Future of Business Automation
In the age of digital transformation, businesses are increasingly turning to artificial intelligence (AI) to streamline operations, enhance decision-making, and boost productivity. AI agents—autonomous digital assistants powered by advanced machine learning and natural language processing (NLP)—are revolutionizing the way organizations function. These AI-driven entities can perform complex tasks with minimal human intervention, making them a game-changer for business automation.
This blog explores the development of AI agents, their role in business automation, and how they are shaping the future of enterprises across various industries.
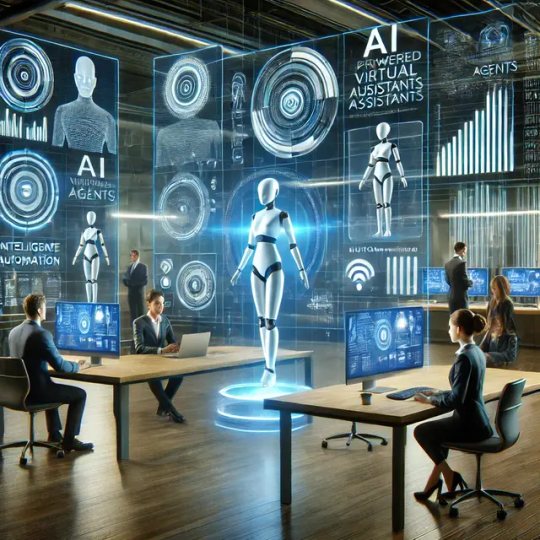
Understanding AI Agents and Their Capabilities
AI agents are software-based entities designed to perceive their environment, process information, and take actions to achieve predefined goals. They leverage artificial intelligence techniques such as deep learning, reinforcement learning, and NLP to perform tasks efficiently. Some key capabilities of AI agents include:
Automated Decision-Making: AI agents analyze vast amounts of data in real time to make informed business decisions.
Process Automation: From scheduling meetings to managing workflows, AI agents handle routine tasks seamlessly.
Conversational AI: Equipped with NLP, AI agents engage in human-like conversations, providing customer support and assisting employees.
Predictive Analytics: AI-driven insights help businesses forecast trends and optimize operations proactively.
Integration with Business Systems: AI agents integrate with enterprise software such as CRM, ERP, and HRM to enhance efficiency.
How AI Agents Are Revolutionizing Business Automation
1. Customer Service Automation
AI-powered chatbots and virtual assistants are transforming customer interactions. These agents handle inquiries, resolve issues, and provide 24/7 support, reducing the need for human intervention. Companies like Amazon and Google use AI agents to enhance customer engagement.
2. Intelligent Process Automation (IPA)
AI agents go beyond robotic process automation (RPA) by incorporating machine learning and cognitive capabilities. They automate end-to-end business processes, such as invoice processing, data entry, and compliance management, with high accuracy and minimal errors.
3. Sales and Marketing Optimization
AI agents personalize customer experiences by analyzing behavior and preferences. They recommend products, optimize ad campaigns, and generate leads, helping businesses drive revenue growth. AI-powered tools like HubSpot and Salesforce Einstein demonstrate how AI can refine sales strategies.
4. Human Resource Management
From recruitment to employee engagement, AI agents streamline HR tasks. They automate resume screening, conduct initial interviews, and even assist in performance evaluations, allowing HR teams to focus on strategic initiatives.
5. Supply Chain and Logistics Automation
AI agents enhance supply chain efficiency by predicting demand, optimizing inventory levels, and managing logistics in real-time. Companies like FedEx and DHL leverage AI-powered solutions to improve delivery accuracy and reduce operational costs.
6. Financial and Risk Management
AI agents assist in fraud detection, credit risk assessment, and financial planning by analyzing historical data and identifying patterns. Banks and financial institutions use AI to mitigate risks and enhance security measures.
The Future of AI Agent Development
1. Evolution of Autonomous AI Agents
As AI technology advances, AI agents are evolving into more autonomous and self-learning entities. Future AI agents will require minimal human supervision, continuously improving their performance through reinforcement learning.
2. Multi-Agent Systems (MAS)
Businesses will increasingly deploy multi-agent systems, where multiple AI agents collaborate to accomplish complex tasks. These systems will enhance coordination across different departments, improving overall efficiency.
3. AI Agents in the Metaverse and Web3
With the rise of the metaverse and Web3 technologies, AI agents will play a crucial role in digital interactions, virtual customer support, and decentralized finance (DeFi) applications.
4. Ethical AI and Responsible Automation
As AI adoption grows, businesses must prioritize ethical AI development, ensuring transparency, fairness, and accountability. Regulatory frameworks will evolve to address AI-related risks and challenges.
Conclusion
AI agent development is unlocking the future of business automation by transforming operations across industries. From customer service to financial management, AI agents are streamlining workflows, reducing costs, and enhancing productivity. As technology continues to advance, AI agents will become even more autonomous, intelligent, and indispensable for businesses striving to stay ahead in the digital era.
Now is the time for enterprises to embrace AI-driven automation and harness the full potential of AI agents to drive innovation and growth.
0 notes
Text
Self-supervised Learning Market Growth: A Deep Dive Into Trends and Insights
The global self-supervised learning market size is estimated to reach USD 89.68 billion by 2030, expanding at a CAGR of 35.2% from 2025 to 2030, according to a new report by Grand View Research, Inc. Self-supervised learning is a machine learning technique used prominently in Natural Language Processing (NLP), followed by computer vision and speech processing applications. Applications of self-supervised learning include paraphrasing, colorization, and speech recognition.
The COVID-19 pandemic had a positive impact on the market. More businesses adopted AI and Machine Learning as a response to the COVID-19 pandemic. Many prominent market players such as U.S.-based Amazon Web Services, Inc., Google, and Microsoft witnessed a rise in revenue during the pandemic. Moreover, accelerated digitalization also contributed to the adoption of self-supervised learning applications. For instance, in April 2020, Google Cloud, a business segment of Google, launched an Artificial Intelligence (AI) chatbot that provides critical information to fight the COVID-19 pandemic.
Many market players offer solutions for various applications such as text-to-speech and language translation & prediction. Moreover, these players are researching in self-supervised learning. For instance, U.S.-based Meta has been advancing in self-supervised learning research and has developed various algorithms and models. In February 2022, Meta announced new advances in the company’s self-supervised computer vision model SEER. The model is more powerful and is expected to enable the company in building computer vision products.
Request Free Sample PDF of Self-supervised Learning Market Size, Share & Trends Analysis Report
Self-supervised Learning Market Report Highlights
• In terms of end-use, the BFSI segment accounted for the largest revenue share of 18.3% in 2024 and is expected to retain its position over the forecast period. This can be attributed to the increasing adoption of technologies such as AI and ML in the segment. The Advertising & Media segment is anticipated to register lucrative growth over the forecast period.
• Based on technology, the natural language processing segment accounted for the dominant share in 2024 due to its ability to handle vast amounts of unstructured text data across multiple industries.. This can be attributed to the variety and penetration of NLP applications.
• North America held the largest share of 35.7% in 2024 and is expected to retain its position over the forecast period. This can be attributed to the presence of a large number of market players in the region. Moreover, the presence of specialists and developed technology infrastructure are aiding the growth of the market.
• In July 2024, Google LLC launched the Agricultural Landscape Understanding (ALU) tool in India, an AI-based platform that uses high-resolution satellite imagery and machine learning to provide detailed insights on drought preparedness, irrigation, and crop management at an individual farm level.
• In May 2024, Researchers from Meta AI, Google, INRIA, and University Paris Saclay created an automatic dataset curation technique for self-supervised learning (SSL) using embedding models and hierarchical k-means clustering. This method improves model performance by ensuring balanced datasets and reducing the costs and time associated with manual curation.
Self-supervised Learning Market Segmentation
Grand View Research has segmented the global Self-supervised Learning market based on application and region:
Self-supervised Learning End Use Outlook (Revenue, USD Million, 2018 - 2030)
• Healthcare
• BFSI
• Automotive & Transportation
• Software Development (IT)
• Advertising & Media
• Others
Self-supervised Learning Technology Outlook (Revenue, USD Million, 2018 - 2030)
• Natural Language Processing (NLP)
• Computer Vision
• Speech Processing
Self-supervised Learning Regional Outlook (Revenue, USD Million, 2018 - 2030)
• North America
o U.S.
o Canada
o Mexico
• Europe
o UK
o Germany
o France
• Asia Pacific
o China
o Japan
o India
o Australia
o South Korea
• Latin America
o Brazil
• Middle East & Africa (MEA)
o KSA
o UAE
o South Africa
List of Key Players in Self-supervised Learning Market
• Amazon Web Services, Inc.
• Apple Inc.
• Baidu, Inc.
• Dataiku
• Databricks
• DataRobot, Inc.
• IBM Corporation
• Meta
• Microsoft
• SAS Institute Inc.
• Tesla
• The MathWorks, Inc.
Order a free sample PDF of the Self-supervised Learning Market Intelligence Study, published by Grand View Research.
#Self-supervised Learning Market#Self-supervised Learning Market Analysis#Self-supervised Learning Market Report#Self-supervised Learning Market Size#Self-supervised Learning Market Share
0 notes
Text
When to use each type of machine learning algorithm
When to Use Each Type of Machine Learning Algorithm Machine learning (ML) algorithms can be broadly categorized into supervised, unsupervised, and reinforcement learning techniques.
Choosing the right algorithm depends on the type of data available and the problem you are trying to solve.
Let’s explore when to use each type of machine learning algorithm. 1. Supervised Learning Supervised learning involves labeled data, meaning the input data has corresponding output labels.
It is used when you have historical data with known outcomes and want to make predictions based on new data.
Use Cases for Supervised Learning Algorithms a. Regression Algorithms (Predicting Continuous Values)
When to use: Predicting a numeric value based on past data.
When the relationship between input features and output is continuous.
Common algorithms:
Linear Regression (e.g., predicting house prices)
Polynomial Regression (e.g., modeling non-linear trends)
Support Vector Regression (SVR) (e.g., stock price prediction)
Example Use Cases:
✔ House price prediction
✔ Sales forecasting
✔ Temperature prediction
b. Classification Algorithms (Categorizing Data) When to use: When the output falls into predefined categories (e.g., spam vs. non-spam). When you need to make decisions based on distinct classes.
Common algorithms: Logistic Regression (e.g., predicting customer churn) Decision Trees & Random Forests (e.g., diagnosing diseases) Support Vector Machines (SVM) (e.g., image classification) Neural Networks (Deep Learning) (e.g., facial recognition)
Example Use Cases:
✔ Email spam detection
✔ Fraud detection in banking
✔ Sentiment analysis of customer reviews
2. Unsupervised Learning Unsupervised learning is used when you have unlabeled data and need to find hidden patterns or structure within it.
Use Cases for Unsupervised Learning Algorithms
a. Clustering Algorithms (Grouping Similar Data) When to use:
When you need to segment or group data based on similarities.
When you don’t have predefined categories.
Common algorithms:
K-Means Clustering (e.g., customer segmentation)
Hierarchical Clustering (e.g., grouping genetic data)
DBSCAN (e.g., anomaly detection in networks)
Example Use Cases:
✔ Customer segmentation for marketing
✔ Anomaly detection in cybersecurity
✔ Identifying patterns in medical images b. Dimensionality Reduction (Feature Selection & Compression)
When to use: When you have high-dimensional data that needs simplification.
To improve model performance by reducing unnecessary features.
Common algorithms:
Principal Component Analysis (PCA) (e.g., image compression)
t-SNE (t-Distributed Stochastic Neighbor Embedding) (e.g., visualizing high-dimensional data)
Example
Use Cases:
✔ Reducing noise in data for better ML performance
✔ Visualizing complex datasets
✔ Improving computational efficiency in AI models
3. Reinforcement Learning Reinforcement learning (RL) is used when an agent learns by interacting with an environment and receiving rewards or penalties based on its actions.
Use Cases for Reinforcement Learning Algorithms
a. Decision-Making & Strategy Optimization When to use:
When the problem involves sequential decision-making.
When an AI system needs to learn through trial and error.
Common algorithms:
Q-Learning (e.g., robotics and game playing)
Deep Q Networks (DQN) (e.g., self-driving cars)
Proximal Policy Optimization (PPO) (e.g., automated trading)
Example Use Cases:
✔ Self-driving cars learning to navigate
✔ AI playing games (e.g., AlphaGo)
✔ Optimizing dynamic pricing strategies
How to Choose the Right Algorithm?
Problem Type Best Algorithm Type Example Use Case Predict a continuous value Regression (Linear, Polynomial, SVR)
House price prediction Categorize data Classification (Logistic, Decision Tree, SVM, Neural Networks)
Spam detection Find hidden patterns Clustering (K-Means, DBSCAN, Hierarchical)
Customer segmentation Reduce dataset complexity Dimensionality Reduction (PCA, t-SNE)
Feature selection in big data Optimize sequential decisions Reinforcement Learning (Q-Learning, PPO) Self-driving cars
Conclusion
Choosing the right machine learning algorithm depends on: The type of data you have (labeled vs. unlabeled) The problem you’re solving (prediction, classification, clustering, etc.)
The complexity and size of the dataset The need for interpretability and computational efficiency Understanding these factors will help you make informed decisions when selecting ML algorithms for real-world applications.
WEBSITE: https://www.ficusoft.in/deep-learning-training-in-chennai/
0 notes
Text
Data Center Accelerator Market Analysis: Meeting the Demand for Real-Time Data Processing
The global data center accelerator market size is anticipated to reach USD 63.22 billion by 2030, according to a new report by Grand View Research, Inc. The market is expected to grow at a CAGR of 24.7% from 2025 to 2030. The demand for data center accelerators is likely to grow owing to increasing adoption of technologies such as AI, IoT, & big data analytics. The COVID-19 pandemic had a positive impact on the data center accelerator market. Factors such as increased corporate awareness of the advantages that cloud services can offer, increased board pressure to provide more secure & robust IT environments, as well as the establishment of local data centers contributed to the growth of data center accelerators. Demand for businesses that rely on digital infrastructure has increased, which has led to significant growth in demand for data center network services in many industries. Data centers are now maintaining program availability and data security as more businesses and educational institutions already moved online.
Top industries using HPC are healthcare, manufacturing aerospace, urban planning, and finance. The University of Texas at Austin researchers are advancing the science of cancer treatment through the use of HPC. In a ground-breaking 2017 project, researchers examined petabytes of data to look for connections between the genomes of cancer patients and the characteristics of their tumors. This paved the way for the university to apply HPC in additional cancer research, which has now expanded to include efforts to diagnose and treat cases of prostate, blood-related, liver, and skin cancers.
Data Center Accelerator Market Report Highlights
Based on processor, the GPU segment accounted for the maximum revenue share of 44% in 2024. This can be attributed to the increasing use of GPU acceleration in IoT computing, bitcoin mining, AI and machine learning, etc. Moreover, GPU acceleration’s parallel processing architecture is useful in life science analytics such as a genome sequencing.
Based on type, the HPC data center segment is expected to grow at the highest CAGR of 26.0% over the forecast period. This can be attributed to a rising preference for hybrid and cloud-based high performance computing (HPC) solutions, use of HPC in vaccine development, advances in virtualization, etc.
Based on application, the deep learning training segment dominated the market in 2024. This can be attributed to increasing adoption of deep learning in hybrid model integration, self-supervised learning, high performance natural language process (NLP) models, and neuroscience based deep learning.
North America held the largest share of 37.0% in 2024 and is expected to retain its position over the forecast period. Presence of several data center accelerator solution and service providers makes North America a promising region for the market.
Asia Pacific is anticipated to expand at the highest CAGR of over 27.8% over the forecast period. Suitable government policies and the need for data center infrastructure upgradation in Asia Pacific are driving the growth of the data center accelerator market in the region.
In October 2020 Intel Corporation launched Intel Xeon Scalable Platform to assist secure sensitive workloads. This platform has new features that include Intel Platform Firmware Resilience (Intel PFR), Intel Total Memory Encryption (Intel TME), and new cryptographic accelerators to support the platform and advance the overall integrity and confidentiality of data.
Data Center Accelerator Market Segmentation
Grand View Research has segmented the global data center accelerator market report based on processor, type, application, and region:
Data Center Accelerator Processor Outlook (Revenue, USD Billion, 2018 - 2030)
GPU
CPU
FPGA
ASIC
Data Center Accelerator Type Outlook (Revenue, USD Billion, 2018 - 2030)
HPC Data Center
Cloud Data Center
Data Center Accelerator Application Outlook (Revenue, USD Billion, 2018 - 2030)
Deep Learning Training
Public Cloud Interface
Enterprise Interface
Data Center Accelerator Regional Outlook (Revenue, USD Billion, 2018 - 2030)
North America
US
Canada
Mexico
Europe
UK
Germany
France
Asia Pacific
China
India
Japan
Australia
South Korea
Latin America
Brazil
Middle East & Africa (MEA)
UAE
Saudi Arabia
South Africa
List of Key Players
Advanced Micro Devices, Inc.
Dell Inc.
IBM Corporation
Intel Corporation
Lattice Semiconductor
Lenovo Ltd.
Marvell Technology Inc.
Microchip Technology Inc.
Micron Technology, Inc.
NEC Corporation
NVIDIA Corporation
Qualcomm Incorporated
Synopsys Inc.
Order a free sample PDF of the Data Center Accelerator Market Intelligence Study, published by Grand View Research.
0 notes
Text
AI Coding Tutorials
The way we live and work is being redefined by artificial intelligence (AI), which is also changing industries. AI is at the core of contemporary innovation, from allowing self-driving cars to powering virtual assistants like Alexa. AI coding lessons can be your first step toward comprehending and becoming an expert in the discipline of creating intelligent systems if you're keen to explore this exciting topic.
Why Learn AI?
Skills in high demand: AI experts are in great demand in sectors like healthcare, finance, education, and entertainment.
Capabilities for problem-solving: AI gives you the tools to approach challenging issues creatively.
Investing in your career's future: Since AI is propelling technological development, learning AI skills will help you remain competitive in the work market.
Essential Prerequisites for AI Coding
Programming Languages: Because of its ease of use and extensive library, Python is the most often used language for artificial intelligence.
Mathematics: To comprehend AI algorithms, one must have a firm grasp of linear algebra, calculus, and probability.
Learn the fundamentals of supervised, unsupervised, and reinforcement learning in machine learning.
Data Handling: Working with AI models requires the ability to preprocess, clean, and visualize data.
Tips for Learning AI Coding
Begin with minor tasks.
Start with easy AI projects like building a chatbot or forecasting real estate values. Advance gradually to more challenging tasks like language translation or picture recognition.
Become a Member of AI Communities
To share your efforts and learn from others, participate in forums such as AI-focused Discord groups or Reddit's r/MachineLearning.
Take part in hackathons.
You can test your abilities and obtain practical experience by participating in AI hackathons hosted by platforms like Devpost and Kaggle.
Keep abreast
To keep up with the newest developments and trends, follow AI blogs, research papers, and industry news.
A great method to start learning about artificial intelligence is using AI coding tutorials. With the correct tools and a persistent study strategy, you can open up countless opportunities in this revolutionary field. Learning AI is a step toward influencing the future, regardless of your goals—whether they are to further your profession or develop ground-breaking inventions.
What are you waiting for, then? Join the intelligent technology revolution by beginning your AI journey right now!
To know more, click here.
0 notes
Text
What Role Does Machine Learning Play in AI Development?

Artificial Intelligence (AI) has made significant strides in recent years, thanks to innovations in machine learning (ML), which has emerged as one of the most powerful drivers behind AI’s capabilities. Machine learning, a subset of AI, involves developing algorithms that allow computers to learn from and make decisions based on data. It is one of the key components enabling AI to solve complex problems, automate tasks, and drive intelligent decision-making. But how exactly does machine learning contribute to AI development? In this blog, we will explore the vital role of machine learning in shaping the future of AI and its impact across industries.
1. Understanding Machine Learning: The Foundation of AI Development
At its core, machine learning is about creating algorithms and models that allow machines to learn from data without being explicitly programmed. In traditional programming, human engineers write detailed instructions for computers to follow. In machine learning, the machine uses historical data to identify patterns, make predictions, and improve over time without needing explicit human intervention for every task.
Key Types of Machine Learning:
Supervised Learning: The most common form of machine learning, where the algorithm is trained on labeled data (data that has input-output pairs). It "learns" the mapping between inputs and outputs, enabling it to make predictions on new, unseen data.
Unsupervised Learning: In this case, the machine learns from unlabeled data, trying to identify patterns and relationships within the data, such as clustering similar data points together.
Reinforcement Learning: This type of machine learning involves training algorithms to make decisions based on rewards or penalties. The system learns to achieve a goal through trial and error.
Semi-supervised and Self-supervised Learning: These are hybrid learning techniques where the model is trained with a mix of labeled and unlabeled data or with minimal supervision, respectively.
Machine learning plays a pivotal role in AI development by providing the foundation for systems to learn from data, improving their performance as more data becomes available.
2. Empowering AI with Data-Driven Decision Making
Machine learning enables AI systems to make smarter, more informed decisions by learning directly from vast datasets. Traditional AI systems, built on rule-based logic, rely on predefined sets of instructions. Machine learning, on the other hand, empowers AI to process large amounts of data, identify patterns, and generate insights in real time.
How Machine Learning Drives Decision Making:
Data Analysis: Machine learning algorithms can process and analyze large datasets far more efficiently than humans. By extracting valuable insights from structured or unstructured data, ML models can provide data-driven predictions, classifications, and recommendations.
Real-Time Adaptation: ML allows AI systems to adapt to new data continuously. For example, in recommendation systems (such as Netflix or Amazon), machine learning algorithms constantly learn from users’ behavior and feedback, improving recommendations over time.
Predictive Analytics: Machine learning models, particularly in supervised learning, are used for predicting outcomes based on historical data. This is vital in fields like healthcare (predicting patient conditions), finance (forecasting stock prices), and marketing (predicting customer preferences).
Impact on AI Development:
Increased Accuracy: As machine learning algorithms are exposed to more data, they refine their predictions, improving the accuracy and reliability of AI systems.
Improved Decision Making: AI can make faster, more accurate decisions by leveraging data, enabling businesses to react quickly to changes and trends in their environment.
3. Enabling Automation and Efficiency
One of the most transformative impacts of machine learning on AI development is its ability to automate tasks that would traditionally require human intervention. By leveraging ML models, businesses can optimize operations, reduce labor costs, and improve overall efficiency.
Key Areas of Automation:
Customer Support Automation: Machine learning algorithms power chatbots and virtual assistants, enabling them to handle customer queries, provide personalized responses, and resolve issues without human input. These systems learn from customer interactions, continually improving their accuracy and response quality.
Automated Content Creation: Machine learning is used in content generation tools that can produce text, images, and even videos. ML algorithms are trained on large datasets and can create high-quality, human-like content for marketing, social media, or customer communications.
Robotics and Process Automation: In manufacturing and logistics, machine learning algorithms help robots learn how to perform tasks like assembly, sorting, or packaging. By using reinforcement learning, robots improve their performance by trial and error, optimizing production lines.
Impact on Efficiency:
Cost Savings: By automating routine and repetitive tasks, machine learning reduces the need for manual labor, which leads to significant cost savings.
Faster Operations: Machines can operate 24/7, perform tasks without breaks, and process data at a much faster rate than humans, resulting in faster completion times and improved productivity.
4. Personalization at Scale
Machine learning is at the heart of personalized experiences in AI, helping businesses deliver tailored content, products, and services to customers. Personalization improves customer engagement, satisfaction, and conversion rates, making it a key element of modern AI development.
Personalization Powered by Machine Learning:
Recommendation Systems: One of the most widely used ML applications is the recommendation engine, which powers platforms like Netflix, Amazon, and Spotify. These systems analyze user behavior, preferences, and past interactions to suggest products, movies, or songs that users are likely to enjoy.
Targeted Advertising: ML models are used to create targeted advertising campaigns, predicting which ads will be most effective based on users' interests and behavior. These systems learn from past interactions and optimize ad placements, ensuring more relevant and personalized advertisements.
Customer Segmentation: By analyzing customer data, machine learning can group individuals into distinct segments, allowing businesses to deliver customized offers and messages that resonate with each group.
Impact on AI Development:
Increased Engagement: Personalization powered by machine learning results in higher user engagement, as customers feel that services and products are tailored to their needs and preferences.
Higher Conversion Rates: By targeting the right products or services at the right time, businesses can significantly boost sales and customer retention.
5. Enhancing Natural Language Processing (NLP) Capabilities
Natural Language Processing (NLP) is a field of AI that allows machines to understand, interpret, and generate human language. Machine learning plays a crucial role in advancing NLP, enabling more sophisticated and accurate language models.
Key ML Applications in NLP:
Speech Recognition: ML algorithms are used in voice assistants like Siri, Alexa, and Google Assistant to convert spoken language into text and respond to user queries. These systems continuously learn from conversations to improve their accuracy.
Text Understanding and Translation: Machine learning algorithms can process and analyze text, allowing for tasks like sentiment analysis, machine translation, and summarization. This is vital in industries like customer service, content creation, and global business communication.
Chatbots and Virtual Assistants: Using ML and NLP techniques, chatbots can simulate human-like conversations, answering questions, booking appointments, and handling customer support queries with remarkable accuracy.
Impact on AI Development:
Better Human-Machine Interaction: By improving NLP capabilities through machine learning, AI systems can engage in more natural and meaningful conversations with humans.
Multi-Language Support: Machine learning enables AI systems to understand and respond in multiple languages, which is critical for global businesses.
6. Improving AI with Deep Learning
Deep learning is a subset of machine learning that uses artificial neural networks to model and solve complex problems. It plays a pivotal role in AI development, particularly in fields like image and speech recognition, autonomous driving, and healthcare diagnostics.
Deep Learning Applications:
Image and Video Recognition: Deep learning algorithms can identify objects, faces, and scenes in images and videos. This is useful in industries such as security, retail (for inventory management), and healthcare (for medical image analysis).
Autonomous Vehicles: Deep learning models enable self-driving cars to perceive their surroundings, make decisions, and navigate roads safely.
Medical Diagnostics: Machine learning, particularly deep learning, is increasingly used in medical fields to analyze diagnostic images (e.g., X-rays, MRIs) and detect diseases like cancer at early stages.
Impact on AI Development:
High-Performance AI: Deep learning models have achieved remarkable accuracy in tasks like image and speech recognition, pushing AI systems to new levels of performance.
Breakthrough Innovations: Deep learning is unlocking new possibilities, such as autonomous systems and advanced medical technologies, that were once thought to be science fiction.
Conclusion
Machine learning is the driving force behind many of AI’s most transformative capabilities. It enables AI systems to learn from data, adapt to new situations, and solve complex problems in ways that traditional algorithms cannot. From automating tasks and enabling personalized experiences to enhancing decision-making and improving human-machine interaction, machine learning is central to the continued evolution of AI. As machine learning continues to advance, the potential applications for AI will only expand, shaping industries, creating new opportunities, and changing the way we live and work. Understanding the role of machine learning in AI development is key for businesses and developers looking to stay competitive and innovative in the future.
0 notes
Text
Data Science with AI: Revolutionizing the Future

In today’s rapidly evolving digital landscape, Data Science with AI (Artificial Intelligence) has emerged as a critical field, unlocking immense potential for businesses and industries across the globe. By leveraging vast amounts of data through intelligent systems, organizations are making better decisions, automating processes, and innovating faster than ever. In this article, we will explore how Data Science integrates with AI, the key trends, its applications in various industries, and why it is transforming the world as we know it.
What is Data Science with AI?
Data Science involves extracting meaningful insights from large datasets by using statistical methods, data processing techniques, and machine learning algorithms. AI, on the other hand, refers to machines that simulate human intelligence, including reasoning, learning, and self-correction. The combination of these two powerful fields enables machines to not only analyze data but also make decisions and predictions, driving remarkable progress in various domains.
In Data Science with AI, the role of Machine Learning (ML) is crucial, as it enables computers to learn from data without being explicitly programmed. This leads to smarter systems that improve over time, unlocking opportunities in diverse fields such as healthcare, finance, marketing, and beyond.
How Data Science with AI Works
Data Collection and Preprocessing
Data collection is the first and one of the most crucial stages in the Data Science pipeline. Without quality data, even the most advanced AI algorithms fail to deliver. Organizations gather vast amounts of structured and unstructured data from various sources such as databases, web platforms, social media, and sensors. Once collected, the data needs to be cleaned, transformed, and preprocessed to ensure accuracy and consistency.
Exploratory Data Analysis (EDA)
Before diving into advanced AI models, data scientists conduct Exploratory Data Analysis (EDA). This involves visualizing and summarizing the key patterns and relationships in the dataset. EDA is essential to understand the underlying trends, outliers, and biases in data that might affect model performance.
Model Building with Machine Learning Algorithms
Once the data is ready, AI-powered Machine Learning models are developed. These models can be supervised (trained with labeled data) or unsupervised (trained without labeled data). Commonly used algorithms include Linear Regression, Decision Trees, Random Forests, and Neural Networks. In recent years, Deep Learning — a subset of ML — has become a game-changer due to its ability to handle highly complex data.
Deep Learning models, especially Convolutional Neural Networks (CNNs) and Recurrent Neural Networks (RNNs), are widely used in image and speech recognition tasks, while Natural Language Processing (NLP) models are increasingly being employed to understand and generate human language.
Model Evaluation and Optimization
After building a model, it’s essential to evaluate its performance using metrics such as accuracy, precision, recall, and F1-score. Often, data scientists use cross-validation and hyperparameter tuning to optimize the model’s performance further. This ensures that the model generalizes well to new data and can deliver robust predictions in real-world scenarios.
Key Applications of Data Science with AI
1. Healthcare
In the healthcare industry, Data Science with AI is leading to breakthrough innovations. From drug discovery to personalized medicine, AI algorithms analyze massive amounts of clinical data to provide predictive insights, improving patient outcomes. For example, AI-powered imaging systems can detect diseases such as cancer, while predictive analytics can forecast patient deterioration in real time.
2. Finance
The finance industry is leveraging Data Science with AI to detect fraud, automate trading, and enhance risk management. Algorithms can sift through transaction data to flag suspicious activities, while Robo-advisors use machine learning to recommend investment strategies based on market conditions and individual goals.
3. Marketing and Customer Insights
Data Science with AI is transforming marketing by enabling hyper-personalized customer experiences. Businesses use AI to analyze consumer behavior, preferences, and feedback to offer tailored recommendations. Predictive analytics helps companies identify potential customers, optimize pricing strategies, and improve customer retention rates.
4. Autonomous Vehicles
Self-driving cars rely heavily on AI and Data Science. These vehicles use sensors, cameras, and complex algorithms to navigate roads, recognize objects, and make driving decisions without human intervention. By continuously learning from new data, autonomous systems are becoming safer and more reliable.
5. Manufacturing and Industry 4.0
In manufacturing, AI-driven predictive maintenance minimizes downtime by analyzing data from machinery sensors and predicting failures before they occur. Additionally, smart factories powered by AI and Internet of Things (IoT) devices are optimizing supply chains, improving product quality, and increasing operational efficiency.
6. Retail and E-commerce
Retailers are using Data Science with AI to enhance customer experiences both online and in physical stores. From recommendation engines to inventory management, AI is helping retailers understand customer preferences, forecast demand, and streamline operations, resulting in higher sales and improved customer satisfaction.
Challenges in Data Science with AI
While the opportunities in Data Science with AI are immense, there are challenges that organizations need to address. One of the main hurdles is ensuring the quality of data. Poor or biased data can lead to flawed models, producing inaccurate predictions. Additionally, ethical concerns such as data privacy and algorithmic bias need to be carefully considered, especially when AI is applied to sensitive areas like healthcare or criminal justice.
Another challenge is the black-box nature of some advanced AI models. For example, Deep Learning models can make highly accurate predictions but are often difficult to interpret. This lack of transparency can be problematic in fields that require explainability, such as law and medicine.
Future Trends in Data Science with AI
As AI continues to evolve, several trends are set to shape the future of Data Science:
1. Explainable AI (XAI)
With growing concerns about the interpretability of AI decisions, Explainable AI (XAI) is becoming a priority. XAI focuses on creating models that not only perform well but also offer insights into how they arrived at a decision. This will be particularly important in regulated industries.
2. Edge AI
The rise of Edge AI is another major trend. Rather than relying on cloud servers for AI processing, Edge AI enables devices to process data locally, offering real-time insights and reducing latency. This is especially critical for applications like autonomous vehicles, drones, and IoT devices.
3. AI in Cybersecurity
As cyber threats become more sophisticated, AI is playing a key role in detecting and responding to attacks. AI-driven cybersecurity solutions analyze patterns in network traffic to identify potential breaches and anomalies, enabling faster and more effective responses.
4. Quantum Computing and AI
The convergence of Quantum Computing and AI is expected to revolutionize fields such as cryptography, optimization, and material science. Quantum computers will be able to process complex datasets and run AI algorithms much faster than classical computers, unlocking new possibilities in AI-driven innovation.
Conclusion
Data Science with AI is undeniably a transformative force in today’s digital era. Its ability to analyze vast amounts of data, learn from it, and make intelligent decisions is reshaping industries, from healthcare to retail. As AI continues to evolve, its applications will only grow, offering unparalleled opportunities for innovation and growth. Organizations that embrace Data Science with AI will be better positioned to stay competitive in the years to come.
0 notes
Text
The Rise of AI Agents

In the ever-evolving landscape of technology, artificial intelligence is no longer a futuristic concept—it’s a present-day force reshaping how professionals work, communicate, and innovate. One of the most transformative developments in this field is the rise of AI agents—intelligent systems designed to operate autonomously, collaborate with humans, and complete complex tasks across industries. These agents are becoming indispensable tools for professionals, offering efficiency, accuracy, and innovation at scale.
For those looking to leverage this emerging technology in their careers, educational credentials such as the Certified Agentic AI Expert™, AI Course, Gen AI Course, ChatGPT Course, and Blockchain Certification are paving the way for deep understanding and practical application.
What Are AI Agents?
AI agents are software programs equipped with the ability to perceive their environment, interpret goals, plan actions, execute tasks, and learn from outcomes—all with minimal human supervision. Unlike traditional AI systems that rely on specific commands or narrow tasks, AI agents can operate in dynamic environments, solve problems proactively, and make decisions in real time.
Whether it's managing emails, summarizing documents, scheduling meetings, analyzing financial reports, or writing code, AI agents can serve as digital assistants that not only perform routine tasks but also adapt and improve over time.
Why Professionals Are Turning to AI Agents
Professionals across industries—whether in finance, marketing, law, healthcare, or tech—are increasingly embracing AI agents for several reasons:
Time-Saving Automation: AI agents handle repetitive and administrative tasks, freeing up time for strategic thinking.
Data-Driven Decision Making: Agents can analyze massive datasets, extract insights, and present actionable recommendations in seconds.
Scalability: Unlike human teams that scale with headcount, AI agents scale with code—enabling one individual to do the work of many.
24/7 Availability: AI agents don’t sleep, take breaks, or need vacation—making them ideal for global businesses that operate across time zones.
Core Capabilities of AI Agents
Natural Language Understanding: Many agents are powered by large language models (LLMs), like OpenAI’s GPT or Google’s Gemini, allowing them to understand and generate human language fluently. This makes them ideal for tasks like drafting emails, responding to queries, and summarizing long-form content.
Multi-Step Reasoning: Unlike basic chatbots, modern AI agents can reason through multi-step problems. For example, a legal assistant agent could review a contract, identify risk clauses, cross-reference regulatory guidelines, and draft revisions.
Tool Integration: AI agents can be programmed to use tools like web browsers, spreadsheets, APIs, and databases. This allows them to go beyond static responses and perform actions—booking appointments, sending reports, or pulling data from external systems.
Memory and Personalization: Some agents are equipped with memory modules that allow them to remember user preferences, prior interactions, and contextual details. This enables more personalized and efficient workflows over time.
Self-Improvement: Through feedback and training loops, AI agents can refine their behavior, improve accuracy, and adapt to changing environments—similar to how humans learn from experience.
Real-World Applications by Role
Marketers use AI agents to generate social media content, analyze campaign performance, and automate customer segmentation.
Lawyers deploy agents to review case files, extract legal precedents, and draft initial versions of legal documents.
Doctors and medical researchers benefit from AI agents that summarize medical journals, flag anomalies in patient records, or suggest treatment options.
Financial analysts rely on AI agents to monitor markets, forecast trends, and create dynamic reports for clients.
Software developers can delegate bug tracking, documentation, and even code writing to intelligent agents.
Building and Managing AI Agents: Training & Credentials
To work effectively with AI agents—or build your own—it’s essential to acquire the right training. Here are some key credentials that provide the necessary skills:
Certified Agentic AI Expert™: This certification, ideal for business leaders, strategists, and consultants, focuses on understanding agentic systems, ethical frameworks, AI governance, and implementation strategies across sectors.
Certified Agentic AI Developer™: This program, geared toward developers and technical professionals, offers hands-on training in building autonomous AI systems. It includes working with Python, LLMs, APIs, and frameworks like LangChain to develop production-ready agents.
AI Course (Fundamentals to Advanced): A general-purpose course for beginners or professionals, covering everything from machine learning basics to advanced neural networks and deployment strategies.
Gen AI Course (Generative AI): Specializes in using AI to create content, images, text, and code. Professionals in the creative, marketing, and media fields benefit enormously from these skills.
ChatGPT Course: Focused on prompt engineering, fine-tuning, and deploying GPT-based systems for customer support, knowledge management, and task automation.
Blockchain Certification: As AI agents increasingly interact with decentralized platforms, a blockchain certification helps professionals understand how to integrate agents with smart contracts, decentralized identity systems, and secure data layers.
Challenges and Considerations
While AI agents offer substantial benefits, they also come with challenges:
Ethical Use: It’s vital to ensure that AI agents act within legal and ethical boundaries—especially in sensitive industries like healthcare or finance.
Security: Since agents often access sensitive data, robust cybersecurity and access controls are a must.
Bias and Fairness: Developers must be vigilant about bias in training data and ensure fairness in decision-making processes.
Oversight: Professionals should treat AI agents as collaborators, not replacements. Human oversight is crucial for quality control and strategic alignment.
Final Thoughts
AI agents are transforming the professional world—not by replacing humans, but by augmenting their capabilities. As organizations look for faster, smarter, and more adaptive ways to operate, the demand for intelligent agents—and those who can build and manage them—is surging.
Whether you're a beginner exploring an AI course, enhancing your creative edge through a Gen AI course, diving deep with a ChatGPT course, or specializing through a Certified Agentic AI Expert™, Certified Agentic AI Developer™, or Blockchain Certification, there’s never been a better time to integrate AI agents into your professional journey.
The workplace of the future will be built not just by humans, but by teams of humans and AI agents working side by side. And the professionals who master this collaboration will lead the way.
0 notes
Text
Unlock the Power of Machine Learning with Eliora Techno's Online Course
Machine learning (ML) has transformed industries worldwide, enabling businesses to make smarter decisions by analyzing data patterns and predicting future trends. If you're looking to step into the world of artificial intelligence and advanced algorithms, Eliora Techno offers the best online machine-learning course in Nagpur, designed for both beginners and professionals.

This comprehensive course gives you hands-on experience with the latest tools and techniques in machine learning, empowering you to become an industry-ready professional. Whether you're a data scientist, software engineer, or simply interested in understanding how machines learn, this course can be your stepping stone into a booming field.
Why Choose Machine Learning?
Machine learning is one of the most sought-after skills in today's tech world. It's used in various domains, such as:
1. Healthcare – Machine learning is utilized for diagnosing diseases, predicting outcomes, and personalizing treatments.
2. Finance – In financial services, machine learning powers fraud detection, automated trading systems, and personalized financial advice.
3. Marketing – Businesses use ML to forecast customer behavior, optimize marketing strategies, and recommend products to users.
4. Automation – From self-driving cars to robotic process automation, ML is at the heart of modern innovation.
By learning machine learning, you can open doors to diverse career opportunities across multiple industries.
What Eliora Techno’s Online Machine Learning Course Offers
At Eliora Techno, we believe in offering more than just theoretical knowledge. Our online machine learning course is packed with practical, real-world applications, designed to equip you with the skills and confidence to excel in the tech industry.
Here's what you can expect from our program:
1. Comprehensive Curriculum
Our curriculum covers all the essentials of machine learning, from basic concepts to advanced algorithms. You will learn about supervised and unsupervised learning, neural networks, regression, classification, clustering, and reinforcement learning.
2. Hands-On Projects
Learning is more effective when you apply the concepts to real-world problems. Eliora Techno’s course features multiple projects where you can work with real datasets, solve practical problems, and showcase your work in your portfolio.
3. Expert Instructors
Our online machine learning course is taught by experienced professionals who have worked in the industry. They bring in-depth knowledge and offer personalized guidance to help you master the subject.
4. Flexible Learning
At Eliora Techno, we understand that flexibility is key for online learners. Our machine learning course offers a self-paced structure, allowing you to study at your own speed and balance your learning with other commitments. Whether you're a full-time student or a working professional, you can tailor the course to fit your schedule.
5. Cutting-Edge Tools
During the course, you will gain experience working with popular machine learning libraries and tools such as Python, TensorFlow, and Scikit-learn. You'll also explore cloud platforms for ML like Google Cloud and AWS.
Career Opportunities After Completion
After completing the machine learning course at Eliora Techno, you will be ready to step into a wide range of career roles, such as:
- Machine Learning Engineer
- Data Scientist
- AI Researcher
- Business Intelligence Developer
- Data Analyst
These are just a few examples of the job opportunities available in this rapidly growing field. With the right skills and certifications, you could be on your way to a high-paying and fulfilling career in machine learning.
Why Choose Eliora Techno?
When it comes to online machine learning courses in Nagpur, Eliora Techno stands out for several reasons:
- Industry-Recognized Certification: After completing the course, you will receive a certification that holds value in the job market.
- Affordable Pricing: Our goal is to make high-quality education accessible to all, which is why our machine learning course is affordably priced.
- Ongoing Support: Learning doesn't stop after the course ends. We offer continuous support through forums, mentorship, and career guidance to help you achieve long-term success.
Conclusion
Machine learning is the future, and by enrolling in the best online machine learning course in Nagpur with Eliora Techno, you are investing in your career. Whether you're a beginner looking to start your journey or a professional aiming to upskill, this course offers everything you need to excel in the world of AI and machine learning.
Don't wait to take the first step toward a rewarding career. Visit Eliora Techno today and enroll in our online machine learning course to unlock a world of opportunities!
#onlinelearningeducation#educationonlinelearning#distanceeducationonlinelearning#onlineclassesmachinelearningcourse#onlinelearningplatforms#onlineeducation#coursesonline
0 notes
Text
What types of machine learning algorithms are used in solving some popular real-world problems
Machine learning (ML) algorithms are used in various real-world applications to solve complex problems. Here are some of the popular types of machine learning algorithms and the real-world problems they address:

1. Supervised Learning Algorithms
These algorithms learn from labeled data and make predictions based on that data.
a. Linear Regression
Use Case: Predicting house prices.
Description: This algorithm predicts a continuous value. For example, given historical data on house prices, linear regression can predict the price of a new house based on its features (e.g., size, location, number of bedrooms).
b. Logistic Regression
Use Case: Spam detection in emails.
Description: This algorithm predicts a binary outcome (e.g., spam or not spam). Logistic regression is used to classify emails into spam or non-spam based on features like word frequency and sender information.
c. Decision Trees
Use Case: Customer segmentation.
Description: Decision trees split the data into subsets based on feature values, creating a tree-like model. This can be used to segment customers based on their purchasing behavior to target them with personalized marketing.
d. Support Vector Machines (SVM)
Use Case: Image classification.
Description: SVMs find the hyperplane that best separates different classes in the data. For instance, they can be used to classify images of cats and dogs based on pixel values.
2. Unsupervised Learning Algorithms
These algorithms find hidden patterns or intrinsic structures in input data without labeled responses.
a. K-Means Clustering
Use Case: Market basket analysis.
Description: K-means clustering groups similar data points together. Retailers use it to understand customer purchase behavior and group similar items together for better inventory management.
b. Principal Component Analysis (PCA)
Use Case: Facial recognition.
Description: PCA reduces the dimensionality of the data, making it easier to analyze. It’s used in facial recognition systems to identify the key features of faces for accurate identification.
3. Reinforcement Learning Algorithms
These algorithms learn by interacting with an environment and receiving feedback in the form of rewards or penalties.
a. Q-Learning
Use Case: Game playing.
Description: Q-learning is a model-free reinforcement learning algorithm. It’s used in developing AI agents that play games (e.g., AlphaGo), where the agent learns the best actions to take to maximize its score.
b. Deep Q-Networks (DQN)
Use Case: Autonomous driving.
Description: DQNs combine Q-learning with deep neural networks. They are used in self-driving cars to navigate and make decisions in complex environments.
4. Neural Networks and Deep Learning
These algorithms are inspired by the structure and function of the human brain.
a. Convolutional Neural Networks (CNNs)
Use Case: Medical image analysis.
Description: CNNs are designed for processing structured grid data like images. They are used in medical diagnostics to analyze X-rays, MRI scans, and other medical images for detecting diseases.
b. Recurrent Neural Networks (RNNs)
Use Case: Language translation.
Description: RNNs are used for sequential data. They are employed in natural language processing tasks such as translating text from one language to another (e.g., Google Translate).
5. Ensemble Learning Algorithms
These algorithms combine multiple models to improve performance.
a. Random Forest
Use Case: Fraud detection.
Description: Random forests combine multiple decision trees to improve accuracy. They are used in banking and finance to detect fraudulent transactions based on transaction patterns.
b. Gradient Boosting Machines (GBMs)
Use Case: Predictive maintenance.
Description: GBMs build models sequentially to correct errors made by previous models. They are used in predictive maintenance to forecast equipment failures and schedule timely maintenance.
Conclusion
Machine learning algorithms are crucial in solving a wide range of real-world problems, from predicting prices and detecting fraud to diagnosing diseases and enabling self-driving cars. The choice of algorithm depends on the nature of the problem, the type of data available, and the specific requirements of the application.
0 notes
Text
From Beginner to Badass: Your Roadmap to Becoming an Aspiring Data Scientist
In today's digital era, the role of a data scientist is not just pivotal but indispensable across industries. Aspiring data scientists embark on a transformative journey that melds technical acumen, continuous learning, and a fervent dedication to solving complex challenges. Looking for top-notch Data Science Training in Pune Discover comprehensive courses tailored to industry demands.
Let's explore what it truly means to aspire to become a data scientist.
Cultivating Essential Skills
Becoming a proficient data scientist hinges on mastering a spectrum of crucial skills:
Technical Mastery: Proficiency in programming languages such as Python, R, and SQL forms the bedrock for manipulating and analyzing vast datasets. Data scientists harness these languages to clean, organize, and derive actionable insights from data, essential for building scalable solutions and driving informed decision-making.
Statistical Insight: A deep understanding of statistical methods is vital for interpreting data and extracting meaningful patterns. Data scientists apply statistical techniques to validate hypotheses, conduct rigorous analyses, and ensure the reliability of their findings, pivotal for guiding strategic business decisions.
Advanced Machine Learning: Expertise in machine learning algorithms empowers data scientists to develop predictive models and AI applications that uncover trends, forecast outcomes, and optimize processes. From supervised to unsupervised learning, mastering these techniques enables data scientists to transform raw data into predictive insights that drive innovation.
Commitment to Lifelong Learning
The journey towards becoming a data scientist is characterized by a relentless pursuit of knowledge and skills:
Formal Education and Specialized Training: Pursuing degrees in data science or related disciplines provides a foundational understanding of data analysis principles and methodologies. Additionally, undertaking specialized courses, bootcamps, and certifications equips aspiring data scientists with cutting-edge tools and techniques essential for staying ahead in this rapidly evolving field.
Self-Directed Exploration: Engaging in self-study through research papers, online resources, and practical experimentation fosters creativity and innovation. Exploring new frameworks, methodologies, and emerging technologies not only broadens expertise but also cultivates adaptive problem-solving skills crucial for addressing complex challenges in data science. Explore the depths of data analytics with a premier Data Science Course Online, accessible from anywhere, anytime.
Problem-Solving Focus
Data scientists excel in translating data into actionable insights that drive impactful decisions:
Insightful Data Analysis: Leveraging exploratory data analysis (EDA) and data visualization techniques, data scientists uncover hidden insights within intricate datasets. By applying machine learning algorithms and statistical models, they distill complex data into clear, actionable insights that inform strategic initiatives and enhance operational efficiencies.
Strategic Decision Support: Empowering organizations to make informed decisions based on data-driven evidence is at the core of a data scientist's role. Whether optimizing marketing strategies, predicting consumer behavior, or enhancing product development, data-driven insights enable organizations to achieve measurable outcomes and gain competitive advantages.
Application Across Diverse Industries
Aspiring data scientists seek to make a tangible impact across diverse sectors:
Industry Adaptability: Pursuing opportunities in healthcare, finance, e-commerce, and beyond, where data science can revolutionize processes and outcomes. By applying their expertise to industry-specific challenges, data scientists drive innovation in personalized healthcare, financial forecasting, customer segmentation, and more.
Value Creation: Harnessing the power of data to streamline operations, improve decision-making, and drive innovation fuels organizational success. Whether developing predictive models for risk management or deploying AI-driven solutions for operational efficiency, data scientists play a pivotal role in translating data into strategic initiatives that deliver measurable value.
Collaboration and Professional Growth
Beyond technical prowess, aspiring data scientists thrive in collaborative environments:
Networking and Mentorship: Engaging with peers, mentors, and industry leaders through networking events, forums, and online communities fosters knowledge exchange and professional growth. Building strong relationships within the data science community provides insights into emerging trends, career opportunities, and best practices.
Practical Experience: Participating in collaborative projects, hackathons, and open-source initiatives cultivates hands-on experience and teamwork skills. Contributing to real-world challenges not only enhances technical proficiency but also nurtures creativity and fosters interdisciplinary collaborations essential for advancing in the field of data science.
Becoming an aspiring data scientist is a journey defined by curiosity, perseverance, and a passion for leveraging data to drive meaningful change. Whether you're embarking on this path or advancing in your career, embracing continuous learning, problem-solving, and collaboration sets the foundation for success in the dynamic field of data science.
#data science course#datascience#data science certification#data science training#tech#technology#education
0 notes
Text
The global self-supervised learning market is projected to have a moderate-paced CAGR of 33.4% over the forecast period. The current valuation of the self-supervised learning market is US$ 12.46 billion in 2023. The value of the self-supervised learning market is anticipated to reach a high of US$ 222.31 billion by the year 2033.
Self-reinforcement learning has emerged as a viable machine learning technique to address the challenges brought on by an overreliance on labelled data. For a very long time, creating intelligent systems using machine learning techniques has required the availability of high-quality tagged data. Because of this, it will be difficult to overcome the high cost of high-quality annotations during the training process.
0 notes