#biometric spoofing and deepfake detection
Explore tagged Tumblr posts
Text
#spoof detection#fake fingerprint detection#biometric security#biometric solutions#optical fingerprint scanner#optical scanner#capacitive fingerprint sensor#capacitive fingerprint scanner#capacitive scanner#biometric spoofing#fingerprint spoofing#anti-spoofing technology#what is spoof detection#spoofing biometrics#anti spoofing technology#spoofing detection#what is spoof#fingerprint spoof#spoof proof#spoof identity#biometric spoofing and deepfake detection#spoof fingerprint
0 notes
Text
Face Liveness Detection SDK for Spoof-Proof Security
How a Face Liveness Detection SDK Beats Spoof Attacks
In a world where unlocking a phone with your face feels like sci-fi, the reality behind that magic is far more complex and vulnerable. Face authentication is no longer just about recognizing a face. It’s about knowing whether that face is real, live, and in front of the camera. This is where a Face Liveness Detection SDK steps into the spotlight, acting like a bouncer at the digital door letting in the real deal and kicking out imposters.
Let’s explore what it is, why it’s important, and how it helps protect both your users and your reputation.
What Is a Face Liveness Detection SDK?
At its core, a face liveness detection SDK is a software development kit that developers can embed into mobile apps, websites, or biometric systems to detect whether a face presented to the camera is a real, live human or a spoof (like a photo, mask, or video replay). It’s not just looking for a face it’s watching for signs of life.
Key Features
Here’s what most high-quality liveness detection SDKs offer:
Passive liveness detection (no blinking or head-turning needed)
Anti-spoofing against photos, videos, deepfakes, and masks
Real-time verification within seconds
Compatibility with iOS, Android, and web platforms
Compliance with global data privacy standards (like GDPR & CCPA)
This SDK works silently and swiftly in the background no cheesy prompts or awkward user interactions are required. The goal? Seamless security.
Why Is Liveness Detection Crucial in Modern Face Authentication?
Passwords are passé. Face authentication is now the go-to for many industries, from fintech to healthcare. But where there's convenience, there's also risk. A Face Liveness Detection SDK prevents spoof attacks, which are surprisingly easy without the right tech in place.
Real-World Risks
Consider these scenarios:
Photo attacks: A printed photo or digital image fools basic facial recognition.
Video replay: Someone plays a video of the real user to unlock a system.
3D mask spoofing: Hyper-realistic masks impersonate a user’s facial structure.
In 2022, the iProov Biometric Threat Intelligence Report found that presentation attacks increased by over 300% compared to the previous year.
How a Face Liveness Detection SDK Works (Without Giving Hackers a Cheat Sheet)
Without going too far into the weeds (or tipping off the bad guys), here’s a peek into how these SDKs spot a real face versus a fake:
1. Texture and Light Analysis
Live skin has dynamic textures and reflects light differently than paper, screens, or silicone masks.
2. Micro-Movement Detection
Real faces have involuntary muscle twitches and eye micro-movements. Fakes? Not so much.
3. Depth Mapping
Using 2D or 3D sensors, the SDK checks for depth cues. Flat images just don’t cut it.
4. AI-Powered Behavior Tracking
Machine learning models look for inconsistencies in facial expressions, blinking, and head positioning.
Top Use Cases Across Industries
Face liveness detection isn’t just a cool trick it’s rapidly becoming a regulatory requirement and a user expectation, particularly in security-conscious industries. Here’s how it’s being utilized across various sectors:
Banking: Remote account opening. Face liveness detection helps prevent identity fraud by verifying the user’s physical presence.
Healthcare: Telehealth logins. It secures access to patient records, ensuring only authorized users can log in.
E-commerce: KYC (Know Your Customer) during high-value purchases. This helps reduce fraudulent transactions, protecting both customers and merchants.
Education: Online exam proctoring. Face liveness detection ensures the presence of test-takers, maintaining the integrity of the exam process.
Government: ePassport verification. It strengthens border control by confirming the identity of travelers.
Spoiler alert: This isn’t just for big players. Even small apps can (and should) use a lightweight Face Liveness Detection SDK to boost trust and protect their users.
What to Look for in a Face Liveness Detection SDK
Not all SDKs are created equal. If you're evaluating options, here’s a checklist to keep handy:
Accuracy & Speed
Low false positives, fast responses. Users shouldn’t wait 10 seconds to be verified.
Passive Liveness
No weird prompts like “turn your head” or “blink twice.” Less friction = better UX.
Spoof Detection Versatility
Should block everything from printed photos to high-res video replays and 3D masks.
On-Device or Cloud Processing
Choose based on your app’s needs: on-device for privacy, cloud for scalability.
SDK Size & Performance
No bloatware, please. Look for a lean, well-documented SDK that won’t slow down your app.
Compliance & Ethics
Ensure the vendor follows ethical AI practices and is transparent about how data is used.
Case Study: How Fintech Apps Are Reducing Fraud with Liveness Detection
A mid-size mobile banking app in Southeast Asia integrated a liveness detection SDK after experiencing a spike in fraudulent account registrations. Within six months:
Fraudulent attempts dropped by 78%
Customer support tickets related to login issues fell by 42%
User trust and app ratings improved by 1.3 stars on average
This wasn’t magic, it was a well-placed layer of invisible security.
Conclusion: Liveness Detection Isn't Optional Anymore
Face recognition is powerful but without liveness detection, it’s like locking your front door and leaving the window wide open. A face liveness detection SDK offers that missing layer of real-world, real-time protection that separates the serious from the spoofed.And if you’re serious about secure, seamless facial authentication, it’s time to start building smarter. Recognito is here to help you do just that.
1 note
·
View note
Text
What Are the Latest Advancements in Biometric Authentication for Fintech?
Biometric authentication is revolutionizing the Fintech Industry, providing secure, efficient, and seamless user experiences. With financial transactions becoming increasingly digital, the demand for advanced security measures has surged. Traditional password-based authentication methods are being replaced by biometric solutions that offer higher accuracy, convenience, and fraud prevention. This article explores the latest fintech technologies in biometric authentication and their impact on the financial sector.
1. Facial Recognition for Seamless Authentication
Facial recognition technology has evolved significantly in recent years, becoming a mainstream method of authentication in fintech applications. Enhanced by artificial intelligence (AI) and machine learning, facial recognition systems now offer:
Liveness detection to prevent spoofing attempts with photos or deepfake videos.
Infrared scanning to detect real human presence, even in low-light conditions.
AI-driven adaptive learning, which improves recognition accuracy over time.
Many banking apps and payment platforms have integrated facial recognition as a primary authentication method, making transactions faster and more secure.
2. Fingerprint Scanning with Advanced Sensors
Fingerprint recognition remains one of the most widely used biometric authentication methods in the Fintech Industry. The latest advancements in fingerprint scanning include:
Ultrasonic fingerprint sensors, which create 3D maps of fingerprints for greater accuracy.
In-display fingerprint scanning, integrated into smartphones and smart cards.
Multi-fingerprint authentication, requiring multiple fingerprints for added security.
These innovations are particularly useful for mobile banking, digital wallets, and contactless payments, enhancing user convenience and security.
3. Voice Recognition for Secure Transactions
Voice biometrics is emerging as a powerful authentication tool in fintech. The latest advancements include:
AI-driven voiceprint analysis, which recognizes unique vocal characteristics.
Continuous authentication, which monitors voice patterns throughout a conversation for additional security.
Multi-accent and multilingual support, ensuring global accessibility.
Voice recognition is being widely adopted in call centers, mobile banking apps, and virtual assistants, reducing reliance on PINs and passwords.
4. Iris and Retina Scanning for High-Security Authentication
Iris and retina scanning offer some of the most secure biometric authentication methods available today. Financial institutions and high-security environments are increasingly adopting these technologies due to their:
Extreme accuracy, as iris and retina patterns are unique and nearly impossible to replicate.
Contactless verification, making them ideal for hygienic authentication in public spaces.
AI-enhanced matching, improving recognition even with variations in lighting or eye movement.
Banks and payment service providers are integrating iris scanning into ATMs and mobile banking platforms for ultra-secure authentication.
5. Behavioral Biometrics for Continuous Authentication
Behavioral biometrics is a cutting-edge approach that analyzes user behavior to authenticate transactions. It includes:
Typing patterns, detecting unique keystroke dynamics.
Mouse movement and touch gestures, identifying individual usage habits.
Gait recognition, analyzing the way users walk.
Unlike traditional biometric authentication, behavioral biometrics provide continuous security, detecting anomalies in real-time to prevent fraud and unauthorized access.
6. Biometric Payment Cards for Secure Transactions
The introduction of biometric payment cards is one of the latest fintech technologies enhancing financial security. These cards integrate fingerprint scanners directly into credit or debit cards, allowing users to authenticate transactions with their fingerprint instead of a PIN. Benefits include:
Increased security, as fingerprints cannot be easily stolen or replicated.
Faster checkout process, eliminating the need for PIN entry.
Enhanced user convenience, reducing reliance on physical authentication devices.
Major financial institutions and fintech companies are rapidly adopting biometric payment cards to combat fraud and enhance customer experience.
7. Biometric Authentication in Blockchain and Decentralized Finance (DeFi)
With the rise of blockchain and decentralized finance, biometric authentication is being integrated into digital wallets and smart contracts. Key advancements include:
Biometric encryption, securing private keys with fingerprint or facial recognition.
Multi-factor authentication (MFA) with biometrics, combining traditional and biometric security measures.
Blockchain-based identity verification, allowing secure and decentralized user authentication.
These innovations enhance the security of cryptocurrency transactions and digital asset management, making them more accessible and user-friendly.
8. AI and Machine Learning Enhancements in Biometric Security
Artificial intelligence and machine learning are playing a crucial role in improving biometric authentication. Advancements include:
Adaptive learning algorithms, which refine biometric matching accuracy over time.
Deepfake detection, preventing fraudulent attempts using synthetic media.
Fraud prevention analytics, identifying suspicious behavior before unauthorized transactions occur.
AI-driven biometric authentication is helping financial institutions stay ahead of cyber threats while ensuring a seamless customer experience.
9. Xettle Technologies and the Future of Biometric Fintech Security
A leading player in the Fintech Industry, Xettle Technologies is at the forefront of biometric authentication innovations. Their advanced solutions integrate AI-powered facial recognition, fingerprint encryption, and blockchain security to provide a comprehensive and secure financial ecosystem. By continuously evolving their biometric technologies, Xettle Technologies is setting new standards in fintech security.
Conclusion
The latest fintech technologies in biometric authentication are transforming the financial sector by enhancing security, convenience, and user experience. Innovations in facial recognition, fingerprint scanning, voice recognition, and behavioral biometrics are redefining how financial institutions protect customer data and prevent fraud. With companies like Xettle Technologies leading the charge, the future of biometric authentication in fintech promises greater security and seamless financial interactions. As these technologies continue to advance, they will play a crucial role in shaping the next generation of digital banking and financial services.
0 notes
Text
Face Image Datasets: Advancing AI in Facial Recognition and Analysis
Facial recognition technology has evolved rapidly, transforming industries such as security, healthcare, and personalized marketing. At the core of this transformation lies face image datasets, the essential building blocks that enable artificial intelligence (AI) to learn, recognize, and analyze human faces with remarkable accuracy.
High-quality face datasets help train AI models to detect identities, recognize emotions, and even assess age or gender. In this article, we explore how face image datasets are shaping the future of AI-driven facial recognition and analysis.
The Role of Face Image Datasets in AI Development
AI-powered facial recognition systems rely on extensive datasets of human faces to function effectively. These datasets serve multiple purposes, including:
Training AI Models – Machine learning algorithms need vast amounts of labeled facial images to recognize patterns in human faces.
Improving Accuracy – A diverse dataset helps minimize errors and biases, ensuring better recognition across different demographics.
Advancing Security Systems – Facial recognition is used in surveillance, fraud detection, and access control systems.
Enhancing User Experience – AI-driven personalization, such as facial filters in social media apps, depends on high-quality image datasets.
Without well-structured face image datasets, AI systems would struggle with misidentifications, leading to inaccurate results and potential security risks.
Sourcing and Preparing Face Image Datasets
1. Collecting Facial Data
Face image datasets can be sourced from:
Public datasets released by research institutions.
Crowdsourced contributions, where volunteers submit images.
Web scraping, although it raises ethical concerns.
2. Data Annotation and Labeling
For AI to understand facial features, datasets need proper annotation, including:
Bounding boxes to define facial regions.
Landmark detection to identify key facial points (e.g., eyes, nose, mouth).
Expression labeling to categorize emotions.
3. Data Augmentation
To increase dataset diversity and prevent overfitting, AI developers use data augmentation techniques, such as:
Rotating and flipping images to simulate different angles.
Adjusting brightness and contrast to handle lighting variations.
Adding artificial noise to make models more robust.
By preparing and augmenting data properly, AI models achieve higher accuracy and reliability.
Challenges in Face Image Datasets
While facial recognition technology offers incredible potential, working with face image datasets comes with significant challenges:
1. Bias in Facial Recognition
AI models trained on biased datasets may struggle with accurate recognition across different demographics. Studies have shown that some facial recognition systems perform poorly on people of color due to insufficient diversity in training data.
Solution: Ensuring datasets include ethnically and geographically diverse images helps reduce bias and improve accuracy.
2. Privacy and Ethical Concerns
Facial recognition technology raises privacy issues, especially when data is collected without consent.
Solution: Adhering to strict data protection regulations, such as GDPR and CCPA, and obtaining explicit user consent for data collection.
3. Deepfake and Security Risks
Face datasets can be misused to create deepfake videos, leading to misinformation and fraud.
Solution: Developing AI models capable of detecting synthetic images and implementing anti-spoofing techniques in recognition systems.
Addressing these challenges ensures ethical and responsible use of AI in facial recognition.
Real-World Applications of Face Image Datasets
1. Security and Authentication
Face image datasets power biometric authentication systems, such as:
Facial unlock features on smartphones.
Airport security checks using face scanning.
Fraud detection in banking and financial transactions.
2. Healthcare and Wellness
AI-driven facial analysis is used in:
Medical diagnostics, detecting conditions like Parkinson’s disease.
Mental health assessments, analyzing facial expressions for emotional well-being.
3. Retail and Personalized Marketing
Retailers use facial recognition to:
Analyze customer demographics for targeted advertising.
Enhance in-store experiences by identifying returning customers.
The ability to process and analyze facial images is transforming multiple industries.
The Future of Face Image Datasets in AI
The next phase of AI in facial recognition will focus on:
Bias-free datasets for fair and inclusive AI.
Real-time face analysis for security and behavioral insights.
AI-powered emotion recognition to improve human-computer interaction.
As technology evolves, ensuring data privacy, ethical AI use, and unbiased datasets will be critical for shaping a responsible future for facial recognition.
Conclusion
Face image datasets are the driving force behind AI-powered facial recognition and analysis. From security applications to healthcare innovations, the ability to accurately detect and analyze faces is revolutionizing industries.
However, ethical concerns surrounding privacy, bias, and security risks must be addressed to ensure AI is fair, accurate, and responsibly developed. With continuous advancements in data collection, annotation, and deep learning, face image datasets will continue to push the boundaries of intelligent AI systems.
Visit Globose Technology Solutions to see how the team can speed up your facial recognition projects.
0 notes
Text

Secure Your Space with AI-Powered Biometrics!
Star Link’s Bio-Face Reader combines advanced AI, 3D facial recognition, and anti-spoofing tech for top-tier security. From detecting deepfakes to real-time data updates, this device is built for modern attendance management and access control. Plus, its night vision ensures 24/7 reliability.
Upgrade your security game today!
0 notes
Text
Face Anti-Spoofing Datasets in Biometric Security
As facial recognition technology continues to evolve and integrate into various facets of our digital lives, it faces persistent challenges from sophisticated spoofing attacks. These attacks aim to deceive biometric systems using fake facial data, ranging from simple photographs to advanced deep fakes. To counteract these threats, the development and utilization of robust face anti-spoofing datasets are crucial. In this blog, we will explore the role of these datasets in strengthening biometric security, their current state, and the future directions for enhancing face anti-spoofing technologies.
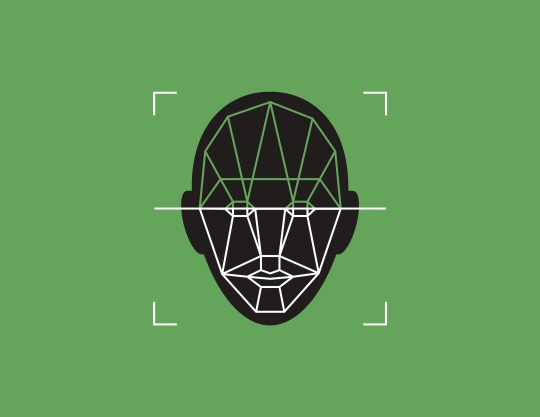
Understanding Face Spoofing Attacks
Face spoofing, or presentation attacks, involves presenting fake facial representations to bypass biometric systems. Common methods include:
Static 2D Attacks: Using photos or printed images of faces to trick systems.
Static 3D Attacks: Employing 3D-printed masks or models to mimic a person’s face.
Dynamic Attacks: Leveraging videos or deepfakes to simulate real-time facial movements.
As these methods become increasingly sophisticated, they pose a significant risk to the integrity of facial recognition systems.
The Role of Anti-Spoofing Datasets
Anti-spoofing datasets are crucial in developing and training systems to detect and mitigate spoofing attempts. These datasets typically include a diverse range of facial images and videos, encompassing both genuine and spoofed samples. The effectiveness of anti-spoofing technologies heavily relies on the quality and variety of these datasets. Here’s why they matter:
Diversity and Representation: A well-rounded dataset must represent various demographic groups, lighting conditions, and spoofing techniques to ensure that anti-spoofing systems perform reliably across different scenarios.
Training Robust Models: Machine learning models used for detecting spoofing attempts are trained on these datasets. The more comprehensive the dataset, the better the model can generalize and accurately identify spoofing attempts.
Benchmarking and Evaluation: Datasets provide a standard for evaluating the performance of anti-spoofing technologies. They help in benchmarking different systems and identifying areas for improvement.
Key Face Anti-Spoofing Datasets
Several datasets have been established to support the development of anti-spoofing technologies. Notable examples include:
CASIA-FASD: Developed by the Chinese Academy of Sciences, this dataset includes a wide range of face images captured under various conditions, providing a solid foundation for training anti-spoofing models.
REPLAY-ATTACK: This dataset features videos of subjects presenting both genuine and spoofed faces, collected under different lighting conditions and using various spoofing techniques.
MSU Mobile Face Spoofing: Designed for mobile device applications, this dataset includes both still images and videos of spoofing attacks, tailored to scenarios involving mobile biometric systems.
FaceForensics++: Focused on deepfake detection, this dataset provides a large collection of manipulated video footage, offering valuable resources for training models to identify and counteract deepfake attacks.
Characteristics of Good Training Dataset
1. Diversity and Representativeness: Wide range of facial variations, demographics, ethnicities, age groups, genders, skin tones, and facial expressions to avoid bias and improve generalization.
2. Quality and Consistency: High-quality images with consistent resolutions, clear facial features, and minimal noise, ensuring the model learns relevant features without being influenced by noise or variations.
3. Balanced and Adequate Volume: Well-balanced dataset with sufficient samples for each class or category, ensuring the model receives adequate exposure to all facial variations and avoiding biased models.
4. Annotation and Labeling: Accurate and detailed annotations of facial attributes, including key facial landmarks, expressions, gestures, and emotions, enabling the model to learn distinct facial features and variations.
5. Ethical and Privacy Compliance: Compliance with ethical guidelines and privacy regulations, respecting individuals' privacy rights, obtaining necessary consent, and ensuring anonymization of personal information.
6. Realistic and Varied Scenarios: Datasets reflecting real-world scenarios, including different environments, camera angles, occlusions, and facial occlusions, enhancing the model's adaptability to various conditions and scenarios.
7. Preprocessing and Standardization: Preprocessing techniques ensure uniformity and consistency in the dataset, optimizing data for effective model training, and reducing noise and irrelevant variations.
8. Adaptability and Generalization: The dataset allows the model to adapt and generalize well to unseen or new facial variations, performing better in real-world applications and diverse user groups.
9. Continuous Updates and Maintenance: Regular updates and maintenance of the dataset, keeping the model current with evolving facial characteristics, trends, and technology changes.
Current Challenges and Future Directions
Despite the advancements in face anti-spoofing datasets, several challenges remain:
Evolving Spoofing Techniques: As spoofing methods become more sophisticated, datasets must be continuously updated to include new types of attacks and countermeasures.
Quality vs. Quantity: Balancing the quality and diversity of dataset samples is crucial. While larger datasets can provide more coverage, they must also maintain high standards of data quality.
Real-World Application: Ensuring that datasets reflect real-world scenarios is essential for developing practical anti-spoofing solutions. This includes considering variations in lighting, camera angles, and user behavior.
Conclusion
Face anti-spoofing datasets play a pivotal role in enhancing the security and reliability of facial recognition systems. By providing a foundation for training and evaluating anti-spoofing technologies, these datasets help in developing robust solutions to combat evolving spoofing techniques. As the field progresses, ongoing efforts to improve and expand these datasets will be essential in staying ahead of fraudulent activities and ensuring the integrity of biometric security systems. At TagX, we're committed to supporting the advancement of biometric security through our innovative data solutions. Stay tuned to our blog for more insights into biometric security and the latest advancements in anti-spoofing technologies, and discover how TagX can help you unlock the full potential of your facial recognition systems.
Visit Us, https://www.tagxdata.com/
Original Source, https://www.tagxdata.com/face-anti-spoofing-datasets-in-biometric-security
0 notes
Text
Briefly respond to all the following questions
Briefly respond to all the following questions
Emerging Technologies for Digital Forensics: Security by Blockchain Technology Text based Mining in Digital Evidences Deleted Data Recovery Mechanism Comprehensive Study of Darknet Evidence based Reconstruction Biometric Spoofing Deepfake Detection Implementation of Artificial intelligence Forensics Analysis on Streaming Multimedia IoT and Underwater Networks Forensics Choose 2 or 3 three items…
View On WordPress
0 notes
Text
Singapore adds face verification, multi-user SMS to SingPass 2FA
Data protection privacy concept. GDPR. EU. Cyber security network. Business man protecting data personal information on tablet. Padlock icon and internet technology networking connection on digital dark blue background.Data protection privacy concept. GDPR. EU. Cyber security network. Business man protecting data personal information on tablet. Padlock icon and internet technology networking connection on digital dark blue background.Singapore has added face verification as a two-factor authentication (2FA) option to log into SingPass, an account residents use to access e-government services. They also can choose to send their SMS one-time password (OTP) to another SingPass user's mobile number, which is offered to help less digitally savvy users navigate the platform with external assistance. The two additional 2FA options were introduced as part of the government's efforts to support a digitally inclusive society, said Government Technology Agency of Singapore (GovTech) in a statement Wednesday. The government agency is responsible for the country's ICT and smart nation rollouts,SingPass users will be able to log into their account first by entering their ID and password, then by scanning their face on an internet-connected computer equipped with a webcam or a mobile device with a front-facing camera. If they do not have access to any of these systems, they can visit selected public locations equipped with the service, including IRAS Taxpayer and Business Service Centre and CPFB's Bishan Service Centre, autel al619 review. with more locations to be added progressively.GovTech said the face verification technology was integrated with security features to safeguard against fraud, such as liveness detection capabilities to detect and block the use of photographs, videos, or masks during the verification process.The added option not only would be useful to support less digitally savvy users who would not need to key in additional information such as OTPs, but also could facilitate Singaporeans living abroad and might not have a locally-registered number to receive SMS OTPs, said National Digital Identity's senior director Kwok Quek Sin. The need to better assist the less digitally savvy also led to the inclusion of "multi-user SMS OTP", where SingPass users can link their account to another user's mobile number such as their child to receive their OTPs. The addition of the two 2FA options follows plans to discontinue the OneKey token by the end of March next year. Some 120,000 users of the physical 2FA device, introduced in 2013, currently were being transitioned to the other options, said GovTech. There are four million SingPass users here who can tap their account to access 500 digital services provided by more than 180 government agencies as well as commercial entities, such as banks. Singapore's Immigration & Checkpoints Authority (ICA) in October said it had been rolling out iris and facial scanners since July at all automated and manual immigration points located at the passenger halls of Singapore's land, sea, and air checkpoints. These included Changi Airport Terminal 4, Tanah Merah Ferry Terminal, and the Tuas and Woodlands checkpoints that border Northern neighbour Malaysia. The systems would use travellers' iris and facial data, replacing fingerprints, as the primary biometric identifiers for immigration clearance. Singapore in September inked a deal with British vendor iProov to provide face verification technology for use in the country's national digital identity system. The security feature was launched as a pilot earlier this year, allowing SingPass users to access e-government services via biometric.iProov's Genuine Presence Assurance technology is touted to have the ability to determine if an individual's face is an actual person, and not a photograph, mask or digital spoof, and authenticate that it is not a deepfake or injected video. Its agreement with the Singapore government also marked the first time the vendor's cloud facial verification technology was used to secure a country's national digital id...
0 notes
Text
Deep Truths of Deepfakes — Tech That Can Fool Anyone
In its most basic sense, a deepfake is a combination of face- and voice-cloning AI technologies that allow for the creation of life-like, computer-generated videos of a real person.
In order to develop a high-quality deepfake of an individual, developers need to accumulate tens of hours of video footage associated with the person whose face/voice is to be cloned, as well as a human imitator who has learned the facial mannerisms and voice of the target.
There are two humans involved in the creation of a deepfake, such that the target face/voice is that of the famous person while the other belongs to an unknown individual who is generally closely associated with the project.
From tech to reality
From a technical standpoint, visual deepfakes are devised through the use of machine learning tools that are able to decode and strip down the images of all the facial expressions related to the two individuals into a matrix consisting of certain key attributes, such as the position of the target’s nose, eyes and mouth. Additionally, finer details, such as skin texture and facial hair, are given less importance and can be thought of as secondary.
The deconstruction, in general, is performed in such a way that it is mostly always possible to fully recreate the original image of each face from its stripped elements. Additionally, one of the primary aspects of creating a quality deepfake is how well the final image is reconstructed — such that any movements in the face of the imitator are realized in the target’s face as well.
To elaborate on the matter, Matthew Dixon, an assistant professor and researcher at the Illinois Institute of Technology’s Stuart School of Business, told Cointelegraph that both face and voice can be easily reconstructed through certain programs and techniques, adding that:
“Once a person has been digitally cloned it is possible to then generate fake video footage of them saying anything, including speaking words of malicious propaganda on social media. The average social-media follower would be unable to discern that the video was fake.”
Similarly, speaking on the finer aspects of deepfake technology, Vlad Miller, CEO of Ethereum Express — a cross-platform solution that is based on an innovative model with its own blockchain and uses a proof-of-authority consensus protocol — told Cointelegraph that deepfakes are simply a way of synthesizing human images by making use of a machine learning technique called GAN, an algorithm that deploys a combination of two neural networks.
The first generates the image samples, while the second distinguishes the real samples from the fake ones. GAN’s operational utility can be compared to the work of two people, such that the first person is engaged in counterfeiting while the other tries to distinguish the copies from the originals. If the first algorithm offers an obvious fake, the second will immediately determine it, after which the first will improve its work by offering a more realistic image.
Regarding the negative social and political implications that deepfake videos can have on the masses, Steve McNew, a MIT trained blockchain/cryptocurrency expert and senior managing director at FTI Consulting, told Cointelegraph:
“Online videos are exploding as a mainstream source of information. Imagine social media and news outlets frantically and perhaps unknowingly sharing altered clips — of police bodycam video, politicians in unsavory situations or world leaders delivering inflammatory speeches — to create an alternate truth. The possibilities for deepfakes to create malicious propaganda and other forms of fraud are significant.”
Examples of deepfakes being used for nefarious purposes
Since deepfake technology is able to manipulate and imitate the facial features and personality characteristics of real-world individuals, it raises many legitimate concerns, especially in relation to its use for various shady activities.
Additionally, for many years now, the internet has been flooded with simple tutorials that teach people how to create digitally altered audio/video data that can fool various facial recognition systems.
Not only that, but some truly disturbing instances of audio/video manipulation have recently surfaced that have called into question the utility of deepfakes. For example, a recent article claims that since 2014, deepfake technology has advanced to such levels that today, it can be used to produce videos in which the target can not only be made to express certain emotions but also bear resemblance to certain ethnic groups as well as look a certain age. On the subject, Martin Zizi, CEO of Aerendir, a physiological biometric technology provider, pointed out to Cointelegraph:
“AI does not learn from mistakes, but from plain statistics. It may seem like a small detail, but AI-based on plain statistics — even with trillion bytes of data — is just that, a statistical analysis of many dimensions. So, if you play with statistics, you can die by statistics.”
Zizi then went on to add that another key facet of facial recognition is that it is based on neural networks that are quite fragile in nature. From a structural standpoint, these networks can be thought of as cathedrals, wherein once you remove one cornerstone, the whole edifice crumbles. To further elaborate on the subject, Zizi stated:
“By removing 3 to 5 pixels from a 12 million pixels image of someone’s face brings recognition to zero! Researchers have found that adversarial attacks on neural net attacks can find those 3 to 5 pixels that represent the ‘cornerstones’ in the image.”
One last big example of deepfake tech being misused for financial reasons was when the CEO of an unnamed United Kingdom-based energy firm was recently scammed into transferring 220,000 euros ($243,000) to an unknown bank account because he believed he was on the phone with his boss, the chief executive of the firm’s parent company. In reality, the voice belonged to a scammer who had made use of deepfake voice technology to spoof the executive.
Blockchain may help against deepfakes
As per a recent 72-page report issued by Witness Media Lab, blockchain has been cited as being a legitimate tool for countering the various digital threats put forth by deepfake technology.
In this regard, using blockchain, people can digitally sign and confirm the authenticity of various video or audio files that are directly or indirectly related to them. Thus, the more digital signatures that are added to a particular video, the more likely it will be considered authentic.
Related: As Deepfake Videos Spread, Blockchain Can Be Used to Stop Them
Commenting on the matter, Greg Forst, director of marketing for Factom Protocol, told Cointelegraph that when it comes to deepfakes, blockchain has the potential to offer the global tech community with a unique solution — or at least a major part of it. He pointed out:
“If video content is on the blockchain once it has been created, along with a verifying tag or graphic, it puts a roadblock in front of deepfake endeavors. However, this hinges on video content being added to the blockchain from the outset. From there, digital identities must underline the origins and creator of the content. Securing data at source and having some standardization for media will go a long way.”
McNew also believes that owing to the blockchain’s overall immutability, once a particular data block has been confirmed by the network, its contents cannot be altered. Thus, if videos (or even photos, for that matter) are made to flow immediately into a blockchain verification application before being made available for sharing, altered videos could be easily identified as fake.
Lastly, a similar idea was shared by Miller, who is of the opinion that blockchain technology in conjunction with artificial intelligence can help solve many of the privacy and security concerns put forth by deepfakes. He added:
“AI perfectly copes with the collection, analysis, sorting and transmission of data, improving the speed and quality of execution of internal processes. The blockchain, in turn, ‘makes sure’ that no one intervenes in the work of AI — it protects data and its sequence from any encroachment.”
Blockchain technology has its own limitations
As things stand, there are a few small drawbacks that are preventing blockchain technology from being actively used to monitor deepfakes on the internet. For starters, the technology is limited in its overall scalability, as the amount of computational resources and memory required to combat digitally manipulated A/V data in real-time is quite intense.
Another potential issue that could arise as a result of blockchain being used for deepfake detection is a substantial curbing of crowdsourced video content (such as the material that is currently available on YouTube). On the issue, Dixon pointed out:
“How does someone in a poor country reach the world with their message if they have to be approved by a Silicon Valley-based company? Should we be entrusting tech companies with such power? Liberty is always at stake when trust weakens.”
A similar opinion is shared by Hibryda, creator and founder of Bitlattice, a distributed ledger system that uses a multidimensional lattice structure to address issues such as scalability, security, timing, etc. In his view:
“The biggest drawback of blockchain tech lies in its inability to determine whether the signed media is really genuine or not. But that isn’t an internal issue of blockchain or related technologies — they only provide ledgers that are extremely hard to manipulate. It’s external and there’s no good way to solve that. While crowd-powered verification could be a partial solution, given crowds can be manipulated it’s rather impossible to build a system that provides reliable and objective fact-checking.”
However, Forst told Cointelegraph that while the majority of people tend to believe that leveraging blockchain might be too expensive for deepfake detection, there are several open-source solutions that seek to do this. Forst then added that, “The biggest drawback is that blockchain doesn’t solve the problem with deepfakes in its entirety, rather it can be a piece of the solution.”
0 notes
Text
Deep Truths of Deepfakes — Tech That Can Fool Anyone
Deep Truths of Deepfakes — Tech That Can Fool Anyone:
In its most basic sense, a deepfake is a combination of face- and voice-cloning AI technologies that allow for the creation of life-like, computer-generated videos of a real person.
In order to develop a high-quality deepfake of an individual, developers need to accumulate tens of hours of video footage associated with the person whose face/voice is to be cloned, as well as a human imitator who has learned the facial mannerisms and voice of the target.
There are two humans involved in the creation of a deepfake, such that the target face/voice is that of the famous person while the other belongs to an unknown individual who is generally closely associated with the project.
From tech to reality
From a technical standpoint, visual deepfakes are devised through the use of machine learning tools that are able to decode and strip down the images of all the facial expressions related to the two individuals into a matrix consisting of certain key attributes, such as the position of the target’s nose, eyes and mouth. Additionally, finer details, such as skin texture and facial hair, are given less importance and can be thought of as secondary.
The deconstruction, in general, is performed in such a way that it is mostly always possible to fully recreate the original image of each face from its stripped elements. Additionally, one of the primary aspects of creating a quality deepfake is how well the final image is reconstructed — such that any movements in the face of the imitator are realized in the target’s face as well.
To elaborate on the matter, Matthew Dixon, an assistant professor and researcher at the Illinois Institute of Technology’s Stuart School of Business, told Cointelegraph that both face and voice can be easily reconstructed through certain programs and techniques, adding that:
“Once a person has been digitally cloned it is possible to then generate fake video footage of them saying anything, including speaking words of malicious propaganda on social media. The average social-media follower would be unable to discern that the video was fake.”
Similarly, speaking on the finer aspects of deepfake technology, Vlad Miller, CEO of Ethereum Express — a cross-platform solution that is based on an innovative model with its own blockchain and uses a proof-of-authority consensus protocol — told Cointelegraph that deepfakes are simply a way of synthesizing human images by making use of a machine learning technique called GAN, an algorithm that deploys a combination of two neural networks.
The first generates the image samples, while the second distinguishes the real samples from the fake ones. GAN’s operational utility can be compared to the work of two people, such that the first person is engaged in counterfeiting while the other tries to distinguish the copies from the originals. If the first algorithm offers an obvious fake, the second will immediately determine it, after which the first will improve its work by offering a more realistic image.
Regarding the negative social and political implications that deepfake videos can have on the masses, Steve McNew, a MIT trained blockchain/cryptocurrency expert and senior managing director at FTI Consulting, told Cointelegraph:
“Online videos are exploding as a mainstream source of information. Imagine social media and news outlets frantically and perhaps unknowingly sharing altered clips — of police bodycam video, politicians in unsavory situations or world leaders delivering inflammatory speeches — to create an alternate truth. The possibilities for deepfakes to create malicious propaganda and other forms of fraud are significant.”
Examples of deepfakes being used for nefarious purposes
Since deepfake technology is able to manipulate and imitate the facial features and personality characteristics of real-world individuals, it raises many legitimate concerns, especially in relation to its use for various shady activities.
Additionally, for many years now, the internet has been flooded with simple tutorials that teach people how to create digitally altered audio/video data that can fool various facial recognition systems.
Not only that, but some truly disturbing instances of audio/video manipulation have recently surfaced that have called into question the utility of deepfakes. For example, a recent article claims that since 2014, deepfake technology has advanced to such levels that today, it can be used to produce videos in which the target can not only be made to express certain emotions but also bear resemblance to certain ethnic groups as well as look a certain age. On the subject, Martin Zizi, CEO of Aerendir, a physiological biometric technology provider, pointed out to Cointelegraph:
“AI does not learn from mistakes, but from plain statistics. It may seem like a small detail, but AI-based on plain statistics — even with trillion bytes of data — is just that, a statistical analysis of many dimensions. So, if you play with statistics, you can die by statistics.”
Zizi then went on to add that another key facet of facial recognition is that it is based on neural networks that are quite fragile in nature. From a structural standpoint, these networks can be thought of as cathedrals, wherein once you remove one cornerstone, the whole edifice crumbles. To further elaborate on the subject, Zizi stated:
“By removing 3 to 5 pixels from a 12 million pixels image of someone’s face brings recognition to zero! Researchers have found that adversarial attacks on neural net attacks can find those 3 to 5 pixels that represent the ‘cornerstones’ in the image.”
One last big example of deepfake tech being misused for financial reasons was when the CEO of an unnamed United Kingdom-based energy firm was recently scammed into transferring 220,000 euros ($243,000) to an unknown bank account because he believed he was on the phone with his boss, the chief executive of the firm’s parent company. In reality, the voice belonged to a scammer who had made use of deepfake voice technology to spoof the executive.
Blockchain may help against deepfakes
As per a recent 72-page report issued by Witness Media Lab, blockchain has been cited as being a legitimate tool for countering the various digital threats put forth by deepfake technology.
In this regard, using blockchain, people can digitally sign and confirm the authenticity of various video or audio files that are directly or indirectly related to them. Thus, the more digital signatures that are added to a particular video, the more likely it will be considered authentic.
Related: As Deepfake Videos Spread, Blockchain Can Be Used to Stop Them
Commenting on the matter, Greg Forst, director of marketing for Factom Protocol, told Cointelegraph that when it comes to deepfakes, blockchain has the potential to offer the global tech community with a unique solution — or at least a major part of it. He pointed out:
“If video content is on the blockchain once it has been created, along with a verifying tag or graphic, it puts a roadblock in front of deepfake endeavors. However, this hinges on video content being added to the blockchain from the outset. From there, digital identities must underline the origins and creator of the content. Securing data at source and having some standardization for media will go a long way.”
McNew also believes that owing to the blockchain’s overall immutability, once a particular data block has been confirmed by the network, its contents cannot be altered. Thus, if videos (or even photos, for that matter) are made to flow immediately into a blockchain verification application before being made available for sharing, altered videos could be easily identified as fake.
Lastly, a similar idea was shared by Miller, who is of the opinion that blockchain technology in conjunction with artificial intelligence can help solve many of the privacy and security concerns put forth by deepfakes. He added:
“AI perfectly copes with the collection, analysis, sorting and transmission of data, improving the speed and quality of execution of internal processes. The blockchain, in turn, ‘makes sure’ that no one intervenes in the work of AI — it protects data and its sequence from any encroachment.”
Blockchain technology has its own limitations
As things stand, there are a few small drawbacks that are preventing blockchain technology from being actively used to monitor deepfakes on the internet. For starters, the technology is limited in its overall scalability, as the amount of computational resources and memory required to combat digitally manipulated A/V data in real-time is quite intense.
Another potential issue that could arise as a result of blockchain being used for deepfake detection is a substantial curbing of crowdsourced video content (such as the material that is currently available on YouTube). On the issue, Dixon pointed out:
“How does someone in a poor country reach the world with their message if they have to be approved by a Silicon Valley-based company? Should we be entrusting tech companies with such power? Liberty is always at stake when trust weakens.”
A similar opinion is shared by Hibryda, creator and founder of Bitlattice, a distributed ledger system that uses a multidimensional lattice structure to address issues such as scalability, security, timing, etc. In his view:
“The biggest drawback of blockchain tech lies in its inability to determine whether the signed media is really genuine or not. But that isn’t an internal issue of blockchain or related technologies — they only provide ledgers that are extremely hard to manipulate. It’s external and there’s no good way to solve that. While crowd-powered verification could be a partial solution, given crowds can be manipulated it’s rather impossible to build a system that provides reliable and objective fact-checking.”
However, Forst told Cointelegraph that while the majority of people tend to believe that leveraging blockchain might be too expensive for deepfake detection, there are several open-source solutions that seek to do this. Forst then added that, “The biggest drawback is that blockchain doesn’t solve the problem with deepfakes in its entirety, rather it can be a piece of the solution.”
0 notes
Link
In its most basic sense, a deepfake is a combination of face- and voice-cloning AI technologies that allow for the creation of life-like, computer-generated videos of a real person.
In order to develop a high-quality deepfake of an individual, developers need to accumulate tens of hours of video footage associated with the person whose face/voice is to be cloned, as well as a human imitator who has learned the facial mannerisms and voice of the target.
There are two humans involved in the creation of a deepfake, such that the target face/voice is that of the famous person while the other belongs to an unknown individual who is generally closely associated with the project.
From tech to reality
From a technical standpoint, visual deepfakes are devised through the use of machine learning tools that are able to decode and strip down the images of all the facial expressions related to the two individuals into a matrix consisting of certain key attributes, such as the position of the target’s nose, eyes and mouth. Additionally, finer details, such as skin texture and facial hair, are given less importance and can be thought of as secondary.
The deconstruction, in general, is performed in such a way that it is mostly always possible to fully recreate the original image of each face from its stripped elements. Additionally, one of the primary aspects of creating a quality deepfake is how well the final image is reconstructed — such that any movements in the face of the imitator are realized in the target’s face as well.
To elaborate on the matter, Matthew Dixon, an assistant professor and researcher at the Illinois Institute of Technology’s Stuart School of Business, told Cointelegraph that both face and voice can be easily reconstructed through certain programs and techniques, adding that:
“Once a person has been digitally cloned it is possible to then generate fake video footage of them saying anything, including speaking words of malicious propaganda on social media. The average social-media follower would be unable to discern that the video was fake.”
Similarly, speaking on the finer aspects of deepfake technology, Vlad Miller, CEO of Ethereum Express — a cross-platform solution that is based on an innovative model with its own blockchain and uses a proof-of-authority consensus protocol — told Cointelegraph that deepfakes are simply a way of synthesizing human images by making use of a machine learning technique called GAN, an algorithm that deploys a combination of two neural networks.
The first generates the image samples, while the second distinguishes the real samples from the fake ones. GAN’s operational utility can be compared to the work of two people, such that the first person is engaged in counterfeiting while the other tries to distinguish the copies from the originals. If the first algorithm offers an obvious fake, the second will immediately determine it, after which the first will improve its work by offering a more realistic image.
Regarding the negative social and political implications that deepfake videos can have on the masses, Steve McNew, a MIT trained blockchain/cryptocurrency expert and senior managing director at FTI Consulting, told Cointelegraph:
“Online videos are exploding as a mainstream source of information. Imagine social media and news outlets frantically and perhaps unknowingly sharing altered clips — of police bodycam video, politicians in unsavory situations or world leaders delivering inflammatory speeches — to create an alternate truth. The possibilities for deepfakes to create malicious propaganda and other forms of fraud are significant.”
Examples of deepfakes being used for nefarious purposes
Since deepfake technology is able to manipulate and imitate the facial features and personality characteristics of real-world individuals, it raises many legitimate concerns, especially in relation to its use for various shady activities.
Additionally, for many years now, the internet has been flooded with simple tutorials that teach people how to create digitally altered audio/video data that can fool various facial recognition systems.
Not only that, but some truly disturbing instances of audio/video manipulation have recently surfaced that have called into question the utility of deepfakes. For example, a recent article claims that since 2014, deepfake technology has advanced to such levels that today, it can be used to produce videos in which the target can not only be made to express certain emotions but also bear resemblance to certain ethnic groups as well as look a certain age. On the subject, Martin Zizi, CEO of Aerendir, a physiological biometric technology provider, pointed out to Cointelegraph:
“AI does not learn from mistakes, but from plain statistics. It may seem like a small detail, but AI-based on plain statistics — even with trillion bytes of data — is just that, a statistical analysis of many dimensions. So, if you play with statistics, you can die by statistics.”
Zizi then went on to add that another key facet of facial recognition is that it is based on neural networks that are quite fragile in nature. From a structural standpoint, these networks can be thought of as cathedrals, wherein once you remove one cornerstone, the whole edifice crumbles. To further elaborate on the subject, Zizi stated:
“By removing 3 to 5 pixels from a 12 million pixels image of someone’s face brings recognition to zero! Researchers have found that adversarial attacks on neural net attacks can find those 3 to 5 pixels that represent the ‘cornerstones’ in the image.”
One last big example of deepfake tech being misused for financial reasons was when the CEO of an unnamed United Kingdom-based energy firm was recently scammed into transferring 220,000 euros ($243,000) to an unknown bank account because he believed he was on the phone with his boss, the chief executive of the firm’s parent company. In reality, the voice belonged to a scammer who had made use of deepfake voice technology to spoof the executive.
Blockchain may help against deepfakes
As per a recent 72-page report issued by Witness Media Lab, blockchain has been cited as being a legitimate tool for countering the various digital threats put forth by deepfake technology.
In this regard, using blockchain, people can digitally sign and confirm the authenticity of various video or audio files that are directly or indirectly related to them. Thus, the more digital signatures that are added to a particular video, the more likely it will be considered authentic.
Related: As Deepfake Videos Spread, Blockchain Can Be Used to Stop Them
Commenting on the matter, Greg Forst, director of marketing for Factom Protocol, told Cointelegraph that when it comes to deepfakes, blockchain has the potential to offer the global tech community with a unique solution — or at least a major part of it. He pointed out:
“If video content is on the blockchain once it has been created, along with a verifying tag or graphic, it puts a roadblock in front of deepfake endeavors. However, this hinges on video content being added to the blockchain from the outset. From there, digital identities must underline the origins and creator of the content. Securing data at source and having some standardization for media will go a long way.”
McNew also believes that owing to the blockchain’s overall immutability, once a particular data block has been confirmed by the network, its contents cannot be altered. Thus, if videos (or even photos, for that matter) are made to flow immediately into a blockchain verification application before being made available for sharing, altered videos could be easily identified as fake.
Lastly, a similar idea was shared by Miller, who is of the opinion that blockchain technology in conjunction with artificial intelligence can help solve many of the privacy and security concerns put forth by deepfakes. He added:
“AI perfectly copes with the collection, analysis, sorting and transmission of data, improving the speed and quality of execution of internal processes. The blockchain, in turn, ‘makes sure’ that no one intervenes in the work of AI — it protects data and its sequence from any encroachment.”
Blockchain technology has its own limitations
As things stand, there are a few small drawbacks that are preventing blockchain technology from being actively used to monitor deepfakes on the internet. For starters, the technology is limited in its overall scalability, as the amount of computational resources and memory required to combat digitally manipulated A/V data in real-time is quite intense.
Another potential issue that could arise as a result of blockchain being used for deepfake detection is a substantial curbing of crowdsourced video content (such as the material that is currently available on YouTube). On the issue, Dixon pointed out:
“How does someone in a poor country reach the world with their message if they have to be approved by a Silicon Valley-based company? Should we be entrusting tech companies with such power? Liberty is always at stake when trust weakens.”
A similar opinion is shared by Hibryda, creator and founder of Bitlattice, a distributed ledger system that uses a multidimensional lattice structure to address issues such as scalability, security, timing, etc. In his view:
“The biggest drawback of blockchain tech lies in its inability to determine whether the signed media is really genuine or not. But that isn’t an internal issue of blockchain or related technologies — they only provide ledgers that are extremely hard to manipulate. It’s external and there’s no good way to solve that. While crowd-powered verification could be a partial solution, given crowds can be manipulated it’s rather impossible to build a system that provides reliable and objective fact-checking.”
However, Forst told Cointelegraph that while the majority of people tend to believe that leveraging blockchain might be too expensive for deepfake detection, there are several open-source solutions that seek to do this. Forst then added that, “The biggest drawback is that blockchain doesn’t solve the problem with deepfakes in its entirety, rather it can be a piece of the solution.”
0 notes