#dataops
Explore tagged Tumblr posts
Text
What sets Konnect Insights apart from other data orchestration and analysis tools available in the market for improving customer experiences in the aviation industry?
I can highlight some general factors that may set Konnect Insights apart from other data orchestration and analysis tools available in the market for improving customer experiences in the aviation industry. Keep in mind that the competitive landscape and product offerings may have evolved since my last knowledge update. Here are some potential differentiators:
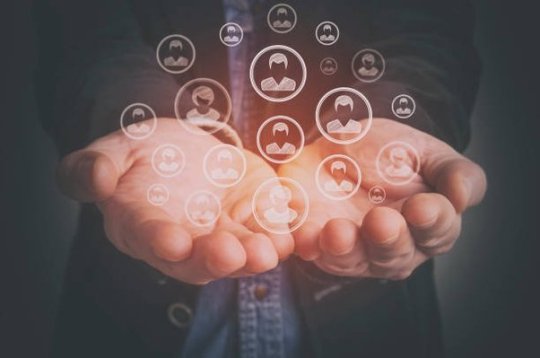
Aviation Industry Expertise: Konnect Insights may offer specialized features and expertise tailored to the unique needs and challenges of the aviation industry, including airports, airlines, and related businesses.
Multi-Channel Data Integration: Konnect Insights may excel in its ability to integrate data from a wide range of sources, including social media, online platforms, offline locations within airports, and more. This comprehensive data collection can provide a holistic view of the customer journey.
Real-Time Monitoring: The platform may provide real-time monitoring and alerting capabilities, allowing airports to respond swiftly to emerging issues or trends and enhance customer satisfaction.
Customization: Konnect Insights may offer extensive customization options, allowing airports to tailor the solution to their specific needs, adapt to unique workflows, and focus on the most relevant KPIs.
Actionable Insights: The platform may be designed to provide actionable insights and recommendations, guiding airports on concrete steps to improve the customer experience and operational efficiency.
Competitor Benchmarking: Konnect Insights may offer benchmarking capabilities that allow airports to compare their performance to industry peers or competitors, helping them identify areas for differentiation.
Security and Compliance: Given the sensitive nature of data in the aviation industry, Konnect Insights may include robust security features and compliance measures to ensure data protection and adherence to industry regulations.
Scalability: The platform may be designed to scale effectively to accommodate the data needs of large and busy airports, ensuring it can handle high volumes of data and interactions.
Customer Support and Training: Konnect Insights may offer strong customer support, training, and consulting services to help airports maximize the value of the platform and implement best practices for customer experience improvement.
Integration Capabilities: It may provide seamless integration with existing airport systems, such as CRM, ERP, and database systems, to ensure data interoperability and process efficiency.
Historical Analysis: The platform may enable airports to conduct historical analysis to track the impact of improvements and initiatives over time, helping measure progress and refine strategies.
User-Friendly Interface: Konnect Insights may prioritize a user-friendly and intuitive interface, making it accessible to a wide range of airport staff without requiring extensive technical expertise.
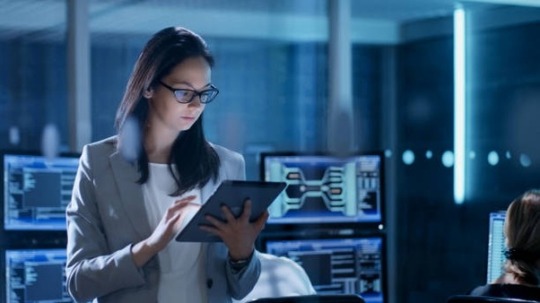
It's important for airports and organizations in the aviation industry to thoroughly evaluate their specific needs and conduct a comparative analysis of available solutions to determine which one aligns best with their goals and requirements. Additionally, staying updated with the latest developments and customer feedback regarding Konnect Insights and other similar tools can provide valuable insights when making a decision.
#DataOrchestration#DataManagement#DataOps#DataIntegration#DataEngineering#DataPipeline#DataAutomation#DataWorkflow#ETL (Extract#Transform#Load)#DataIntegrationPlatform#BigData#CloudComputing#Analytics#DataScience#AI (Artificial Intelligence)#MachineLearning#IoT (Internet of Things)#DataGovernance#DataQuality#DataSecurity
2 notes
·
View notes
Text
youtube
Power Platform Bootcamp Buenos Aires
Evento de la comunidad Argentina con grandes sesiones.
El Poder de Python y R en Power BI por Mike Ramirez
Transforme datos con buenas prácticas de Power Query por Ignacio Barrau
Agentes en Power Platform: El Futuro de la IA por Andrés Arias Falcón
Power Virtual Agent - Charlando con tu base de datos por Matias Molina y Nicolas Muñoz
¿Por qué deberían los desarrolladores automatizar? por Mauro Gioberti
Microsoft Fabric + Power BI: Arquitecturas y Licenciamiento - Todo lo que necesitas saber por Gonzalo Bissio y Maximiliano Accotto
El auge de los copilotos: De la adopción del low-code a la maestría en análisis de datos por Gaston Cruz y Alex Rostan
Libérate de las Tareas Repetitivas: Potencia tu Talento con Power Apps y Power Automate por Anderson Estrada
Introducción a Microsoft Fabric - Data analytics para la era de al Inteligencia Artificial por Javier Villegas
Tu Primer despliegue con PBIP Power BI Control de versiones CI/CD GIT en GITHUB por Vicente Antonio Juan Magallanes
Espero que lo disfruten
#power bi#power platform#powerbi#power bi argentina#power bi cordoba#power bi jujuy#power bi tips#power bi tutorial#power bi training#fabric#microsoft fabric#dataops#power bi cicd#Youtube
0 notes
Text

Agile data systems enable businesses to innovate and scale with confidence. At #RoundTheClockTechnologies, data engineering services are designed to provide clean, integrated, and business-aligned datasets that fuel innovation across every department. From setting up reliable data lakes to configuring BI-friendly data marts, our solutions bridge the gap between raw inputs and strategic outcomes.
We automate complex transformations, eliminate data duplication, and ensure that every pipeline is optimized for speed and accuracy. Leveraging platforms like AWS, Snowflake, and Azure, we create secure and high-performing data environments tailored to business needs. Whether supporting real-time analytics or feeding predictive models, our goal is to help organizations unlock the full value of their data assets—efficiently, consistently, and securely.
Learn more about our data engineering services at https://rtctek.com/data-engineering-services/
#rtctek#roundtheclocktechnologies#dataengineering#dataanalytics#datadriven#etlprocesses#cloudataengineering#dataintegration#businessintelligence#dataops
0 notes
Text
In today’s world, data is considered one of the most valuable assets any business can have. However, to truly unlock the power of data, it’s not enough to simply collect it—organizations need to ensure that the data they are working with is accurate, consistent, and reliable. That’s where Data Quality Observability comes in.
Data Quality Observability is the ability to monitor, understand, and proactively manage the state of data across an entire ecosystem. With the growing complexity of data pipelines and the increasing reliance on data-driven decisions, organizations can no longer afford to ignore the health of their data. Data quality observability helps businesses identify issues before they impact operations, making it a critical part of any data strategy.
#datagaps#data quality#data#dataops#dataops suite#data quality observability#QA tester#Data Analysts#BI Experts
0 notes
Text
𝐓𝐡𝐞 𝐔𝐥𝐭𝐢𝐦𝐚𝐭𝐞 𝐃𝐚𝐭𝐚 𝐏𝐥𝐚𝐲𝐛𝐨𝐨𝐤 𝐢𝐧 2025 (𝐖𝐡𝐚𝐭'𝐬 𝐈𝐧, 𝐖𝐡𝐚𝐭'𝐬 𝐎𝐮𝐭)
The modern data stack is evolving—fast. In this video, we’ll break down the essential tools, trends, and architectures defining data in 2025. From Snowflake vs Databricks to ELT 2.0, metadata layers, and real-time infra—this is your executive cheat sheet.
Whether you're building a data platform, leading a team, or just staying ahead, this is the future-proof playbook.
Watch more https://youtu.be/EyTmxn4xHrU
#moderndatastack#datainfrastructure#dataengineering#dataanalytics#elt#datapipeline#dbt#snowflake#databricks#dagster#realdata#data2025#futureofdata#dataops#apacheiceberg#duckdb#vectordatabase#langchain#analyticsstack#dataarchitecture
0 notes
Text
Maven Essential Tutorial for Beginners with Demo 2021 | Part -1
AiOps & MLOps School empowers IT professionals through hands-on training, certifications, and expert mentorship, combining practical skills with industry insights. We offer training, certification, guidance, and consulting for DevOps, Big Data, Cloud, dataops, AiOps, MLOps, DevSecOps, GitOps, DataOps, ITOps, SysOps, SecOps, ModelOps, NoOps, FinOps, XOps, BizDevOps, CloudOps, SRE and PlatformOps. 🔔 Don't Miss Out! Hit Subscribe and Ring the Bell! 🔔 👉 Subscribe Now
0 notes
Text

Siloed data can hinder your journey to becoming a truly data-driven business. DataOps accelerates this process by bringing Data and Ops teams together on a unified platform. This collaboration streamlines workflows, enabling data to flow through the pipeline faster and continuously delivering actionable insights and measurable value to your business. Take a look at this infographic for a quick introduction to DataOps.
0 notes
Text
Creole Studios Launches Comprehensive Data Engineering Services with Azure Databricks Partnership
Creole Studios introduces its cutting-edge Data Engineering Services, aimed at empowering organizations to extract actionable insights from their data. Backed by certified experts and a strategic partnership with Azure Databricks, we offer comprehensive solutions spanning data strategy, advanced analytics, and secure data warehousing. Unlock the full potential of your data with Creole Studios' tailored data engineering services, designed to drive innovation and efficiency across industries.
#DataEngineering#BigDataAnalytics#DataStrategy#DataOps#DataQuality#AzureDatabricks#DataWarehousing#MachineLearning#DataAnalytics#BusinessIntelligence
0 notes
Text
Why Big Data needs DevOps
#BigDataDevOps#DataOps#TechIntegration#DataEngineering#DevOpsCulture#DataScience#ContinuousIntegration#DataManagement
0 notes
Text
Estrategia para licencias PowerBi - Fabric
La popularidad de Fabric no para de extenderse y eso hace que cada vez más aparezcan confusiones y dudas sobre licencias. Cada día llegan más dudas sobre el formato de licencias y como encaja lo nuevo en lo viejo. Dentro de esas dudas aparecen muchas alternativas para manejo de licencias.
En este artículo vamos a hablar de puntos medios y grises para optimizar costos de nuestras licencias encontrando el mejor balance con los nuevos planes que nos ofrece Fabric.
Primero vamos con conocimientos básicos de licencias en este antiguo artículo que escribí. En el mismo vamos a conocer detalles de las licencias disponibles en julio 2024.
Si ya conocen las de PowerBi, lo nuevo es Fabric. Una licencia por capacidad que viene a expandir los artefactos o contenidos que podemos crear dentro de un área de trabajo. Sus planes inician con valores más económicos que las capacidades anteriores, lo que permiten que todo tipo de organización pueda acceder al uso de éstos artefactos. Sin embargo, el uso de los tradicionales contenidos de PowerBi siguen bajo el mismo esquema.
La estrategía de optimización se base específicamente en los usuarios. Si tenemos capacidades dedicadas... ¿Por qué pagamos licencias por usuarios? esta es la pregunta que trae el principal contexto y razón del artículo. A las organizaciones les cuesta este concepto, más aún viendo que los contenidos de Fabric no necesitan licencias de usuario. No necesitamos una licencia PRO para desarrollar un notebook, escribir en Onelake o consultar un warehouse con SQL. Para resolver esta paradoja vamos a dividir nuestro enfoque en los dos tipos de usuarios, desarrolladores y visores.
Enfoque de Visores
Algo que suele trae mucho dolor a organizaciones que usan PowerBi es que los visores en entornos de capacidad compartida (pro o ppu), tengan que pagar licencia. Uno puede pensar que pagando una "Capacidad Dedicada" como premium lo soluciona, sin embargo premium va a desaparecer y quedará Fabric. Muchos podrán pensar que esta es la solución definitiva, puesto que Fabric comienza con planes muy económicos, pero no es cierto. La característica de compartir con usuarios gratuitos comienza en un plan F64, cuyo costo ronda entre 5000 y 8000 dolares (dependiendo el tipo de pago). Lo que nos hace pensar que valdría la pena si nuestra operación interna cuenta con más de 500 usuarios visores de PowerBi (puesto que una licencia pro sale 10 dolares). Ciertamente, un número que solo grandes empresas pueden considerar.
Entonces, ¿Qué hacemos cuando no podemos pagar ese monto? Si podemos comprar capacidades dedicadas más chicas, ¿Cómo podríamos compartir a usuarios visores sin pagarles licencias Pro? La respuesta es una característica bastante olvidada en el entorno de analytics. Estoy hablando de la feature de "Embedded". PowerBi cuenta con una compleja pero poderosa posibilidad de embeber informes en aplicaciones web con grandes posibilidades. Una de esas posibilidades es delegar la seguridad y loguear a la aplicación que refleja los informes. De ese modo, podríamos compartir a todos los usuarios visores que querramos sin pagar licencias. Sin embargo, no es tan simple puesto que necesitaríamos un potente equipo de desarrollo que construya esta web app aprendiendo todos los enfoques de power bi con especial atención a la seguridad, dado que ahora es responsabilidad nuestra (nos la delega microsoft al usar embedded).
Si no tenemos equipo de desarrollo y queremos aprovechar esa capacidad. ¿Cómo lo resolvemos?. La opción más viable sería comprando un producto de un tercero. Varias instituciones proveen este tipo de software. Por ejemplo, una que conozco en español es PiBi. PiBi es una plataforma web como servicio (SaaS) que usa la característica de Power Bi Embedded. Una construcción que interactúa con tu entorno de Azure y te permite distribuir informes de PowerBi de manera segura, simple y eficiente.
¿Qué ventajas encontramos si nos embarcamos en este camino?
Operación de informes en un solo lugar
Más sencillo de usar que Fabric/PowerBi Service
Compartir con usuarios externos a la organización
Logins SSO Google/Microsoft
Mantener seguridad y privacidad
El requerimiento para usar Embedded es tener una licencia por capacidad. Herramientas como ésts pueden partir de valores como 450 dolares por mes, que junto a una licencia básica de Fabric con pago anual de 150 dolares son 600 dolares. Si nuestra operación de usuarios internos ronda entre 60 y 500 usuarios, encaja perfectamente para optimizar nuestra estrategia de Power Bi. Por supuesto, que habría detalles como si tenemos modelos y mucho volumen de datos, puede que necesitemos un Fabric un poco más elevado, pero deberíamos pensar a ese como costo de almacenamiento y no de distribución de contenido a los usuarios.
Enfoque de desarrolladores
En la mayoría de los casos, lo primero que normalmente tendería a evolucionar, son los visores. Una vez encontrado un punto de madurez en ellos, seguirá esta propuesta. Esto quiere decir que lo más probable es que YA contemos con capacidad dedicada (para usar la feature embedded). Pero no desesperen si no la tienen, esta estretegia es totalmente viable sin capacidad tal como lo explico en este otro artículo. Las estrategias no estan ligadas una a otra, aunque son poderosas juntas.
El eje para liberar a los usuarios desarrolladores de sus licencias es pensar en desarrollo de software convencional. Si pensamos en la industra de software, un desarrollador, sea backend o frontend, tiene como trabajo solamente desarrollar. Su eje y foco esta en construir. Hoy esos roles no hacen integración de soluciones, implementaciones o deploys, puestas en producción, etc. Existen roles como DevOps que ayudan a delegar la construcción final.
Tomando este enfoque como guía, si queremos que los desarrolladores de PowerBi no usen licencias, debemos concentrarnos en lo básico, un repositorio. Hoy Fabric nos da la posibilidad de integrar repositorios de GIT con Áreas de Trabajo. Esto no solo nos ayuda a que el desarrollador ni piense en Fabric o PowerBi Service, sino que también almacena los desarrollos en formato Power Bi Project que nos deja mergear código. Si queremos ver eso en más profundidad pueden abrir éste artículo.
Así los desarrolladores de Power Bi. Solo desarrollarán. Comienzan el día armando o con un pull a su branch de repositorio, comiteando cambios y al terminar un push. Ni piensa ni escucha quienes ven que informes y donde está. Luego podemos pensar en otro perfil que podemos decirle Admin del Area o DataOps que garantice que los reportes están en el lugar apropiado o sean automáticamente deployados. Veamos un poco más gráfico esto:
Vean como Local Machine nunca ve Fabric. El desarrollador no necesita ingresar al portal web. Solo necesitamos licencias para DataOps o Administradores de areas. Así es como reducimos licencias y ganamos robustez y soluciones tanto versionables como escalables.
Se que fue mucho texto pero espero que haya sido a meno y les traigan nuevas ideas para optimizar efectivamente la operación y licencias de sus entornos.
#fabric#microsoft fabric#powerbi#power bi#dataops#pibi#ladataweb#power bi embedded#power bi training#power bi tutorial#power bi tips#power bi argentina#power bi cordoba#power bi jujuy#power bi isv
0 notes
Text
Navigating the World of Data Engineering
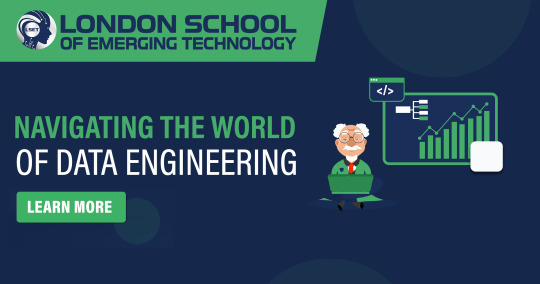
Navigating the World of Data Engineering. An Overview of Data Infrastructure! Dive into the dynamic field of data engineering to learn the fundamental ideas and strategies for developing strong data systems. LSET's thorough handbook delves into data modelling, processing, and pipeline creation, providing a road map for creating scalable and efficient data infrastructure. In addition, the London School of Emerging Technology (LSET) Data Engineer Course will provide expert-led training and hands-on experience in mastering data engineering tools and processes. Join on a path to becoming a skilled data engineer, prepared to face difficult data issues and drive digital innovation.
Enrol @ https://lset.uk/ for admission.
0 notes
Text
How Databricks Unity Catalog and Datagaps Automate Governance and Validation

Data quality is the backbone of accurate analytics, regulatory compliance, and efficient business operations. As organizations scale their data ecosystems, maintaining high data integrity becomes more challenging.
The seamless integration between Databricks Unity Catalog and Datagaps DataOps Suite provides a powerful framework for automated governance and validation, ensuring that data remains accurate, complete, and compliant at all times.
In our previous discussion, we highlighted how Datagaps enhances metadata management, lineage tracking, and automation within Unity Catalog. This article takes the next step by diving into data quality assurance – a crucial component of enterprise-wide data governance.
By leveraging Datagaps Data Quality Monitor, organizations can implement automated validation strategies, reduce manual effort, and integrate real-time data quality scores into Unity Catalog for proactive governance. Let’s explore how these technologies work together to ensure high-quality, reliable data that drives better decision-making and compliance.
The Growing Need for Automated Data Quality Assurance
Modern enterprises manage vast amounts of structured and unstructured data across multiple platforms. Ensuring data accuracy, completeness, and consistency is no longer just a best practice – it’s a necessity for regulatory compliance and business intelligence.
Databricks Unity Catalog provides a centralized governance framework for managing metadata, access controls, and data lineage across an organization. By integrating with Datagaps Data Quality Monitor, enterprises can automate data validation, reduce errors, and gain deeper insights into data health and integrity.
6 Key Data Quality Dimensions

Effective data quality management revolves around six fundamental dimensions:
Accuracy – Ensuring data reflects real-world values without discrepancies.
Completeness – Verifying that all required fields and records are present.
Consistency – Maintaining uniformity across multiple data sources and systems.
Timeliness – Ensuring data is up-to-date and available when needed.
Uniqueness – Eliminating duplicate records and redundant data entries.
Validity – Enforcing compliance with defined formats, business rules, and constraints.
By addressing these dimensions, organizations can improve the trustworthiness of their data assets, enhance AI/ML outcomes, and comply with industry regulations.
Automating Data Quality Validation with White-Box and Black-Box Testing
Ensuring data integrity at scale requires a systematic approach to validation. Two widely used methodologies are:
1. White-Box Testing
Examines internal data transformations, lineage, and business rules.
Ensures that every step in the ETL (Extract, Transform, Load) process adheres to defined standards.
Provides deeper insights into data processing logic to catch issues at the source.
2. Black-Box Testing
Focuses on output validation by comparing actual results against expected benchmarks.
Useful for detecting anomalies, missing records, and schema mismatches.
Works well for regulatory compliance and end-to-end data pipeline testing.
A hybrid approach combining both techniques ensures robust validation and proactive anomaly detection.
How Unity Catalog and Datagaps Data Quality Monitor Work Together
1. Unified Governance and Automated Validation
Databricks Unity Catalog centralizes metadata management, access control, and lineage tracking.
Datagaps Data Quality Monitor extends these capabilities with automated quality checks, reducing manual efforts.
2. Mapping Manager Utility: Simplifying Test Case Automation
One of the standout features of Datagaps Data Quality Monitor is the Mapping Manager Utility, which:
Extracts mapping configurations from Databricks Unity Catalog.
Automatically generates white-box and black-box test cases.
Reduces the need for manual intervention, increasing efficiency and scalability.
3. Real-Time Data Quality Scores for Proactive Governance
After test execution, a data quality score is generated.
These scores are seamlessly integrated into Databricks Unity Catalog, allowing real-time monitoring.
Organizations can visualize data quality insights through dashboards and take corrective actions before issues impact business operations.
Key Use Cases
ETL and Data Pipeline Validation – Ensuring data transformations adhere to defined business rules.
Regulatory Compliance and Audit Readiness – Mitigating risks associated with inaccurate reporting.
Enterprise Data Lakehouse Governance – Enhancing consistency across distributed datasets.
AI/ML Data Preprocessing – Ensuring clean, high-quality data for better model performance.
Automated Data Quality Checks – Reducing manual data validation efforts for faster, more reliable insights.
Scalability for Large Datasets – Efficiently managing high-volume, high-velocity enterprise data.
Faster QA Cycles – Automating test case execution for rapid turnaround.
Lower Operational Resources – Reducing human intervention, saving time and resources.
The Business Impact: Why This Integration Matters
Enhanced Automation – Eliminates manual quality checks and increases efficiency.
Real-Time Monitoring – Provides instant visibility into data quality metrics.
Stronger Compliance – Supports industry standards and regulations effortlessly.
Scalability – Designed for large-scale, complex data ecosystems.
Cost Efficiency – Reduces operational overhead and improves ROI on data management initiatives.
Ensuring data quality at scale requires a combination of automated governance, real-time monitoring, and seamless integration. The connection between Databricks Unity Catalog and Datagaps Data Quality Monitor provides a comprehensive solution to achieve this goal.
With automated test case generation, continuous data validation, and integrated governance, organizations can ensure their data is always accurate, complete, and compliant—laying the foundation for data-driven decision-making and regulatory confidence.
0 notes
Text
𝐃𝐚𝐭𝐚 𝐋𝐚𝐤𝐞𝐡𝐨𝐮𝐬𝐞 𝐯𝐬 𝐖𝐚𝐫𝐞𝐡𝐨𝐮𝐬𝐞 𝐢𝐧 2025
Data warehouses and lakehouses are battling for dominance in modern analytics. In this video, we’ll compare performance, cost, scalability, and use cases—using real-world data and expert insights.
Whether you're a data engineer, CTO, or just data-curious, this breakdown will help you choose the right architecture for 2025 and beyond.
Watch https://youtu.be/lsBGbW7ExD4
Drop your stack! Are you Team Warehouse, Team Lakehouse, or Team Hybrid? Let's talk strategy
#DataLakehouse#DataWarehouse#Databricks#Snowflake#ModernDataStack#AnalyticsArchitecture#BigData#DataEngineering#CloudComputing#DataOps#AIAnalytics#RealTimeAnalytics#SQL#BusinessIntelligence
1 note
·
View note
Text
Artifactory Fundamental Tutorial - 2023 | Part - 01/04
scmgalaxy empowers IT professionals with hands-on training, certifications, and expert mentorship, blending real-world experience with industry insights. We offer training, certification, guidance, and consulting for DevOps, Big Data, Cloud, dataops, AiOps, MLOps, DevSecOps, GitOps, DataOps, ITOps, SysOps, SecOps, ModelOps, NoOps, FinOps, XOps, BizDevOps, CloudOps, SRE and PlatformOps. 🔔 Don't Miss Out! Hit Subscribe and Ring the Bell! 🔔 👉 Subscribe Now
0 notes
Text
Creole Studios Launches Comprehensive Data Engineering Services with Azure Databricks Partnership
Creole Studios launches comprehensive data engineering services in partnership with Azure Databricks, empowering businesses to unlock insights, ensure compliance, and drive growth with expert solutions. From data strategy and consulting to advanced analytics and data quality assurance, Creole Studios offers tailored services designed to maximize the potential of your data.
#DataEngineering#AzureDatabricks#DataStrategy#AdvancedAnalytics#BigData#DataQuality#DataOps#DataGovernance#Compliance#BusinessInsights#CreoleStudios
0 notes