#dataops suite
Explore tagged Tumblr posts
Text
In today’s world, data is considered one of the most valuable assets any business can have. However, to truly unlock the power of data, it’s not enough to simply collect it—organizations need to ensure that the data they are working with is accurate, consistent, and reliable. That’s where Data Quality Observability comes in.
Data Quality Observability is the ability to monitor, understand, and proactively manage the state of data across an entire ecosystem. With the growing complexity of data pipelines and the increasing reliance on data-driven decisions, organizations can no longer afford to ignore the health of their data. Data quality observability helps businesses identify issues before they impact operations, making it a critical part of any data strategy.
#datagaps#data quality#data#dataops#dataops suite#data quality observability#QA tester#Data Analysts#BI Experts
0 notes
Text
Simplifying Complex Data Operations with Smart Tools: Match Data Pro LLC Leading the Charge
In the data-driven economy, businesses are increasingly relying on accurate, actionable, and streamlined data to make informed decisions. But as the volume of data grows, so do the challenges: mismatched entries, inconsistent formats, manual data handling, and disconnected systems. That’s where Match Data Pro LLC steps in with robust, user-friendly solutions built to simplify the most complex data tasks.
From intuitive point-and-click data tools to enterprise-ready on-premise data software, Match Data Pro LLC offers a full suite of data ops software that helps businesses regain control of their data environments. Let’s explore how our tools are transforming data workflows and improving business intelligence across industries.
The Challenge of Mismatched Data
Modern businesses often operate across multiple platforms — CRMs, ERPs, marketing suites, accounting software, and more. With so many systems exchanging data, inconsistencies and mismatches are inevitable.
Common mismatched data issues include:
Duplicated records with minor variations
Inconsistent formatting across platforms
Incomplete or outdated entries
Data schema conflicts
These mismatches don’t just clutter your systems — they lead to flawed analytics, poor customer experiences, and inefficient operations. Match Data Pro LLC offers specialized mismatched data solutions that identify, resolve, and prevent these inconsistencies before they impact your business.
Mismatched Data Solutions That Actually Work
Our intelligent matching algorithms use fuzzy logic, pattern recognition, and customizable rules to identify mismatches across large datasets — whether it's customer records, product inventories, or financial transactions.
With our solutions, you can:
Detect and correct field-level mismatches
Merge records with varying structures
Align data formats across multiple systems
Automate reconciliation and cleanup processes
Whether your data is siloed in spreadsheets or flowing through APIs, Match Data Pro LLC helps you achieve consistency and reliability.
Empowering Users with Point-and-Click Data Tools
Not every business has a dedicated IT or data science team. That’s why Match Data Pro LLC designed point-and-click data tools — intuitive interfaces that empower non-technical users to manage, match, and clean data without writing a single line of code.
With our user-friendly dashboard, you can:
Drag and drop datasets for instant processing
Match records with customizable logic
Filter, group, and sort data visually
Schedule automated data operations
Generate real-time reports with one click
These tools are perfect for marketing teams, sales professionals, analysts, and operations managers who need quick results without technical overhead.
Optimize Workflow with Data Ops Software
DataOps, or data operations, is the practice of automating, monitoring, and improving the data pipeline across your organization. Match Data Pro LLC offers scalable data ops software that bridges the gap between IT and business, ensuring that clean, accurate data flows freely across systems.
Our DataOps platform supports:
Data ingestion and transformation
Real-time validation and matching
Workflow automation
Custom pipelines with REST API integration
End-to-end visibility into data flow
By implementing a robust DataOps framework, organizations can break down silos, accelerate decision-making, and reduce the time from data collection to business action.
On-Premise Data Software for Total Control
While cloud-based solutions offer flexibility, some businesses — especially in finance, healthcare, and government — require strict control over their data infrastructure. For these clients, Match Data Pro LLC provides secure, customizable on-premise data software.
With our on-premise solution, you get:
Full ownership of your data and environment
Greater compliance with regulatory standards (GDPR, HIPAA, etc.)
No dependency on third-party cloud providers
Seamless integration with legacy systems
Offline capabilities for remote or secure locations
Whether you're managing sensitive customer data or maintaining a private data warehouse, our on-premise offerings ensure peace of mind and operational integrity.
How Match Data Pro LLC Delivers Value
We understand that every organization’s data landscape is unique. That’s why we offer flexible configurations, expert support, and scalable features that grow with your business.
Key benefits of our platform:
Accurate data matching and cleaning
Automation that saves hours of manual effort
Tools accessible to both technical and non-technical users
API integration for seamless system connectivity
On-premise and cloud deployment options
Whether you’re a startup seeking better customer segmentation or a multinational enterprise trying to unify datasets across geographies, Match Data Pro LLC has a solution that fits.
Real-World Use Cases
Marketing: Clean up and deduplicate lead lists from multiple sources using point-and-click tools
Finance: Reconcile transactions across multiple ledgers with automated workflows
Retail: Align product data across warehouses, stores, and e-commerce platforms
Healthcare: Match patient records across systems while complying with data privacy regulations
Government: Maintain accurate citizen records with secure on-premise deployment
Our flexible software supports use cases across every industry — because clean, reliable data is a universal need.
Match Data Pro LLC: Your Data, Unified and Simplified
In a world driven by data, the ability to unify and streamline your information is what sets top-performing companies apart. Match Data Pro LLC provides the tools, support, and infrastructure to turn messy, mismatched datasets into clean, actionable intelligence.
With point-and-click data tools, mismatched data solutions, data ops software, and on-premise data software, we give you the power to take control of your data — and your business future.
0 notes
Text
CloudifyOps: Unlocking Cloud Potential
Empowering businesses with a comprehensive suite of cloud and DevOps services, driving innovation and seamless digital transformation.
We help organizations become more agile and innovative by implementing cloud security best practices and managing IT operations 24/7.
CloudifyOps  services include Cloud Migration, FinOps, DevSecOps, Application Modernization, Site Reliability Engineering, and DataOps, ensuring efficient and secure cloud operations.
An end-to-end technology lifecycle partner, we offer in-house solutions such as Cloud Migration Factory, Well-Architected, and DevOptymize frameworks, that accelerate the process of building secure, scalable, and high-performing cloud infrastructure.Â
Whether you’re looking to migrate to the cloud or optimize your existing infrastructure, CloudifyOps has you covered.
Ready to elevate your business with powerful cloud solutions? Reach out to CloudifyOps today at [email protected] or schedule a meeting with us now!
0 notes
Text
Conquer Your Data Challenges with Web Age Solutions' Data Engineering Courses
Organizations are constantly bombarded with information. The ability to effectively collect, store, transform, and analyze this information is crucial for gaining valuable insights and making informed decisions. This is where data engineering comes in. Data engineers are the architects of the data pipelines that power these insights.
Web Age Solutions empowers you to build a skilled data engineering team with our comprehensive suite of data engineering courses. Led by industry experts, our courses provide a blend of theoretical knowledge and practical application, equipping you with the skills to tackle critical data challenges head-on.
What You'll Gain from Our Data Engineering Courses:
Master Data Wrangling with Python: The "Data Engineering with Python" course delves into the practical application of Python, a widely used programming language in data engineering. You'll gain proficiency in popular Python libraries like NumPy, pandas, and Matplotlib, enabling you to manipulate, analyze, and visualize data effectively.
Bridge the Gap for Data-Driven Leaders: The "Data Engineering for Managers" course is designed for business leaders who want to understand the fundamentals of data engineering and its role in driving business outcomes. This course equips you to make informed decisions about data infrastructure and effectively communicate with your data engineering team.
Harness the Power of Operational Data Analytics: "Operational Data Analytics with Splunk" introduces you to the Splunk platform, a powerful tool for analyzing machine-generated data. Through hands-on exercises, you'll learn to onboard and forward data, monitor systems in real-time, and create informative data visualizations.
Embrace Cloud-Based Data Processing: "Cloud Data Engineering with NiFi on AWS or GCP" equips you with the skills to leverage Apache NiFi, a visual programming tool, for data processing on cloud platforms like AWS and GCP. This course blends theoretical concepts with practical guidance to help you automate data movement and transformation within cloud environments.
Build a Solid Foundation in Data Engineering: "Data Engineering, ETL and DataOps" provides a comprehensive introduction to core data engineering principles. You'll gain hands-on experience with ETL (Extract, Transform, Load) processes, data manipulation with pandas, data visualization using Python, and essential data operations (DataOps) practices. Additionally, the course covers Apache Spark and Spark SQL, further equipping you for success in data engineering and data analytics roles.
Investing in Your Data Engineering Skills:
By empowering your team with data engineering expertise, you unlock a wealth of benefits for your organization. Data engineers can help you:
Optimize Risk and Performance: Analyze data to identify potential risks and opportunities, allowing for proactive decision-making.
Enhance Data Quality: Implement processes and tools to ensure data accuracy and consistency, leading to reliable insights.
Forecast with Precision: Leverage data to predict future trends and customer behavior, enabling informed business strategies.
Streamline Business Processes: Automate data-driven workflows, improving efficiency and productivity across your organization.
Web Age Solutions: Your Partner in Data Engineering Education
Our data engineering courses are designed to cater to a wide range of learners, from beginners seeking a foundational understanding to experienced professionals looking to refine their skillset. We offer flexible delivery options, including on-site and instructor-led virtual classes, to accommodate your team's learning preferences.
Ready to unlock the power of your data? Browse our complete course catalog or contact Web Age Solutions today to discuss your data engineering training needs. With expert-led instruction and a practical approach, we will equip your team with the skills to transform your data into actionable insights. Â Â
For more information visit: https://www.webagesolutions.com/courses/data-engineering-training
0 notes
Text
Technology Acceleration
The 6 pillars of data engineering needed for a 360-degree view of your business

Countless organizations have completed a digital transformation within the past handful of years, but this has led to many of them pulling in more raw data than they know what to do with.
This is a good problem to have, but a problem nonetheless.
Business leaders know that data is one of the most valuable resources an organization can have. But in order to leverage that data, you need the proper architecture, tools, and processes to make it usable for analytics purposes and to identify actionable insights that drive business value.
That’s where the six pillars of data engineering come into play.
This article will focus on the six pillars of data engineering that are needed to leverage your organization’s data effectively, as well as some of the tools that can help you in your data endeavors.
With these pillars firmly in place, you’ll be able to transform that data into a crystal clear picture of what can be improved within your organization and what opportunities can be taken advantage of to gain a competitive advantage.
1. Data Ingestion
It’s likely your business has a multitude of data streams that originate from different sources — from databases, applications, and IoT devices, for example. Before you can analyze that data and obtain actionable insights for your business, you need a way of gathering all that data in one place.
It needs to be ingested.
Data ingestion is the process of transporting data from those sources to a unified environment, such as a cloud data lake.
But more needs to be done . . .
Tools: Apache Kafka/PyKafka, AWS Kinesis/Kinesis Data Streams/Kinesis Firehose, Spark Streaming, RabbitMQ, Google PubSub, Azure Data Bricks
2. Data Integration
Once the data is transported to a unified environment, data integration takes it a step further.
Data integration ensures that all data that was ingested is compatible with each other in order to be used for downstream applications or analytics.
There are different approaches when it comes to data integration, and they both have their strengths and weaknesses.
One approach, ETL (extract, transform, load), is the more traditional one and is ideal when security, quality, and compliance are concerns.
ELT (extract, load, transform) has become an increasingly popular method of data integration with the adoption of cloud and agile methodologies. This approach is best suited for large volumes of unstructured data.
But there’s also another integration method, which is a hybrid of the two: ETLT (extract, transform, load, transform). This method aims to get the best of both worlds of ETL and ELT.
Tools: Spark – PySpark, Flink – PyFlink, AWS Glue, Apache Beam, GCP Data Flow, Apache Hive, Azure Data Factory
3. Data Modeling
The third pillar of data engineering is data modeling. It’s essentially a way to visualize the data that was pulled so that it’s easier to see the relationships between data sources and how data flows between those sources.
Data modeling is integral to fully understand your data and to support your business’s analytical needs.
Tools: Amazon Athena, Amazon Redshift, Google Cloud BigQuery, Azure Synapse Analytics, Azure Cosmos DB, MongoDB
4. Data Observability
Data observability provides users with visibility into the health and state of data. It can detect situations your team wasn’t aware of or even thought to plan for. With data observability, data teams can be alerted in real time in order to identify, troubleshoot, and resolve data issues, such as schema drift and duplicate values, before they have time to impact the business.
As different departments within an organization often need to make decisions based on the same data, it’s imperative that the data is accurate and reliable in order to inform those decisions and prevent costly errors and downtime.
Tools: AWS Wrangler, AWS Glue Databrew, Apache Airflow, Apache Sqoop
5. DataOps
The rise of Big Data has necessitated yet another Ops in addition to DevOps: DataOps.
Emphasizing collaboration, DataOps is an agile-based method that bridges the gaps between data engineers, data scientists, and other stakeholders within the organization who rely on data. It brings together the tools, people, processes, and the data itself.
What DevOps did for software development is what DataOps is doing for organizations that want to be truly data driven.
The end goal of DataOps is to streamline the creation and maintenance of data pipelines, ensure the quality of that data for the applications that depend on it, and to create the most business value from that data.
Tools: AWS Wrangler, AWS Glue Databrew, Apache Airflow, Apache Sqoop
6. Data Delivery
We’ve reached the final pillar of data engineering — and the final output of a data pipeline. This is the “connect between data pipeline and data analytics.  Data delivery is where data pipelines meet data analytics. This pillar entails making the data available in specific ways so that the data can be consumed by downstream systems. Once the data is made available, it can be consumed and used for the three types of analytics that drive decision-making: descriptive (what’s happened), predictive (what could happen), and prescriptive analytics (what should happen).
Tools: Amazon Quicksight, Tableau, Microsoft Power BI, Graffana, Plotly, Matplotlib
Conclusion
With organizations across every industry becoming data driven and it becoming increasingly difficult to build and maintain data pipelines, it’s important to implement automated processes and tools that can make that data usable and more valuable.
What Relevantz Can Do for You
Relevantz helps enterprises get more from their data. With our data platform services, we build end-to-end data engineering pipelines — covering all six pillars of data engineering — as well as perform modernization, migration, and maintenance of those pipelines to keep the data flowing like it should.
0 notes
Text
Data Validation Testing
At a recent TDWI virtual summit on “Data Integration and Data Quality”, I attended a session titled “Continuous Data Validation: Five Best Practices” by Andrew Cardno.
In this session, Andrew Cardno, one of the adjunct faculty at TDWI talked about the importance of validating data from the whole to the part, which means that the metrics or total should be validated before reconciling the detailed data or drill-downs. For example, revenue totals by product type should be the same in Finance, CRM, and Reporting systems.
Attending this talk reminded me of a Data Warehouse project I worked on at one of the federal agencies. The source system was a Case Management system with a Data Warehouse for reporting. We noticed that one of the key metrics “Number of Cases by Case Type” yielded different results when queried on the source database, the data warehouse, and the reports. Such discrepancies undermine the trust in the reports and the underlying data. The reason for the mismatch can be an unwanted filter or wrong join or error during the ETL process.
When it comes to the federal agency this report is sent to congress and they have a congressional mandate to ensure that the numbers are correct. For other industries such as Healthcare and Financial, compliance requirements require the data to be consistent across multiple systems in the enterprise. It is essential to reconcile the metrics and the underlying data across various systems in the enterprise.
Andrew talks about two primary methods for performing Data Validation testing techniques to help instill trust in the data and analytics.
Glassbox Data Validation Testing
Blackbox Data Validation Testing
I will go over these Data Validation testing techniques in more detail below and explain how the Datagaps DataOps suite can help automate Data Validation testing.
#data validation#data validation testing#Glassbox Data Validation Testing#Blackbox Data Validation Testing
0 notes
Text
Schmarzo’s Big, Hairy, Audacious Data Analytics Predictions for 2020
Ah, it’s that time of year when everyone is making predictions about next year and extrapolating from the previous year’s trends to create logical, pragmatic predictions. I’ve already made my own predictions for 2020 in the article, “AI, Analytics, Machine Learning, Data Science, Deep Learning Technology Main Developments in 2019 and Key Trends for 2020,”where I predicted the following
Main 2019 Developments:
Growing "consumer proof points" with respect to AI integration into our everyday lives via smart phones, web sites, home devices and vehicles.
Formalization of DataOps category as an acknowledgment of the growing importance of the Data Engineering role.
Growing respect for the business potential of data science within the executive suite.
CIOs continue to struggle to deliver on the data monetization promise; Data Lake disillusionment leading to Data Lake "second surgeries."
Key 2020 Trends:
More real-world examples of industrial companies leveraging sensors, edge analytics and AI to create products that get more intelligent through usage; they appreciate, not depreciate, in value with usage.
Grandiose smart spaces projects continue to struggle to grow beyond initial pilots due to inability to deliver reasonable financial or operational impact.
Recession will drive chasm between "Have's" and "Have Not's" with respect to organizations that leverage data and analytics to drive meaningful business results.
But let’s have some fun with these 2020 predictions that are at the very edge of logical and pragmatic while offering a buzzkill perspective. We’ll create a new category of predictions: Big, Hairy, Audacious Predictions or BHAPs (a takeoff on Jim Collin’s famous BHAG writings) and sprinkle in some quotes from one of America’s most famous philosophers – Yogi Berra – to spice up the BHAPs! And I’ll even leave a place at the end for you to create your own 2020 BHAP because as Yogi Berra said, “The game isn't over until it's over”.
Schmarzo’s BHAPs
BHAP #1: Blockchain fuels definitive source of truth. Deep fakes that influence elections worldwide and lead to front-page grabbing headlines necessitates government’s dictating Blockchain as the only definitive and validated source of truth. Technology advances such as 5G and quantum computing underpin Blockchain’s capabilities. BHAP Buzzkill #1: Unfortunately, the government, driven by special interest groups, screws up the Blockchain definition (for fear of losing their ability to spy on their own citizens) and the potential of Blockchain to provide a definitive source of truth implodes on itself like a dying blackhole.
“I never said most of the things I said.” – Yogi Berra
BHAP #2: AI-driven autonomous entities become mainstream. Yes, Elon Musk is right in that one can create assets – vehicles, trains, compressors, turbines, etc. – that appreciate, not depreciate, in value the more that they are used. The more these assets are used, the more observations they collect and the smarter (and more valuable) they become. BHAP Buzzkill #2: Unfortunately, the AI function around which AI success is defined is so poorly defined by senior management consultants, that these assets end up becoming lazy, ineffective and finally unionize.
“90% of the game is half mental.” – Yogi Berra
BHAP #3: Â AI transforms the Data Management landscape. Data engineering is the biggest hindrance to leverage data science to derive and drive new sources of customer, product and operational value. In an effort to alleviate these pains, DataOps providers add Machine Learning automation to underpin and automate many data engineering tasks. BHAP Buzzkill #2: Unfortunately, Data Engineers quickly realize that these efforts threaten their job security and sabotage the Machine Learning algorithms in a way that actually increases the demand in data engineers. Data Scientists consequently end up working on research papers in hopes of landing tenure at local, prestigious university.
“'It's tough to make predictions, especially about the future'” – Yogi Berra
BHAP #4: Co-creation becomes the only differentiated and sustainable business model.  Co-creation occurs when two organizations from different but complementary backgrounds collaborate to create a solution that benefits both. Co-creation between technology and commercial companies become a fundamental business model in identifying, codifying and operationalizing new sources customer, product and operational value. BHAP Buzzkill #4: Unfortunately, greed will raise its ugly head and passive aggressive behaviors will emerge from those who aren’t so enthusiastic in sharing credit, or the cover of Fortune Magazine, for their success with others.
“In theory there is no difference between theory and practice. In practice there is.” – Yogi Berra
BHAP #5: Â AI success driven by grass root efforts, not management mandates. AI success will gain roots in companies where the rank-and-file, not senior management, are the driving factors. The rank-and-file will see the opportunity to liberate themselves from their daily drudgery by empowering AI devices that free them up for more value-added and creative work.
BHAP Buzzkill #5: Unfortunately, the rank-and-file try to push the AI initiative too far and AI actually replaces senior management. But the boring meetings that senior management is forced to endure drives the AI devices crazy, leading to them to self-destruct.
“I usually take a two-hour nap from 1 to 4.” – Yogi Berra
BHAP #6: Transition of SME tribal knowledge to AI algorithms fuels the next industrial renaissance. With millions of subject matter experts retiring across all industries with the resulting loss of the tribal knowledge necessary to keep operational running smoothly, organizations seek to capture, validate, codify (in AI algorithms) and operationalize this tribal knowledge, turning every Subject Matter Expert (engineer, technician, physician, nurse, teacher, lawyer, accountant) into the best Subject Matter Expert. BHAP Buzzkill #6: Unfortunately, the lack of health care in retirement forces many SME’s to unretire and put the AI algorithms out of work instead.
“You’ve got to be very careful if you don’t know where you are going, because you might not get there.” – Yogi Berra
BHAP #7:  GAAP introduces new financial rules for defining the financial value of data. The Accounting industry finally realizes the traditional accounting principles don’t work to define the value of big data and embrace economic principles to define the value of assets that never deplete, never wear out and can be used across an unlimited number of use cases. “Value in Use” wins out over “Value in Exchanges.” BHAP Buzzkill #7: Unfortunately, the value of data accounting principles is so complicated, that it takes an army of accountants to accurately make the value determination. The new law becomes known as the “Accountant Full-time Employment Act”.
“If the world was perfect, it wouldn’t be.” – Yogi Berra
BHAP #8:  Economics becomes Sexiest Job. As Gen Z starts to realize the unique characteristics of assets – like data and analytics – that appreciate, not depreciate, in value through usage and the associated collective learning, Economist replies Data Scientist as the Sexiest Job of the 21st Century. The “Economies of Learning” are truly more powerful than the “Economies of Scale” in knowledge-based industries. BHAP Buzzkill #8: Unfortunately, there graduates soon learn that companies pay for actual work, and most of these economics majors take jobs as coffee baristas (the modern bartender gig).
“It's like Deja vu all over again.” – Yogi Berra
BHAP #9: William Schmarzo wins the Economics Nobel Prize.  After his ground-breaking research paper on the Economic Value of Data at the University of San Francisco, followed up with this industry-leading perspective on "Schmarzo Digital Asset Valuation Theorem," the Nobel Prize committee has no choice but to award William Schmarzo the Nobel Prize in economics. BHAP Buzzkill #9: Unfortunately, the award is made posthumously…
Readmore:Â Does Big Data Impact Business Mobile App Development?
0 notes
Text
#Tableau#Tableau Reporting#Data Validation#DataOps Suite#Datagaps#Automating Tableau Reports Validation
0 notes
Text
How Databricks Unity Catalog and Datagaps Automate Governance and ValidationÂ

Data quality is the backbone of accurate analytics, regulatory compliance, and efficient business operations. As organizations scale their data ecosystems, maintaining high data integrity becomes more challenging.
The seamless integration between Databricks Unity Catalog and Datagaps DataOps Suite provides a powerful framework for automated governance and validation, ensuring that data remains accurate, complete, and compliant at all times.
In our previous discussion, we highlighted how Datagaps enhances metadata management, lineage tracking, and automation within Unity Catalog. This article takes the next step by diving into data quality assurance – a crucial component of enterprise-wide data governance.Â
By leveraging Datagaps Data Quality Monitor, organizations can implement automated validation strategies, reduce manual effort, and integrate real-time data quality scores into Unity Catalog for proactive governance. Let’s explore how these technologies work together to ensure high-quality, reliable data that drives better decision-making and compliance.
The Growing Need for Automated Data Quality Assurance
Modern enterprises manage vast amounts of structured and unstructured data across multiple platforms. Ensuring data accuracy, completeness, and consistency is no longer just a best practice – it’s a necessity for regulatory compliance and business intelligence.Â
Databricks Unity Catalog provides a centralized governance framework for managing metadata, access controls, and data lineage across an organization. By integrating with Datagaps Data Quality Monitor, enterprises can automate data validation, reduce errors, and gain deeper insights into data health and integrity.Â
6 Key Data Quality Dimensions

Effective data quality management revolves around six fundamental dimensions:Â
Accuracy – Ensuring data reflects real-world values without discrepancies.Â
Completeness – Verifying that all required fields and records are present.Â
Consistency – Maintaining uniformity across multiple data sources and systems.Â
Timeliness – Ensuring data is up-to-date and available when needed.Â
Uniqueness – Eliminating duplicate records and redundant data entries.Â
Validity – Enforcing compliance with defined formats, business rules, and constraints.Â
By addressing these dimensions, organizations can improve the trustworthiness of their data assets, enhance AI/ML outcomes, and comply with industry regulations.Â
Automating Data Quality Validation with White-Box and Black-Box Testing
Ensuring data integrity at scale requires a systematic approach to validation. Two widely used methodologies are:Â
1. White-Box Testing
Examines internal data transformations, lineage, and business rules.Â
Ensures that every step in the ETL (Extract, Transform, Load) process adheres to defined standards.Â
Provides deeper insights into data processing logic to catch issues at the source.
2. Black-Box Testing
Focuses on output validation by comparing actual results against expected benchmarks.Â
Useful for detecting anomalies, missing records, and schema mismatches.Â
Works well for regulatory compliance and end-to-end data pipeline testing.Â
A hybrid approach combining both techniques ensures robust validation and proactive anomaly detection.Â
How Unity Catalog and Datagaps Data Quality Monitor Work Together
1. Unified Governance and Automated Validation
Databricks Unity Catalog centralizes metadata management, access control, and lineage tracking.Â
Datagaps Data Quality Monitor extends these capabilities with automated quality checks, reducing manual efforts.Â
2. Mapping Manager Utility: Simplifying Test Case Automation
One of the standout features of Datagaps Data Quality Monitor is the Mapping Manager Utility, which:Â
Extracts mapping configurations from Databricks Unity Catalog.Â
Automatically generates white-box and black-box test cases.Â
Reduces the need for manual intervention, increasing efficiency and scalability.Â
3. Real-Time Data Quality Scores for Proactive Governance
After test execution, a data quality score is generated.Â
These scores are seamlessly integrated into Databricks Unity Catalog, allowing real-time monitoring.Â
Organizations can visualize data quality insights through dashboards and take corrective actions before issues impact business operations.Â
Key Use Cases
ETL and Data Pipeline Validation – Ensuring data transformations adhere to defined business rules.Â
Regulatory Compliance and Audit Readiness – Mitigating risks associated with inaccurate reporting.
Enterprise Data Lakehouse Governance – Enhancing consistency across distributed datasets.
AI/ML Data Preprocessing – Ensuring clean, high-quality data for better model performance.Â
Automated Data Quality Checks – Reducing manual data validation efforts for faster, more reliable insights.Â
Scalability for Large Datasets – Efficiently managing high-volume, high-velocity enterprise data.Â
Faster QA Cycles – Automating test case execution for rapid turnaround.
Lower Operational Resources – Reducing human intervention, saving time and resources.Â
The Business Impact: Why This Integration Matters
Enhanced Automation – Eliminates manual quality checks and increases efficiency.Â
Real-Time Monitoring – Provides instant visibility into data quality metrics.Â
Stronger Compliance – Supports industry standards and regulations effortlessly.Â
Scalability – Designed for large-scale, complex data ecosystems.Â
Cost Efficiency – Reduces operational overhead and improves ROI on data management initiatives.Â
Ensuring data quality at scale requires a combination of automated governance, real-time monitoring, and seamless integration. The connection between Databricks Unity Catalog and Datagaps Data Quality Monitor provides a comprehensive solution to achieve this goal.
With automated test case generation, continuous data validation, and integrated governance, organizations can ensure their data is always accurate, complete, and compliant—laying the foundation for data-driven decision-making and regulatory confidence.
0 notes
Text
#dataquality#Databricks#cloud data testing#DataOps#Datagaps#Catalog#Unity Catalog#Datagaps BI Validator
0 notes
Text
Optimizing Data Management with Mismatched Data Solutions and Data Ops Software
In the fast-paced world of data management, organizations struggle with data inconsistencies, inefficient processing, and mismatched data issues that hinder operations. Businesses need robust mismatched data solutions and advanced data ops software to optimize workflows, ensure data accuracy, and improve decision-making. Match Data Pro LLC provides cutting-edge solutions to handle data challenges effectively, offering seamless integration and automation capabilities for streamlined data processing.
Understanding Mismatched Data Challenges
Data mismatches occur when datasets fail to align correctly due to discrepancies in formats, missing values, or duplicate entries. These inconsistencies can result in poor analytics, incorrect reporting, and flawed decision-making. Organizations dealing with vast amounts of information require mismatched data solutions that can identify, correct, and prevent errors efficiently.
Common Causes of Mismatched Data
Inconsistent Formatting – Variations in date formats, numerical representation, and naming conventions.
Duplicate Records – Repetitive entries causing redundancy and inaccuracy.
Data Entry Errors – Human errors leading to incorrect or incomplete data.
Integration Issues – Discrepancies arising when merging data from multiple sources.
Data Decay – Outdated or obsolete information affecting relevance and accuracy.
The Role of Mismatched Data Solutions
To overcome these challenges, businesses rely on specialized mismatched data solutionsthat offer:
Automated Data Cleansing – Identifying and correcting errors with minimal manual intervention.
Data Matching Algorithms – Ensuring accurate record linkage across different datasets.
Standardization Processes – Unifying data formats to maintain consistency.
Data Validation Techniques – Ensuring the reliability and completeness of datasets.
Match Data Pro LLC delivers state-of-the-art mismatched data solutions, providing businesses with powerful tools to enhance data quality and integrity.
Data Ops Software: Enhancing Operational Efficiency
Data operations, commonly known as DataOps, involve the automation, monitoring, and governance of data workflows. Data ops software plays a crucial role in optimizing data pipelines, reducing errors, and accelerating data-driven decision-making.
Key Features of Data Ops Software
Automated Workflow Orchestration – Streamlining data processing tasks without manual intervention.
Real-Time Monitoring & Alerts – Identifying anomalies and performance issues promptly.
Data Integration Capabilities – Seamlessly connecting various data sources.
Scalability & Flexibility – Adapting to growing data demands.
Security & Compliance – Ensuring data governance and regulatory compliance.
Benefits of Implementing Data Ops Software
Businesses leveraging data ops software gain several advantages, including:
Improved Data Accuracy – Ensuring reliable and consistent datasets.
Increased Productivity – Reducing time spent on manual data processing.
Faster Decision-Making – Providing real-time insights for strategic planning.
Enhanced Collaboration – Facilitating seamless communication between data teams.
Cost Efficiency – Reducing expenses associated with data errors and inefficiencies.
Match Data Pro LLC: Your Trusted Partner for Data Management
As a leading provider of mismatched data solutions and data ops software, Match Data Pro LLC helps businesses streamline data operations, reduce mismatches, and enhance efficiency. Their comprehensive solutions cater to organizations of all sizes, ensuring seamless data management through:
On-Premise & Cloud-Based Solutions – Flexible deployment options to suit business needs.
Bulk Data Processing Capabilities – Handling large datasets efficiently.
Point-and-Click Data Tools – User-friendly interfaces for simplified data management.
REST API Integration – Seamless connectivity with existing systems.
Automated Data Scheduling & Pipelines – Enabling end-to-end automation.
Conclusion
Data inconsistencies and inefficiencies can severely impact business operations. Implementing robust mismatched data solutions and leveraging data ops software can enhance data accuracy, streamline workflows, and boost decision-making. Match Data Pro LLC offers industry-leading solutions that empower businesses with automated, scalable, and efficient data management tools.
0 notes
Text
Automating Tableau Reports Validation: The Easy Path to Trusted InsightsÂ
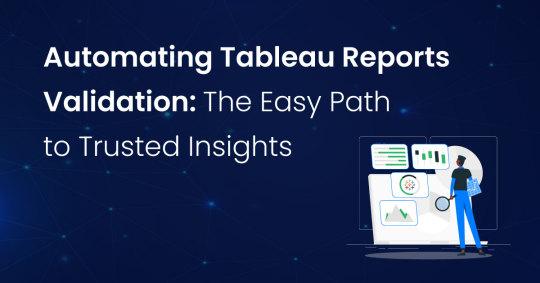
Automating Tableau Reports Validation is essential to ensure data accuracy, consistency, and reliability across multiple scenarios. Manual validation can be time-consuming and prone to human error, especially when dealing with complex dashboards and large datasets. By leveraging automation, organizations can streamline the validation process, quickly detect discrepancies, and enhance overall data integrity.
Going ahead, we’ll explore automation of Tableau reports validation and how it is done.
Importance of Automating Tableau Reports Validation
Automating Tableau report validation provides several benefits, ensuring accuracy, efficiency, and reliability in BI reporting.
Automating the reports validation reduces the time and effort, which allows analysts to focus on insights rather than troubleshooting the errors
Automation prevents data discrepancies and ensures all reports are pulling in consistent data
Many Organizations deal with high volumes of reports and dashboards. It is difficult to manually validate each report. Automating the reports validation becomes critical to maintain efficiency.
Organizations update their Tableau dashboards very frequently, sometimes daily. On automating the reports validation process, a direct comparison is made between the previous and current data to detect changes or discrepancies. This ensures metrics remain consistent after each data refresh.
BI Validator simplifies BI testing by providing a platform for automated BI report testing. It enables seamless regression, stress, and performance testing, making the process faster and more reliable.
Tableau reports to Database data comparison ensures that the records from the source data are reflected accurately in the visuals of Tableau reports.
This validation process extracts data from Tableau report visuals and compares it with SQL Server, Oracle, Snowflake, or other databases. Datagaps DataOps Suite BI Validator streamlines this by pulling report data, applying transformations, and verifying consistency through automated row-by-row and aggregate comparisons (e.g., counts, sums, averages).
The errors detected usually identify missing, duplicate or mismatched records.
Automation ensures these issues are caught early, reducing manual effort and improving trust in reporting.
Tableau Regression
In the DataOps suite, Regression testing is done by comparing the benchmarked version of tableau report with the live version of the report through Tableau Regression component.
This Tableau regression component can be very useful for automating the testing of Tableau reports or Dashboards during in-place upgrades or changes.
A diagram of a process AI-generated content may be incorrect.
Tableau Upgrade
Tableau Upgrade Component in BI validator helps in automated report testing by comparing the same or different reports of same or different Tableau sources.
The comparison is done in the same manner as regression testing where the differences between the reports can be pointed out both in terms of text as well as appearance.
Generate BI DataFlows is a handy and convenient feature provided by Datagaps DataOps suite to generate multiple dataflows at once for Business Intelligence components like Tableau.
Generate BI DataFlows feature is beneficial in migration scenarios as it enables efficient data comparison between the original and migrated platforms and supports the validations like BI source, Regression and Upgrade. By generating multiple dataflows based on selected reports, users can quickly detect discrepancies or inconsistencies that may arise during the migration process, ensuring data integrity and accuracy while minimizing potential errors. Furthermore, when dealing with a large volume of reports, this feature speeds up the validation process, minimizes manual effort, and improves overall efficiency in detecting and resolving inconsistencies.
As seen from the image, the wizard starts by generating the Dataflow details. The connection details like the engine, validation type, Source-Data Source and Target-Data Source are to be provided by users.
Note: BI source validation and Regression validation types do not prompt for Target-Data source
Let’s take a closer look at the steps involved in “Generate BI Dataflows”
Reports
The Reports section prompts users to select pages from the required reports in the validation process. For Data Compare validation and Upgrade Validation, both source and target pages will be required. For other cases, only the source page will be needed.
Here is a sample screenshot of the extraction of source and target pages from the source and target report respectively
Visual Mapping and Column Mapping (only in Data Compare Validation)
The "Visual Mapping" section allows users to load and compare source and target pages and then establish connections between corresponding tables.
It consists of three sections namely Source Page, Target Page, and Mapping.
In the source page and target page, respective Tableau worksheets are loaded and on selecting the worksheets option, users can preview the data.
After loading the source and target pages, in the mapping section, the dataset columns of source and target will be automatically mapped for each mapping.
After Visual Mapping, the "Column Mapping" section displays the columns of the source dataset and target dataset that were selected for the data comparison. It provides a count of the number of dataset columns that are mapped and unmapped in the "Mapped" and "Unmapped" tabs respectively.
Filters (for the rest of the validation types)
The filters section enables users to apply the filters and parameters on the reports to help in validating them. These filters can either be applied and selected directly through reports or they can be parameterized as well.
Options section varies depending on the type of validation selected by the user. Options section is the pre final stage of generating the flows where some of the advanced options and comparison options are prompted to be selected as per the liking of the user to get the results as they like.
Here’s a sample screenshot of options section before generating the dataflows
This screenshot indicates report to report comparison options to be selected.
Generate section helps to generate multiple dataflows with the selected type of validation depending on the number of selected workbooks for tableau.
The above screenshot indicates that four dataflows are set to be generated on clicking the Generate BI Dataflows button. These dataflows are the same type of validation (Tableau Regression Validation in this case)
Stress Test Plan
To automate the stress testing and performance testing of Tableau Reports, Datagaps DataOps suite BI Validator comes with a component called Stress Test Plan to simulate the number of users actively accessing the reports to analyze how Tableau reports and dashboards perform under heavy load. Results of the stress test plan can be used to point out performance issues, optimize data models and queries to ensure the robustness of the Tableau environment to handle heavy usage patterns. Stress Test Plan allows users to perform the stress testing for multiple views from multiple workbooks at once enabling the flexibility and automation to check for performance bottlenecks of Tableau reports.
For more information on Stress Test Plan, check out “Tableau Performance Testing”.
Integration with CI/CD tools and Pipelines
In addition to these features, DataOps Suite comes with other interesting features like application in built pipelines where the set of Tableau BI dataflows can be run automatically in a certain order either in sequence or parallel.
Also, there’s an inbuilt scheduler in the application where the users can schedule the run of these pipelines involving these BI dataflows well in advance. The jobs can be scheduled to run once or repeatedly as well.
Achieve the seamless and automated Tableau report validation with the advanced capabilities of Datagaps DataOps Suite BI Validator.
0 notes
Text
The Ultimate Tableau Dashboard Testing Checklist

Ensuring the quality of a Tableau dashboard goes beyond building. It requires thorough testing to validate its reliability and usability. This tableau dashboard testing checklist focuses on essential aspects like verifying data accuracy, evaluating functionality, security testing to protect sensitive data, stress testing for performance under load, and visual testing to maintain clarity and design standards.
Aspects Involved in Testing the Tableau Dashboard

Testing Data Sources:
Ensure the dashboard is connected to the correct data sources and that credentials are set up properly.
Checking Data Accuracy:
1. Check whether the source data is reflected in the dashboard. This involves cross-checking the data on the dashboard with the data from the sources.
2. Verify that the calculated fields, aggregates, and measures are as expected.
Functionality Testing:
1. Report or dashboard design check.
2. Filters and parameters testing to see if they work as expected and do not display incorrect data. Also, if the dynamic changes to data visuals are applied and reflected.
3. Drilldown reports checking.
4. Ease of navigation, interactivity, and responsiveness in terms of usability.
Security Testing:
1. To check the security for report access and Row Level Security Permissions.
2. Integration of Single Sign On (SSO) security.
3. Multi-factor authentication.
Regression Testing:
Any change to the BI Dashboard/Model can impact the existing reports. It is important to perform regression testing so that after updates or modifications, the data/visuals shown in the dashboard remain the same before and after the changes.
youtube
Stress Testing:
To test the load time, run time, filter application time, and to simulate the access of reports and user behavior.
Visual Testing:
To check alignments, layouts, consistencies in terms of texts, visuals, or images to ensure all the elements are properly aligned.
How Does DataOps Suite BI Validator Enable Testing of Tableau Dashboards?

BI Validator is a no-code testing tool to automate the regression, stress, and functional testing of Tableau reports and dashboards.Â
Checking Data Accuracy:
DataOps Suite allows users to validate the data from the visuals of the report to be compared to the source databases. On connecting to Tableau and selecting a report, the datasets underlying the visuals of the reports are accessible through the suite as well. Each Visual has its own dataset, which can be compared to a source database used to build the tableau report.
This is possible with the help of the Data Compare component in the suite, which can compare the aggregated data from the databases and the datasets of the visuals. The differences are captured, thus allowing users to check the data accuracy between the reports and databases.
Functionality Testing and Visual Testing:
Once the connection is established, the users can access the reports and the exact workspace to work with. The specific workbook/report is accessible. The report will be loaded without any changes, and the visuals and filters of the report can be accessed from the BI Validator itself, thus verifying the responsiveness of the visuals and filters and verifying whether the dynamic changes are reflected.
The BI Validator comes with the Tableau Upgrade component to compare two reports, which can be the same or different from one or different data sources.
A common use case is the comparison of views and worksheets across multiple environments. Comparison can be done in terms of both text and appearance, where the differences are captured and pointed out wherever mismatch occurs in both reports. Also, BI Validator allows the differences in the filters of both reports to be pointed out on enabling “Capture filters after applying.”.
youtube
Security Testing:
BI Validator connects with Tableau Testing through direct trust authentication, default authentication, or personal access token, where the users must provide their respective Tableau credentials, secret key, and secret ID (in direct trust connection) and the necessary web login commands (for default or personal access token connection). Thus, ensuring the authentication is secure so that only the users with valid credentials are accessing their reports to be validated.
BI Validator restricts the users from downloading the testing results when it comes to BI Reports to prevent the sensitive information from being downloaded.
The DataOps suite also has user-based access through roles and containers to prevent access to reports for everyone. The BI Validator can only allow users with defined roles and permissions to access reports.
Regression Testing:
BI Validator supports regression testing of the reports through the Tableau Regression component, which automates the testing of Tableau reports during any in-place upgrades and workbook deployments. This testing happens by comparing a benchmarked/baseline version of the dashboard/report with the live version. The filters can be changed accordingly if needed before/after the benchmarking. These filter changes can be bookmarked as the latest checkpoint before running the test.
Similar to upgrades, regression test runs can validate the differences in terms of appearance or text. Also, differences in the filters can be pointed out on enabling “capture filters after applying.”
Stress Testing:
BI Validator comes with a stress test plan to simulate concurrent users accessing reports to evaluate how reports and dashboards perform under heavy load. The plan typically involves running multiple users through different types of interactions, such as viewing reports, applying filters, refreshing data, and interacting with custom visuals.
The stress test plan allows the users to select the pages/dashboards from required workspaces to perform stress testing. These pages can be either from the same or different report.
Users can run the stress test plan on specific run options like number of parallel users, time spent on each page, total simulation run time, number of seconds to reach parallel users, refresh time, and other options to run the stress test plan.
The runs will result in showing metrics like Average Open Time and Max Open Time, Average Filter Apply Time, SLA Failures.
#datagaps#Tableau#Tableau Testing#Tableau dashboard#Tableau Testing Checklist#BI Validator#BI#Youtube
0 notes
Text
Optimizing business operations with Match Data Pro LLC
With the present digital age, companies are required to have efficient tools and platforms that help manage large volumes of data seamlessly. Among these, Match Data Pro LLC stands as a leader in delivering innovative data management solutions that meet such requirements. The company's experience includes working with a range of advanced technologies, such as data ops software, on-premise data software, bulk data processing, SaaS data solutions, and much more. This article describes how Match Data Pro's offerings deal with the unique challenges that modern businesses face.

Data Ops Software: Revolutionizing Data Management
Data operations, or DataOps, is an essential framework in improving collaboration between data engineers, analysts, and business users. Match Data Pro's data ops software is designed to help organizations streamline data workflows by automating repetitive tasks, enhance data quality through integrated validation tools, and enable real-time data pipeline monitoring for proactive issue resolution.
With robust DataOps solutions, businesses can increase efficiency and improve the reliability of data insights. For example, an e-commerce platform can use Match Data Pro's software to automate product recommendation systems so that a smooth customer experience is guaranteed.
On-Premise Data Software: Total Control of Your Data
For organizations that require security and control, Match Data Pro offers on-premise data software solutions. These tools allow businesses to:
•Maintain full ownership and control over sensitive data.
•Adhere to sector-specific rules, such as GDPR or HIPAA.
•Tailor the software environment to suit particular operational needs.
Companies that are healthcare providers, among others, can keep their patients' information safe yet still use powerful analytics when they host on-premise solutions.
Bulk Data Processing: Fast and Accurate Handling of Huge Amounts
In any industry, the processing of enormous datasets must be fast and precise. Bulk data processing capabilities of Match Data Pro shine in:
•Mass processing of millions of records with no compromise on accuracy.
•Support for multiple data formats like CSV, JSON, and XML.
•Effortless scaling to match increasing data demands.
For example, a financial services firm can use these tools to analyze transaction data in bulk, identifying trends and anomalies that inform strategic decisions.
SaaS Data Solutions: Flexibility and Scalability in the Cloud
As businesses shift toward cloud-based solutions, Match Data Pro’s SaaS data solutions provide unmatched flexibility and scalability. These services enable organizations to:
•            Access data tools anytime, anywhere through a secure cloud platform.
•            Scale resources up or down based on current demand.
•            Integrate seamlessly with existing cloud infrastructures.
A retail chain, for example, can leverage SaaS data solutions to consolidate sales data from multiple locations, improving inventory management and forecasting.
Data Cleansing Software: Ensuring Data Accuracy
Clean and accurate data are the foundation of meaningful analytics. Match Data Pro's data cleansing software/tool offers features like:
• Automatic detection and removal of duplicates.
• Correction of formatting errors for standardized datasets.
• Identification of missing or inconsistent values for better data integrity.
These tools are invaluable for businesses looking to make data-driven decisions. Marketing teams, for instance, will be able to use data cleansing software to ensure the accuracy of customer segmentation in their campaigns.
Data Cleansing Tools: Easy Data Preparation
In addition to specialized software, Match Data Pro offers intuitive data cleansing tools that:
•Provide point-and-click interfaces for non-technical users.
•Allow for rapid profiling of datasets to identify anomalies.
•Support batch processing for large-scale cleansing operations.
By empowering employees at all levels of skill, these tools reduce the dependency on IT teams, allowing faster turnaround times for projects.
Data Scrubbing Software: Advanced Cleaning Capabilities
Match Data Pro's data scrubbing software takes data preparation to the next level by:
•Real-time correction of inaccuracies
•Fuzzy matching to merge records with slight variations
•Validating datasets against external sources for improved reliability.
The organization with extensive customer databases like telecom companies benefits hugely through these capabilities by having correct billing and increased customer satisfaction.
Data Integration Platform: Harmonize Your Data Ecosystem
Integration of all forms of data is a vital need for many businesses. The data integration platform offered by Match Data Pro simplifies the process through:
•API-based support for integrations with the most widely used tools and platforms.
•Unification of data from disparate systems in a unified view.
•Allow automated workflows to minimize manual intervention.
For instance, a logistics firm can combine data from warehouse management systems, delivery platforms, and customer portals so that the operations can have end-to-end visibility.
Why Match Data Pro LLC?
Match Data Pro LLC is distinguished by its ability to provide customized, scalable, and user-friendly data management solutions. The differences include:
•Tailored Solutions: Every tool and platform is made to suit the unique requirements of your business.
•High Performance: Processes more than 20,000 records per second; results are produced within required time.
•Ease of Use: Very user-friendly interfaces, resulting in less time for everyone to learn and adapt to the application.
•Robust Support: Customer support teams help achieve smooth implementation and long-term success.
Match Data Pro: Changing Industries
Solutions offered by Match Data Pro have changed the scenario of several industries:
•Healthcare: Patient records are streamlined while ensuring regulatory compliance.
•Retail: Customer analytics have improved and inventory management.
•Finance: Enhancing fraud detection and regulatory reporting.
•Manufacturing: Aligning production data to better manage the supply chain.
Conclusion
In a world of data-driven success, what makes all the difference are the right tools and platforms. Match Data Pro LLC's all-in-one set of solutions, ranging from data ops software to integration platforms, enable businesses to take on their biggest data challenges with confidence.
0 notes
Text
CloudifyOps: Unlocking Cloud Potential
Empowering businesses with a comprehensive suite of cloud and DevOps services, driving innovation and seamless digital transformation.
We help organizations become more agile and innovative by implementing cloud security best practices and managing IT operations 24/7.
CloudifyOps  services include Cloud Migration, FinOps, , DevSecOps, , Application Modernization, Site Reliability Engineering, and DataOps, ensuring efficient and secure cloud operations.
An end-to-end technology lifecycle partner, we offer in-house solutions such as Cloud Migration Factory, Well-Architected, and DevOptymize frameworks, that accelerate the process of building secure, scalable, and high-performing cloud infrastructure.Â
Whether you’re looking to migrate to the cloud or optimize your existing infrastructure, CloudifyOps has you covered.
Ready to elevate your business with powerful cloud solutions? Reach out to CloudifyOps today at [email protected] or schedule a meeting with us now!
0 notes
Text
CloudifyOps: Unlocking Cloud Potential
Empowering businesses with a comprehensive suite of cloud and DevOps services, driving innovation and seamless digital transformation.
We help organizations become more agile and innovative by implementing cloud security best practices and managing IT operations 24/7.
CloudifyOps  services include Cloud Migration, FinOps, , DevSecOps, , Application Modernization, Site Reliability Engineering, and DataOps, ensuring efficient and secure cloud operations.
An end-to-end technology lifecycle partner, we offer in-house solutions such as Cloud Migration Factory, Well-Architected, and DevOptymize frameworks, that accelerate the process of building secure, scalable, and high-performing cloud infrastructure.Â
Whether you’re looking to migrate to the cloud or optimize your existing infrastructure, CloudifyOps has you covered.
Ready to elevate your business with powerful cloud solutions? Reach out to CloudifyOps today at [email protected] or schedule a meeting with us now!
0 notes