#dataplatform
Explore tagged Tumblr posts
Text
#PowerBI#DataAnalytics#BusinessIntelligence#DAX#DataVisualization#OnlineWebinar#PowerQuery#Reporting#MicrosoftPowerBI#SQL#Insights#DataEngineering#DataScience#LearnWithCommunity#DataPlatform#OrlandoPowerBI#DataDriven#AnalyticsWebinar
0 notes
Text

🌆 𝐀𝐧 𝐚𝐥𝐥-𝐢𝐧-𝐨𝐧𝐞 𝐝𝐚𝐭𝐚 𝐚𝐧𝐚𝐥𝐲𝐬𝐢𝐬 𝐩𝐥𝐚𝐭𝐟𝐨𝐫𝐦 𝐚𝐥𝐥𝐨𝐰𝐬 𝐲𝐨𝐮 𝐭𝐨 𝐚𝐧𝐚𝐥𝐲𝐳𝐞 𝐭𝐡𝐞 𝐥𝐚𝐭𝐞𝐬𝐭 𝐔𝐫𝐛𝐚𝐧 𝐜𝐨𝐧𝐬𝐭𝐫𝐮𝐜𝐭𝐢𝐨𝐧 𝐚𝐧𝐝 𝐝𝐞𝐯𝐞𝐥𝐨𝐩𝐦𝐞𝐧𝐭 𝐩𝐫𝐨𝐣𝐞𝐜𝐭𝐬. 𝐏𝐑𝐎𝐉𝐄𝐂𝐓 𝐈𝐍𝐓𝐄𝐋 𝐟𝐨𝐜𝐮𝐬𝐞𝐬 𝐨𝐧 𝐔𝐫𝐛𝐚𝐧 𝐜𝐨𝐧𝐬𝐭𝐫𝐮𝐜𝐭𝐢𝐨𝐧 𝐝𝐞𝐯𝐞𝐥𝐨𝐩𝐦𝐞𝐧𝐭 𝐚𝐧𝐚𝐥𝐲𝐬𝐢𝐬 𝐭𝐨 𝐡𝐞𝐥𝐩 𝐜𝐥𝐢𝐞𝐧𝐭𝐬 𝐝𝐞𝐥𝐢𝐯𝐞𝐫 𝐰𝐨𝐫𝐥𝐝-𝐜𝐥𝐚𝐬𝐬 𝐩𝐫𝐨𝐣𝐞𝐜𝐭𝐬. 𝐖𝐞 ��𝐚𝐧 𝐩𝐫𝐨𝐯𝐢𝐝𝐞 𝐟𝐨𝐫𝐞𝐜𝐚𝐬𝐭𝐢𝐧𝐠, 𝐚 𝐥𝐢𝐬𝐭 𝐨𝐟 𝐦𝐚𝐣𝐨𝐫 𝐩𝐫𝐨𝐣𝐞𝐜𝐭𝐬, 𝐚𝐧𝐝 𝐝𝐞𝐭𝐚𝐢𝐥𝐬 𝐨𝐟 𝐥𝐞𝐚𝐝𝐢𝐧𝐠 𝐜𝐨𝐧𝐭𝐫𝐚𝐜𝐭𝐨𝐫𝐬 𝐚𝐧𝐝 𝐜𝐨𝐧𝐬𝐮𝐥𝐭𝐚𝐧𝐭𝐬. 𝐀𝐥𝐬𝐨, 𝐨𝐮𝐫 𝐩𝐥𝐚𝐭𝐟𝐨𝐫𝐦 𝐡𝐞𝐥𝐩𝐬 𝐲𝐨𝐮𝐫 𝐬𝐚𝐥𝐞𝐬 𝐭𝐞𝐚𝐦 𝐠𝐞𝐭 𝐚𝐜𝐜𝐮𝐫𝐚𝐭𝐞 𝐥𝐞𝐚𝐝𝐬. 🚀 𝐌𝐨𝐫𝐞𝐨𝐯𝐞𝐫, 𝐲𝐨𝐮 𝐜𝐚𝐧 𝐞𝐯𝐞𝐧 𝐭𝐫𝐚𝐜𝐤 𝐨𝐯𝐞𝐫 𝟗,𝟕𝟐𝟔 𝐔𝐫𝐛𝐚𝐧 𝐝𝐞𝐯𝐞𝐥𝐨𝐩𝐦𝐞𝐧𝐭 𝐩𝐫𝐨𝐣𝐞𝐜𝐭𝐬 𝐯𝐚𝐥𝐮𝐞𝐝 𝐚𝐭 𝐚𝐧 𝐞𝐬𝐭𝐢𝐦𝐚𝐭𝐞𝐝 $𝟗𝟗𝟓 𝐁𝐧. 𝐅𝐨𝐫 𝐦𝐨𝐫𝐞 𝐝𝐞𝐭𝐚𝐢𝐥𝐬, 𝐯𝐢𝐬𝐢𝐭 https://www.projectintel.net/urban-development-projects
0 notes
Text

data🔔platform
1 note
·
View note
Text
Elevate Your Data Strategy with Dataplex Solutions
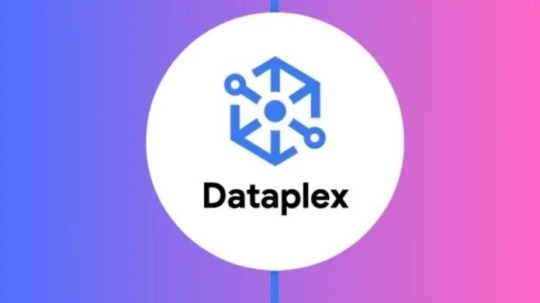
Scalability, agility, and governance issues are limiting the use of traditional centralised data architectures in the rapidly changing field of data engineering and analytics. A new paradigm known as “data mesh” has arisen to address these issues and enables organisations to adopt a decentralised approach to data architecture. This blog post describes the idea of data mesh and explains how Dataplex, a BigQuery suite data fabric feature, may be utilised to achieve the advantages of this decentralised data architecture.
What is Data mesh
An architectural framework called “data mesh” encourages treating data like a product and decentralises infrastructure and ownership of data. More autonomy, scalability, and data democratisation are made possible by empowering teams throughout an organisation to take ownership of their respective data domains. Individual teams or data products assume control of their data, including its quality, schema, and governance, as opposed to depending on a centralised data team. Faster insights, simpler data integration, and enhanced data discovery are all facilitated by this dispersed responsibility paradigm.
An overview of the essential components of data mesh is provided in Figure 1.Image credit to Google Cloud
Data mesh architecture
Let’s talk about the fundamentals of data mesh architecture and see how they affect how we use and manage data.
Domain-oriented ownership:
Data mesh places a strong emphasis on assigning accountability to specific domains or business units inside an organisation as well as decentralising data ownership. Every domain is in charge of overseeing its own data, including governance, access controls, and data quality. Domain specialists gain authority in this way, which promotes a sense of accountability and ownership. Better data quality and decision-making are ensured by this approach, which links data management with the particular requirements and domain expertise of each domain.
Self-serve data infrastructure:
Within a data mesh architecture, domain teams can access data infrastructure as a product that offers self-serve features. Domain teams can select and oversee their own data processing, storage, and analysis tools without depending on a centralised data team or platform. With this method, teams may customise their data architecture to meet their unique needs, which speeds up operations and lessens reliance on centralised resources.
Federated computational governance:
In a data mesh, a federated model governs data governance instead of being imposed by a central authority. Data governance procedures are jointly defined and implemented by each domain team in accordance with the demands of their particular domain. This methodology guarantees that the people closest to the data make governance decisions, and it permits adaptation to domain-specific requirements with flexibility. Federated computational governance encourages responsibility, trust, and adaptability in the administration of digital assets.
Data as a product:
Data platforms are developed and maintained with a product mentality, and data within a data mesh is handled as such. This entails concentrating on adding value for the domain teams, or end users, and iteratively and continuously enhancing the data infrastructure in response to input. Teams who employ a product thinking methodology make data platforms scalable, dependable, and easy to use. They provide observable value to the company and adapt to changing requirements.
Google Dataplex
Dataplex is a cloud-native intelligent data fabric platform that simplifies, integrates, and analyses large, complex data sets. It standardises data lineage, governance, and discovery to help enterprises maximise data value.
Dataplex’s multi-cloud support allows you to leverage data from different cloud providers. Its scalability and flexibility allow you to handle large volumes of data in real-time. Its robust data governance capabilities help ensure security and compliance. Finally, its efficient metadata management improves data organisation and accessibility. Dataplex integrates data from various sources into a unified data fabric.
How to apply Dataplex on a data mesh
Step 1: Establish the data domain and create a data lake.
We specify the data domain, or data boundaries, when building a Google Cloud data lake. Data lakes are adaptable and scalable big data storage and analytics systems that store structured, semi-structured, and unstructured data in its original format.
Domains are represented in the following diagram as Dataplex lakes, each controlled by a different data provider. Data producers keep creation, curation, and access under control within their respective domains. In contrast, data consumers are able to make requests for access to these subdomains or lakes in order to perform analysis.Image credit to Google Cloud
Step 2: Define the data zones and create zones in your data lake.
We create zones within the data lake in this stage. Every zone has distinct qualities and fulfils a certain function. Zones facilitate the organisation of data according to criteria such as processing demands, data type, and access needs. In the context of a data lake, creating data zones improves data governance, security, and efficiency.
Typical data zones consist of the following:
The raw zone is intended for the consumption and storage of unfiltered, raw data. It serves as the point of arrival for fresh data that enters the data lake. Because the data in this zone is usually preserved in its original format, it is perfect for data lineage and archiving.
Data preparation and cleaning occurs in the curated zone prior to data transfer to other zones. To guarantee data quality, this zone might include data transformation, normalisation, or deduplication.
Zone of transformation:This area contains high-quality, structured, and converted data that is prepared for use by data analysts and other users. This zone’s data is arranged and enhanced for analytical uses
Image credit to Google Cloud
Step 3: Fill the data lake zones with assets
We concentrate on adding assets to the various data lake zones in this step. The resources, data files, and data sets that are ingested into the data lake and kept in their designated zones are referred to as assets. You can fill the data lake with useful information for analysis, reporting, and other data-driven procedures by adding assets to the zones.
Step 4: Protect your data lake
We put strong security measures in place in this stage to protect your data lake and the sensitive information it contains. Protecting sensitive data, assisting in ensuring compliance with data regulations, and upholding the confidence of your users and stakeholders all depend on having a safe data lake.
With Dataplex’s security approach, you can manage access to carry out the following actions:
Establishing zones, building up more data lakes, and developing and linking assets are all part of managing a data lake.obtaining data connected to a data lake through the mapped asset (storage buckets and BigQuery data sets, for example)obtaining metadata related to the information connected to a data lake
By designating the appropriate fundamental and preset roles, the administrator of a data lake controls access to Dataplex resources (such as the lake, zones, and assets).Table schemas are among the metadata that metadata roles can access and inspect.The ability to read and write data in the underlying resources that the assets in the data lake reference is granted to those who are assigned data responsibilities.
Benefits of creating a data mesh
Enhanced accountability and ownership of data:
The transfer of data ownership and accountability to individual domain teams is one of the fundamental benefits of a data mesh. Every team now has accountability for the security, integrity, and quality of their data products thanks to the decentralisation of data governance.
Flexibility and agility:
Data meshes provide domain teams the freedom to make decisions on their own, enabling them to react quickly to changing business requirements. Iterative upgrades to existing data products and faster time to market for new ones are made possible by this agility.
Scalability and decreased bottlenecks:
By dividing up data processing and analysis among domain teams, a data mesh removes bottlenecks related to scalability. To effectively handle growing data volumes, each team can extend its data infrastructure on its own terms according to its own requirements.
Improved data discoverability and accessibility:
By placing a strong emphasis on metadata management, data meshes improve both of these metrics. Teams can find and comprehend available data assets with ease when they have access to thorough metadata.
Collaboration and empowerment:
Domain experts are enabled to make data-driven decisions that are in line with their business goals by sharing decision-making authority and data knowledge.
Cloud technologies enable scalable cloud-native infrastructure for data meshes.Serverless computing and elastic storage let companies scale their data infrastructure on demand for maximum performance and cost-efficiency.
Strong and comprehensive data governance: Dataplex provides a wide range of data governance solutions to assure data security, compliance, and transparency. Dataplex secures data and simplifies regulatory compliance via policy-driven data management, encryption, and fine-grained access restrictions. Through lineage tracing, the platform offers visibility into the complete data lifecycle, encouraging accountability and transparency. By enforcing uniform governance principles, organisations may guarantee consistency and dependability throughout their data landscape.
Effective data governance procedures are further enhanced by Dataplex’s centralised data catalogue governance and data quality monitoring capabilities.Businesses can gain a number of advantages by adopting the concepts of decentralisation, data ownership, and autonomy.Better data quality, accountability, agility, scalability, and decision-making are benefits. This innovative strategy may put firms at the forefront of the data revolution, boosting growth, creativity, and competitiveness.
Read more on govindhtech.com
#Dataplex#BigQuery#DataIntegration#datamanagement#architecturalframework#DataGovernance#dataplatforms#GoogleCloud#strongsecurity#news#technews#technology#technologynews#technologytrends#govindhtech
0 notes
Text
#AI#BigData#CloudComputing#DataAnalytics#DataPlatforms#DataScience#IoT#IIoT#PyToh#Python#RStats#TensorFlow#Java#JavaScript#ReactJS#GoLang#Serverless#4typesofdataanalytics#analytics#awsiotanalytics#bigdata#bigdataanalytics#dataanalysis#dataanalytics#dataanalyticscareer#dataanalyticsjob#dataanalyticsproject#dataanalyticsroadmap#dataanalyticstrends#datahandlinginiot
0 notes
Text

Uitgelezen “De techcoup” van Marietje Schaake. In De tech coup onderzoekt Marietje Schaake hoe technologiebedrijven steeds vaker besluiten nemen die voorheen aan overheden waren voorbehouden. Die verschuiving, zo stelt zij, vormt een serieuze bedreiging voor de democratie. Schaake, voormalig Europarlementariër, maakt zich zorgen over de groeiende invloed van grote techbedrijven en pleit voor meer transparantie, publieke controle en heldere spelregels.
Aan de hand van sprekende voorbeelden – zoals de rol van Elon Musk in de oorlog in Oekraïne via Starlink, de lobbystrategieën van Microsoft en Meta, en de betrokkenheid van dataplatform Palantir bij de Amerikaanse overheid – laat ze zien hoe ver de invloed van deze bedrijven inmiddels reikt. De balans tussen publieke en private macht is zoekgeraakt, zeker in de Verenigde Staten, waar bedrijven veel ruimte krijgen om te innoveren zonder dat daar stevige regulering tegenover staat.
Ook dichter bij huis signaleert Schaake risico’s, zoals het ondoordachte gebruik van AI door Nederlandse gemeenten. Digitale technologie verandert de spelregels, maar democratische controle en publieke waarden blijven achter. Daarom pleit ze voor een Europese digitale infrastructuur die op publieke leest is geschoeid, en voor coalities die samenwerken op basis van gemeenschappelijk belang in plaats van commerciële winst.
Naast technologie gaat het boek ook over bredere machtsstructuren: over geld, politieke invloed, mensenrechten, verkiezingen en digitale oorlogsvoering. Schaake laat zien hoe technologiebedrijven zich vaak aan nationale wetgeving weten te onttrekken, en benadrukt het belang van brede, internationale regelgeving en versterkt toezicht.
Toch is haar toon niet pessimistisch. Ze ziet volop kansen voor technologie, mits we als samenleving bewust kiezen voor systemen die het publieke belang dienen. Vooral voor de Europese Unie ziet ze een cruciale rol weggelegd: als hoeder van democratische waarden en als tegenwicht tegen de macht van Silicon Valley.
0 notes
Text
Eendaagse workshop voor opzetten Nationaal Georuimtelijke Dataplatform

Het ministerie van Ruimtelijke Ordening en Milieu (ROM) heeft het initiatief genomen om een eendaagse workshop te organiseren voor het opzetten van een National Geospatial Intelligence Hub, of Nationaal Georuimtelijke Dataplatform. Met het opzetten van een zo’n platform wordt georuimtelijke data van verschillende organisaties en overheidsinstanties toegankelijk op een centraal punt. De workshop heeft plaatsgevonden op donderdag 12 oktober 2023 in het Lalla Rookh Gebouw. ROM-minister Marciano Dasai zegt dat het hebben van een centraal platform voor georuimtelijke data belangrijk is voor onder meer besluitvormingsprocessen en het schrijven van projecten ter ontwikkeling van gebieden. Hierdoor zal er een beter overzicht zijn van de data die zit bij de verschillende NGO’s, en overheidsinstanties. “Bij het maken van een probleemanalyse ben je afhankelijk van data die beschikbaar is, om op basis daarvan het probleem te omschrijven. Zonder data zijn we zowel blind als doof en kunnen we niets doen”, aldus de bewindsman. Minister Dasai noemt als voorbeeld een situatie waarbij bodemdata nodig is voor een agrarisch project. Hij legt uit dat er geen tijd verspild hoeft te worden om weer een bodemonderzoek te doen als de meest recente data beschikbaar is op het platform. Voor het opzetten van het Nationaal Georuimtelijke Dataplatform wordt samengewerkt met het Braziliaanse bedrijf Codex die gespecialiseerd is in het opzetten van zulke informatie hubs. Zij zullen samenwerken met hun counterpart in Suriname GISsat (Geographical Information Systems Software, Application and Training). Het project wordt ondersteund door de inter-Amerikaanse Ontwikkelingsbank (IDB). Read the full article
0 notes
Text
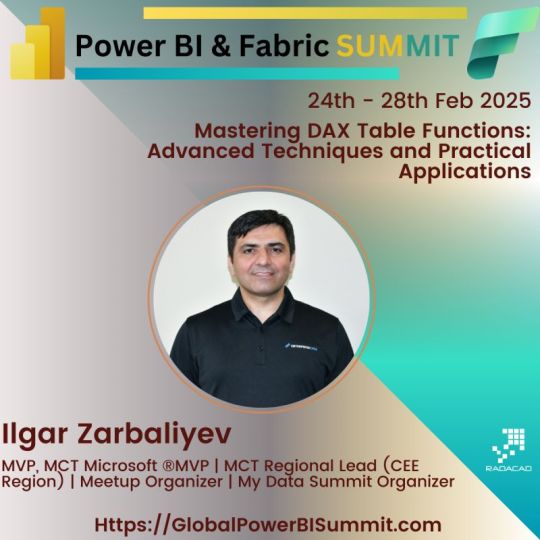
#DataAnalytics#Analytics#Conference#Training#DataWarehousing#Azure#Database#BusinessIntelligence#Realtime#Microsoft#Fabric#DataPlatform#DataEngineering#SQL#Reporting#Insights#Visualization#DAX#PowerQuery#Administration#DBA#DataScience#MachineLearning#AI#MicrosoftAI#Architecture#BestPractices
0 notes
Text
#iSportz#sportstechnology#sportsmembermanagement#sportseventregistration#sportslms#digitalexperience#dataplatform#sportsexperience#sportsconnect#sportsenhance#sportsdatainsight#sportsdelight#sports#sportsindustry#sportsmanagement#sportssoftware#Saas#saasproduct#saasgrowth#saasplatform
2 notes
·
View notes
Text
Are Data Lakes And Data Warehouses The Two Sides Of A Modern Cloud Data Platform

A true cloud data platform is capable of providing a plethora of functions, which complement and overlap one another. The majority of the business organizations consolidate the data from different resources into the singular customizable platform for big data analytics.
A separate platform for data analytics offers the prerequisite choice to create the dashboards for analyzing, aggregating, and segmenting high-dimensional data. It provides a helping hand in creating low-latency queries for performing real-time analytics.
Data lakes and Data warehouses are also known to be common alternatives. Data warehouses and data lakes are believed to be the two different sides of the recent cloud data platform, which offers a wide array of benefits.
What is Data Lake?
Data Lake Solutions refers to a term which was introduced in the year 2011 by James Dixon, Pentaho CTO. It contributes to being the large data repository in the unstructured and natural form.
Raw data is known to flow into the data lake. Also, users have the opportunity to correlate, segregate, and analyze various data parts, following the needs.
Data Lake depends on low-cost storage options, which are beneficial in storing the raw data. Data gets collected from different sources in real-time, after which it is transferred into the data lake in the original format.
It is possible to update the data in the data lake in batches and real-time, thereby creating the volatile structure.
What is Data Warehouses?
A data warehouse contributes to being the central repository of the data, which is collected from a vast array of diverse sources, such as in-house repositories and cloud-based applications.
The data warehouse makes use of column-oriented storage, referred to as the columnar database. The database is capable of storing the data by the columns and not by rows.
Hence, it is believed to be an excellent choice for data warehousing. If there is a data warehouse enriched with historical and current data, people in the business organization will use it to create trend reports and forecasting dashboards with the aid of different tools.
A data warehouse boasts of certain characteristics, which include scalable, structured, non-volatile, and integrated. The scalable data warehouse is capable of accomplishing the enhanced demands for the storage space.
The structured data warehouse uses a columnar data store to bring an improvement in the analytical query speed. As the data present in the data warehouse is uploaded periodically, the momentary change does not affect decision making.
The integrated data warehouse involves the extraction and cleansing of data uniformly, instead of the original source.
The data warehouse serves as the data-tier application known to define the schemas, instance-level objects, and database objects used by the client-server or three-tier application.
Data Warehouse and Data lakes- Two sides of the cloud data platform
Data lakes and Data Warehouses are recognized to be the two sides of the cloud data platform, which offers a helping hand in making an informed purchase decision.
There are specific use cases that boast of a data analytics engine in which the data warehouse and data lake will co-exist. However, it depends on the area's different functional requirements, which include data adaptability, data structure, and performance.
Data Performance
As you aim to create a data warehouse, the data source analysis happens to be a significant time-consuming factor. It is useful in the creation of an organized and structured data model, which is meant for individual reporting needs.
A crucial part of the process is deciding the type of data which should be included, and things, that need to be excluded from the data warehouses.
It includes the data collected from different resources, after which the data should be aggregating and cleansing. Also referred to as data scrubbing, data cleaning refers to the technique of data clean up.
It happens before the data is loaded into the data warehouse. The objective of data cleansing is the elimination of outdated data.
After the completion of data cleansing, it is ready for analysis. However, it takes the prerequisite energy and time, owing to the sequence involved in data cleansing techniques.
Data warehouse works wonders in cleaning the data. However, it is a bit pricey. A data lake includes relevant data sets, regardless of the structure and source. It is responsible for the data storage in the original form.
Data warehouses are created for the purpose of faster analytical processing. The columnar and underlying RDBMS provides accelerated performance, which is optimized for the purpose of analytical query processing.
It is inclusive of high concurrency and complicated joins. However, it would be best if you keep in mind that data lakes are not performance-optimized. However, if you have any access to it, you will be capable of exploring the data at their own discretion, leading to a non-uniformed data representation technique.
Adaptability
A robust data warehouse has the ability to change faster and adapt to various scenarios. However, the data lake is faster to adapt to different changing requirements to adjust to multiple scenarios.
The complications of the upfront tasks need the resources and time of the developer. Data Lake can adapt to other changing requirements owing to the fact that data is present in the raw and unstructured form.
Such type of unstructured data is available to the potential audience, which has the power to use and access it for forming the analysis, catering to the requirements. The developers should devote and hump the resources and time, which is necessary to get meaningful information from the data.
Microsoft, Google, and Amazon confer Data Lake and Data Warehouse services, which offer platforms, against which the business organizations will run the BI reporting and analytics in real-time.
Microsoft Azure and Amazon Redshift are developed on the relational database model's top. It also provides large-scale and elastic data warehouse solutions. Google Cloud Datastore contributes to being the NoSQL Database as a Service of SaaS capable of automatically scaling. Every data warehouse is equipped with BI tools, which are integrated into the services.
1 note
·
View note
Text
Best Data Platform And Analytics Services
Helping organizations unlock growth through data. Construct a modern data ecosystem through a consultative approach by experts. Transform Your Data Into Actionable Intelligence. For more information visit, Data Platform and Analytics.
0 notes
Text

Why are data platforms on top of the minds of Enterprise CIOs? We have the answers:
0 notes
Text
0 notes
Text
#AI#BigData#CloudComputing#DataAnalytics#DataPlatforms#DataScience#IoT#IIoT#PyTorch#Python#RStats#TensorFlow#Java#JavaScript#ReactJS#GoLang#Serverless#4 types of data analytics#analytics#aws iot analytics#big data#big data analytics#data analysis#data analytics#data analytics career#data analytics job#data analytics project#data analytics roadmap#data analytics trends#data handling in iot
0 notes