#matrices class 12
Explore tagged Tumblr posts
Video
youtube
Matrices and Determinants Class 12 | PUC Mathematics | NCERT Solutions ...
1 note
·
View note
Text

sex for homework
luigi mangione x reader
。𖦹°‧ you ask your cute tutor to help you study for your math final.
word count: 5.5k • part of my study buddies series (read here!) • nsfw • read on ao3
warnings : f! reader; EXPLICIT; dumbification if U squint; praise; oral (m! receiving); pre calc lol
notes : crossposting my shit to tumblr and starting with arguably one of my greatest uses of free will in history. title frommm:
You have a bit of a dilemma.
Well, it would be more accurate to say that you had a dilemma, have had one for quite a while now—your current grievances are merely extensions of a constant, one raging, blood-thirsty, borderline psychopathic problem of a class. MTH121, Concepts & Applications, is the only remaining mathematics credit required for your degree, and, coincidentally, absolutely no one told you that that’s really just a fancy name for pre-calculus. Because the universe hates you.
Your final is tomorrow. Yes, tomorrow. If that wasn’t bad enough, your brain has utterly fucked you; months spent poring over formulas and right triangles amounts to nothing in the moment, every relevant fragment of knowledge completely foreign to your burnt out, sleep deprived, caffeine ridden psyche. So here you sit, “studying”, armed with just your textbook and Khan Academy tutorials.
Is it too late to switch majors? Yes, you decide, massaging your temples as you take another glance at your notes. A mass of numbers, variables, and scribbled matrices clogs the pages, complete with your near ineligible annotations, details added in the heat of a lecture. You never knew there could be so many different types of numbers. Solve for x. 5 + 2x to the 2nd power = 8x. Factor x3 - 3x to the 2nd power - 4x + 12. Find the vertex of the function f(x) = x to the 2nd power + 4x + 3. Determine the value of x if the sum of the following sequence converges to 5. How any of this is relevant to your future non-mathematics degree is beyond you.
What the hell is a vertex again? And what does it matter? You’d rather be sleeping, or drunk. Whatever.
You have one saving grace. Since your freshman year you’ve been employing a little cheat-sheet, your one-way ticket to having math explained to you in a language understood by plebeians like yourself: one Luigi Mangione, a friend of a friend of a friend, possibly the smartest guy you know (and you’re far from the only person to voice that opinion). Your self-appointed tutor—and unfortunately for you, probably the most appetizing of any of the frat guys you’ve met in college, to put it chastely. The actual knowledge is just a bonus, really, because unlike other tutors you’ve worked with Luigi seems to actually care; he wants you to walk away from him with a solid understanding of the material, rather than a temporary knowledge that gets your homework done but is absent from your memory by the time of your exams. And it’s hard to write off the fact that he’s easy on the eyes.
…Pretty damn hard, actually. Because—in all honesty—you’re really into Luigi. Another thing that’s hard to do is get your math homework done when you’re busy fucking yourself with your fingers, like you tend to do after your time with him, thinking about his cock, his hands, the way he would fill you, pin you down underneath him, smirk at you and tell you dirty things like that’s my girl, that’s my good fucking girl, that’s it, give it to me, show me how pretty you look when you come all over me like this…
Great. At this pace, you’ll never get anything done.
Your phone buzzes.
About an hour ago, you sent him a photo of your current predicament: your laptop and notebook open, and you sitting criss-crossed in front of it, an exaggerated pout on your lips. A few moments later, you sent another, this time of your middle finger pointed directly at your professor’s official portrait. Now, he responds:
Academic Weapon (Luigi) : Smh
Who studies the night before their final?? Dummy
You smile, replying:
i do :(
help pls :((
Academic Weapon (Luigi) : You poor thing
And then:
Academic Weapon (Luigi) : Come over. In like 15
We’ll work it out together
Score. He adds:
Academic Weapon (Luigi) : And I better not hear any complaining when I make you actually do the math
Your crush feels elementary, like you’ve got the hots for the nerdy jock on the playground that’s miles out of your league and that every girl on planet Earth is fighting tooth and nail for. You respond:
no promises :P
You pray to your lucky stars that you can study as nonchalantly as humanly possible.
You told him you wouldn’t complain, and you’ve tried, you really have. But dividing radicals is fucking stupid and useless and the more you look at your paper the more these numbers and symbols really start to look all the same to you, just scribbles, meaningless scribbles of made-up concepts that have nothing to do with your career prospects whatsoever. Who gives a flying fuck about solving equations with these weird ass numbers that normal people don’t even use?
You must be thinking out loud, because Luigi laughs next to you on the couch. He is laughing at your frustration. What an emotionally supportive tutor. You groan and thread your fingers through your hair, massaging your temples.
Still smiling just slightly, he starts to gather up your things. “Alright, look, how about we take a break?” He glances over at you, still holding your head in your hands. “Yeah, let’s take a break for a minute.”
He gets up from the couch, disappears into the kitchen for just a moment. Comes back with a glass of orange juice. For you. You try not to think about how pathetic it is that the most romantic gesture a man has done for you in the past three years is bring you juice. Instead you watch him, sipping slowly—no pulp, he knows you so well—and peeking through your eyelashes as he scuttles around his dorm, just the two of you alone together, while he throws some laundry into a basket and absentmindedly closes doors of unoccupied rooms. You have never noticed how defined his calves are before, nor how his curls bounce just slightly when he walks fast or how his shorts sag on his hips just right, just enough for you to get a peek of his V-line and the waistband of his boxers when he raises his arms to stretch—
Nonchalant. Demure. Mindful. You are failing so hard at the one thing you’ve forbidden yourself from doing: staring at him until your eyes are practically burning holes in his clothes and he’s melting into the floor. Not entirely your fault. He should’ve known to dress modestly around you. Around anybody, for that matter.
Luigi comes to sit by you now. As you tuck your hair behind your ears you can feel his arm move to rest along the back of the couch, almost around you, but not quite.
“Hi,” you say, propping your head up on your arm.
He smiles at you. You can’t even look him in the eye. “Did you think more about your radicals?”
“Don’t remind me,” you groan, rolling your eyes. “No. I didn’t.”
“Well, what were you thinking about?”
You swallow the conspiratorial intuition that he has to be fucking with you. Maybe he sees it on your face. Can smell it on you. Something.
“I was trying to think of some things I’d rather be doing,” you offer. “Instead of math.”
Your heart feels three beats faster all of a sudden, and when did he get so close to you? Your thighs are touching, his knee brushing against yours. “And what did you come up with?” he asks.
Oh, fuck. He’s definitely fucking with you. Right? He has that goddamn smirk on his face, that one that makes your insides twist with a feeling reserved only for boys who look at you just like this, like you’re busted, like he knows exactly what you’ve been thinking about every second you’ve spent sitting next to him doing algebra. You want to kiss it right off of him.
“Nothing,” you lie, sitting up straight and trying to pretend like you really are interested in your studies. “Here, will you show me how to do it again?”
He calls your name. He doesn’t even have to ask for you to look at him; the tone of his voice and the tilt of his head makes his intentions entirely clear. When your eyes meet his he inches closer, and all you can manage to do is stare at his lips.
“Tell me what you want,” he demands, stern and warm enough to boil.
If he truly knew what he was asking for he wouldn’t be asking at all, you think. Not unless he was prepared for whatever your fervent need has in store for him. Embarrassment feels bright red and prickly on your skin. “I shouldn’t say.”
”But I think you should,” he whispers.
Oh. Oh. All bets are off, now. This has officially progressed from studying to “studying”.
Luigi lets you lead, his hand settling on the small of your back as you come a little closer to kiss him, properly. You hear him giggle before your lips meet; the curve of his smile against you is unmistakable, casting sparks through your body and down your thighs. He tastes like spearmint. You learn quickly that he is a fantastic kisser, and his tongue finds yours with curious excitement when your breathing starts to pick up. Without question, he claims the expanse of you, drinking in your essence, licking, biting. Those irresistible curls demand attention, and so you thread your fingers through his hair, your hand sweeping from behind his ear to the nape of his neck. Luigi shivers under your touch, exhaling softly against you.
When the fingers of his left hand raise to grasp your leg, you stop kissing him only to swing your body over his lap so that you’re straddling him. Luigi breathes in deep then, like his nervous system collectively seizes at the feeling of you so close. To give him room to breathe you stop short of settling all your weight onto him. Lips meeting once more, his hands greet your hips; his touch is warm, and timid, like you’re made of sand, like you might collapse and dissolve into immeasurable particles between his fingers.
He groans into your mouth. Murmurs your name. “This isn’t very productive,” he quips.
“Intellectually, no,” you agree, nails brushing the back of his neck. He has goosebumps. A ghost of a smile dancing on your lips, you slowly lower yourself down onto his lap; there are two layers of clothes between your bare skin but he is impossibly warm against you. “But what about physically?”
Luigi smiles, and fuck, he is too fucking beautiful. “I guess I can’t argue with that.”
And so you kiss him again and again and again, your heart doing backflips inside your chest when his big hands glide lower, and lower, thumb toying with the waistband of your skirt, and lower still, until he’s gripping your ass. You can’t help but nuzzle against the growing stiffness underneath you, poking between your thighs—and you definitely can’t help but love the way he grinds back, hips meeting yours with just as much enthusiasm. Fuck. About an hour ago you were working through polynomials and linear equations, and now the dreamiest guy you’ve ever met is hard for you, holding you in his lap. You might as well thank your professor.
When Luigi sucks at your bottom lip for a few euphoric moments, you make the most pathetic sound into his mouth, and he growls, his hands suddenly coming up to grasp your hips and hold them steady. “Was this your plan all along?” he rasps, his lips moving swiftly to the side of your face, your jaw, the junction between your neck and shoulder.
Sharp teeth graze skin and you whimper. “What do you mean?”
“What, now you’re playing coy?” Luigi finds the pulse point in your throat and bites, softly at first, then harder when your fingers curl into the hair at the back of his head. “You didn’t want to study. You called me because you wanted to get fucked, because you knew I’d want to touch you just like this, didn’t you?”
This boy is out of his mind. First he practically eye-fucks you while schooling you about imaginary numbers, and then he “scolds” you like he’s disappointed in your lack of interest in algebra, like he’s mad that you can’t resist him for being so damn gorgeous. That half-hearted meanness in his tone leaves butterflies in your stomach, in no way helped by the feeling of his tongue sliding over your collarbone.
“No,” you mutter. It’s not completely a lie. You really did need his help with the math, which he is really good at…but you can’t deny that you were really hoping you two would end up like this, with him kissing your neck all over until you’re speckled with purple and pink. You don’t even care about the obvious evidence of him on your skin—you want his entire dorm hall to know just how well-acquainted the two of you are by the time he’s done with you. The thought of everyone knowing you’re his makes you weak.
Luigi is kissing you again, slowly and deeply, one hand coming up to cup your breast through your shirt. His touch is too much and not enough simultaneously, your need overwhelming, and your hips are searching desperately for friction, rolling against him eagerly. So much for nonchalance.
He grasps your chin, firm but not at all painful, and flashes you that pretty smile, tutting, “I don’t believe you.”
Your mind is far too preoccupied with thoughts of his touch in other places to try to formulate a witty rebut. You opt instead to kiss him harder and sneak a hand between your bodies, tracing over his chest, down his carefully crafted abdomen, and then over the front of his shorts, groping his hard cock through polyester. Luigi groans into your mouth. He is big, almost intimidating, and imagining him inside of you has your body feeling hot all over.
As you palm the outline of his length through his trousers, his hands make their way underneath your sweater, the sudden warmth of him jolting through your torso. You look up at him through your lashes and he smirks.
“Do you want to sit on it?” he asks you, entirely stoic despite the weight of his words.
You kiss him, still squeezing his cock. “Can I put it in my mouth first?”
Fuck. You have him wrapped around your finger. How could he possibly say no when you ask so sweetly? Luigi is instantly pulling down his shorts for you, the rustle of fabric making your head spin. He’s left in just his boxers and a sweater that you quickly help him shrug off, too. Once you have him undressed, he takes a moment to survey you, your cheeks flushed, eyes lidded, hair tousled from his hands. You feel a surge of confidence now that you have his full attention and so you pull your top up and over your head, smiling when he reaches behind you to help you with your bra. He has it and your skirt off in just a few seconds, leaving your combined clothes to pile up next to the couch.
You shift so that you’re kneeling on the floor in front of him, wearing only your panties, watching him watching you. He is grinning, his cock standing proud, and you know you must be blushing by the way his teeth flash from under the curve of his lips. You feel gooey and hot in the pit of your stomach. Swallowing your shyness, you reach forward to take him in your hand. He’s already sticky at the tip, precum glistening on his slit, and so you begin to stroke him, starting at the head of his dick and spreading slick down his shaft. His cock is probably the most gorgeous thing you’ve ever seen, at the very least a runner-up for his face: tan and thick, his girth evenly distributed, and big enough to have you feeling your heartbeat between your legs. There is a prominent vein along the underside of him, ending at his frenulum. He pulses with each movement of your hand.
Once he’s as wet as you like, you come closer to tease him with your tongue, licking up the base, tracing his vein, passing over his slit. Luigi groans—“fuuuuuck, baby,”—and threads his fingers into your hair, tugging hard.
“Don’t be a fucking tease,” he rasps. “You asked for this. Show me what that mouth can do.”
Your lips are halfway wrapped around the head of him and when you moan at his words it vibrates through him, his abs flexing deliciously. You move further down, then, mouth closed around his length, applying light pressure on your way back up. He’s too big to take all of him at once and so your left hand grasps the length you can’t reach, pumping gently. You start a subtle, easy rhythm, evenly paced and obviously satisfying enough to have Luigi panting and swearing above you: your mouth starts at his tip, sucking gently, then gliding lower, until you can feel him in the back of your throat and you’re nearly gagging on him—and then you move upward again, cheeks hollowing around him, finally reaching the head of him once more. Rinse and repeat. It is organized. Formulaic. Your process leaves you practically drooling on his cock, spit collecting at the base where you are stroking him. Fuck. You haven’t pleased a guy like this in quite a while, and under any other circumstances you’d probably feel a bit insecure about your work; but it’s difficult to justify any doubts you might have, what with the noises coming from above you:
“Oh, fuck, yes, baby, yes, just like that, fuck yes,” Luigi moans, fingers knotted tightly in your hair. “Oh my god, your mouth…”
You slip your free hand into your panties, middle and ring finger rubbing your clit.
As your ministrations intensify, his reactions do, too. You can feel his thighs and hips tensing in an effort not to fuck into your throat. But you made a promise to yourself; you want to take the entirety of his length in your mouth before all of this is over, and so you move your left hand down to his balls, kneading him and carefully lowering your face until your nose is pressed into the curly hairs of his groin, his cock as deep as it can reach. And Luigi keens, head thrown back against the couch, one hand in your hair and the other gripping the armrest tight. You can feel him twitching in your throat.
There are a few blissful moments of you sucking him just like this, sinking him deep into your throat and pinching your lips around his tip, and you almost wish the two of you were recording because the sounds he makes are top tier jerk material for at least the next few months. He’d be a natural on camera. You want to commit every second of this to your memory.
When he goes quiet for a moment you open your eyes to look at him. You find him staring down at you, mouth agape. “Are you touching yourself?” he asks.
It’s difficult to answer with his dick in your mouth, so you settle for moaning around him again, eyes fluttering shut.
“Holy fuck,” he grunts, his voice sweeter than sugar.
You could sit here sucking him off for the rest of your life—you could die with his dick in your mouth—but you regrettably begin to feel your jaw aching, knowing full well that keeping this up will have you hurting. Not that you really mind. When you begin to sputter and tear up around him, he grabs both sides of your face and pulls your mouth off of his cock. You are crying, just a little, crocodile tears streaming down your cheeks, your throat raw.
Luigi looks down at you sweetly. “Oh, baby,” he coos, wiping your wet face dry with his thumbs. “That’s my perfect girl. So good to me. Come here.”
He welcomes you back onto his lap with open arms and a smile. He is warm, so warm and soft against you, you could fall asleep just like this. But he is kissing you now, so slowly that you feel dizzy, and so you ground yourself, fingers embracing his curls. His hands move to your hips, grasping the waistband of your panties, teasing you, rubbing the fabric against your heat. When he finally has them off his fingers are instantly examining you, collecting your slick, slipping through your folds.
“Let’s see about a little reward for you, hm?” he whispers, capturing your lips with his.
You kiss him eagerly and arch your back so that your thighs spread wide enough for his fingers to enter you with ease—not that it would be difficult without, considering that you’re so wet you can hear him touching you, even over the sound of your blood rushing in your ears. Two long digits move inside of you, stretching you, massaging that spot that makes your knees buckle and your eyes cross, plus a few more that you never knew existed. His touch feels so good, just how you imagined, and you have to lean forward into the crook of his neck to keep yourself upright, your teeth sinking into a firm shoulder. Luigi makes a gruff sound, almost a chuckle, and his cock jumps at your whiny, choked noises when he adds a third finger into your pussy.
“So needy, aren’t you?” he teases. “Have you been thinking about this, gorgeous? About sucking my cock and taking my fingers like this?”
You nod, because of course you have. In that exact order. Who wouldn’t?
Luigi smiles at you, soft and adoring. You make a curious sound and his fingers depart from you, lingering at your entrance until you grind down into his lap. Your cunt brushes against him, raw, hungry, slathering his cock with your slick.
“I want you,” you whine, grabbing his face and kissing him again. “I want all of you.”
“Yeah, baby?” His hands are guiding your hips, moving you slowly against him. “Tell me about it.”
Well, you would, if your brain weren’t short-circuiting at the moment. His fault. You mumble into his ear, something about infinity, something about the way you hug your pillow at night and all the times you’ve fucked yourself stupid thinking about this very image of you and him together like this. But there are countless words for your endless feelings, words you would preach to him from high places if your body had the agency to; your attraction to him is primal, but neatly arranged, layered, wrapped up with variables galore and multiplying with each moment you spend in his presence. A mess, no doubt about it, but one you can control, a tangle to unravel, an equation to solve. Nothing less. You aren’t sure of how this ends but you know that you need him, bad, more than you knew was possible before.
You crash into him, mouths colliding, everything that you left unsaid spilling into your embrace. Words are hard. Kissing Luigi and grinding your warm, throbbing cunt against him takes much less brainpower.
He is speaking to you when you pull away: “Baby, just a second, wait right here, let me get something.” Gently you are pushed from his lap and he disappears into his room momentarily, leaving you waiting, alone, aching for him, until he rounds the corner again with a familiar foil packet, finding his way back to the couch and sweeping you on top of him once more.
“Hi. Sorry.” And now he is fully yours.
You whine and wiggle against him the second the condom is on.
“Shh,” Luigi whispers, “I got you, ‘s okay, gorgeous. Gonna take good care of you, yeah? Don’t you worry. Gonna give you just what you need, baby.”
The tip of his cock is pressing into you, then, slowly easing himself inside, and fuck, he fits just right, fills you up perfectly, has you seeing stars already. The sound you make when he bottoms out is a hop, skip, and a jump away from pornographic. Luigi purrs underneath you.
“Oh, I know, baby, I know.” His hand slides down to grip your ass, spreading you, and from this angle you can feel just how much he stretches you out. And then, as he begins to roll his hips: “My sweet girl, working so hard, can’t even think for yourself, can you, beautiful? That’s okay, baby. I can do all the thinking for you, you just sit back and let me work it out for you, yeah? Don’t think. Just let me please this pussy.”
It’s like he’s trying to kill you. Every single word he says into your ear shoots straight to your cunt, the mere sound of his voice so near you electrifying. He’s deep, and with your thighs spread wide like this you just have to take advantage of the perfect angle to rub your clit against him. You can’t help but squeal into the crook of his neck each time his hips ram up into you, thighs clapping against your ass; by the way his muscles tense you assume it must take much of his energy, and yet he pounds you like you weigh nothing in his lap, exerting himself like it’s a cakewalk so long as he can watch your face shrivel up with overwhelming delectation. You can tell that he loves it when you tug his hair or bite him, and so you do it every chance you get, just in case your hushed utterances in his ear fail to make your message clear enough:
“Luigi, fuckfuckfuck, oh my god, oh, fuck…”
As he paces himself Luigi wraps his strong arms around you, one caging your waist and the other pulling tight at your hair. Your neck is arched and exposed, leaving him free to smother his love all over you in sharp, uneven hickeys. You needed this, so, so bad, and you tell him exactly that, chanting thank you, thank you, thank you and holding him tight.
“Whatever you want,” he whispers. “You can have whatever you want with me. Anything.” His lips meet yours, fleeting, and then, with the slightest hint of a grin: “You earned this, baby.”
You groan directly into his ear. It’s straight from your dreams, you think, like you’ve been swept from your bed in the midst of the night and dropped right here, in the lap of the sweetest, smartest, most handsome boy you’ve ever so much as looked at, bouncing on his cock while he kisses you like you’ll float away if he lets go. The two of you work together to heighten each other’s inevitable undoing, like a function of sorts; Luigi pushes and you push back, meeting his hips every time, your clit brushing against him just right, and him breaching unknown depths of you, hands roaming, learning you inside and out.
“My sweet girl,” he grabs your face and rests his forehead against yours, driving into you with precision. “This is all yours, baby.”
Sweat starts to gather at his hairline and you can feel him shuddering in your arms. Kissing him, you press down on his toned chest, pinning him against the couch, and Luigi is practically singing for you, little grunts and babys and murmurs of your name traveling through your ears and echoing in your mind. You want this to last forever. His hips slow to a stop when you begin to move on your own; you raise yourself up, resting all your weight on your knees, with him sliding out of your cunt until just the tip is still inside—and then you drop down, letting him sink back into you quickly, slick and smooth, his cock so deep you can nearly feel it in your stomach.
Fuck. You love this. You love the way his hands grip your ass, your thighs, rubbing your back, moaning your name and kissing behind your ear. You love the way he looks at you. The pupils of those dark eyes are blown wide, watching you move, worshipping how your tits bounce, the gyration of your hips, the blush of arousal all over you, your bottom lip wedged between your teeth. The sounds of sex and the shameless way he takes in every feature of your body have you feeling hot and ready to burst. You moan his name, drawn out and raspy.
“Yes,” Luigi groans. “You’re so pretty on top of me.”
Even through the haze of your pleasure you smile at his praise. He is telling you everything, every single thought that passes by in his mind, as if there will be no proof of how good he fucked you once you leave his dorm, as if every word will dissipate into thin air and leave you waiting, unsatisfied, hanging on the edge: “You take it so well, baby, my sweet girl, so perfect, so perfect just for me.”
His big hands are all over you. One cups your breast, sucking your nipple into his mouth, with the other splayed over your hip. You start to feel dizzy, anxious for his attention, a little bit crazy. Close. Luigi must notice the way your eyes screw shut and your pussy squeezes him tight, because his hand moves down your chest, over your stomach, and then to your clit, circling his fingers with purpose. He wishes—almost—that you were beneath him, so that he could replace his hand with his mouth, trace down your body with his lips and bring you to your very edge with his tongue, over and over again, until you’re begging him to stop.
He settles instead for kissing you, hard, slowly, lingering. “You have no fucking idea how bad I’ve been wanting this, baby.”
You nod, moaning, “yes, yes, me too,” your noises pained and rough in your throat.
The way his cock slams into you with each movement of your hips is ruthless, bruising; he’s kissing you so sweetly and you can feel your climax churning in your abdomen, rippling through you. It knocks the air from your lungs. Sex with him hurts so good. It’s like nothing you’ve ever felt before.
“Gonna come,” you huff. There are fingernail-sized dents in his skin. “Gonna come for you.”
Luigi nods, whispers, “good girl, such a good girl,” and circles his fingers over your clit as fast as he can manage.
You tense around him at that. You can’t even count how many times you’ve come imagining those very words whispered in your ear by the very man that you’re riding right now.
“Fuck,” he hisses. “Yeah? You like that? You like being a good girl for me?”
You nod wildly, and everything feels so real all of a sudden, like you’ve been floating mindlessly in space and you are crashing down into reality. His teeth dig into the sensitive skin of your neck and his hips start to pump again and by the time he’s meeting your thrusts you’ve had enough, thighs shaking, and he starts moaning into your ear so that you know he’s right there with you, and fuck, he’s really trying to kill you—
Your orgasm hits you like a truck. A 5’11, dark haired and brown eyed muscle truck that looks at you like you are the only good thing left in the world.
For a moment there is only your deep panting and his equally spent breaths as the both of you rest, his hand tracing gentle patterns on your back, yours combing through his sweat-soaked curls. The dorm is quiet, calm, almost with an air of innocence, completely unswayed by the heady aftermath of what the two of you just did right there on the couch. You lean back and look into his eyes, brooding and trained entirely on you. And he has that stupid grin on his face, the one that gives both of you away for good, the one that screams we’re not the only ones who know what we’ve been up to.
You want to kiss it right off of his beautiful, beautiful face. But right now you just sigh, lean into his shoulder, and let him hold you tight. Tonight you will walk back to your dorm, all the way on the other side of campus, where your roommates will be waiting for you, likely getting ready for bed. You will walk inside and they will watch you without a clue as to whose hands have been on you, whose name has been on your lips, whose cock has been buried to the hilt inside of you for the past hour. Your legs will be aching—you are sure of it.
Your roommates will ask you, “how’d it go?”, completely unaware of what your wobbly smile really means, how you really spent your time with your cute tutor.
And you will respond, “oh, great,” with a barely masked giggle. “I’m gonna ace my test tomorrow.”
#luigi mangione x reader#luigi mangione imagine#luigi mangione fanfiction#luigi mangione fic#flig’s work
327 notes
·
View notes
Text
Countdown to JEE (Main): Week 2/33
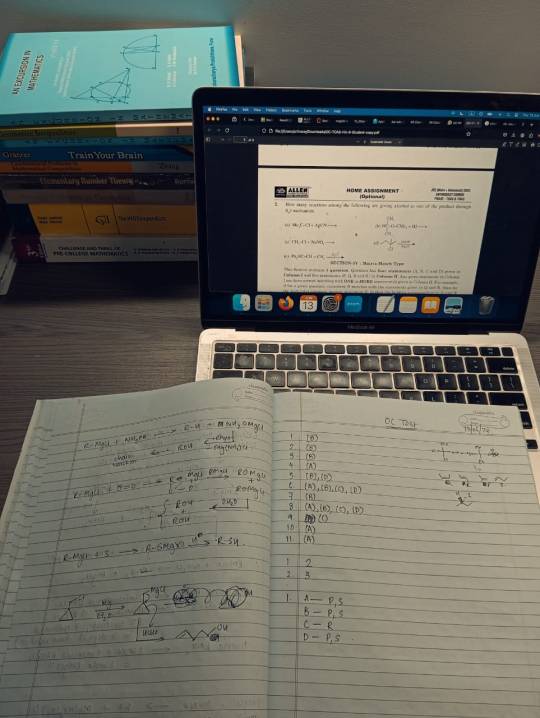
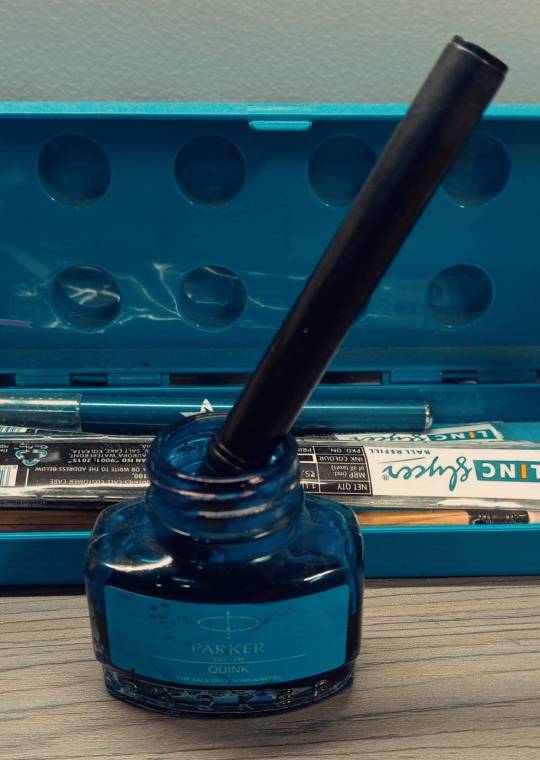
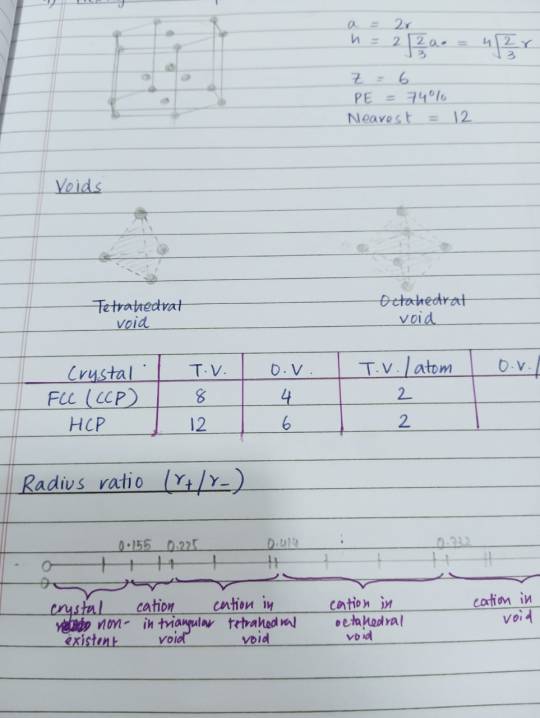
I've done a lot this week! Hit almost all the topics I wanted to. You'll notice that there are some topics I've covered but not solved questions for — these are the ones I studied for the first time and/or made notes for.
Other than that, I have my school unit tests from next Wednesday, so there's that. Sadness.....
Test results:
Test at physics tuition center: 116/120, rank 1/67! Yay!
Aryabhatta National Mathematics Competition: score unknown, result expected on 10/07/2024.
Topics covered:
Physics: Potential and Capacitance; Electromagnetic Waves, Waves on a String; Modern Physics; Gravitation; Electromagnetic Induction (6/3)
Chemistry: Chemical Thermodynamics; Atomic Structure; Chemical Equilibrium; Halogen Derivatives; Solid State (5/3)
Mathematics: Differential Equations; Area Under a Curve; Applications of Derivatives; Determinants; Complex Numbers; Ellipses; Hyperbola; Binomial Theorem; Functions (9/3)
Questions solved:
Physics: - FIITJEE Electrostatics* module, Assignment section — 56 questions, 52 correct - Allen Potential and Capacitance module, O1 and O2 — 88 questions, 77 correct -FIITJEE JEE (Main) archives, Electromagnetic Waves — 12 questions, 10 correct - Allen Waves on a String module, O1 and O2 — 67 questions, 59 correct - FIITJEE JEE (Main) archives, Modern Physics — 40 questions, 34 correct - Allen Gravitation module, O1 and O2 — 61 questions, 55 correct Total: 383/60 questions, 287 correct *FIITJEE includes Potential and Capacitance under Electrostatics, while Allen does not.
Chemistry: - Allen Chemical Thermodynamics module, S1 and S2 — 40 questions, 37 correct - R. N. Sarin, Atomic Structure — 18 questions, 18 correct - R. N. Sarin, Chemical Equilibrium — 11 questions, 11 correct - Allen Halogen Derivatives module, JEE (Advanced) archives — 24 questions, 22 correct Total: 93/60 questions, 88 correct
Mathematics: - Allen Differential Equations module, O1 — 30 questions, 25 correct - Allen Area Under a Curve module, Do Yourself 1, 2, 3, 4, 5 and O2 — 51 questions, 46 correct - FIITJEE JEE (Main) archives, Applications of Derivatives — 45 questions, 39 correct - FIITJEE JEE (Main) archives, Determinants — 22 questions, 17 correct - Yellow Book, Complex Numbers, single-choice questions — 20 questions, 17 correct - Pink Book, Ellipses, single-choice questions — 16 questions, 14 correct - Yellow Book, Binomial Theorem, single-choice questions — 15 questions, 12 correct - Pink Book, Hyperbolas, single-choice questions — 21 questions, 18 correct - Allen Functions module, O1 and JEE (Main) archives — 48 questions, 44 correct Total: 268/60 questions, 232 correct
GRAND TOTAL: 744/400 questions, 607 correct
Upcoming tests:
23/06/2024 (next Sunday) — Allen monthly test. Topics: Kinetic Theory of Gases; Physical Thermodynamics; Electrostatics; Potential and Capacitance; Current Electricity; Electromagnetic Induction; Alternating Current; Electromagnetic Waves; Waves on a String; Sound Waves; Ray Optics; Wave Optics; Circle; Functions; Differentiation; Applications of Derivatives; Indefinite Integrals; Definite Integrals; Area Under a Curve; Differential Equations; Matrices; Silicates; Molecules That Do Not Exist; Coordination Chemistry; Metallurgy; Electronic Displacement Effects; Halogen Derivatives; Atomic Structure; Chemical Equilibrium; Solid State; Solutions; Chemical Kinetics; Chemical Thermodynamics. Yeah. I've no idea how I'm going to finish the syllabus in time, since I haven't even started Coordination Chemistry, Metallurgy or Alternating Current — they finished the classes in Allen before I even joined the batch. Anyway, let's see.
That'll be all till next week — see you again!
#studyblr#desi studyblr#study blog#jee 2025#joint entrance examination#jee mains#jee advanced#weekly studyposting#porashona
26 notes
·
View notes
Text
◇ 38'th Day of Productivity ◇


10/03/2024
To Do List:-
□ Physics Homework.
□ Revise Matrices.
□ Correct Maths Notebook.
□ Write Psychology Notes.
□ Pratice some questions from English.
□ Read 'Solutions' from Chemistry.
Update:-
Classes actually started on 29'th of February, but I decided to take a break from posting as it would take some time to get used to the more rigorous schedule of 12'th standard.
I finally saw a psychologist today after a long time so let's see how that goes!
I will change the godawful color scheme of this page as soon as I can, lol.
Remark/s 1:-
A bit demotivated today
Remark/s:-
Not bad for being so tired today!
Song of the Day:-
Such a simple composition yet captures so many complex feelings!
#studyblr#study blog#desi studyblr#indian studyblr#study aesthetic#studyspo#study#studying#11th std#100 days of productivity#studystudystudy#study notes#study motivation#science#studygram#spotify#song#music recs#rammstein#wilder wein
39 notes
·
View notes
Text
UNLOCKING THE POWER OF AI WITH EASYLIBPAL 2/2
EXPANDED COMPONENTS AND DETAILS OF EASYLIBPAL:
1. Easylibpal Class: The core component of the library, responsible for handling algorithm selection, model fitting, and prediction generation
2. Algorithm Selection and Support:
Supports classic AI algorithms such as Linear Regression, Logistic Regression, Support Vector Machine (SVM), Naive Bayes, and K-Nearest Neighbors (K-NN).
and
- Decision Trees
- Random Forest
- AdaBoost
- Gradient Boosting
3. Integration with Popular Libraries: Seamless integration with essential Python libraries like NumPy, Pandas, Matplotlib, and Scikit-learn for enhanced functionality.
4. Data Handling:
- DataLoader class for importing and preprocessing data from various formats (CSV, JSON, SQL databases).
- DataTransformer class for feature scaling, normalization, and encoding categorical variables.
- Includes functions for loading and preprocessing datasets to prepare them for training and testing.
- `FeatureSelector` class: Provides methods for feature selection and dimensionality reduction.
5. Model Evaluation:
- Evaluator class to assess model performance using metrics like accuracy, precision, recall, F1-score, and ROC-AUC.
- Methods for generating confusion matrices and classification reports.
6. Model Training: Contains methods for fitting the selected algorithm with the training data.
- `fit` method: Trains the selected algorithm on the provided training data.
7. Prediction Generation: Allows users to make predictions using the trained model on new data.
- `predict` method: Makes predictions using the trained model on new data.
- `predict_proba` method: Returns the predicted probabilities for classification tasks.
8. Model Evaluation:
- `Evaluator` class: Assesses model performance using various metrics (e.g., accuracy, precision, recall, F1-score, ROC-AUC).
- `cross_validate` method: Performs cross-validation to evaluate the model's performance.
- `confusion_matrix` method: Generates a confusion matrix for classification tasks.
- `classification_report` method: Provides a detailed classification report.
9. Hyperparameter Tuning:
- Tuner class that uses techniques likes Grid Search and Random Search for hyperparameter optimization.
10. Visualization:
- Integration with Matplotlib and Seaborn for generating plots to analyze model performance and data characteristics.
- Visualization support: Enables users to visualize data, model performance, and predictions using plotting functionalities.
- `Visualizer` class: Integrates with Matplotlib and Seaborn to generate plots for model performance analysis and data visualization.
- `plot_confusion_matrix` method: Visualizes the confusion matrix.
- `plot_roc_curve` method: Plots the Receiver Operating Characteristic (ROC) curve.
- `plot_feature_importance` method: Visualizes feature importance for applicable algorithms.
11. Utility Functions:
- Functions for saving and loading trained models.
- Logging functionalities to track the model training and prediction processes.
- `save_model` method: Saves the trained model to a file.
- `load_model` method: Loads a previously trained model from a file.
- `set_logger` method: Configures logging functionality for tracking model training and prediction processes.
12. User-Friendly Interface: Provides a simplified and intuitive interface for users to interact with and apply classic AI algorithms without extensive knowledge or configuration.
13.. Error Handling: Incorporates mechanisms to handle invalid inputs, errors during training, and other potential issues during algorithm usage.
- Custom exception classes for handling specific errors and providing informative error messages to users.
14. Documentation: Comprehensive documentation to guide users on how to use Easylibpal effectively and efficiently
- Comprehensive documentation explaining the usage and functionality of each component.
- Example scripts demonstrating how to use Easylibpal for various AI tasks and datasets.
15. Testing Suite:
- Unit tests for each component to ensure code reliability and maintainability.
- Integration tests to verify the smooth interaction between different components.
IMPLEMENTATION EXAMPLE WITH ADDITIONAL FEATURES:
Here is an example of how the expanded Easylibpal library could be structured and used:
```python
import numpy as np
import pandas as pd
from sklearn.model_selection import train_test_split
from sklearn.preprocessing import StandardScaler
from easylibpal import Easylibpal, DataLoader, Evaluator, Tuner
# Example DataLoader
class DataLoader:
def load_data(self, filepath, file_type='csv'):
if file_type == 'csv':
return pd.read_csv(filepath)
else:
raise ValueError("Unsupported file type provided.")
# Example Evaluator
class Evaluator:
def evaluate(self, model, X_test, y_test):
predictions = model.predict(X_test)
accuracy = np.mean(predictions == y_test)
return {'accuracy': accuracy}
# Example usage of Easylibpal with DataLoader and Evaluator
if __name__ == "__main__":
# Load and prepare the data
data_loader = DataLoader()
data = data_loader.load_data('path/to/your/data.csv')
X = data.iloc[:, :-1]
y = data.iloc[:, -1]
X_train, X_test, y_train, y_test = train_test_split(X, y, test_size=0.2, random_state=42)
# Scale features
scaler = StandardScaler()
X_train_scaled = scaler.fit_transform(X_train)
X_test_scaled = scaler.transform(X_test)
# Initialize Easylibpal with the desired algorithm
model = Easylibpal('Random Forest')
model.fit(X_train_scaled, y_train)
# Evaluate the model
evaluator = Evaluator()
results = evaluator.evaluate(model, X_test_scaled, y_test)
print(f"Model Accuracy: {results['accuracy']}")
# Optional: Use Tuner for hyperparameter optimization
tuner = Tuner(model, param_grid={'n_estimators': [100, 200], 'max_depth': [10, 20, 30]})
best_params = tuner.optimize(X_train_scaled, y_train)
print(f"Best Parameters: {best_params}")
```
This example demonstrates the structured approach to using Easylibpal with enhanced data handling, model evaluation, and optional hyperparameter tuning. The library empowers users to handle real-world datasets, apply various machine learning algorithms, and evaluate their performance with ease, making it an invaluable tool for developers and data scientists aiming to implement AI solutions efficiently.
Easylibpal is dedicated to making the latest AI technology accessible to everyone, regardless of their background or expertise. Our platform simplifies the process of selecting and implementing classic AI algorithms, enabling users across various industries to harness the power of artificial intelligence with ease. By democratizing access to AI, we aim to accelerate innovation and empower users to achieve their goals with confidence. Easylibpal's approach involves a democratization framework that reduces entry barriers, lowers the cost of building AI solutions, and speeds up the adoption of AI in both academic and business settings.
Below are examples showcasing how each main component of the Easylibpal library could be implemented and used in practice to provide a user-friendly interface for utilizing classic AI algorithms.
1. Core Components
Easylibpal Class Example:
```python
class Easylibpal:
def __init__(self, algorithm):
self.algorithm = algorithm
self.model = None
def fit(self, X, y):
# Simplified example: Instantiate and train a model based on the selected algorithm
if self.algorithm == 'Linear Regression':
from sklearn.linear_model import LinearRegression
self.model = LinearRegression()
elif self.algorithm == 'Random Forest':
from sklearn.ensemble import RandomForestClassifier
self.model = RandomForestClassifier()
self.model.fit(X, y)
def predict(self, X):
return self.model.predict(X)
```
2. Data Handling
DataLoader Class Example:
```python
class DataLoader:
def load_data(self, filepath, file_type='csv'):
if file_type == 'csv':
import pandas as pd
return pd.read_csv(filepath)
else:
raise ValueError("Unsupported file type provided.")
```
3. Model Evaluation
Evaluator Class Example:
```python
from sklearn.metrics import accuracy_score, classification_report
class Evaluator:
def evaluate(self, model, X_test, y_test):
predictions = model.predict(X_test)
accuracy = accuracy_score(y_test, predictions)
report = classification_report(y_test, predictions)
return {'accuracy': accuracy, 'report': report}
```
4. Hyperparameter Tuning
Tuner Class Example:
```python
from sklearn.model_selection import GridSearchCV
class Tuner:
def __init__(self, model, param_grid):
self.model = model
self.param_grid = param_grid
def optimize(self, X, y):
grid_search = GridSearchCV(self.model, self.param_grid, cv=5)
grid_search.fit(X, y)
return grid_search.best_params_
```
5. Visualization
Visualizer Class Example:
```python
import matplotlib.pyplot as plt
class Visualizer:
def plot_confusion_matrix(self, cm, classes, normalize=False, title='Confusion matrix'):
plt.imshow(cm, interpolation='nearest', cmap=plt.cm.Blues)
plt.title(title)
plt.colorbar()
tick_marks = np.arange(len(classes))
plt.xticks(tick_marks, classes, rotation=45)
plt.yticks(tick_marks, classes)
plt.ylabel('True label')
plt.xlabel('Predicted label')
plt.show()
```
6. Utility Functions
Save and Load Model Example:
```python
import joblib
def save_model(model, filename):
joblib.dump(model, filename)
def load_model(filename):
return joblib.load(filename)
```
7. Example Usage Script
Using Easylibpal in a Script:
```python
# Assuming Easylibpal and other classes have been imported
data_loader = DataLoader()
data = data_loader.load_data('data.csv')
X = data.drop('Target', axis=1)
y = data['Target']
model = Easylibpal('Random Forest')
model.fit(X, y)
evaluator = Evaluator()
results = evaluator.evaluate(model, X, y)
print("Accuracy:", results['accuracy'])
print("Report:", results['report'])
visualizer = Visualizer()
visualizer.plot_confusion_matrix(results['cm'], classes=['Class1', 'Class2'])
save_model(model, 'trained_model.pkl')
loaded_model = load_model('trained_model.pkl')
```
These examples illustrate the practical implementation and use of the Easylibpal library components, aiming to simplify the application of AI algorithms for users with varying levels of expertise in machine learning.
EASYLIBPAL IMPLEMENTATION:
Step 1: Define the Problem
First, we need to define the problem we want to solve. For this POC, let's assume we want to predict house prices based on various features like the number of bedrooms, square footage, and location.
Step 2: Choose an Appropriate Algorithm
Given our problem, a supervised learning algorithm like linear regression would be suitable. We'll use Scikit-learn, a popular library for machine learning in Python, to implement this algorithm.
Step 3: Prepare Your Data
We'll use Pandas to load and prepare our dataset. This involves cleaning the data, handling missing values, and splitting the dataset into training and testing sets.
Step 4: Implement the Algorithm
Now, we'll use Scikit-learn to implement the linear regression algorithm. We'll train the model on our training data and then test its performance on the testing data.
Step 5: Evaluate the Model
Finally, we'll evaluate the performance of our model using metrics like Mean Squared Error (MSE) and R-squared.
Python Code POC
```python
import numpy as np
import pandas as pd
from sklearn.model_selection import train_test_split
from sklearn.linear_model import LinearRegression
from sklearn.metrics import mean_squared_error, r2_score
# Load the dataset
data = pd.read_csv('house_prices.csv')
# Prepare the data
X = data'bedrooms', 'square_footage', 'location'
y = data['price']
# Split the data into training and testing sets
X_train, X_test, y_train, y_test = train_test_split(X, y, test_size=0.2, random_state=42)
# Create and train the model
model = LinearRegression()
model.fit(X_train, y_train)
# Make predictions
predictions = model.predict(X_test)
# Evaluate the model
mse = mean_squared_error(y_test, predictions)
r2 = r2_score(y_test, predictions)
print(f'Mean Squared Error: {mse}')
print(f'R-squared: {r2}')
```
Below is an implementation, Easylibpal provides a simple interface to instantiate and utilize classic AI algorithms such as Linear Regression, Logistic Regression, SVM, Naive Bayes, and K-NN. Users can easily create an instance of Easylibpal with their desired algorithm, fit the model with training data, and make predictions, all with minimal code and hassle. This demonstrates the power of Easylibpal in simplifying the integration of AI algorithms for various tasks.
```python
# Import necessary libraries
import numpy as np
import pandas as pd
import matplotlib.pyplot as plt
from sklearn.linear_model import LinearRegression
from sklearn.linear_model import LogisticRegression
from sklearn.svm import SVC
from sklearn.naive_bayes import GaussianNB
from sklearn.neighbors import KNeighborsClassifier
class Easylibpal:
def __init__(self, algorithm):
self.algorithm = algorithm
def fit(self, X, y):
if self.algorithm == 'Linear Regression':
self.model = LinearRegression()
elif self.algorithm == 'Logistic Regression':
self.model = LogisticRegression()
elif self.algorithm == 'SVM':
self.model = SVC()
elif self.algorithm == 'Naive Bayes':
self.model = GaussianNB()
elif self.algorithm == 'K-NN':
self.model = KNeighborsClassifier()
else:
raise ValueError("Invalid algorithm specified.")
self.model.fit(X, y)
def predict(self, X):
return self.model.predict(X)
# Example usage:
# Initialize Easylibpal with the desired algorithm
easy_algo = Easylibpal('Linear Regression')
# Generate some sample data
X = np.array([[1], [2], [3], [4]])
y = np.array([2, 4, 6, 8])
# Fit the model
easy_algo.fit(X, y)
# Make predictions
predictions = easy_algo.predict(X)
# Plot the results
plt.scatter(X, y)
plt.plot(X, predictions, color='red')
plt.title('Linear Regression with Easylibpal')
plt.xlabel('X')
plt.ylabel('y')
plt.show()
```
Easylibpal is an innovative Python library designed to simplify the integration and use of classic AI algorithms in a user-friendly manner. It aims to bridge the gap between the complexity of AI libraries and the ease of use, making it accessible for developers and data scientists alike. Easylibpal abstracts the underlying complexity of each algorithm, providing a unified interface that allows users to apply these algorithms with minimal configuration and understanding of the underlying mechanisms.
ENHANCED DATASET HANDLING
Easylibpal should be able to handle datasets more efficiently. This includes loading datasets from various sources (e.g., CSV files, databases), preprocessing data (e.g., normalization, handling missing values), and splitting data into training and testing sets.
```python
import os
from sklearn.model_selection import train_test_split
class Easylibpal:
# Existing code...
def load_dataset(self, filepath):
"""Loads a dataset from a CSV file."""
if not os.path.exists(filepath):
raise FileNotFoundError("Dataset file not found.")
return pd.read_csv(filepath)
def preprocess_data(self, dataset):
"""Preprocesses the dataset."""
# Implement data preprocessing steps here
return dataset
def split_data(self, X, y, test_size=0.2):
"""Splits the dataset into training and testing sets."""
return train_test_split(X, y, test_size=test_size)
```
Additional Algorithms
Easylibpal should support a wider range of algorithms. This includes decision trees, random forests, and gradient boosting machines.
```python
from sklearn.tree import DecisionTreeClassifier
from sklearn.ensemble import RandomForestClassifier
from sklearn.ensemble import GradientBoostingClassifier
class Easylibpal:
# Existing code...
def fit(self, X, y):
# Existing code...
elif self.algorithm == 'Decision Tree':
self.model = DecisionTreeClassifier()
elif self.algorithm == 'Random Forest':
self.model = RandomForestClassifier()
elif self.algorithm == 'Gradient Boosting':
self.model = GradientBoostingClassifier()
# Add more algorithms as needed
```
User-Friendly Features
To make Easylibpal even more user-friendly, consider adding features like:
- Automatic hyperparameter tuning: Implementing a simple interface for hyperparameter tuning using GridSearchCV or RandomizedSearchCV.
- Model evaluation metrics: Providing easy access to common evaluation metrics like accuracy, precision, recall, and F1 score.
- Visualization tools: Adding methods for plotting model performance, confusion matrices, and feature importance.
```python
from sklearn.metrics import accuracy_score, classification_report
from sklearn.model_selection import GridSearchCV
class Easylibpal:
# Existing code...
def evaluate_model(self, X_test, y_test):
"""Evaluates the model using accuracy and classification report."""
y_pred = self.predict(X_test)
print("Accuracy:", accuracy_score(y_test, y_pred))
print(classification_report(y_test, y_pred))
def tune_hyperparameters(self, X, y, param_grid):
"""Tunes the model's hyperparameters using GridSearchCV."""
grid_search = GridSearchCV(self.model, param_grid, cv=5)
grid_search.fit(X, y)
self.model = grid_search.best_estimator_
```
Easylibpal leverages the power of Python and its rich ecosystem of AI and machine learning libraries, such as scikit-learn, to implement the classic algorithms. It provides a high-level API that abstracts the specifics of each algorithm, allowing users to focus on the problem at hand rather than the intricacies of the algorithm.
Python Code Snippets for Easylibpal
Below are Python code snippets demonstrating the use of Easylibpal with classic AI algorithms. Each snippet demonstrates how to use Easylibpal to apply a specific algorithm to a dataset.
# Linear Regression
```python
from Easylibpal import Easylibpal
# Initialize Easylibpal with a dataset
Easylibpal = Easylibpal(dataset='your_dataset.csv')
# Apply Linear Regression
result = Easylibpal.apply_algorithm('linear_regression', target_column='target')
# Print the result
print(result)
```
# Logistic Regression
```python
from Easylibpal import Easylibpal
# Initialize Easylibpal with a dataset
Easylibpal = Easylibpal(dataset='your_dataset.csv')
# Apply Logistic Regression
result = Easylibpal.apply_algorithm('logistic_regression', target_column='target')
# Print the result
print(result)
```
# Support Vector Machines (SVM)
```python
from Easylibpal import Easylibpal
# Initialize Easylibpal with a dataset
Easylibpal = Easylibpal(dataset='your_dataset.csv')
# Apply SVM
result = Easylibpal.apply_algorithm('svm', target_column='target')
# Print the result
print(result)
```
# Naive Bayes
```python
from Easylibpal import Easylibpal
# Initialize Easylibpal with a dataset
Easylibpal = Easylibpal(dataset='your_dataset.csv')
# Apply Naive Bayes
result = Easylibpal.apply_algorithm('naive_bayes', target_column='target')
# Print the result
print(result)
```
# K-Nearest Neighbors (K-NN)
```python
from Easylibpal import Easylibpal
# Initialize Easylibpal with a dataset
Easylibpal = Easylibpal(dataset='your_dataset.csv')
# Apply K-NN
result = Easylibpal.apply_algorithm('knn', target_column='target')
# Print the result
print(result)
```
ABSTRACTION AND ESSENTIAL COMPLEXITY
- Essential Complexity: This refers to the inherent complexity of the problem domain, which cannot be reduced regardless of the programming language or framework used. It includes the logic and algorithm needed to solve the problem. For example, the essential complexity of sorting a list remains the same across different programming languages.
- Accidental Complexity: This is the complexity introduced by the choice of programming language, framework, or libraries. It can be reduced or eliminated through abstraction. For instance, using a high-level API in Python can hide the complexity of lower-level operations, making the code more readable and maintainable.
HOW EASYLIBPAL ABSTRACTS COMPLEXITY
Easylibpal aims to reduce accidental complexity by providing a high-level API that encapsulates the details of each classic AI algorithm. This abstraction allows users to apply these algorithms without needing to understand the underlying mechanisms or the specifics of the algorithm's implementation.
- Simplified Interface: Easylibpal offers a unified interface for applying various algorithms, such as Linear Regression, Logistic Regression, SVM, Naive Bayes, and K-NN. This interface abstracts the complexity of each algorithm, making it easier for users to apply them to their datasets.
- Runtime Fusion: By evaluating sub-expressions and sharing them across multiple terms, Easylibpal can optimize the execution of algorithms. This approach, similar to runtime fusion in abstract algorithms, allows for efficient computation without duplicating work, thereby reducing the computational complexity.
- Focus on Essential Complexity: While Easylibpal abstracts away the accidental complexity; it ensures that the essential complexity of the problem domain remains at the forefront. This means that while the implementation details are hidden, the core logic and algorithmic approach are still accessible and understandable to the user.
To implement Easylibpal, one would need to create a Python class that encapsulates the functionality of each classic AI algorithm. This class would provide methods for loading datasets, preprocessing data, and applying the algorithm with minimal configuration required from the user. The implementation would leverage existing libraries like scikit-learn for the actual algorithmic computations, abstracting away the complexity of these libraries.
Here's a conceptual example of how the Easylibpal class might be structured for applying a Linear Regression algorithm:
```python
class Easylibpal:
def __init__(self, dataset):
self.dataset = dataset
# Load and preprocess the dataset
def apply_linear_regression(self, target_column):
# Abstracted implementation of Linear Regression
# This method would internally use scikit-learn or another library
# to perform the actual computation, abstracting the complexity
pass
# Usage
Easylibpal = Easylibpal(dataset='your_dataset.csv')
result = Easylibpal.apply_linear_regression(target_column='target')
```
This example demonstrates the concept of Easylibpal by abstracting the complexity of applying a Linear Regression algorithm. The actual implementation would need to include the specifics of loading the dataset, preprocessing it, and applying the algorithm using an underlying library like scikit-learn.
Easylibpal abstracts the complexity of classic AI algorithms by providing a simplified interface that hides the intricacies of each algorithm's implementation. This abstraction allows users to apply these algorithms with minimal configuration and understanding of the underlying mechanisms. Here are examples of specific algorithms that Easylibpal abstracts:
To implement Easylibpal, one would need to create a Python class that encapsulates the functionality of each classic AI algorithm. This class would provide methods for loading datasets, preprocessing data, and applying the algorithm with minimal configuration required from the user. The implementation would leverage existing libraries like scikit-learn for the actual algorithmic computations, abstracting away the complexity of these libraries.
Here's a conceptual example of how the Easylibpal class might be structured for applying a Linear Regression algorithm:
```python
class Easylibpal:
def __init__(self, dataset):
self.dataset = dataset
# Load and preprocess the dataset
def apply_linear_regression(self, target_column):
# Abstracted implementation of Linear Regression
# This method would internally use scikit-learn or another library
# to perform the actual computation, abstracting the complexity
pass
# Usage
Easylibpal = Easylibpal(dataset='your_dataset.csv')
result = Easylibpal.apply_linear_regression(target_column='target')
```
This example demonstrates the concept of Easylibpal by abstracting the complexity of applying a Linear Regression algorithm. The actual implementation would need to include the specifics of loading the dataset, preprocessing it, and applying the algorithm using an underlying library like scikit-learn.
Easylibpal abstracts the complexity of feature selection for classic AI algorithms by providing a simplified interface that automates the process of selecting the most relevant features for each algorithm. This abstraction is crucial because feature selection is a critical step in machine learning that can significantly impact the performance of a model. Here's how Easylibpal handles feature selection for the mentioned algorithms:
To implement feature selection in Easylibpal, one could use scikit-learn's `SelectKBest` or `RFE` classes for feature selection based on statistical tests or model coefficients. Here's a conceptual example of how feature selection might be integrated into the Easylibpal class for Linear Regression:
```python
from sklearn.feature_selection import SelectKBest, f_regression
from sklearn.linear_model import LinearRegression
class Easylibpal:
def __init__(self, dataset):
self.dataset = dataset
# Load and preprocess the dataset
def apply_linear_regression(self, target_column):
# Feature selection using SelectKBest
selector = SelectKBest(score_func=f_regression, k=10)
X_new = selector.fit_transform(self.dataset.drop(target_column, axis=1), self.dataset[target_column])
# Train Linear Regression model
model = LinearRegression()
model.fit(X_new, self.dataset[target_column])
# Return the trained model
return model
# Usage
Easylibpal = Easylibpal(dataset='your_dataset.csv')
model = Easylibpal.apply_linear_regression(target_column='target')
```
This example demonstrates how Easylibpal abstracts the complexity of feature selection for Linear Regression by using scikit-learn's `SelectKBest` to select the top 10 features based on their statistical significance in predicting the target variable. The actual implementation would need to adapt this approach for each algorithm, considering the specific characteristics and requirements of each algorithm.
To implement feature selection in Easylibpal, one could use scikit-learn's `SelectKBest`, `RFE`, or other feature selection classes based on the algorithm's requirements. Here's a conceptual example of how feature selection might be integrated into the Easylibpal class for Logistic Regression using RFE:
```python
from sklearn.feature_selection import RFE
from sklearn.linear_model import LogisticRegression
class Easylibpal:
def __init__(self, dataset):
self.dataset = dataset
# Load and preprocess the dataset
def apply_logistic_regression(self, target_column):
# Feature selection using RFE
model = LogisticRegression()
rfe = RFE(model, n_features_to_select=10)
rfe.fit(self.dataset.drop(target_column, axis=1), self.dataset[target_column])
# Train Logistic Regression model
model.fit(self.dataset.drop(target_column, axis=1), self.dataset[target_column])
# Return the trained model
return model
# Usage
Easylibpal = Easylibpal(dataset='your_dataset.csv')
model = Easylibpal.apply_logistic_regression(target_column='target')
```
This example demonstrates how Easylibpal abstracts the complexity of feature selection for Logistic Regression by using scikit-learn's `RFE` to select the top 10 features based on their importance in the model. The actual implementation would need to adapt this approach for each algorithm, considering the specific characteristics and requirements of each algorithm.
EASYLIBPAL HANDLES DIFFERENT TYPES OF DATASETS
Easylibpal handles different types of datasets with varying structures by adopting a flexible and adaptable approach to data preprocessing and transformation. This approach is inspired by the principles of tidy data and the need to ensure data is in a consistent, usable format before applying AI algorithms. Here's how Easylibpal addresses the challenges posed by varying dataset structures:
One Type in Multiple Tables
When datasets contain different variables, the same variables with different names, different file formats, or different conventions for missing values, Easylibpal employs a process similar to tidying data. This involves identifying and standardizing the structure of each dataset, ensuring that each variable is consistently named and formatted across datasets. This process might include renaming columns, converting data types, and handling missing values in a uniform manner. For datasets stored in different file formats, Easylibpal would use appropriate libraries (e.g., pandas for CSV, Excel files, and SQL databases) to load and preprocess the data before applying the algorithms.
Multiple Types in One Table
For datasets that involve values collected at multiple levels or on different types of observational units, Easylibpal applies a normalization process. This involves breaking down the dataset into multiple tables, each representing a distinct type of observational unit. For example, if a dataset contains information about songs and their rankings over time, Easylibpal would separate this into two tables: one for song details and another for rankings. This normalization ensures that each fact is expressed in only one place, reducing inconsistencies and making the data more manageable for analysis.
Data Semantics
Easylibpal ensures that the data is organized in a way that aligns with the principles of data semantics, where every value belongs to a variable and an observation. This organization is crucial for the algorithms to interpret the data correctly. Easylibpal might use functions like `pivot_longer` and `pivot_wider` from the tidyverse or equivalent functions in pandas to reshape the data into a long format, where each row represents a single observation and each column represents a single variable. This format is particularly useful for algorithms that require a consistent structure for input data.
Messy Data
Dealing with messy data, which can include inconsistent data types, missing values, and outliers, is a common challenge in data science. Easylibpal addresses this by implementing robust data cleaning and preprocessing steps. This includes handling missing values (e.g., imputation or deletion), converting data types to ensure consistency, and identifying and removing outliers. These steps are crucial for preparing the data in a format that is suitable for the algorithms, ensuring that the algorithms can effectively learn from the data without being hindered by its inconsistencies.
To implement these principles in Python, Easylibpal would leverage libraries like pandas for data manipulation and preprocessing. Here's a conceptual example of how Easylibpal might handle a dataset with multiple types in one table:
```python
import pandas as pd
# Load the dataset
dataset = pd.read_csv('your_dataset.csv')
# Normalize the dataset by separating it into two tables
song_table = dataset'artist', 'track'.drop_duplicates().reset_index(drop=True)
song_table['song_id'] = range(1, len(song_table) + 1)
ranking_table = dataset'artist', 'track', 'week', 'rank'.drop_duplicates().reset_index(drop=True)
# Now, song_table and ranking_table can be used separately for analysis
```
This example demonstrates how Easylibpal might normalize a dataset with multiple types of observational units into separate tables, ensuring that each type of observational unit is stored in its own table. The actual implementation would need to adapt this approach based on the specific structure and requirements of the dataset being processed.
CLEAN DATA
Easylibpal employs a comprehensive set of data cleaning and preprocessing steps to handle messy data, ensuring that the data is in a suitable format for machine learning algorithms. These steps are crucial for improving the accuracy and reliability of the models, as well as preventing misleading results and conclusions. Here's a detailed look at the specific steps Easylibpal might employ:
1. Remove Irrelevant Data
The first step involves identifying and removing data that is not relevant to the analysis or modeling task at hand. This could include columns or rows that do not contribute to the predictive power of the model or are not necessary for the analysis .
2. Deduplicate Data
Deduplication is the process of removing duplicate entries from the dataset. Duplicates can skew the analysis and lead to incorrect conclusions. Easylibpal would use appropriate methods to identify and remove duplicates, ensuring that each entry in the dataset is unique.
3. Fix Structural Errors
Structural errors in the dataset, such as inconsistent data types, incorrect values, or formatting issues, can significantly impact the performance of machine learning algorithms. Easylibpal would employ data cleaning techniques to correct these errors, ensuring that the data is consistent and correctly formatted.
4. Deal with Missing Data
Handling missing data is a common challenge in data preprocessing. Easylibpal might use techniques such as imputation (filling missing values with statistical estimates like mean, median, or mode) or deletion (removing rows or columns with missing values) to address this issue. The choice of method depends on the nature of the data and the specific requirements of the analysis.
5. Filter Out Data Outliers
Outliers can significantly affect the performance of machine learning models. Easylibpal would use statistical methods to identify and filter out outliers, ensuring that the data is more representative of the population being analyzed.
6. Validate Data
The final step involves validating the cleaned and preprocessed data to ensure its quality and accuracy. This could include checking for consistency, verifying the correctness of the data, and ensuring that the data meets the requirements of the machine learning algorithms. Easylibpal would employ validation techniques to confirm that the data is ready for analysis.
To implement these data cleaning and preprocessing steps in Python, Easylibpal would leverage libraries like pandas and scikit-learn. Here's a conceptual example of how these steps might be integrated into the Easylibpal class:
```python
import pandas as pd
from sklearn.impute import SimpleImputer
from sklearn.preprocessing import StandardScaler
class Easylibpal:
def __init__(self, dataset):
self.dataset = dataset
# Load and preprocess the dataset
def clean_and_preprocess(self):
# Remove irrelevant data
self.dataset = self.dataset.drop(['irrelevant_column'], axis=1)
# Deduplicate data
self.dataset = self.dataset.drop_duplicates()
# Fix structural errors (example: correct data type)
self.dataset['correct_data_type_column'] = self.dataset['correct_data_type_column'].astype(float)
# Deal with missing data (example: imputation)
imputer = SimpleImputer(strategy='mean')
self.dataset['missing_data_column'] = imputer.fit_transform(self.dataset'missing_data_column')
# Filter out data outliers (example: using Z-score)
# This step requires a more detailed implementation based on the specific dataset
# Validate data (example: checking for NaN values)
assert not self.dataset.isnull().values.any(), "Data still contains NaN values"
# Return the cleaned and preprocessed dataset
return self.dataset
# Usage
Easylibpal = Easylibpal(dataset=pd.read_csv('your_dataset.csv'))
cleaned_dataset = Easylibpal.clean_and_preprocess()
```
This example demonstrates a simplified approach to data cleaning and preprocessing within Easylibpal. The actual implementation would need to adapt these steps based on the specific characteristics and requirements of the dataset being processed.
VALUE DATA
Easylibpal determines which data is irrelevant and can be removed through a combination of domain knowledge, data analysis, and automated techniques. The process involves identifying data that does not contribute to the analysis, research, or goals of the project, and removing it to improve the quality, efficiency, and clarity of the data. Here's how Easylibpal might approach this:
Domain Knowledge
Easylibpal leverages domain knowledge to identify data that is not relevant to the specific goals of the analysis or modeling task. This could include data that is out of scope, outdated, duplicated, or erroneous. By understanding the context and objectives of the project, Easylibpal can systematically exclude data that does not add value to the analysis.
Data Analysis
Easylibpal employs data analysis techniques to identify irrelevant data. This involves examining the dataset to understand the relationships between variables, the distribution of data, and the presence of outliers or anomalies. Data that does not have a significant impact on the predictive power of the model or the insights derived from the analysis is considered irrelevant.
Automated Techniques
Easylibpal uses automated tools and methods to remove irrelevant data. This includes filtering techniques to select or exclude certain rows or columns based on criteria or conditions, aggregating data to reduce its complexity, and deduplicating to remove duplicate entries. Tools like Excel, Google Sheets, Tableau, Power BI, OpenRefine, Python, R, Data Linter, Data Cleaner, and Data Wrangler can be employed for these purposes .
Examples of Irrelevant Data
- Personal Identifiable Information (PII): Data such as names, addresses, and phone numbers are irrelevant for most analytical purposes and should be removed to protect privacy and comply with data protection regulations .
- URLs and HTML Tags: These are typically not relevant to the analysis and can be removed to clean up the dataset.
- Boilerplate Text: Excessive blank space or boilerplate text (e.g., in emails) adds noise to the data and can be removed.
- Tracking Codes: These are used for tracking user interactions and do not contribute to the analysis.
To implement these steps in Python, Easylibpal might use pandas for data manipulation and filtering. Here's a conceptual example of how to remove irrelevant data:
```python
import pandas as pd
# Load the dataset
dataset = pd.read_csv('your_dataset.csv')
# Remove irrelevant columns (example: email addresses)
dataset = dataset.drop(['email_address'], axis=1)
# Remove rows with missing values (example: if a column is required for analysis)
dataset = dataset.dropna(subset=['required_column'])
# Deduplicate data
dataset = dataset.drop_duplicates()
# Return the cleaned dataset
cleaned_dataset = dataset
```
This example demonstrates how Easylibpal might remove irrelevant data from a dataset using Python and pandas. The actual implementation would need to adapt these steps based on the specific characteristics and requirements of the dataset being processed.
Detecting Inconsistencies
Easylibpal starts by detecting inconsistencies in the data. This involves identifying discrepancies in data types, missing values, duplicates, and formatting errors. By detecting these inconsistencies, Easylibpal can take targeted actions to address them.
Handling Formatting Errors
Formatting errors, such as inconsistent data types for the same feature, can significantly impact the analysis. Easylibpal uses functions like `astype()` in pandas to convert data types, ensuring uniformity and consistency across the dataset. This step is crucial for preparing the data for analysis, as it ensures that each feature is in the correct format expected by the algorithms.
Handling Missing Values
Missing values are a common issue in datasets. Easylibpal addresses this by consulting with subject matter experts to understand why data might be missing. If the missing data is missing completely at random, Easylibpal might choose to drop it. However, for other cases, Easylibpal might employ imputation techniques to fill in missing values, ensuring that the dataset is complete and ready for analysis.
Handling Duplicates
Duplicate entries can skew the analysis and lead to incorrect conclusions. Easylibpal uses pandas to identify and remove duplicates, ensuring that each entry in the dataset is unique. This step is crucial for maintaining the integrity of the data and ensuring that the analysis is based on distinct observations.
Handling Inconsistent Values
Inconsistent values, such as different representations of the same concept (e.g., "yes" vs. "y" for a binary variable), can also pose challenges. Easylibpal employs data cleaning techniques to standardize these values, ensuring that the data is consistent and can be accurately analyzed.
To implement these steps in Python, Easylibpal would leverage pandas for data manipulation and preprocessing. Here's a conceptual example of how these steps might be integrated into the Easylibpal class:
```python
import pandas as pd
class Easylibpal:
def __init__(self, dataset):
self.dataset = dataset
# Load and preprocess the dataset
def clean_and_preprocess(self):
# Detect inconsistencies (example: check data types)
print(self.dataset.dtypes)
# Handle formatting errors (example: convert data types)
self.dataset['date_column'] = pd.to_datetime(self.dataset['date_column'])
# Handle missing values (example: drop rows with missing values)
self.dataset = self.dataset.dropna(subset=['required_column'])
# Handle duplicates (example: drop duplicates)
self.dataset = self.dataset.drop_duplicates()
# Handle inconsistent values (example: standardize values)
self.dataset['binary_column'] = self.dataset['binary_column'].map({'yes': 1, 'no': 0})
# Return the cleaned and preprocessed dataset
return self.dataset
# Usage
Easylibpal = Easylibpal(dataset=pd.read_csv('your_dataset.csv'))
cleaned_dataset = Easylibpal.clean_and_preprocess()
```
This example demonstrates a simplified approach to handling inconsistent or messy data within Easylibpal. The actual implementation would need to adapt these steps based on the specific characteristics and requirements of the dataset being processed.
Statistical Imputation
Statistical imputation involves replacing missing values with statistical estimates such as the mean, median, or mode of the available data. This method is straightforward and can be effective for numerical data. For categorical data, mode imputation is commonly used. The choice of imputation method depends on the distribution of the data and the nature of the missing values.
Model-Based Imputation
Model-based imputation uses machine learning models to predict missing values. This approach can be more sophisticated and potentially more accurate than statistical imputation, especially for complex datasets. Techniques like K-Nearest Neighbors (KNN) imputation can be used, where the missing values are replaced with the values of the K nearest neighbors in the feature space.
Using SimpleImputer in scikit-learn
The scikit-learn library provides the `SimpleImputer` class, which supports both statistical and model-based imputation. `SimpleImputer` can be used to replace missing values with the mean, median, or most frequent value (mode) of the column. It also supports more advanced imputation methods like KNN imputation.
To implement these imputation techniques in Python, Easylibpal might use the `SimpleImputer` class from scikit-learn. Here's an example of how to use `SimpleImputer` for statistical imputation:
```python
from sklearn.impute import SimpleImputer
import pandas as pd
# Load the dataset
dataset = pd.read_csv('your_dataset.csv')
# Initialize SimpleImputer for numerical columns
num_imputer = SimpleImputer(strategy='mean')
# Fit and transform the numerical columns
dataset'numerical_column1', 'numerical_column2' = num_imputer.fit_transform(dataset'numerical_column1', 'numerical_column2')
# Initialize SimpleImputer for categorical columns
cat_imputer = SimpleImputer(strategy='most_frequent')
# Fit and transform the categorical columns
dataset'categorical_column1', 'categorical_column2' = cat_imputer.fit_transform(dataset'categorical_column1', 'categorical_column2')
# The dataset now has missing values imputed
```
This example demonstrates how to use `SimpleImputer` to fill in missing values in both numerical and categorical columns of a dataset. The actual implementation would need to adapt these steps based on the specific characteristics and requirements of the dataset being processed.
Model-based imputation techniques, such as Multiple Imputation by Chained Equations (MICE), offer powerful ways to handle missing data by using statistical models to predict missing values. However, these techniques come with their own set of limitations and potential drawbacks:
1. Complexity and Computational Cost
Model-based imputation methods can be computationally intensive, especially for large datasets or complex models. This can lead to longer processing times and increased computational resources required for imputation.
2. Overfitting and Convergence Issues
These methods are prone to overfitting, where the imputation model captures noise in the data rather than the underlying pattern. Overfitting can lead to imputed values that are too closely aligned with the observed data, potentially introducing bias into the analysis. Additionally, convergence issues may arise, where the imputation process does not settle on a stable solution.
3. Assumptions About Missing Data
Model-based imputation techniques often assume that the data is missing at random (MAR), which means that the probability of a value being missing is not related to the values of other variables. However, this assumption may not hold true in all cases, leading to biased imputations if the data is missing not at random (MNAR).
4. Need for Suitable Regression Models
For each variable with missing values, a suitable regression model must be chosen. Selecting the wrong model can lead to inaccurate imputations. The choice of model depends on the nature of the data and the relationship between the variable with missing values and other variables.
5. Combining Imputed Datasets
After imputing missing values, there is a challenge in combining the multiple imputed datasets to produce a single, final dataset. This requires careful consideration of how to aggregate the imputed values and can introduce additional complexity and uncertainty into the analysis.
6. Lack of Transparency
The process of model-based imputation can be less transparent than simpler imputation methods, such as mean or median imputation. This can make it harder to justify the imputation process, especially in contexts where the reasons for missing data are important, such as in healthcare research.
Despite these limitations, model-based imputation techniques can be highly effective for handling missing data in datasets where a amusingness is MAR and where the relationships between variables are complex. Careful consideration of the assumptions, the choice of models, and the methods for combining imputed datasets are crucial to mitigate these drawbacks and ensure the validity of the imputation process.
USING EASYLIBPAL FOR AI ALGORITHM INTEGRATION OFFERS SEVERAL SIGNIFICANT BENEFITS, PARTICULARLY IN ENHANCING EVERYDAY LIFE AND REVOLUTIONIZING VARIOUS SECTORS. HERE'S A DETAILED LOOK AT THE ADVANTAGES:
1. Enhanced Communication: AI, through Easylibpal, can significantly improve communication by categorizing messages, prioritizing inboxes, and providing instant customer support through chatbots. This ensures that critical information is not missed and that customer queries are resolved promptly.
2. Creative Endeavors: Beyond mundane tasks, AI can also contribute to creative endeavors. For instance, photo editing applications can use AI algorithms to enhance images, suggesting edits that align with aesthetic preferences. Music composition tools can generate melodies based on user input, inspiring musicians and amateurs alike to explore new artistic horizons. These innovations empower individuals to express themselves creatively with AI as a collaborative partner.
3. Daily Life Enhancement: AI, integrated through Easylibpal, has the potential to enhance daily life exponentially. Smart homes equipped with AI-driven systems can adjust lighting, temperature, and security settings according to user preferences. Autonomous vehicles promise safer and more efficient commuting experiences. Predictive analytics can optimize supply chains, reducing waste and ensuring goods reach users when needed.
4. Paradigm Shift in Technology Interaction: The integration of AI into our daily lives is not just a trend; it's a paradigm shift that's redefining how we interact with technology. By streamlining routine tasks, personalizing experiences, revolutionizing healthcare, enhancing communication, and fueling creativity, AI is opening doors to a more convenient, efficient, and tailored existence.
5. Responsible Benefit Harnessing: As we embrace AI's transformational power, it's essential to approach its integration with a sense of responsibility, ensuring that its benefits are harnessed for the betterment of society as a whole. This approach aligns with the ethical considerations of using AI, emphasizing the importance of using AI in a way that benefits all stakeholders.
In summary, Easylibpal facilitates the integration and use of AI algorithms in a manner that is accessible and beneficial across various domains, from enhancing communication and creative endeavors to revolutionizing daily life and promoting a paradigm shift in technology interaction. This integration not only streamlines the application of AI but also ensures that its benefits are harnessed responsibly for the betterment of society.
USING EASYLIBPAL OVER TRADITIONAL AI LIBRARIES OFFERS SEVERAL BENEFITS, PARTICULARLY IN TERMS OF EASE OF USE, EFFICIENCY, AND THE ABILITY TO APPLY AI ALGORITHMS WITH MINIMAL CONFIGURATION. HERE ARE THE KEY ADVANTAGES:
- Simplified Integration: Easylibpal abstracts the complexity of traditional AI libraries, making it easier for users to integrate classic AI algorithms into their projects. This simplification reduces the learning curve and allows developers and data scientists to focus on their core tasks without getting bogged down by the intricacies of AI implementation.
- User-Friendly Interface: By providing a unified platform for various AI algorithms, Easylibpal offers a user-friendly interface that streamlines the process of selecting and applying algorithms. This interface is designed to be intuitive and accessible, enabling users to experiment with different algorithms with minimal effort.
- Enhanced Productivity: The ability to effortlessly instantiate algorithms, fit models with training data, and make predictions with minimal configuration significantly enhances productivity. This efficiency allows for rapid prototyping and deployment of AI solutions, enabling users to bring their ideas to life more quickly.
- Democratization of AI: Easylibpal democratizes access to classic AI algorithms, making them accessible to a wider range of users, including those with limited programming experience. This democratization empowers users to leverage AI in various domains, fostering innovation and creativity.
- Automation of Repetitive Tasks: By automating the process of applying AI algorithms, Easylibpal helps users save time on repetitive tasks, allowing them to focus on more complex and creative aspects of their projects. This automation is particularly beneficial for users who may not have extensive experience with AI but still wish to incorporate AI capabilities into their work.
- Personalized Learning and Discovery: Easylibpal can be used to enhance personalized learning experiences and discovery mechanisms, similar to the benefits seen in academic libraries. By analyzing user behaviors and preferences, Easylibpal can tailor recommendations and resource suggestions to individual needs, fostering a more engaging and relevant learning journey.
- Data Management and Analysis: Easylibpal aids in managing large datasets efficiently and deriving meaningful insights from data. This capability is crucial in today's data-driven world, where the ability to analyze and interpret large volumes of data can significantly impact research outcomes and decision-making processes.
In summary, Easylibpal offers a simplified, user-friendly approach to applying classic AI algorithms, enhancing productivity, democratizing access to AI, and automating repetitive tasks. These benefits make Easylibpal a valuable tool for developers, data scientists, and users looking to leverage AI in their projects without the complexities associated with traditional AI libraries.
2 notes
·
View notes
Text

Railway Recruitment Board (RRB)
Railway Assistant Loco Pilot (ALP) Recruitment 2025
Job search services
RRB Advt No. : CEN 01/2025 : Short Details of Notification
Important Dates
Application Begin : 12/04/2025
Last Date for Apply Online : 11/05/2025
Last Date Pay Exam Fee : 13/05/2025
Correction / Modified Form : 14-23 May 2025
Exam Date : As per Schedule
Admit Card Available : Notified Soon
Application Fee
General / OBC / EWS : 500/-
SC / ST / PH : 250/-
All Category Female : 250/-
After Appear the Stage I Exam
UR/OBC/EWS Fee Refund : 400/-
SC / ST / PH / Female Refund : 250/-
Pay the Examination Fee Through Debit Card, Credit Card, Net Banking Fee Mode Only.
Railway Assistant Loco Pilot Notification 2025: Age Limit as on 01/07/2025
Minimum Age : 18 Years
Maximum Age : 30 Years.
Age Relaxation Extra as per Railway Recruitment Board RRB ALP Recruitment Advt No. CEN 01/2025 Vacancy Rules.
RRB ALP Recruitment 2025 : Vacancy Details Total : 9970 Post
Post Name
Total Post
Railway RRB ALP Eligibility 2025
Assistant Loco Pilot
9970
Class 10th Matric with ITI from NCVT / SCVT Certificate in Fitter, Electrician, Instrument Mechanic, Millwright/Maintenance Mechanic, Mechanic (Radio & TV), Electronics Mechanic, Mechanic (Motor Vehicle), Wireman, Tractor Mechanic, Armature & Coil Winder, Mechanic (Diesel), Heat Engine, Turner, Machinist, Refrigeration & Air- Conditioning Mechanic. OR
Class 10th with Diploma in Mechanical / Electrical / Electronics / Automobile Engineering (OR) combination of various streams of these Engineering disciplines from a recognised Institution in lieu of ITI. OR
BE / B.Tech Degree in Mechanical / Electrical / Electronics / Automobile Engineering.
More Eligibility Details Read the Notification.
Railway RRB ALP 2025 Zone Wise Vacancy Details: Category Wise Vacancy Details
RRB Name
UR
EWS
OBC
SC
ST
Total
RRB Ahemdabad WR
223
33
130
74
37
497
RRB Ajmer NWR
162
49
133
262
73
679
RRB Ajmer WCR
109
14
14
4
0
141
RRB Bangalore SWR NA NA NA NA NA NA
RRB Bhopal WCR
23
0
11
12
0
46
RRB Bhopal WR
221
111
130
103
53
618
RRB Bhubaneswar ECOR
454
29
121
205
119
928
RRB Bilaspur CR
228
56
155
86
43
568
RRB Chandigarh NR
188
44
117
56
28
433
RRB Chennai SR
155
41
73
56
37
362
RRB Gorakhpur NER
32
7
21
12
28
100
RRB Guwahati NFR
13
3
8
4
2
30
RRB Jammu and Srinagar NR
4
0
0
3
1
8
RRB Kolkata ER
194
51
103
71
39
262
RRB Kolkata SER
95
48
61
39
19
458
RRB Malda ER
171
33
103
66
37
410
RRB Malda SER
10
2
6
4
2
24
RRB Mumbai SCR
9
2
6
3
2
22
RRB Mumbai CR
152
38
102
56
28
376
RRB Mumbai WR
138
34
93
51
26
342
RRB Muzaffarpur ECR
36
9
24
13
7
89
RRB Patna ECR
14
3
9
5
2
33
RRB Prayagraj NR
33
8
21
12
6
80
RRB Prayagraj NCR
218
58
110
72
50
508
RRB Ranchi ECR
234
58
156
87
43
578
RRB Ranchi SER
255
66
164
105
45
635
RRB Secunderabad SCR
435
110
216
136
70
967
RRB Secunderabad ECOR
216
53
144
80
40
533
RRB Siliuguri NFR
39
10
26
14
6
95
RRB Thiruvanathapuram SR
55
21
32
25
15
148
How to Fill Railway RRB ALP Assistant Loco Pilot Online Form 2025
Railway Recruitment Board RRB Online Released Assistant Loco Pilot ALP Recruitment Advt No. CEN 01/2025 Exam. Candidate Can Apply Between 12/04/2025 to 11/05/2025.
Candidate Read the Notification Before Apply the Recruitment Application Form in Railway Board ALP Exam 2025 CEN Advt No. 01/2025 Exam Online Form 2025.
Kindly Check and Collect the All Document - Eligibility, ID Proof, Address Details, Basic Details.
Kindly Ready Scan Document Related to Admission Entrance Form - Photo, Sign, ID Proof, Etc.
Before Submit the Application Form Must Check the Preview and All Column Carefully.
Take A Print Out of Final Submitted Form.
0 notes
Text
JEE Mains Answer Key 2025 out jeemain.nta.nic.in

Name of Post: JEE Mains Answer Key 2025 Out- Session 2 Response Sheet Download at jeemain.nta.nic.in Post Date: 12 April 2025 | 12:00 PM Exam Information: JEE Mains Answer Key 2025 Session 2 : नेशनल टेस्टिंग एजेंसी (NTA) ने अपनी आधिकारिक वेबसाइट jeemain.nta.nic.in पर JEE Main Answer Key 2025 Session 2 और Response Sheet जारी कर दी है। JEE Mains 2025 Response Sheet और Answer Key डाउनलोड लिंक अब उपलब्ध है। 2 अप्रैल से 9 अप्रैल, 2025 तक आयोजित JEE Main Session 2 परीक्षा 2025 में उपस्थित होने वा��े उम्मीदवार आधिकारिक वेबसाइट या अंत में दिए गए सीधे लिंक से अपने आवेदन संख्या और पासवर्ड का उपयोग करके अपने JEE Mains Session 2 Answer Key 2025 और Response Sheet PDF डाउनलोड कर सकते हैं। यदि आप किसी उत्तर से संतुष्ट नहीं हैं, तो आप चुनौती सुविधा का उपयोग करके मामूली शुल्क के लिए आधिकारिक उत्तर के खिलाफ आपत्ति भी उठा सकते हैं। JEE Main Answer Key 2025 Session 2 Joint Entrance Examination (JEE) Main Session II 2025 National Testing Agency (NTA) jeemain.nta.nic.inImportant DatesJEE Mains Application Start Date01 February 2025NTA JEE Application Last Date25 February 2025JEE Mains Session 2 Admit Card date29 March 2025Session 2 Exam date02 – 09 April 2025JEE Mains Session 2 Answer Key Release Date12 April 2025JEE Main Session 2 Result Date17 April 2025JEE Mains Response Sheet 2025 Session 2संयुक्त प्रवेश परीक्षा (जेईई मेन) सत्र 2 2025 देने वाले सभी उम्मीदवारों के लिए अच्छी खबर है, क्योंकि एनटीए ने आज, 12 अप्रैल, 2025 को जेईई मेन 2025 रिस्पॉन्स शीट और उत्तर कुंजी जारी कर दी है। परीक्षा 2 अप्रैल से 9 अप्रैल, 2025 तक सफलतापूर्वक आयोजित की गई थी। उम्मीदवार आधिकारिक वेबसाइट या सीधे लिंक से जेईई मेन उत्तर कुंजी 2025 लिंक का उपयोग करके अपनी उत्तर कुंजी और प्रतिक्रिया पत्रक पीडीएफ डाउनलोड कर सकते हैं।JEE Main Marking Scheme 2025JEE Main 2025 marking scheme प्रत्येक सही उत्तर के लिए निर्धारित अंक और गलत उत्तरों के लिए दंड निर्दिष्ट करती है। यहाँ सामान्य अंकन योजना का संक्षिप्त विवरण दिया गया है: ● सही उत्तर: +4 अंक ● गलत उत्तर: -1 अंक ● अनुत्तरित प्रश्न: 0 अंक उम्मीदवार अपनी प्रतिक्रिया पत्रक को उत्तर कुंजी से मिलान करके अपने स्कोर की गणना करने के लिए इस योजना का उपयोग कर सकते हैं। उदाहरण के लिए, यदि किसी उम्मीदवार ने 50 प्रश्नों के सही और 10 प्रश्नों के गलत उत्तर दिए हैं, तो वे प्रत्येक सही उत्तर के लिए 4 अंक जोड़कर और प्रत्येक गलत उत्तर के लिए 1 अंक घटाकर अपने कुल स्कोर की गणना कर सकते हैं।JEE Main Answer Key 2025 Session 2 : Short DetailsOrganization NameNational Testing Agency (NTA)Exam NameJoint Entrance Exam (JEE) Mains Session 2, 2025Course NameB.E./ B. Tech/ B. Arch/ B. PlanningJEE Mains Response Sheet 2025JEE Response Sheet PdfNTA JEE Main Official Websitejeemain.nta.nic.inHow to Check JEE Main 2025 Session 2 Answer Key/ Response Sheet● सबसे पहले, आधिकारिक वेबसाइट @jeemain.nta.nic.in पर जाएं या नीचे दिए गए डायरेक्ट लिंक पर जाएं। ● इसके बाद, JEE Mains 2025 सेशन 2 आंसर की/रिस्पॉन्स शीट लिंक पर क्लिक करें। ● अपना आवेदन नंबर, जन्म तिथि और दिए गए सिक्योरिटी पिन जैसे आवश्यक विवरण भरें, फिर सबमिट बटन पर क्लिक करें। ● लॉग इन करने के बाद, एक डैशबोर्ड खुलेगा जहाँ साइडबार में आंसर की का विकल्प दिखाई देगा; उस पर टैप करें। ● अब, आप दिए गए आंसर शीट से तुलना करने के लिए आंसर की और रिस्पॉन्स शीट पीडीएफ डाउनलोड कर सकते हैं। ● आप इसे भविष्य में उपयोग के लिए डाउनलोड, सेव या प्रिंट भी कर सकते हैं।◔ Bihar Board Class 10th Matric Exam Results ◔ Rajasthan Police Constable Recruitment 2025 for 9617 PostBihar DElEd Entrance Exam Admit Card 2025 DownloadDownload Session 2 Answer Key/ Response SheetClick HereDownload Session 2 Answer Key NoticeClick HereCheck JEE Mains Session 2 ResultClick HereDownload NTA JEE Session 2 Full SyllabusClick HereJEE Main Official WebsiteClick HereHome PageClick HereHow to Raise Objections Against the JEE Main 2025 Answer Keyयदि कोई उम्मीदवार jee mains answer key and response sheet 2025 में दिए गए उत्तरों से संतुष्ट नहीं है, तो एनटीए ने एक चुनौती विंडो खोली है जहाँ उम्मीदवार आपत्तियाँ दर्ज कर सकते हैं। उत्तर कुंजी को चुनौती देने की सुविधा 13 अप्रैल, 2025 तक उपलब्ध है। ● आधिकारिक वेबसाइट पर लॉग इन करें: आधिकारिक JEE मेन लॉगिन पोर्टल jeemain.nta.nic.in पर जाएँ और अपने आवेदन संख्या और पासवर्ड का उपयोग करके लॉग इन करें। ● उत्तर कुंजी चुनौती विकल्प खोजें: लॉग इन करने के बाद, आपको आपत्तियाँ उठाने का विकल्प दिखाई देगा। आगे बढ़ने के लिए इस विकल्प पर क्लिक करें। ● चुनौती देने के लिए प्रश्न(ओं) का चयन करें: उन प्रश्नों को चुनें जिन्हें आप चुनौती देना चाहते हैं। आपसे आपकी आपत्ति का कारण बताने और यदि संभव हो तो सहायक साक्ष्य प्रदान करने के लिए कहा जाएगा। ● आपत्ति शुल्क का भुगतान करें: उम्मीदवारों को प्रत्येक चुनौती प्रस्तुत करने के लिए शुल्क का भुगतान करना होगा। प्रति प्रश्न शुल्क पोर्टल पर उल्लिखित किया जाएगा। सुनिश्चित करें कि आप अपनी आपत्तियाँ प्रस्तुत करने के लिए भुगतान प्रक्रिया पूरी करें। ● आपत्ति प्रस्तुत करें: भुगतान संसाधित होने के बाद, अपनी आपत्ति प्रस्तुत करें। NTA प्रस्तुत चुनौतियों की समीक्षा करेगा और आपत्तियों का मूल्यांकन करने के बाद अंतिम उत्तर कुंजी जारी करेगा।

JEE Mains Answer Key Read the full article
#jeemain2024questionpaperandanswerkey#jeemainanswerkey#jeemainanswerkey2024#jeemainanswerkey2025#jeemainanswerkey202529janshift1#jeemainanswerkey2025bonusquestions#jeemainanswerkey2025howtocheck#jeemainanswerkey2025kaisecheckkare#jeemainanswerkey2025kaisedekhe#jeemainanswerkey2025link#jeemainanswerkey2025live#jeemainanswerkeyand#jeemainanswerkeyandresponsesheet#jeemainanswerkeychallenge#jeemainanswerkeydownloadkaisekaren#jeemainanswerkeyhowtocheck#jeemainanswerkeylink#jeemainanswerkeynotshowing#jeemainsanswerkeyandresponsesheet2025#jeemain.nta.nic.in#whyjeemainanswerkeyisnotshowing
1 note
·
View note
Text
A Level Is Equal to Which Class in Pakistan?
In Pakistan, many students and parents often ask, "A Level is equal to which class in Pakistan?"
or even more basically, "A Level means which class?" These are valid questions, especially for those considering switching from the local Matric/Intermediate system to the international Cambridge education route. Let’s break it all down in a simple way to understand what A Levels are, where they fit in the Pakistani system, and what options students have.
What is A Level in Pakistan?
A Level (short for Advanced Level) is an internationally recognized qualification offered under the British education system. In Pakistan, A Levels are administered mainly through Cambridge Assessment International Education (CAIE) and Pearson Edexcel. Students usually take A Levels after completing their O Levels, which is equivalent to Matriculation (Class 10) in Pakistan.
In simple terms, A Level means Class 11 and Class 12 in Pakistan. So if you're wondering "A Level is equal to which class in Pakistan?" — the answer is that it corresponds to the Intermediate level (FSc, FA, ICom, or ICS).
A Level vs Intermediate in Pakistan
Here’s how the two systems compare: British SystemPakistani SystemCommon TermsAge GroupO LevelMatric (Class 9–10)SSC – Secondary School Certificate14–16 yearsA LevelIntermediate (Class 11–12)HSSC – Higher Secondary School Certificate16–18 years
After completing A Levels, students in Pakistan are eligible to apply to universities just like Intermediate students. However, to do so, they must obtain an equivalence certificate from the IBCC (Inter Board Committee of Chairmen).
A Level Subjects in Pakistan
One of the major advantages of A Levels is the flexibility in subject selection. Students can tailor their subjects based on their interests and future career paths. Here are some common A Level subjects offered in Pakistan:
Sciences: Physics, Chemistry, Biology, Mathematics, Computer Science
Commerce & Business: Accounting, Economics, Business Studies
Humanities: Sociology, Psychology, Law, History, Literature
Creative Subjects: Art & Design, Media Studies
Most schools require students to choose at least 3 A Level subjects, though some take more depending on their goals (especially for competitive programs like medicine or engineering).
A Level Colleges in Karachi
If you're based in Karachi and looking for good A Level colleges, the city offers some of the best options in the country. Here are a few popular A Level institutions in Karachi:
The Lyceum
Foundation Public School
Bay View High School
Nixor College
Keynesian Institute of Management & Sciences
International Grammar School & College
Beaconhouse College Program (BCP)
The Lyceum
The Lyceum International
These colleges offer a wide range of subjects, experienced faculty, and resources to help students succeed in their A Level studies and beyond.
Why Choose A Levels in Pakistan?
Students and parents opt for A Levels for several reasons:
Internationally recognized – Ideal for those aiming to study abroad
Flexible subject choices – Unlike Intermediate, where streams are rigid
Focus on analytical and conceptual understanding
Better preparation for university-level education
However, it’s important to note that A Levels are generally more expensive than the local FSc or FA options. Also, getting equivalence can be a bit of a process and may sometimes put A Level students at a disadvantage in local university admissions (especially for medical and engineering programs).
Equivalence Certificate: A Must for Local University Admissions
To apply to a Pakistani university after A Levels, students must obtain an IBCC equivalence certificate. This certificate officially declares that the student's O and A Level results are equivalent to Matric and Intermediate.
O Level = Matric (SSC)
A Level = Intermediate (HSSC)
To get this equivalence, students must include Pakistan Studies, Islamiyat, and Urdu in their O Level subjects. If not taken, students may need to pass these as separate subjects to qualify.
Final Thoughts
To answer the original question once and for all:
A Level is equal to Class 11 and 12 in Pakistan, or the Intermediate level (FA, FSc, ICS, ICom).
Whether you're wondering “A Level means which class?”, “What is A Level in Pakistan?”, or looking up A Level subjects in Pakistan and A Level colleges in Karachi, the key is understanding how it fits into your academic journey.
Choosing between A Levels and Intermediate depends on your career goals, financial situation, and academic strengths. A Levels open many doors, especially for those targeting foreign universities or looking for a more concept-based learning approach.
If you're still unsure, consult with your school counselors or career advisors — making the right choice at this stage can shape your future path in a big way.
1 note
·
View note
Text
Listing Reference: 138818 Listing Status: Open Position Summary - Company: Nedbank - Industry: Banking & Financial Services - Job Category: Call Centre (Service) - Location: Sandton, Johannesburg, South Africa - Contract Type: Permanent - Remuneration: Market-Related Salary - EE Position: Yes - Closing Date: 18 April 2025 Introduction Nedbank, one of South Africa’s leading financial institutions, is offering a fantastic opportunity for individuals seeking to establish a career in the banking and financial services sector. The Service Centre Agent position is an excellent entry-level role within the bank’s call centre division, designed to provide world-class customer service to Nedbank clients. This role requires professionalism, efficiency, and a keen understanding of customer service principles. Job Description The Service Centre Agent is responsible for handling customer inquiries, resolving queries, and ensuring that every client interaction results in a positive banking experience. The role involves adherence to predefined service level agreements (SLAs) while maintaining Nedbank’s core values, including honesty, integrity, and accountability. Agents are expected to handle customer interactions efficiently, escalate unresolved queries, and provide continuous feedback to management to enhance customer service processes. Key Responsibilities: - Answer inbound calls efficiently and professionally, ensuring an excellent customer experience - Adhere to the call centre's daily schedule to meet performance targets - Escalate unresolved customer queries to management by logging cases into the system - Ensure that 90% of calls are answered within 60 seconds (90/60 SLA) - Promote and sell Nedbank’s products to customers, generating monthly lead targets - Prevent losses by ensuring proper logging and tracking of all customer interactions - Maintain compliance with banking regulations, governance, and risk management practices - Participate in Nedbank’s corporate social responsibility initiatives and culture-building activities - Stay up-to-date with industry changes, policies, and risk standards through training and development - Maintain effective knowledge sharing with the team to ensure consistency in customer service delivery - Provide timely and accurate information to customers, addressing their concerns effectively Ideal Candidate Nedbank is looking for individuals who are passionate about customer service and committed to delivering high-quality support. The ideal candidate should possess strong communication skills, a customer-centric approach, and the ability to work in a fast-paced environment. Minimum Requirements: - Education: - Matric / Grade 12 / National Senior Certificate (compulsory) - A Certificate in Call Centre Operations (preferred but not mandatory) - Experience: - 6 to 12 months of experience in a call centre environment - Experience within a banking or financial services environment is advantageous - Technical & Professional Knowledge: - Administrative procedures and systems - Regulatory knowledge relevant to the banking sector - Banking software and systems familiarity - Business writing skills - Customer service best practices - Governance, risk, and compliance procedures Role Responsibilities - Ensure calls are handled professionally while aligning with Nedbank’s service excellence policies - Maintain an in-depth understanding of the client's inquiry and provide accurate, clear, and concise responses - Provide ongoing feedback to management, identifying opportunities for process improvements - Uphold Nedbank’s reputation by demonstrating a high level of professionalism in every interaction - Stay updated with industry trends, regulatory changes, and compliance requirements - Work collaboratively with internal teams to ensure seamless service delivery - Enhance customer retention and satisfaction by addressing concerns efficiently and effectively Skills & Attributes To excel in this role, candidates should possess the following skills and attributes: - Customer Focus: Ability to engage with customers in a courteous and professional manner - Communication Skills: Strong verbal and written communication skills - Problem-Solving: Ability to think critically and resolve customer issues effectively - Attention to Detail: Ensure accuracy when logging calls and updating customer records - Adaptability: Ability to work in a fast-paced and target-driven environment - Teamwork: Ability to collaborate effectively with colleagues and stakeholders - Technical Proficiency: Familiarity with banking systems, software, and call centre applications - Resilience: Ability to manage high call volumes while maintaining service quality - Sales Ability: Capable of identifying customer needs and promoting suitable banking products Why Join Nedbank? Nedbank offers employees a dynamic work environment that fosters career growth and development. As part of the Sales and Services Job Family, Service Centre Agents gain hands-on experience in banking operations while contributing to customer satisfaction. Nedbank also offers: - Competitive remuneration and performance-based incentives - Opportunities for career advancement within the bank - Access to ongoing learning and development programs - A supportive work culture that values integrity, accountability, and teamwork - Participation in corporate social responsibility initiatives Application Process Interested candidates should apply by submitting their applications online via Nedbank’s official careers portal before the closing date: 18 April 2025. Application Checklist: - Updated CV with relevant experience and qualifications - Certified copies of Matric Certificate and additional qualifications (if applicable) - Contactable references from previous employers If you are passionate about delivering top-tier customer service and are eager to build a career in the banking industry, the Service Centre Agent position at Nedbank is an excellent opportunity. This role provides a solid foundation in banking operations, customer relations, and professional development. Take the first step toward a rewarding career—apply today! Click here to apply Read the full article
0 notes
Text
Bihar Board 10th Result 2025 to be out today: Steps to check BSEB matric result on Times of India
FILE – (AP Photo/Jenny Kane, File) The Bihar School Examination Board (BSEB) will declare the Class 10 Result 2025 today, March 29, at 12 PM. Students can check and download their results from secondary.biharboardonline.com. The exams were conducted from February 17 to 25, 2025, beginning with a language paper and concluding with vocational subjects, held in two shifts daily.This year, The Times…
0 notes
Text
CAREER COUNSELLING AND SUPPORT IN GURGAON NCR | 8181813977 | CAPABLE MANPOWER RECRUITMENT BY SHARPNER

Get personalized career guidance for 9th to 12th class students. Explore education and career pathways, make informed decisions, and seize future opportunities. Our career guidance & support service offers superior guidance for a successful career, based on thorough analysis of students' and parents' aspirations and capabilities, ensuring guaranteed success in career development. For students in grades 9 to 12, SHARPNERR provides advanced career guidance and support to ensure successful career development. The Dron Vs. Dron Test, designed by Dr. Dron Rathore, is a comprehensive assessment that helps students discover their ideal career path based on their strengths, interests, and aspirations.
CONTACT US : Gurgaon-122001, Haryana, India EMAIL US DIRECTLY : [email protected] CALL US DIRECTLY : 8181813977 Join SHARPNERR Today VISIT : https://sharpnerr.com/
Discover a new paradigm in talent acquisition with Talent Farmer. Unlike conventional job consultants, we specialize in cultivating organic talent tailored to your industry needs. We revolutionize talent acquisition by prioritizing organic talent development tailored to your industry needs. Our approach goes beyond traditional recruitment to ensure that candidates are not only a good fit for your organization but also possess the specific skills and competencies required for success in their roles. We meticulously map and finalize the functions and skills requirements of each position within your organization. This step ensures alignment between candidate capabilities and the demands of the role. We identify competency and capability matrices along with measurable parameters to assess candidate suitability accurately. This comprehensive approach allows us to evaluate candidates based on specific criteria relevant to your organization's needs.
0 notes
Text

Join Indian Navy (Nausena Bharti)
Indian Navy Entrance Test INET 2025
Job recruitment
Navy SSR & MR INET 2025: Short Details of Notification
Important Dates
Application Begin : 29/03/2025
Last Date for Apply Online : 10/04/2025 upto 05 PM
Pay Exam Fee Last Date : 10/04/2025
Stage I Exam INET 2025 : May 2025
Admit Card Available : Before Exam
Application Fee
General / OBC / EWS : 550/-
SC / ST :550/-
Pay the Exam Fee Through Debit Card / Credit Card / Net Banking Only.
Navy SSR / MR Agniveer Recruitment INET 2025 : Age Limit Details
Agniveer SSR / MR 02/2025 Batch Age Limit : 01/09/2004 to 29/02/2008
Agniveer SSR / MR 01/2026 Batch Age Limit : 01/02/2005 to 31/07/2008
Agniveer SSR / MR 02/2026 Batch Age Limit : 01/07/2005 to 31/12/2008
Navy Agniveer SSR & MR INET 2025 Exam : Vacancy Details
Post Name
Indian Navy Agniveer SSR & MR Eligibility
Agniveer SSR
10+2 Intermediate Exam with Mathematics, Physics with One of the Following Subject Chemistry / Biology / Computer Science.
Agniveer MR (Chef / Steward / Hygienist)
Class 10 (High School) Exam Passed in Any Recognized Board in India.
Indian Navy 10+2 SSR Agniveer & Matric MR Agniveer INET 2025 : Physical Eligibility Details
Navy Agniveer Height: 157 Cms for Male & 152 cms for Female
Navy Agniveer Running: 1.6 Km. Completed in 6:30 Minutes for Male & 8 Minutes for Female
Navy Agniveer Squat Ups (Uthak Baithak) : 20 Times for Male & 15 Minutes for Female
Navy Agniveer Push Ups: 15 Times for Male & 10 Times for Female
Navy Agniveer Bent Knee Sit-ups: 15 Times for Male & 10 Times for Female
How to Fill Navy Agniveer INET 2025 Batch Online Form 2025 (SSR/MR)
Candidate Live Photo Required During Application Form.
Indian Navy SSR / MR INET 01/2025 Batch Agniveer Recruitment 2025 Notification Released. Candidate Can Apply Between 29/03/2025 to 10/04/2025.
Candidate Read the Notification Before Apply the Recruitment Application Form in Nausena Bharti Agniveer Recruitment 2025.
Kindly Check and Collect the All Document - Eligibility, ID Proof, Address Details, Basic Details.
Kindly Ready Scan Document Related to Admission Entrance Form - Photo, Sign, ID Proof, Etc.
Before Submit the Application Form Must Check the Preview and All Column Carefully.
Take A Print Out of Final Submitted Form.
0 notes
Text
youtube
Class 12 Math | Matrices and Determinants part 2 | JEE Mains, CUET Prep
Matrices and Determinants are fundamental topics in Class 12 Mathematics, playing a crucial role in algebra and real-world applications. Mastering these concepts is essential for students preparing for competitive exams like JEE Mains, CUET, and other entrance tests. In this session, we continue exploring Matrices and Determinants (Part 2) with a deep dive into advanced concepts, problem-solving techniques, and shortcuts to enhance your mathematical skills.
What You’ll Learn in This Session?
In this second part of Matrices and Determinants, we cover: ✔ Types of Matrices & Their Properties – Understanding singular and non-singular matrices, symmetric and skew-symmetric matrices, and orthogonal matrices. ✔ Elementary Operations & Inverse of a Matrix – Step-by-step method to compute the inverse of a matrix using elementary transformations and properties of matrix operations. ✔ Adjoint and Cofactor of a Matrix – Learn how to find the adjoint and use it to compute the inverse efficiently. ✔ Determinants & Their Applications – Mastery of determinant properties and how they apply to solving equations. ✔ Solving Linear Equations using Matrices – Application of matrices in solving system of linear equations using Cramer’s Rule and Matrix Inversion Method. ✔ Shortcut Techniques & Tricks – Learn time-saving strategies to tackle complex determinant and matrix problems in exams.
Why is This Session Important?
Matrices and Determinants are not just theoretical concepts; they have wide applications in physics, computer science, economics, and engineering. Understanding their properties and operations simplifies problems in linear algebra, calculus, and probability. This topic is also heavily weighted in JEE Mains, CUET, and CBSE Board Exams, making it vital for scoring well.
Who Should Watch This?
JEE Mains Aspirants – Get an edge with advanced problem-solving strategies.
CUET & Other Competitive Exam Candidates – Build a strong foundation for entrance exams.
Class 12 CBSE & State Board Students – Strengthen concepts and improve exam performance.
Anyone Seeking Concept Clarity – If you want to master Matrices and Determinants, this session is perfect for you!
How Will This Help You?
Conceptual Clarity – Develop a clear understanding of matrices, their types, and operations.
Stronger Problem-Solving Skills – Learn various techniques and tricks to solve complex determinant problems quickly.
Exam-Focused Approach – Solve previous years' JEE Mains, CUET, and CBSE board-level questions.
Step-by-Step Explanations – Get detailed solutions to frequently asked questions in competitive exams.
Watch Now & Strengthen Your Math Skills!
Don't miss this in-depth session on Matrices and Determinants (Part 2). Strengthen your concepts, learn effective shortcuts, and boost your problem-solving skills to ace JEE Mains, CUET, and board exams.
📌 Watch here 👉 https://youtu.be/
🔔 Subscribe for More Updates! Stay tuned for more quick revision sessions, concept explanations, and exam tricks to excel in mathematics.
0 notes
Text
Bihar Board's Miscellaneous Rules, 1958
The Bihar Board’s Miscellaneous Rules, 1958, are a set of important guidelines that ensure the smooth operation of the Bihar School Examination Board (BSEB). These rules help conduct fair and transparent exams while maintaining high educational standards in Bihar. This article provides an easy-to-understand overview of these rules, their significance, and how they impact students and schools.
What Are the Bihar Board’s Miscellaneous Rules, 1958?
The Bihar Board established these rules in 1958 to organize school examinations and maintain proper procedures. The BSEB is responsible for conducting board exams in Bihar, ensuring that students receive fair and accurate results.
Key Features of the Bihar Board’s Miscellaneous Rules
These rules cover various aspects of how the Bihar Board operates. Here are the key points:
1. Examination Guidelines
The rules explain how board exams should be conducted for Class 10 (Matric) and Class 12 (Intermediate) students.
They define the process of preparing question papers, checking answer sheets, and announcing results.
The Bihar Board is responsible for deciding the exam schedule and ensuring smooth execution.
2. Preventing Malpractices in Exams
The rules ensure that exams are conducted in a fair and transparent manner without cheating.
Strict guidelines help prevent unfair practices and misconduct during exams.
Schools and exam centers must follow security measures to maintain exam integrity.
3. School Recognition and Affiliation
Schools must meet specific eligibility criteria to get recognition from the Bihar Board.
They must follow the approved syllabus and provide adequate facilities.
The Board conducts periodic inspections to ensure compliance with educational standards.
4. Appointment of Teachers and Examination Staff
The Board determines the qualifications for teachers, invigilators (exam supervisors), and examiners (paper checkers).
Responsibilities of exam staff are clearly defined to maintain fairness in the evaluation process.
Strict rules are followed to avoid bias in student assessments.
5. Grievance Redressal System
Students and schools can file complaints regarding exam results or administrative issues.
A structured system is in place to resolve disputes and re-evaluate complaints.
Students can appeal if they believe their exam results are incorrect or unfair.
6. Amendments and Rule Updates
The Bihar Board has the authority to modify or update rules as required.
Any updates are implemented after discussions with education experts.
Why Are the Bihar Board’s Miscellaneous Rules Important?
The Bihar Board’s Miscellaneous Rules, 1958, play a crucial role in ensuring a structured and fair examination system. These rules help:
Maintain the quality of education in Bihar.
Ensure that students receive proper guidance and opportunities.
Keep exam processes transparent and free from misconduct.
Standardize school affiliation requirements.
Improve accountability in the education sector.
Conclusion
The Bihar Board’s Miscellaneous Rules, 1958, have shaped Bihar’s education system by maintaining fairness, discipline, and transparency in board examinations. By following these guidelines, students, teachers, and schools can contribute to an improved and well-regulated education system in Bihar.
0 notes
Text
Investigating the Integrability-to-Chaos Transition in Differential Operators
1. Introduction
The transition between integrability and chaos in quantum systems has been extensively studied using random matrix theory and spectral analysis. A fundamental conjecture, known as the Hilbert-Pólya hypothesis, suggests that the non-trivial zeros of the Riemann zeta function can be interpreted as the eigenvalues of a self-adjoint operator.
This study explores how differential operators of the form:
$$H = -\frac{d^{12}}{dx^{12}} + V(x),$$
exhibit a transition between integrable behavior (Poisson statistics) and chaotic behavior (GUE statistics) based on the nature of the potential (V(x)). Furthermore, we investigate whether the eigenvalues of such an operator align with the statistical distribution of the non-trivial zeros of the Riemann zeta function.
2. Methodology
2.1 Justification for the 12th-Order Operator
The choice of the 12th-order derivative is motivated by several factors:
Symmetry and Structure: Higher-order derivatives introduce more complex spectral structures, potentially capturing transitions from integrability to chaos more effectively than lower-order derivatives.
Preliminary Numerical Results: Initial tests with second and fourth-order derivatives did not exhibit a clear transition between Poisson and GUE statistics.
Analogy with Other Systems: Many studies in quantum chaos use higher-order differential operators to induce stronger mixing properties in the spectrum, aligning with our goal of studying level repulsion.
Non-locality and Chaos Enhancement: High-order derivatives increase non-local interactions in the system, which can accelerate the onset of chaotic behavior.
2.2 Construction of the Differential Matrix
The operator (H) was discretized using a finite difference method of order 12, ensuring a faithful approximation of the higher-order derivative term (-d^{12}/dx^{12}). The resulting Hermitian matrix representation of (H) was used to compute the eigenvalues numerically.
To balance computational cost and accuracy, the matrix size (N) was varied from 100 to 1000.
2.3 Treatment of Boundary Conditions
To ensure numerical stability and prevent artificial reflections at the boundaries, we implemented different boundary conditions:
Periodic Boundary Conditions (PBC): Ensuring the function repeats itself smoothly at the edges.
Dirichlet Conditions: Setting ( \psi(x) = 0 ) at the boundaries to simulate confinement.
Neumann Conditions: Enforcing ( d\psi/dx = 0 ) at the edges to model smooth potentials.
The results were verified to be consistent across boundary condition choices, with minor variations.
2.4 Analysis of Convergence
To ensure that numerical results are not artifacts of finite matrix size, we tested convergence by varying (N) from 100 to 1000. The results are illustrated in Figure 1, which shows the stabilization of the normalized eigenvalue spacings as ( N ) increases.
Key Observations:
The distribution of spacings for ( N = 500 ) is already close to its asymptotic form, justifying its use in subsequent analyses.
There is a significant reduction in statistical noise for ( N \geq 300 ), indicating that matrices menores podem gerar artefatos numéricos.
If discrepancies persist between ( N = 500 ) and ( N = 1000 ), it would suggest the need for further extension.
2.5 Types of Tested Potentials
We considered different classes of polynomial potentials:
Even-degree potentials: (V(x) = x^n + x^2) for (n = 4, 6).
Odd-degree potentials: (V(x) = x^n + x^2) for (n = 3, 5, 7).
Mixed potentials incorporating oscillatory terms: (V(x) = x^2 + 0.3 x^4 + 0.5\sin(2x)).
Non-polynomial potentials: Including logarithmic (V(x) = \log(1 + x^2)) and exponential forms (V(x) = e^{-x^2}) to explore broader transition regimes.
The spacing between consecutive eigenvalues was analyzed and compared with Poisson and Wigner-Dyson (GUE) distributions.
2.6 Statistical Validation: KS-Test
To quantitatively compare the spectral properties of the differential operator with the zeros of the Riemann zeta function, we performed a Kolmogorov-Smirnov (KS) test:
For 100 zeros, the test resulted in ( p \approx 8.79 \times 10^{-59} ), indicating significant statistical differences.
Expanding the comparison to 1000 zeros, the results remained similar, suggesting that while the operator shares qualitative spectral properties with the zeta zeros, there are underlying differences in their distributions.
The distribution of spacings compared to theoretical statistics is shown in Figure 2:
2.7 Overlap Between Operator Eigenvalues and Zeta Zeros
We compare the eigenvalues of the operator for ( n = 5 ) with the first 1000 non-trivial zeros of the Riemann zeta function. The results are visualized in Figure 3:
3. Key Findings
Spectral analysis confirms the integrability-to-chaos transition.
Odd-degree potentials (e.g., (n = 5)) induce stronger chaos compared to even-degree potentials.
The eigenvalue spacings for (n = 5) closely resemble those of the Riemann zeta zeros but exhibit statistical deviations.
The empirical relation (\Delta E_n \sim n^{\alpha}) is better approximated by a logarithmic model rather than an exponential one, suggesting a smooth transition.
Alternative statistical tests (rigidity, number variance) further confirm the transition.
4. Conclusion and Future Work
Differential operators exhibit a predictable transition from integrability to chaos based on the potential's degree.
The fitted model suggests a universal scaling relation for spectral transitions.
Further statistical tests, including Anderson-Darling and large-sample KS-tests, could refine comparisons with the Riemann zeta zeros.
This work bridges quantum chaos and number theory, offering a computational toolkit for exploring spectral transitions in high-dimensional systems.
0 notes
Text
Implementing 2x2 Matrix Multiplication in Java: A Step-by-Step Guide
Matrix multiplication is a fundamental operation in linear algebra and is widely used in various applications such as graphics programming, machine learning, and scientific computing. In this post, I will walk you through implementing 2x2 matrix multiplication in Java, explaining the logic and providing a sample code snippet.
Understanding 2x2 Matrix Multiplication Matrix multiplication follows the row-by-column multiplication rule. Given two 2x2 matrices:
𝐴
[ 𝑎 11 𝑎 12 𝑎 21 𝑎 22 ] A=[ a 11
a 21
a 12
a 22
]
𝐵
[ 𝑏 11 𝑏 12 𝑏 21 𝑏 22 ] B=[ b 11
b 21
b 12
b 22
] The resulting matrix
𝐶
𝐴 × 𝐵 C=A×B is calculated as follows:
𝐶
[ ( 𝑎 11 × 𝑏 11 + 𝑎 12 × 𝑏 21 ) ( 𝑎 11 × 𝑏 12 + 𝑎 12 × 𝑏 22 ) ( 𝑎 21 × 𝑏 11 + 𝑎 22 × 𝑏 21 ) ( 𝑎 21 × 𝑏 12 + 𝑎 22 × 𝑏 22 ) ] C=[ (a 11 ×b 11 +a 12 ×b 21 ) (a 21 ×b 11 +a 22 ×b 21 )
(a 11 ×b 12 +a 12 ×b 22 ) (a 21 ×b 12 +a 22 ×b 22 ) ] Java Implementation Below is a Java program that performs 2x2 matrix multiplication:
java Copy Edit public class MatrixMultiplication { public static void main(String[] args) { // Define two 2x2 matrices int[][] A = { {1, 2}, {3, 4} }; int[][] B = { {5, 6}, {7, 8} }; // Call the multiply function int[][] result = multiplyMatrices(A, B); // Display the result System.out.println("Resultant Matrix:"); for (int i = 0; i < 2; i++) { for (int j = 0; j < 2; j++) { System.out.print(result[i][j] + " "); } System.out.println(); } } // Function to multiply two 2x2 matrices public static int[][] multiplyMatrices(int[][] A, int[][] B) { int[][] C = new int[2][2]; // Resultant 2x2 matrix // Applying matrix multiplication formula C[0][0] = A[0][0] * B[0][0] + A[0][1] * B[1][0]; C[0][1] = A[0][0] * B[0][1] + A[0][1] * B[1][1]; C[1][0] = A[1][0] * B[0][0] + A[1][1] * B[1][0]; C[1][1] = A[1][0] * B[0][1] + A[1][1] * B[1][1]; return C; }
} Explanation of the Code We define two 2x2 matrices (A and B) and initialize them with values. The multiplyMatrices() function computes the resultant 2x2 matrix C using the standard multiplication formula. The resultant matrix is then printed in a formatted way. Output of the Program yaml Copy Edit Resultant Matrix: 19 22 43 50
0 notes