#synthetic data
Explore tagged Tumblr posts
Text
The Rise of Synthetic Data in the Age of AI
Synthetic data is artificially generated data that is used to train machine learning models. It can be used to supplement or replace real-world data, and it has a number of advantages over real-world data.
2 notes
·
View notes
Text
🚨 Stop Believing the AI Hype, that’s the title of my latest conversation on the Localization Fireside Chat with none other than @Dr. Sidney Shapiro, Assistant Professor at the @Dillon School of Business, University of Lethbridge. We dive deep into what AI can actually do, and more importantly, what it can’t. From vibe coders and synthetic data to the real-world consequences of over-trusting black-box models, this episode is packed with insights for anyone navigating the fast-moving AI space. 🧠 Dr. Shapiro brings an academic lens and real-world practicality to an often-hyped conversation. If you're building, deploying, or just curious about AI, this is a must-read. 🎥 catch the full interview on YouTube: 👉 https://youtu.be/wsqN0964neM Would love your thoughts, are we putting too much faith in AI? #LocalizationFiresideChat #AIethics #DataScience #AIstrategy #GenerativeAI #MachineLearning #CanadianTech #HigherEd #Localization #TranslationTechnology #Podcast
#AI and Academia#AI Ethics#AI for Business#AI Hype#AI in Canada#AI Myths#AI Strategy#Artificial Intelligence#Canadian Podcast#Canadian Tech#chatgpt#Data Analytics#Data Science#Dr. Sidney Shapiro#Explainable AI#Future of AI#Generative AI#Localization Fireside Chat#Machine Learning#Robin Ayoub#Synthetic Data#Technology Trends
0 notes
Text

Cybersecurity In Banking In The UK: A Comprehensive Guide
The banking industry, owing to its unfettered access to financial wealth and personally identifiable information (PII) of customers, has always been an attractive target for cyber-attacks. The banking industry has seen a massive upsurge in digital transactions and there has been a corresponding and alarming rise in cybercriminals attacking the banking systems for vulnerabilities as well as duping consumers to reveal their credentials.
The banking industry, owing to its unfettered access to financial wealth and personally identifiable information (PII) of customers, has always been an attractive target for cyber-attacks. Since covid, the banking industry has seen a massive upsurge in digital transactions and there has been a corrAccording to the Verizon Data Breach Investigation Report (DBIR), in 2022, 23,896 security incidents ensued, of which 2,527 incidents and 690 confirmed data disclosures belonged to the financial and insurance industry alone. As the financial industry is becoming more digital and partnership-driven with the launch of new regulatory mandates such as open banking, and the adoption of new business models such as banking-as-a-service and embedded finance, the tables have turned from internal factors being the cause of data breaches to external ones, with 73% of the violations arising from external actors.
Shared banking systems, web apps, widespread use of machine learning algorithms, and API integrations with third-party providers have only added more vulnerabilities for data breach incidents and cyber-attacks. Not only would this put such highly critical information in the wrong hands, but it would also equally cause reputational damage to the bank itself, where it may even need to face regulatory penalties or legal costs. In September 2022, KeyBank publicly announced that an untold number of its mortgage customers had their information stolen. It was later slapped with a lawsuit, claiming that both the bank and a third-party service provider were negligent in monitoring and controlling potential IT security issues.
With incidents related to cybersecurity in banking becoming more instantaneous and sophisticated, we look into why cybersecurity has become a crucial agenda for financial institutions.
Cybersecurity in Banking: The Imperatives for Financial Institutions
Cyber incidents have a multi-faceted impact on a bank’s business. Just one cyber incident may result in a significant amount of financial loss and reputational loss, while also inviting regulatory enforcement actions and lawsuits. Even if cards are cancelled, and fraud is immediately taken care of, banks’ data can still be used to target market strategies or reveal information that can be used against the bank.
The bank itself may have to reimburse the ransom amount in some instances. On 28 May 2018, BMO and CIBC announced that hackers had breached their computer systems and stolen sensitive client information. Fraudulent transactions occurred following this data breach, and both BMO and CIBC reimbursed their clients for over $6.85M and $1.78M, respectively, of money stolen through these transactions.
Strict regulatory requirements only add further to the looming challenges for banks when it comes to cybersecurity practices, with many organisations at risk of inviting enforcement actions if they fail to adapt to the ever-changing laws.
How NayaOne may help you build a robust cyber resilience
NayaOne is a one-stop destination that helps improve cybersecurity in banking and promote cyber resilience by discovering and evaluating modern technology providers for their cybersecurity needs. Through one direct connection, banks have access to pre-vetted datasets and in-built governance rails that support the identification and review of cyber defences while ensuring compliance with cybersecurity guidelines.
Explore cybersecurity in banking, fintech marketplace and understand the challenges faced and measures taken by the leading financial institutions to build cyber resilience.
0 notes
Text
Synthetic Imaging Improves Patient Diagnoses
0 notes
Text

How fintech augmented by Gen AI can drive the future of financial services
Imagine a financial ecosystem where fraud is detected before it can occur, customer interactions are seamless and tailored, and managing finances is as intuitive as having a conversation. This vision is becoming a reality as generative AI reshapes the future of financial services.
Generative AI is already revolutionising industries. In financial services, innovation is being driven by automating processes, enhancing decision-making, and improving security measures. With 66% of organisations leveraging AI and machine learning technologies, the industry is witnessing its critical role in advancing operational efficiency and elevating the customer experience.
This technology extends beyond professional applications, resonating with users on multiple levels. While 75% of generative AI users rely on it to automate tasks and streamline workplace communications, many explore its potential for learning, creativity, and personal growth. These varied applications highlight its versatility and importance in both professional and personal contexts.
When generative AI meets fintech, the future of financial services reaches new heights. From strengthening anti-fraud mechanisms to delivering hyper-personalised experiences and streamlining financial operations, the combination of these technologies sets a new benchmark for the industry.
The fusion of generative AI and fintech is not merely a technological evolution—it is a leap toward a smarter, safer, and more adaptive future of financial services. This partnership is redefining how financial institutions operate, creating a more efficient and customer-centric financial landscape that will continue to shape the years ahead.
Understanding the rise of generative AI in the future of financial services
Generative AI rapidly transforms industries, offering unprecedented capabilities to solve complex problems, engage users, and streamline operations. In fintech, the potential is vast, as the sector is uniquely positioned to leverage these advancements to revolutionise how financial services are delivered.
What are the benefits of integrating generative AI in fintech?
The integration of generative AI into fintech is yielding significant advantages, empowering financial institutions to remain competitive in a fast-changing landscape while driving measurable business outcomes.
Tangible ROI and revenue growth
Generative AI is proving its value, with 74% of institutions already achieving positive ROI from at least one use case. Additionally, 30-35% expect returns within the next 12 months. Among companies actively using this technology, 86% report revenue growth, with many seeing a 6% or more annual increase in income.
Increased efficiency and cost savings
Generative AI enables financial institutions to automate complex tasks, streamline operations, and reduce human error. By optimising workflows—such as compliance reporting, document generation, and data analysis—organisations experience significant cost savings and improved efficiency, allowing them to allocate resources more strategically.
Enhanced customer trust
Transparency and innovation are at the core of generative AI’s impact. By delivering personalised services, improving fraud detection, and providing dynamic insights, financial institutions foster greater customer trust. This trust is further reinforced by the secure and transparent handling of sensitive data, a cornerstone of modern fintech services.
Competitive edge
In a market where technological advancements dictate success, generative AI provides a crucial competitive edge. Financial institutions that embrace this intelligent technology are better equipped to adapt to evolving customer needs, regulatory demands, and market conditions. The ability to innovate quickly ensures long-term relevance and leadership in the industry.
Integrating generative AI is not just a forward-looking strategy; it is a necessity for financial institutions aiming to enhance operational performance, improve customer relationships, and drive sustained growth in the future of financial services.
Experience the future of financial services with NayaOne
NayaOne is revolutionising the financial technology landscape. By providing seamless access to innovative tools and a rich ecosystem of financial services, NayaOne empowers organisations to accelerate their digital transformation.
Discover a new standard and the future of financial services, where collaboration meets innovation. Explore the possibilities.
#financial technology#digitalhackathon platform sandbox#fintech marketplace#synthetic data#esg marketplace
0 notes
Text

Synthetic Data for Respiratory Diseases: Real-World Accuracy Achieved
Ground-glass opacities and other lung lesions pose major challenges for AI diagnostic models, largely because even expert radiologists find them difficult to precisely annotate. This scarcity of data with high-quality annotations makes it tough for AI teams to train robust models on real-world data. RYVER.AI addresses this challenge by generating pre-annotated synthetic medical images on demand. Their previous studies confirmed that synthetically created images containing lung nodules meet real-world standards. Now, they’ve extended this success to respiratory infection cases. In a recent study, synthetic COVID-19 CT scans with ground-glass opacities were used to train a segmentation model, and the results were comparable to those from a model trained solely on real-world data - proving that synthetic images can replace or supplement real cases without compromising AI performance. That’s why Segmed and RYVER. AI is joining forces to develop a comprehensive foundation model for generating diverse medical images tailored to various needs. Which lesions give you the most trouble? Let us know in the comments, or reach out to discuss how synthetic data can help streamline your workflow.
#Medical AI#Synthetic Data#AI Training#Machine Learning#Deep Learning#AI Research#Health Care AI#MedTech#Radiology#Pharma
0 notes
Text

Guidelines for organising a fintech product hackathon in banks and financial institutions
To stay ahead in the competitive world of finance, banks and financial institutions must continuously innovate. A great way to drive this innovation is by hosting a product hackathon. These events create a space for diverse teams to come together, solve complex problems, and unlock new possibilities within the fintech space, encouraging fresh ideas and transformative solutions.
A product-based hackathon is more than just a coding competition; it’s an opportunity to foster collaboration, test new ideas, and develop products that could shape the future of banking. Let’s explore the essential steps involved in organising a successful fintech product-based hackathon within banks and financial institutions.
Define the purpose and goals of the product hackathon
Before you dive into the logistics of planning your hackathon, it’s important to define the purpose and goals of the event. What problem do you want to solve? What challenges are you hoping to overcome in your organisation? A well-defined objective will give participants direction and ensure the event stays focused.
The first step is to clearly identify the challenges or areas where you want innovation. Whether it’s improving customer experience, enhancing security, or developing new payment solutions, setting clear goals will help guide participants as they work towards solving the problem.
For example, if your bank is looking to improve its mobile banking app, the hackathon could be focused on developing new features or enhancing the user interface to create a more seamless experience. Alternatively, you may want to explore ways to improve fraud detection systems or streamline the loan application process.
Once you’ve identified your focus area, make sure to communicate the goals clearly to participants. This will ensure that everyone is on the same page and working toward a common objective.
Choose the right team and stakeholders
A successful hackathon isn’t just about the participants—it’s about having the right team and stakeholders involved. A product hackathon can be a complex event, and having a team that understands both the technical and business sides of things is crucial.
You’ll need to involve key departments such as technology, compliance, marketing, and customer service. Having a diverse set of voices in the planning stages will help you cover all the bases and ensure that the hackathon is well-rounded. This also helps when participants need guidance or input during the event itself.
You’ll also want to engage fintech experts, external mentors, and consultants who can offer industry insights and support throughout the hackathon. These experts can provide valuable feedback on the ideas being developed and help guide participants toward building solutions that are not only innovative but also feasible and scalable.
Finally, it’s important to involve decision-makers who can evaluate the ideas presented at the hackathon and make decisions about which projects to move forward with. This includes senior leaders from your bank’s innovation, product development, or technology teams.
Select a focus area or theme for the hackathon
One of the most important aspects of a product hackathon is selecting a focus area or theme. This helps narrow down the scope and ensures that participants are working on projects that align with your organisation’s goals and challenges.
Themes should be broad enough to encourage creativity but focused enough to ensure participants can develop meaningful solutions within the event’s timeframe. Some popular themes in fintech hackathons include:
Improving customer experience: Developing features that make banking services more accessible, user-friendly, or personalised.
Fraud prevention and security: Creating solutions to improve security and reduce fraudulent activity, which is an ongoing challenge in the financial industry.
Payments and money transfer: Exploring new ways to simplify or innovate payment methods and cross-border transfers.
Blockchain and cryptocurrency: Investigating how distributed ledger technology can be used to create transparent, secure financial products.
Financial inclusion: Developing solutions that help underserved populations access financial services.
You’ll want to ensure the theme you choose aligns with both your current needs and your vision for the future. It’s important that the theme resonates with participants, as their enthusiasm and interest in the topic will directly impact the success of the hackathon.
Organise logistics and infrastructure
Now that you’ve defined the purpose, team, and theme, it’s time to start organising the logistics. A product-based hackathon requires careful planning and the right infrastructure to support participants throughout the event.
First, decide whether the hackathon will be held in-person, virtually, or in a hybrid format. In-person hackathons offer the benefit of face-to-face collaboration and networking, while virtual events can attract a wider range of participants from different locations. A hybrid model, which combines both in-person and remote teams, is another option to consider.
Make sure you have the necessary technology and tools in place. Participants will need access to APIs, development environments, data sets, and other resources to bring their ideas to life. If your bank offers open banking APIs or has access to industry-specific data, make sure this is available to participants. You’ll also need to provide collaboration tools for teams, such as video conferencing platforms, chat apps, and project management software.
Additionally, you’ll need to arrange for mentors and judges who will be available to provide feedback and assess the final solutions. These experts should have deep knowledge of both the technical aspects of fintech and the regulatory considerations specific to banking.
Judging criteria and evaluation
The final product or solution developed during the hackathon is only as good as the judging criteria you establish. Clear, transparent criteria will ensure that the evaluation process is fair and consistent.
When deciding the judging criteria, consider the following factors:
Innovation: Is the solution novel? Does it bring something new to the table?
Feasibility: Is the solution technically feasible? Can it be implemented within the existing infrastructure?
Scalability: How well does the solution scale? Will it be effective across different markets or regions?
Customer impact: How will this solution improve the customer experience or solve a significant pain point?
You’ll want to select judges who are experts in fintech and banking but also who can assess solutions from a business perspective. In many cases, the best ideas might not be the most technically complex, but they may solve a critical problem or offer a high level of value to customers.
Be sure to provide constructive feedback to all participants, as this helps foster an environment of learning and continuous improvement. Hackathons are about more than just winning—they’re about experimentation and growth.
Post-hackathon: Next steps and implementation
The hackathon may be over, but the work doesn’t stop there. After the event, it’s important to assess the winning ideas and determine how they can be integrated into your organisation’s product development pipeline.
Start by identifying the projects with the most potential and begin working on a plan for further development. This could involve partnering with fintech startups or tech companies to help bring these solutions to life. Many successful products come out of hackathons and go on to become integral parts of an organisation’s offering.
It’s also a great time to follow up with participants, offering them mentorship or opportunities to continue developing their ideas. A hackathon is often just the beginning of a much larger journey of innovation and growth.
How NayaOne supports fintech product hackathons
Organising a product-based hackathon can be a powerful way for banks and financial institutions to drive fintech innovation, engage with new talent, and solve pressing industry challenges. But turning ideas into reality requires the right tools and infrastructure.
At NayaOne, we offer the tools, infrastructure, and support to help banks and financial institutions successfully host fintech product-based hackathons. Our platform enables organisations to easily access fintech APIs, data sets, and development tools, making it easier than ever to create innovative solutions.
Whether you’re hosting your hackathon platform or looking for ways to test and scale fintech solutions, NayaOne provides the resources to help you bring your ideas to life. Our platform empowers teams to build and prototype new fintech products in a secure, compliant environment—helping you foster innovation, streamline development, and ultimately create better products for your customers.
0 notes
Text
🚀 Dive into the future of data storytelling! Discover how AI and innovative tech are transforming the way we communicate insights and engage audiences. Explore the essential role of human creativity in this evolving landscape and learn how to leverage these tools for impactful narratives. Read more about it in our latest article! 🌐📊 #DataStorytelling #AI #Innovation #MarketingStrategy
#AI in Data Storytelling#Audience Engagement#Augmented Reality#Creative Data Narratives#Data Analytics#Data Interpretation#Data Storytelling#Data Visualization#Data-Driven Decisions#Digital Transformation#Ethical Data Use#Generative AI#Human Element in Storytelling#Innovation in Storytelling#marketing insights#Narrative Creation#Synthetic Data#Transmedia Storytelling#User-Generated Content#Virtual Reality
0 notes
Text

What are the benefits of digital transformation in the financial services industry?
Digital transformation is not just about adopting new technology—it is about reshaping how businesses operate, compete, and deliver value. Across industries, companies are investing heavily in digital solutions to streamline processes, enhance customer experiences, and drive growth. But in financial services, the stakes are even higher.
With shifting customer expectations, evolving regulations, and rapid advancements in AI, blockchain, and automation, financial institutions cannot afford to stand still. It is no surprise that 61% of business executives now rank digital transformation as a top priority, while 81% of business leaders see it as essential or necessary for long-term success.
For banks, insurers, and investment firms, digital transformation is more than a trend—it is the key to staying competitive in an increasingly digital-first world. But what are the benefits of digital transformation, and why is it a game-changer for financial institutions? The question is no longer if they should transform, but how quickly they can adapt to avoid being left behind.
Understanding digital transformation in financial services
For financial institutions, digital transformation is not just about keeping up—it is about staying ahead. It is the shift towards smarter, more efficient ways of working, using digital tools to improve customer experiences, streamline operations, and make better business decisions.
Right now, 74% of organisations see digital transformation as a top technology priority, and it is paying off—56% of executives say the return on investment has been better than expected. But what are the benefits of digital transformation in financial technology?
It means embracing technologies like cloud computing, AI, blockchain, and data analytics to automate processes, reduce risk, and offer more personalised services. Whether it is speeding up loan approvals, detecting fraud in real-time, or using AI to provide tailored financial advice, the industry is evolving fast. And for those who get it right, the benefits go far beyond just keeping up with the competition.
What are the benefits of digital transformation?
Digital transformation is not just about upgrading technology—it is about working smarter, faster, and more efficiently. Whether it is streamlining operations, enhancing security, or delivering a better customer experience, businesses that embrace digital tools are seeing real results. But what are the benefits of digital transformation beyond just keeping up with the competition?
From mobile banking apps that make life easier to AI-powered fraud detection that keeps finances secure, digital transformation is reshaping industries in ways that directly impact both businesses and customers. The right strategy does not just cut costs—it drives innovation, improves decision-making, and sets companies up for long-term success. If you are wondering what are the benefits of digital transformation for your business, it is time to look at the bigger picture.
Benefit #1: Improved customer experience
For many businesses, digital transformation is all about the customer. 35% of organisations say improving customer experience is their main goal when adopting digital tools. And in financial services, where convenience and speed matter more than ever, meeting these expectations is key to staying competitive.
Today’s customers expect seamless, instant, and personalised interactions—whether they are managing their accounts, applying for a loan, or making payments. That is where digital transformation makes a real difference. Mobile banking apps give users 24/7 access to their finances, AI-powered chatbots provide instant support without wait times, and real-time payment systems make transactions faster and more efficient than ever.
People are no longer willing to deal with long wait times, outdated systems, or one-size-fits-all services. Financial institutions that invest in better digital experiences are not just keeping up with demand—they are building stronger customer relationships and long-term loyalty.
Benefit #2: Increased operational efficiency
Efficiency is everything in financial services, and digital transformation is proving to be a game-changer. By leveraging automation, AI, and data-driven insights, organisations have been able to reduce operational inefficiency by 31%—freeing up time, cutting costs, and minimising errors.
Automation speeds up routine tasks like transaction processing, compliance checks, and fraud detection, while AI helps analyse vast amounts of data in real-time to improve decision-making. Instead of relying on manual processes prone to human error, financial institutions can streamline back-office operations, reduce paperwork, and lower operational costs.
For example, AI-powered systems can flag suspicious activity instantly, reducing fraud risks, while automated workflows ensure loan approvals and payments are processed faster with fewer delays. The result? A more agile, cost-effective business that can focus on innovation instead of inefficiencies.
Benefit #3: Enhanced security and compliance
Despite the push for digital transformation, only about one-third of organisations fully meet their objectives. One major challenge? Security and compliance. As financial services go digital, protecting customer data and meeting strict regulations have become top priorities.
Digital transformation is helping financial institutions strengthen their security measures through technologies like blockchain, encryption, and multi-factor authentication. Blockchain ensures transaction transparency and reduces fraud, while advanced encryption protects sensitive customer information from cyber threats. Multi-factor authentication adds an extra layer of security, making it harder for unauthorised users to gain access.
Beyond security, these technologies also help institutions stay compliant with evolving regulations. Automated compliance tools can monitor transactions in real time, flag suspicious activity, and generate audit-ready reports—reducing the risk of non-compliance and hefty fines. In a world where data breaches can be devastating, digital transformation is not just about innovation—it is about trust.
Benefit #4: Access to real-time data and insights
Data is the backbone of modern financial services, and institutions are doubling down on it. Over 75% of banks plan to invest more in data management and cloud services to support their generative AI strategies. Why? Because access to real-time data is transforming the way decisions are made.
With big data analytics, financial institutions can track market trends, assess risks instantly, and generate predictive insights for smarter financial planning. Whether it is real-time market analysis for investment strategies, AI-driven risk assessments to prevent fraud, or predictive analytics to personalise customer offerings, data is driving better, faster, and more informed decision-making.
By leveraging cloud-based solutions, banks and financial firms can break down data silos, improve efficiency, and deliver insights exactly when they are needed. Access to real-time information is not just an advantage—it is essential for staying ahead.
Benefit #5: Increased agility and innovation
Markets shift, customer expectations change, and new technologies emerge all the time. 34% of organisations see Agile adoption as key to keeping up, and digital transformation makes that possible. Financial institutions that invest in digital tools can respond faster, experiment with new ideas, and bring innovations to market without the usual bottlenecks.
This agility has already led to the rise of new financial products, digital wallets, and AI-powered robo-advisors that offer personalised investment guidance. Cloud-based systems and automation help banks and financial firms test, refine, and scale new solutions quickly, without being held back by outdated infrastructure.
By embracing digital transformation, financial institutions can launch better products, improve customer experiences, and stay ahead of industry shifts. The ability to adapt is not just useful—it is what sets leaders apart.
Benefit #6: Cost savings and scalability
Digital transformation is a major investment, but the long-term savings and scalability make it worth it. 89% of large companies worldwide are implementing digital and AI transformation, yet they have only captured 31% of the expected revenue lift and 25% of expected cost savings so far. The potential for greater efficiency and cost reduction is still huge.
By adopting digital tools, financial institutions can cut costs on physical infrastructure, manual labour, and time-intensive processes. For example, cloud computing eliminates the need for expensive on-site servers, reducing maintenance costs while improving flexibility. Automation streamlines operations, minimising human error and freeing up employees to focus on higher-value tasks.
Beyond cost savings, digital transformation also supports scalability. As financial institutions grow, cloud-based platforms and AI-driven processes make it easier to expand services, handle larger transaction volumes, and adapt to market demands without massive upfront costs. The result? A leaner, more efficient operation that can scale without overspending.
Experience the benefits of digital transformation with NayaOne
Navigating the digital shift can be complex, but the right strategy makes all the difference. NayaOne helps businesses unlock the full potential of digital transformation consulting, providing the tools and expertise needed to streamline operations, enhance security, and drive innovation.
What are the benefits of digital transformation? Faster workflows, smarter decision-making, and a seamless customer experience. Whether you are looking to adopt AI, optimise processes, or scale efficiently, NayaOne makes transformation simple.
1 note
·
View note
Text
So, you want to build AI models that can detect enemy equipment, but you don’t exactly have a military budget lying around? What’s the game plan? Here’s a guide to building highly accurate AI detection models for enemy hardware - without breaking the bank.
By using synthetic data from 3D models, you can train powerful object detection models like YOLOv5 to recognize even the most elusive assets on the cheap.
When gathering real-world images is tough or expensive, synthetic data from 3D models becomes a game-changer for training object detection models like YOLOv5. Known for its speed and accuracy, YOLOv5 processes images in a single pass, making it ideal for real-time applications like video analysis and autonomous systems.
Using 3D models, synthetic images of objects - such as Russian, Chinese, and North Korean T-90 tanks (for example) - can be generated from every angle, under different conditions, and in varied environments. This flexibility lets YOLOv5 learn robust features and generalize well, even for highly specific detection needs.
Ultimately, synthetic data from 3D models offers an efficient, cost-effective way to build accurate, custom detection models where real-world data collection is limited or impractical.
Steps to Building Detection Models Using Synthetic 3D Images
1. Generating Synthetic Images from 3D Models
● Use a 3D graphics tools like Blender or Unity, to render images of the tank model from every conceivable angle. These tools allow control over orientation, distance, lighting, and environment.
● Render the tank against various backgrounds to simulate different settings, or start with plain backgrounds for initial training, adding realistic ones later to improve robustness.
● Add variety by changing:
■ Lighting conditions (daylight, overcast, night)
■ Viewing angles (front, back, top-down, low angle)
■ Distances and zoom levels to simulate visibility and scale variations.
2. Applying Realistic Textures and Weather Effects
● Apply textures (like camouflage) and surface details (scratches, wear) to enhance realism.
● Simulate weather effects—rain, snow, or fog—to make the images resemble real-world conditions, helping the model generalize better.
3. Generating Annotation Labels for Synthetic Images
● Use the 3D rendering software to generate bounding box annotations along with the images. Many tools can output annotation data directly in YOLO format or in formats that are easily converted.
4. Using Domain Randomization for Improved Generalization
● Domain randomization involves varying elements like background, lighting, and texture randomly in each image to force the model to generalize.
● Change the background or adjusting color schemes helps the model perform better when exposed to real-world images, focusing on the shape and structure rather than specific synthetic features.
5. Fine-Tuning with Real Images (recommended)
● While synthetic images provide a strong foundation, adding even a small number of real images during training can boost accuracy and robustness. Real images add natural variations in lighting, texture, and environment that are hard to replicate fully.
● For optimal fine-tuning, aim for 50–100 real images per tank type if available.
YOLOv5 URL: https://github.com/ultralytics/yolov5
Oh, by the way... the video is just one of 365 different rotations for this background and lighting.
0 notes
Text
The Age of the Digital Precog: How AI Predicts Our Every Move
Just like the precogs could glimpse future events, AI sifts through enormous amounts of data to make informed guesses about what we’ll do next.
Whats On My Mind Today? Who Are You? How to passively gather personal information is a fascinating look into how businesses, marketers, and tech giants employ techniques to connect your protected data to the information you freely share. Passive information gathering means collecting data about a person without actively seeking it or requiring direct interaction. This can happen through…
#AI data gathering#ChatGPT#data transparency#digital privacy#digital surveillance#IoT privacy#MidJourney#passive data collection#personal privacy#predictive AI#public records#social media analysis#synthetic data
0 notes
Text
0 notes
Text
Synthetic Data Unlocks Data-Driven Innovation Without Privacy Concerns
Diverse datasets are crucial for data driven-activities activities like testing, training, and development. However, accessing real-world data often comes with hurdles – privacy concerns, data availability limitations, and regulatory restrictions can all stall progress. This is where synthetic data generation emerges as a game-changer. What is Synthetic Data?Where Does Synthetic Data…
0 notes
Text
Synthetic Data: Description, Benefits and Implementation
The quality and volume of data are critical to the success of AI algorithms. Real-world data collection is expensive and time-consuming. Furthermore, due to privacy regulations, real-world data cannot be used for research or training in most situations, such as healthcare and the financial sector. Another disadvantage is the data’s lack of availability and sensitivity. To power deep learning and artificial intelligence algorithms, we need massive data sets.
Synthetic data, a new area of artificial intelligence, relieves you of the burdens of manual data acquisition, annotation, and cleaning. Synthetic data generation solves the problem of acquiring data that would otherwise be impossible to obtain. Synthetic data generation will produce the same results as real-world data in a fraction of the time and with no loss of privacy.
Visual simulations and recreations of real-world environments are the focus of synthetic data generation. It is photorealistic, scalable, and powerful data that was created for training using cutting-edge computer graphics and data generation algorithms. It is highly variable, unbiased, and annotated with absolute accuracy and ground truth, removing the bottlenecks associated with manual data collection and annotation.
Why is synthetic data required?
Businesses can benefit from synthetic data for three reasons: privacy concerns, faster product testing turnaround, and training machine learning algorithms.
Most data privacy laws limit how businesses handle sensitive data. Any leakage or sharing of personally identifiable customer information can result in costly lawsuits that harm the brand’s reputation. As a result, one of the primary reasons why companies invest in synthetic data and synthetic data generation techniques is to reduce privacy concerns.
Any previous data remains unavailable for completely new products. Furthermore, human-annotated data is an expensive and time-consuming process. This can be avoided if businesses invest in synthetic data, which can be generated quickly and used to develop reliable machine learning models.
What is the creation of synthetic data?
Synthetic data generation is the process of creating new data as a replacement for real-world data, either manually using tools like Excel or automatically using computer simulations or algorithms. If the real data is unavailable, the fake data can be generated from an existing data set or created entirely from scratch. The newly generated data is nearly identical to the original data.
Synthetic data can be generated in any size, at any time, and in any location. Despite being artificial, synthetic data mathematically or statistically reflects real-world data. It is similar to real data, which is collected from actual objects, events, or people in order to train an AI model.
Real data vs. synthetic data
Real data is measured or collected in the real world. Such information is generated every time a person uses a smartphone, laptop, or computer, wears a smartwatch, accesses a website, or conducts an online transaction. Furthermore, surveys can be used to generate real data (online and offline).
In digital contexts, synthetic data is produced. With the exception of the portion that was not derived from any real-world occurrences, synthetic data is created in a way that successfully mimics the actual data in terms of fundamental qualities. The idea of using synthetic data as a substitute for actual data is very promising because it may be used to provide the training data that machine learning models require. But it’s not certain that artificial intelligence can solve every issue that arises in the real world. The substantial benefits that synthetic data has to provide are unaffected by this.
Where can you use synthetic data?
Synthetic data has a wide range of applications. When it comes to machine learning, adequate, high-quality data is still required. Access to real data may be restricted due to privacy concerns at times, while there may not be enough data to train the machine learning model satisfactorily at others. Synthetic data is sometimes generated to supplement existing data and aid in the improvement of the machine learning model.
Many sectors can benefit greatly from synthetic data:
1. Banking and financial services
2. Healthcare and pharmaceuticals
3. Internet advertising and digital marketing
4. Intelligence and security firms
5. Robotics
6. Automotive and manufacturing
Benefits of synthetic data
Synthetic data promises to provide the following benefits:
Customizable:
To meet the specific needs of a business, synthetic data can be created.
Cost-effective:
In comparison to genuine data, synthetic data is a more affordable solution. Imagine a producer of automobiles that needs access to crash data for vehicle simulations. In this situation, acquiring real data will cost more than producing fake data.
Quicker to produce:
It is possible to produce and assemble a dataset considerably more quickly with the right software and hardware because synthetic data is not gathered from actual events. This translates to the ability to quickly make a large amount of fabricated data available.
Maintains data privacy:
The ideal synthetic data does not contain any information that may be used to identify the genuine data; it simply closely mimics the real data. This characteristic makes the synthetic data anonymous and suitable for dissemination. Pharmaceutical and healthcare businesses may benefit from this.
Some real-world applications of synthetic data
Here are some real-world examples where synthetic data is being actively used.
Healthcare:
In situations where actual data is lacking, healthcare institutions are modeling and developing a variety of tests using synthetic data. Artificial intelligence (AI) models are being trained in the area of medical imaging while always maintaining patient privacy. In order to forecast and predict disease patterns, they are also using synthetic data.
Agriculture:
In computer vision applications that help with crop production forecasting, crop disease diagnosis, seed/fruit/flower recognition, plant growth models, and more, synthetic data is useful.
Banking and Finance:
As data scientists create and develop more successful fraud detection algorithms employing synthetic data, banks and financial institutions will be better able to detect and prevent online fraud.
Ecommerce:
Through advanced machine learning models trained on synthetic data, businesses gain the benefits of efficient warehousing and inventory management, as well as an improved customer online purchase experiences.
Manufacturing:
Companies are benefiting from synthetic data for predictive maintenance and quality control.
Disaster prediction and risk management:
Government agencies are using synthetic data to predict natural disasters in order to prevent disasters and lower risks.
Automotive & Robotics:
Synthetic data is used by businesses to simulate and train self-driving cars, autonomous vehicles, drones, and robots.
Synthetic Data Generation by TagX
TagX focuses on accelerating the AI development process by generating data synthetically to fulfill every data requirement uniquely. TagX has the ability to provide synthetically generated data that are pixel-perfect, automatically annotated or labeled, and ready to be used as ground truth as well as train data for instant segmentation.
Final Thoughts
In some cases, synthetic data may be used to address a company’s or organization’s lack of relevant data or data scarcity. We also investigated the methods for creating artificial data and the potential users. Along with a few examples from actual fields where synthetic data is used, we discussed some of the challenges associated with working with it.
When making business decisions, the use of actual data is always preferable. When such true raw data is unavailable for analysis, realistic data is the next best option. However, it should be noted that in order to generate synthetic data, data scientists with a solid understanding of data modeling are required. A thorough understanding of the actual data and its surroundings is also required. This is necessary to ensure that, if available, the generated data is as accurate as possible.
0 notes
Text
e438 — We will always have Paris
VR, Quest 3, photorealism, artists using Nightshade for data poisoning to protect their works, code hemophilia from recursive training on synthetic data, Dutchification and much more!
Photo by Rodrigo Kugnharski on Unsplash Published 30 October 2023 Michael, Michael and Andy get together for a lively discussion on VR, AI, another virtual museum and end on a high note with “a touch of Dutch” applied via generative AI. Starting off the episode with VR, the co-hosts explore a couple of articles dealing with the new Quest 3 headset and ways of working with it. The TechCrunch…
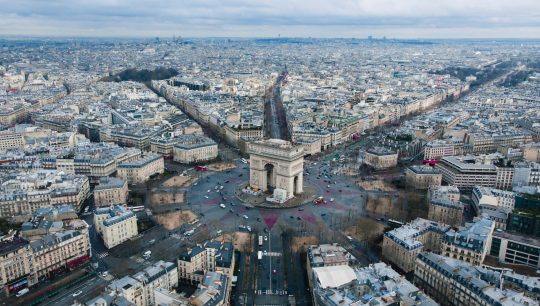
View On WordPress
#ai#code hemophilia#data poisoning#Dutchification#nightshade#Paris#photorealism#quest 3#recursive training#synthetic data#uncanny valley#vr
0 notes