#web scraping using google colab
Explore tagged Tumblr posts
Text
Top Custom Web App Development Company Near You
Zyneto Technologies is a trusted web app development company, providing best and custom web development services that specifically fulfill your business goals. Whichever website developers near me means to you or global partners you’ll gain access to a team of scalable, responsive, and feature rich web development solutions. We design intuitive user interfaces, build powerful web applications that perform seamlessly, providing awesome user experiences. Our expertise in modern technologies and framework enables us to design, develop and customize websites /apps that best fit your brand persona and objectives. The bespoke solution lines up to whether it is a startup or enterprise level project, the Zyneto Technologies delivers robust and innovative solution that will enable your business grow and succeed.
Zyneto Technologies: A Leading Custom Web Development and Web App Development Company
In the digital age, having a well-designed, high-performing website or web application is crucial to a business’s success. Zyneto Technologies stands out as a trusted web app development company, providing top-tier custom web development services tailored to meet the specific goals of your business. Whether you’re searching for “website developers near me” or partnering with global experts, Zyneto offers scalable, responsive, and feature-rich solutions that are designed to help your business grow.
Why Zyneto Technologies is the Top Custom Web Development Company Near You
Zyneto Technologies is a highly regarded name in the world of web development, with a reputation for delivering custom web solutions that perfectly align with your business objectives. Whether you're a startup looking for a personalized web solution or an established enterprise aiming for a digital overhaul, Zyneto offers custom web development services that deliver lasting value. With a focus on modern web technologies and frameworks, their development team crafts innovative and robust web applications and websites that drive business growth.
Expert Web App Development Services to Match Your Business Needs
As one of the leading web app development companies, Zyneto specializes in creating web applications that perform seamlessly across platforms. Their expert team of developers is proficient in designing intuitive user interfaces and building powerful web applications that provide a smooth and engaging user experience. Whether you require a custom website or a sophisticated web app, Zyneto’s expertise ensures that your digital solutions are scalable, responsive, and optimized for the best performance.
Tailored Custom Web Development Solutions for Your Brand
Zyneto Technologies understands that every business is unique, which is why they offer custom web development solutions that align with your brand’s persona and objectives. Their team works closely with clients to understand their vision and create bespoke solutions that fit perfectly within their business model. Whether you're developing a new website or upgrading an existing one, Zyneto delivers web applications and websites that are designed to reflect your brand’s identity while driving engagement and conversions.
Comprehensive Web Development Services for Startups and Enterprises
Zyneto Technologies offers web development solutions that cater to both startups and large enterprises. Their custom approach ensures that every project, regardless of scale, receives the attention it deserves. By leveraging modern technologies, frameworks, and best practices in web development, Zyneto delivers solutions that are not only technically advanced but also tailored to meet the specific needs of your business. Whether you’re building a simple website or a complex web app, their team ensures your project is executed efficiently and effectively.
Why Zyneto Technologies is Your Ideal Web Development Partner
When searching for "website developers near me" or a top custom web app development company, Zyneto Technologies is the ideal choice. Their combination of global expertise, cutting-edge technology, and focus on user experience ensures that every solution they deliver is designed to meet your business goals. Whether you need a custom website, web application, or enterprise-level solution, Zyneto offers the expertise and dedication to bring your digital vision to life.
Elevate Your Business with Zyneto’s Custom Web Development Services
Partnering with Zyneto Technologies means choosing a web development company that is committed to providing high-quality, customized solutions. From start to finish, Zyneto focuses on delivering robust and innovative web applications and websites that support your business objectives. Their team ensures seamless project execution, from initial design to final deployment, making them a trusted partner for businesses of all sizes.
Get Started with Zyneto Technologies Today
Ready to take your business to the next level with custom web development? Zyneto Technologies is here to help. Whether you are in need of website developers near you or a comprehensive web app development company, their team offers scalable, responsive, and user-friendly solutions that are built to last. Connect with Zyneto Technologies today and discover how their web development expertise can help your business grow and succeed.
visit - https://zyneto.com/
#devops automation tools#devops services and solutions#devops solutions and services#devops solution providers#devops solutions company#devops solutions and service provider company#devops services#devops development services#devops consulting service#devops services company#web scraping solutions#web scraping chrome extension free#web scraping using google colab#selenium web scraping#best web scraping tools#node js web scraping#artificial intelligence web scraping#beautiful soup web scraping#best web scraping software#node js for web scraping#web scraping software#web scraping ai#free web scraping tools#web scraping python beautifulsoup#selenium web scraping python#web scraping with selenium and python#web site development#website design company near me#website design companies near me#website developers near me
0 notes
Text
year in review - hockey rpf on ao3
hello!! the annual ao3 year in review had some friends and i thinking - wouldn't it be cool if we had a hockey rpf specific version of that. so i went ahead and collated the data below!!
i start with a broad overview, then dive deeper into the 3 most popular ships this year (with one bonus!)
if any images appear blurry, click on them to expand and they should become clear!
₊˚⊹♡ . ݁₊ ⊹ . ݁˖ . ݁𐙚 ‧₊˚ ⋅. ݁
before we jump in, some key things to highlight: - CREDIT TO: the webscraping part of my code heavily utilized the ao3 wrapped google colab code, as lovingly created by @kyucultures on twitter, as the main skeleton. i tweaked a couple of things but having it as a reference saved me a LOT of time and effort as a first time web scraper!!! thank you stranger <3 - please do NOT, under ANY circumstances, share any part of this collation on any other website. please do not screenshot or repost to twitter, tiktok, or any other public social platform. thank u!!! T_T - but do feel free to send requests to my inbox! if you want more info on a specific ship, tag, or you have a cool idea or wanna see a correlation between two variables, reach out and i should be able to take a look. if you want to take a deeper dive into a specific trope not mentioned here/chapter count/word counts/fic tags/ship tags/ratings/etc, shoot me an ask!
˚ . ˚ . . ✦ ˚ . ★⋆. ࿐࿔
with that all said and done... let's dive into hockey_rpf_2024_wrapped_insanity.ipynb
BIG PICTURE OVERVIEW
i scraped a total of 4266 fanfics that dated themselves as published or finished in the year 2024. of these 4000 odd fanfics, the most popular ships were:
Note: "Minor or Background Relationship(s)" clocked in at #9 with 91 fics, but I removed it as it was always a secondary tag and added no information to the chart. I did not discern between primary ship and secondary ship(s) either!
breaking down the 5 most popular ships over the course of the year, we see:
super interesting to see that HUGE jump for mattdrai in june/july for the stanley cup final. the general lull in the offseason is cool to see as well.
as for the most popular tags in all 2024 hockey rpf fic...
weee like our fluff. and our established relationships. and a little H/C never hurt no one.
i got curious here about which AUs were the most popular, so i filtered down for that. note that i only regex'd for tags that specifically start with "Alternate Universe - ", so A/B/O and some other stuff won't appear here!
idk it was cool to me.
also, here's a quick breakdown of the ratings % for works this year:
and as for the word counts, i pulled up a box plot of the top 20 most popular ships to see how the fic length distribution differed amongst ships:
mattdrai-ers you have some DEDICATION omg. respect
now for the ship by ship break down!!
₊ . ݁ ݁ . ⊹ ࣪ ˖͙͘͡★ ⊹ .
#1 MATTDRAI
most popular ship this year. peaked in june/july with the scf. so what do u people like to write about?
fun fun fun. i love that the scf is tagged there like yes actually she is also a main character
₊ . ݁ ݁ . ⊹ ࣪ ˖͙͘͡★ ⊹ .
#2 SIDGENO
(my babies) top tags for this ship are:
folks, we are a/b/o fiends and we cannot lie. thank you to all the selfless authors for feeding us good a/b/o fic this year. i hope to join your ranks soon.
(also: MPREG. omega sidney crosby. alpha geno. listen, the people have spoken, and like, i am listening.)
₊ . ݁ ݁ . ⊹ ࣪ ˖͙͘͡★ ⊹ .
#3 NICOJACK
top tags!!
it seems nice and cozy over there... room for one more?
₊ . ݁ ݁ . ⊹ ࣪ ˖͙͘͡★ ⊹ .
BONUS: JDTZ.
i wasnt gonna plot this but @marcandreyuri asked me if i could take a look and the results are so compelling i must include it. are yall ok. do u need a hug
top tags being h/c, angst, angst, TRADES, pining, open endings... T_T katie said its a "torture vortex" and i must concurr
₊ . ݁ ݁ . ⊹ ࣪ ˖͙͘͡★ ⊹ .
BONUS BONUS: ALPHA/BETA/OMEGA
as an a/b/o enthusiast myself i got curious as to what the most popular ships were within that tag. if you want me to take a look about this for any other tag lmk, but for a/b/o, as expected, SID GENO ON TOP BABY!:
thats all for now!!! if you have anything else you are interested in seeing the data for, send me an ask and i'll see if i can get it to ya!
#fanfic#sidgeno#evgeni malkin#hockey rpf#sidney crosby/evgeni malkin#hockeyrpf#hrpf fic#sidgeno fic#sidney crosby#hockeyrpf wrapped 2024#leon draisaitl#matthew tkachuk#mattdrai#leon draisaitl/matthew tkachuk#nicojack#nico hischier#nico hischier/jack hughes#jack hughes#jamie drysdale#trevor zegras#jdtz#jamie drysdale/trevor zegras#pittsburgh penguins#edmonton oilers#florida panthers#new jersey devils
466 notes
·
View notes
Text
NO AI
TL;DR: almost all social platforms are stealing your art and use it to train generative AI (or sell your content to AI developers); please beware and do something. Or don’t, if you’re okay with this.
Which platforms are NOT safe to use for sharing you art:
Facebook, Instagram and all Meta products and platforms (although if you live in the EU, you can forbid Meta to use your content for AI training)
Reddit (sold out all its content to OpenAI)
Twitter
Bluesky (it has no protection from AI scraping and you can’t opt out from 3rd party data / content collection yet)
DeviantArt, Flikr and literally every stock image platform (some didn’t bother to protect their content from scraping, some sold it out to AI developers)
Here’s WHAT YOU CAN DO:
1. Just say no:
Block all 3rd party data collection: you can do this here on Tumblr (here’s how); all other platforms are merely taking suggestions, tbh
Use Cara (they can’t stop illegal scraping yet, but they are currently working with Glaze to built in ‘AI poisoning’, so… fingers crossed)
2. Use art style masking tools:
Glaze: you can a) download the app and run it locally or b) use Glaze’s free web service, all you need to do is register. This one is a fav of mine, ‘cause, unlike all the other tools, it doesn’t require any coding skills (also it is 100% non-commercial and was developed by a bunch of enthusiasts at the University of Chicago)
Anti-DreamBooth: free code; it was originally developed to protect personal photos from being used for forging deepfakes, but it works for art to
Mist: free code for Windows; if you use MacOS or don’t have powerful enough GPU, you can run Mist on Google’s Colab Notebook
(art style masking tools change some pixels in digital images so that AI models can’t process them properly; the changes are almost invisible, so it doesn’t affect your audiences perception)
3. Use ‘AI poisoning’ tools
Nightshade: free code for Windows 10/11 and MacOS; you’ll need GPU/CPU and a bunch of machine learning libraries to use it though.
4. Stay safe and fuck all this corporate shit.
75 notes
·
View notes
Text
"how do I keep my art from being scraped for AI from now on?"
if you post images online, there's no 100% guaranteed way to prevent this, and you can probably assume that there's no need to remove/edit existing content. you might contest this as a matter of data privacy and workers' rights, but you might also be looking for smaller, more immediate actions to take.
...so I made this list! I can't vouch for the effectiveness of all of these, but I wanted to compile as many options as possible so you can decide what's best for you.
Discouraging data scraping and "opting out"
robots.txt - This is a file placed in a website's home directory to "ask" web crawlers not to access certain parts of a site. If you have your own website, you can edit this yourself, or you can check which crawlers a site disallows by adding /robots.txt at the end of the URL. This article has instructions for blocking some bots that scrape data for AI.
HTML metadata - DeviantArt (i know) has proposed the "noai" and "noimageai" meta tags for opting images out of machine learning datasets, while Mojeek proposed "noml". To use all three, you'd put the following in your webpages' headers:
<meta name="robots" content="noai, noimageai, noml">
Have I Been Trained? - A tool by Spawning to search for images in the LAION-5B and LAION-400M datasets and opt your images and web domain out of future model training. Spawning claims that Stability AI and Hugging Face have agreed to respect these opt-outs. Try searching for usernames!
Kudurru - A tool by Spawning (currently a Wordpress plugin) in closed beta that purportedly blocks/redirects AI scrapers from your website. I don't know much about how this one works.
ai.txt - Similar to robots.txt. A new type of permissions file for AI training proposed by Spawning.
ArtShield Watermarker - Web-based tool to add Stable Diffusion's "invisible watermark" to images, which may cause an image to be recognized as AI-generated and excluded from data scraping and/or model training. Source available on GitHub. Doesn't seem to have updated/posted on social media since last year.
Image processing... things
these are popular now, but there seems to be some confusion regarding the goal of these tools; these aren't meant to "kill" AI art, and they won't affect existing models. they won't magically guarantee full protection, so you probably shouldn't loudly announce that you're using them to try to bait AI users into responding
Glaze - UChicago's tool to add "adversarial noise" to art to disrupt style mimicry. Devs recommend glazing pictures last. Runs on Windows and Mac (Nvidia GPU required)
WebGlaze - Free browser-based Glaze service for those who can't run Glaze locally. Request an invite by following their instructions.
Mist - Another adversarial noise tool, by Psyker Group. Runs on Windows and Linux (Nvidia GPU required) or on web with a Google Colab Notebook.
Nightshade - UChicago's tool to distort AI's recognition of features and "poison" datasets, with the goal of making it inconvenient to use images scraped without consent. The guide recommends that you do not disclose whether your art is nightshaded. Nightshade chooses a tag that's relevant to your image. You should use this word in the image's caption/alt text when you post the image online. This means the alt text will accurately describe what's in the image-- there is no reason to ever write false/mismatched alt text!!! Runs on Windows and Mac (Nvidia GPU required)
Sanative AI - Web-based "anti-AI watermark"-- maybe comparable to Glaze and Mist. I can't find much about this one except that they won a "Responsible AI Challenge" hosted by Mozilla last year.
Just Add A Regular Watermark - It doesn't take a lot of processing power to add a watermark, so why not? Try adding complexities like warping, changes in color/opacity, and blurring to make it more annoying for an AI (or human) to remove. You could even try testing your watermark against an AI watermark remover. (the privacy policy claims that they don't keep or otherwise use your images, but use your own judgment)
given that energy consumption was the focus of some AI art criticism, I'm not sure if the benefits of these GPU-intensive tools outweigh the cost, and I'd like to know more about that. in any case, I thought that people writing alt text/image descriptions more often would've been a neat side effect of Nightshade being used, so I hope to see more of that in the future, at least!
245 notes
·
View notes
Text
Guide To Scrape Food Data Using Python & Google Colab
Are you tired of manually collecting food data for your recipe app or meal planning service? Look no further! With the power of web scraping and automation, you can easily gather all the necessary information for your food database. In this guide, we will show you how to scrape food data using Python and Google Colab.
What is Web Scraping?
Web scraping is the process of extracting data from websites. It involves using a program or script to automatically navigate through web pages and gather information. This data can then be saved in a structured format, such as a CSV or JSON file, for further analysis or use.
Why Use Python and Google Colab?
Python is a popular programming language for web scraping due to its ease of use and powerful libraries such as BeautifulSoup and Requests. Google Colab, on the other hand, is a free online platform for writing and running Python code. It also offers the ability to store and access data on Google Drive, making it a convenient choice for web scraping projects.
Setting Up Google Colab
Before we begin, make sure you have a Google account and are signed in to Google Drive. Then, go to Google Colab and create a new notebook. You can also upload an existing notebook if you have one.
Installing Libraries
To scrape data from websites, we will need to install two libraries: BeautifulSoup and Requests. In the first cell of your notebook, type the following code and run it:!pip install beautifulsoup4 !pip install requests
Scraping Food Data
Now, we are ready to start scraping food data. For this example, we will scrape data from a popular recipe website, Allrecipes.com. We will extract the recipe name, ingredients, and instructions for each recipe. First, we need to import the necessary libraries and specify the URL we want to scrape:
from bs4 import BeautifulSoup import requests
url = "https://www.allrecipes.com/recipes/84/healthy-recipes/"Next, we will use the Requests library to get the HTML content of the webpage and then use BeautifulSoup to parse it:
page = requests.get(url) soup = BeautifulSoup(page.content, 'html.parser')
Now, we can use BeautifulSoup to find the specific elements we want to scrape. In this case, we will use the "article" tag to find each recipe and then extract the recipe name, ingredients, and instructions:
recipes = soup.find_all('article')
for recipe in recipes: name = recipe.find('h3').text ingredients = recipe.find('ul', class_='ingredients-section').text
instructions = recipe.find('ol', class_='instructions-section').text
print(name) print(ingredients) print(instructions)
Finally, we can save the scraped data in a CSV file for further use:
import csv
with open('recipes.csv', 'w', newline='') as file:
writer = csv.writer(file) writer.writerow("Name", "Ingredients", "Instructions")
for recipe in recipes: name = recipe.find('h3').text ingredients = recipe.find('ul', class_='ingredients-section').text instructions = recipe.find('ol', class_='instructions-section').text writer.writerow([name, ingredients, instructions])
Conclusion
With just a few lines of code, we were able to��scrape food data from a website and save it in a structured format. This process can be automated and repeated for multiple websites to gather a large amount of data for your food database. Happy scraping!
#food data scraping#restaurant data scraping#food data scraping services#restaurantdataextraction#zomato api#grocerydatascraping#fooddatascrapingservices#grocerydatascrapingapi#web scraping services
0 notes
Text
For those who are not as familiar with Python and Google Colab - it's basically a shared notebook that lets you run my code, so you don't need any additional software or anything. I've hopefully commented everything so it should be fairly intuitive to run if you are not as familiar with how this sort of thing works. I've also enabled private outputs, meaning anything you do will not be saved - none of your ao3 data, your graphs, anything will be saved once you exit or even reload the page.
I know there will still be concerns about privacy and entering your data into a 3rd party app - I had concerns too, which is part of why I wanted to code it myself! If you want to know more about what I've done to hopefully maximise security - see below:
The way web scraping (how I get the ao3 data so I can run the stats) works is the code will connect to a web page and retrieve the HTML of the site - so it can get the information/text from the site.
Your Ao3 history page is password-protected however. That means, to access it, it does need a username and password. However, as mentioned, I'm using private outputs so that anything you enter into it cannot be saved or shared in any way. Any information you enter when running the code is deleted after the session is finished! If you are really concerned, you can save the notebook to your own Google Drive to run the code there, or even download the file to your own computer (but if you do that you need an application that can run Python code, such as Anaconda or Visual Studio Code).
A lot of the webscraping code is originally from klipklapper, (originally found here), an extension of teresachenec's wrapped from 2021, updated for the most recent ao3 API (as of 01/01/2024). I have also updated the function that retrieves your Ao3 history page so that the password is not saved after it accesses the page - it's deleted from memory! You can test that yourself by creating a new cell and typing the variable "password" (which is where your password is temporarily stored) or checking with the variable checker. It should come up as "variable not found" meaning it hasn't been stored!
If you really don't want to do it this way, I've also included a way to scrape data from your browser yourself using an extension - no password necessary! As long as you're already logged in (which you can do before you get the extension) you're good to go!
I know my code isn't perfect - and the way Ao3 saves your history (eg. each fic is only stored in your history once) means that the further back you go, the less accurate your stats will be if you've reread fics, and may make comparisons over time less accurate. It also assumes you've read every single work in your history all the way through only once - so word count may be off (I'm hoping the number of works you've not read fully cancels out the rereads in terms of word count).
However, if anyone who is more experienced with Python has any suggestion to optimise the code or improve security, or even ideas for further stats they'd be interested in I'd love to hear it! I can't guarantee that I'll work on it a lot, but any suggestions will be taken into consideration.
Whenever Spotify Wrapped releases, it reminds me of this project I've been thinking about for a while.
And now, The StoryGraph released its version of wrapped for the year (at the actual end of the year, as god intended) and I always think I read a lot less than I used to when I was younger.
Except...I still read on ao3. And I am, at heart, a stats nerd, (see me: nerding out about TSG graphs) so I've always wanted to analyse my ao3 reading history. I've seen people make their own version of ao3 wrapped, but I like having comprehensive stats, so instead of doing any of my actual coding work, I made my own version (with maybe too many stats and graphs according to my horrified non-coding friends I showed this to).
And I figured that there would be some folks out there who are as nerdy about this as I am and would appreciate it as well. So I made the code shareable and hopefully something that anyone can run.
So without further ado, here is my ao3 wrapped:
https://colab.research.google.com/drive/1DikTD0T9YjwPAL-Z3DTD8bdkM5fk_TS9?usp=sharing#scrollTo=cdaded40
Some things that my code can do:
scrape your data from Ao3 and organise it in a nice neat table you can download and keep
allow you to pick a time period
sample a random fic you read from that time period
look at the total number of fics you read, and what percentage of those have been deleted
look at how many works are on your 'Marked for Later' list and see information (top fandom and top characters) about them
show you graphs of the percentage of fics you read with each type of warning, rating, and ship type, as well as your ratio of completed works read
show you the last time you viewed a fic compared with the last time it was updated
the number of fics read over time (sorted by years, months or days depending on whether you are looking at all time, a certain year, or certain month respectively)
analyse the number of words you read and words read over time (similar to fics read over time)
identify your top 20 authors, fandoms, relationships, characters and tags
dynamically choose and plot the changes to your top 10 authors, fandoms, relationships, characters and tags over the time period (by years, months or days depending on the initial overview, similar to , read over time)
see how the rankings of your top 5 have changed from the previous time peroiod, and check the ranking of a specified author/fandom/ship/character/tag in that previous time period
So anyways, have fun!
95 notes
·
View notes
Text
How To Extract Restaurant Data Using Google Maps Data Scraping?
Do you need a comprehensive listing of restaurants having their addresses as well as ratings when you go for some holidays? Certainly, yes because it makes your path much easier and the coolest way to do that is using web scraping.
Data scraping or web scraping extracts data from the website to a local machine. The results are in spreadsheet form so you can have the whole listing of restaurants accessible around me getting their address and ratings in easy spreadsheets!
Here at Web Screen Scraping, we utilize Python 3 scripts for scraping food and restaurant data as well as installing Python might be extremely useful. For script proofreading, we have used Google Colab to run a script because it assists us in running Python scripts using the cloud.
As our purpose is to get a complete list of different places, extracting Google Maps data is the answer! With Google Maps scraping, it’s easy to scrape a place name, kind of place, coordinates, address, phone number, ratings, and other vital data. For starting, we can utilize a Place Scraping API. Using a Place Scraping API, it’s very easy to scrape Places data.
1st Step: Which data is needed?
Here, we would search for the “restaurants around me” phrase in Sanur, Bali in a radius of 1 km. So, the parameters could be ‘restaurants’, ‘Sanur Beach’, and ‘1 km’.
Let’s translate that into Python:
coordinates = ['-8.705833, 115.261377'] keywords = ['restaurant'] radius = '1000' api_key = 'acbhsjbfeur2y8r' #insert your API key here
All the ‘keywords’ will help us get places that are listed as results or restaurants having ‘restaurants’ in them. It’s superior than utilize the ‘types’ or ‘names’ of the places because we can get a complete list of different places that the name and type, has ‘restaurant’. For example, we could use restaurant names like Sushi Tei & Se’i Sapi. In case, we utilize ‘names’, then we’ll have places whose names are having a ‘restaurant’ word in that. In case, we utilize ‘type’, then we’ll have places where any type is a ‘restaurant’. Though, the drawback of utilizing ‘keywords’ is, this will need extra time to clean data.
2nd Step: Create some required libraries, like:
import pandas as pd, numpy as np import requests import json import time from google.colab import files
Have you observed “from imported files of google.colab”? Yes, the usage of the Google Colab requires us to use google.colab library to open or save data files.
3rd Step: Write the code that produces data relying on the given parameters in 1st Step.
for coordinate in coordinates: for keyword in keywords:url = 'https://maps.googleapis.com/maps/api/place/nearbysearch/json?location='+coordinate+'&radius='+str(radius)+'&keyword='+str(keyword)+'&key='+str(api_key)while True: print(url) respon = requests.get(url) jj = json.loads(respon.text) results = jj['results'] for result in results: name = result['name'] place_id = result ['place_id'] lat = result['geometry']['location']['lat'] lng = result['geometry']['location']['lng'] rating = result['rating'] types = result['types'] vicinity = result['vicinity']data = [name, place_id, lat, lng, rating, types, vicinity] final_data.append(data)time.sleep(5)if 'next_page_token' not in jj: break else:next_page_token = jj['next_page_token']url = 'https://maps.googleapis.com/maps/api/place/nearbysearch/json?key='+str(api_key)+'&pagetoken='+str(next_page_token)labels = ['Place Name','Place ID', 'Latitude', 'Longitude', 'Types', 'Vicinity']
The code will help us find a place’s name, ids, ratings, latitude-longitude, kinds, and areas for all keywords as well as their coordinates. Because Google displays merely 20 entries on each page, we had to add ‘next_page_token’ to scrape the data of the next page. Let’s accept we are having 40 restaurants close to Sanur, then Google will display results on two pages. For 65 results, there will be four pages.
The utmost data points, which we extract are only 60 places. It is a rule of Google. For example, 140 restaurants are available around Sanur within a radius of 1 km from where we had started. It means that only 60 of the total 140 restaurants will get produced. So, to avoid inconsistencies, we have to control the radius and also coordinate proficiently. Please make certain that the radius doesn’t become very wide, which results in “only 60 points are made whereas there are several of them”. Moreover, just ensure that the radius isn’t extremely small, which results in listing different coordinates. Both of them could not become well-organized, so we need to understand the context of the location previously.
4th Step: Save this data into a local machine
export_dataframe_1_medium = pd.DataFrame.from_records(final_data, columns=labels) export_dataframe_1_medium.to_csv('export_dataframe_1_medium.csv')
Last Step: Associate all these steps with the complete code:
import pandas as pd, numpy as np import requests import json import time final_data = []# Parameters coordinates = ['-8.705833, 115.261377'] keywords = ['restaurant'] radius = '1000' api_key = 'acbhsjbfeur2y8r' #insert your Places APIfor coordinate in coordinates: for keyword in keywords:url = 'https://maps.googleapis.com/maps/api/place/nearbysearch/json?location='+coordinate+'&radius='+str(radius)+'&keyword='+str(keyword)+'&key='+str(api_key)while True: print(url) respon = requests.get(url) jj = json.loads(respon.text) results = jj['results'] for result in results: name = result['name'] place_id = result ['place_id'] lat = result['geometry']['location']['lat'] lng = result['geometry']['location']['lng'] rating = result['rating'] types = result['types'] vicinity = result['vicinity']data = [name, place_id, lat, lng, rating, types, vicinity] final_data.append(data)time.sleep(5)if 'next_page_token' not in jj: break else:next_page_token = jj['next_page_token']url = 'https://maps.googleapis.com/maps/api/place/nearbysearch/json?key='+str(api_key)+'&pagetoken='+str(next_page_token)labels = ['Place Name','Place ID', 'Latitude', 'Longitude', 'Types', 'Vicinity']export_dataframe_1_medium = pd.DataFrame.from_records(final_data, columns=labels) export_dataframe_1_medium.to_csv('export_dataframe_1_medium.csv')
Now, it’s easy to download data from various Google Colab files. You just need to click on an arrow button provided on the left-side pane as well as click ‘Files’ to download data!
Your extracted data would be saved in CSV format as well as it might be imagined with tools that you’re well aware of! It could be R, Python, Tableau, etc. So, we have imagined that using Kepler.gl; a WebGL authorized, data agnostic, as well as high-performance web apps for geospatial analytical visualizations.
This is how the resulted data would look like in a spreadsheet:
And, this is how it looks in a Kepler.gl map:
We can see 59 restaurants from the Sanur beach. Just require to add names and ratings in the map as well as we’re prepared to search foods around the area!
Still not sure about how to scrape food data with Google Maps Data Scraping? Contact Web Screen Scraping for more details!
1 note
·
View note
Text
How To Extract Restaurant Data Using Google Maps Data Scraping?
Do you need a comprehensive listing of restaurants having their addresses as well as ratings when you go for some holidays? Certainly, yes because it makes your path much easier and the coolest way to do that is using web scraping.
Data scraping or web scraping extracts data from the website to a local machine. The results are in spreadsheet form so you can have the whole listing of restaurants accessible around me getting their address and ratings in easy spreadsheets!
Here at Web Screen Scraping, we utilize Python 3 scripts for scraping food and restaurant data as well as installing Python might be extremely useful. For script proofreading, we have used Google Colab to run a script because it assists us in running Python scripts using the cloud.
As our purpose is to get a complete list of different places, extracting Google Maps data is the answer! With Google Maps scraping, it’s easy to scrape a place name, kind of place, coordinates, address, phone number, ratings, and other vital data. For starting, we can utilize a Place Scraping API. Using a Place Scraping API, it’s very easy to scrape Places data.
1st Step: Which data is needed?
Here, we would search for the “restaurants around me” phrase in Sanur, Bali in a radius of 1 km. So, the parameters could be ‘restaurants’, ‘Sanur Beach’, and ‘1 km’.
Let’s translate that into Python:
coordinates = ['-8.705833, 115.261377'] keywords = ['restaurant'] radius = '1000' api_key = 'acbhsjbfeur2y8r' #insert your API key here
All the ‘keywords’ will help us get places that are listed as results or restaurants having ‘restaurants’ in them. It’s superior than utilize the ‘types’ or ‘names’ of the places because we can get a complete list of different places that the name and type, has ‘restaurant’. For example, we could use restaurant names like Sushi Tei & Se’i Sapi. In case, we utilize ‘names’, then we’ll have places whose names are having a ‘restaurant’ word in that. In case, we utilize ‘type’, then we’ll have places where any type is a ‘restaurant’. Though, the drawback of utilizing ‘keywords’ is, this will need extra time to clean data.
2nd Step: Create some required libraries, like:
import pandas as pd, numpy as np import requests import json import time from google.colab import files
Have you observed “from imported files of google.colab”? Yes, the usage of the Google Colab requires us to use google.colab library to open or save data files.
3rd Step: Write the code that produces data relying on the given parameters in 1st Step.
for coordinate in coordinates: for keyword in keywords:url = 'https://maps.googleapis.com/maps/api/place/nearbysearch/json?location='+coordinate+'&radius='+str(radius)+'&keyword='+str(keyword)+'&key='+str(api_key)while True: print(url) respon = requests.get(url) jj = json.loads(respon.text) results = jj['results'] for result in results: name = result['name'] place_id = result ['place_id'] lat = result['geometry']['location']['lat'] lng = result['geometry']['location']['lng'] rating = result['rating'] types = result['types'] vicinity = result['vicinity']data = [name, place_id, lat, lng, rating, types, vicinity] final_data.append(data)time.sleep(5)if 'next_page_token' not in jj: break else:next_page_token = jj['next_page_token']url = 'https://maps.googleapis.com/maps/api/place/nearbysearch/json?key='+str(api_key)+'&pagetoken='+str(next_page_token)labels = ['Place Name','Place ID', 'Latitude', 'Longitude', 'Types', 'Vicinity']
The code will help us find a place’s name, ids, ratings, latitude-longitude, kinds, and areas for all keywords as well as their coordinates. Because Google displays merely 20 entries on each page, we had to add ‘next_page_token’ to scrape the data of the next page. Let’s accept we are having 40 restaurants close to Sanur, then Google will display results on two pages. For 65 results, there will be four pages.
The utmost data points, which we extract are only 60 places. It is a rule of Google. For example, 140 restaurants are available around Sanur within a radius of 1 km from where we had started. It means that only 60 of the total 140 restaurants will get produced. So, to avoid inconsistencies, we have to control the radius and also coordinate proficiently. Please make certain that the radius doesn’t become very wide, which results in “only 60 points are made whereas there are several of them”. Moreover, just ensure that the radius isn’t extremely small, which results in listing different coordinates. Both of them could not become well-organized, so we need to understand the context of the location previously.
4th Step: Save this data into a local machine
export_dataframe_1_medium = pd.DataFrame.from_records(final_data, columns=labels) export_dataframe_1_medium.to_csv('export_dataframe_1_medium.csv')
Last Step: Associate all these steps with the complete code:
import pandas as pd, numpy as np import requests import json import time final_data = []# Parameters coordinates = ['-8.705833, 115.261377'] keywords = ['restaurant'] radius = '1000' api_key = 'acbhsjbfeur2y8r' #insert your Places APIfor coordinate in coordinates: for keyword in keywords:url = 'https://maps.googleapis.com/maps/api/place/nearbysearch/json?location='+coordinate+'&radius='+str(radius)+'&keyword='+str(keyword)+'&key='+str(api_key)while True: print(url) respon = requests.get(url) jj = json.loads(respon.text) results = jj['results'] for result in results: name = result['name'] place_id = result ['place_id'] lat = result['geometry']['location']['lat'] lng = result['geometry']['location']['lng'] rating = result['rating'] types = result['types'] vicinity = result['vicinity']data = [name, place_id, lat, lng, rating, types, vicinity] final_data.append(data)time.sleep(5)if 'next_page_token' not in jj: break else:next_page_token = jj['next_page_token']url = 'https://maps.googleapis.com/maps/api/place/nearbysearch/json?key='+str(api_key)+'&pagetoken='+str(next_page_token)labels = ['Place Name','Place ID', 'Latitude', 'Longitude', 'Types', 'Vicinity']export_dataframe_1_medium = pd.DataFrame.from_records(final_data, columns=labels) export_dataframe_1_medium.to_csv('export_dataframe_1_medium.csv')
Now, it’s easy to download data from various Google Colab files. You just need to click on an arrow button provided on the left-side pane as well as click ‘Files’ to download data!
Your extracted data would be saved in CSV format as well as it might be imagined with tools that you’re well aware of! It could be R, Python, Tableau, etc. So, we have imagined that using Kepler.gl; a WebGL authorized, data agnostic, as well as high-performance web apps for geospatial analytical visualizations.
This is how the resulted data would look like in a spreadsheet:
And, this is how it looks in a Kepler.gl map:
We can see 59 restaurants from the Sanur beach. Just require to add names and ratings in the map as well as we’re prepared to search foods around the area!
Still not sure about how to scrape food data with Google Maps Data Scraping? Contact Web Screen Scraping for more details!
1 note
·
View note
Text
How To Extract Food Data From Google Maps With Google Colab & Python?
Do you want a comprehensive list of restaurants with reviews and locations every time you visit a new place or go on vacation? Sure you do, because it makes your life so much easier. Data scraping is the most convenient method.
Web scraping, also known as data scraping, is the process of transferring information from a website to a local network. The result is in the form of spreadsheets. So you can get a whole list of restaurants in your area with addresses and ratings in one simple spreadsheet! In this blog, you will learn how to use Python and Google Colab to Extract food data From Google Maps.
WWe are scraping restaurant and food data using Python 3 scripts since installing Python can be pretty handy. We use Google Colab to run the proofreading script since it allows us to run Python scripts on the server.
As our objective is to get a detailed listing of locations, extracting Google Maps data is an ideal solution. Using Google Maps data scraping, you can scrape data like name, area, location, place types, ratings, phone numbers, and other applicable information. For startups, we can utilize a places data scraping API. A places Scraping API makes that very easy to scrape location data.
Step 1: What information would you need?
For example, here we are searching for "restaurants near me" in Sanur, Bali, within 1 kilometer. So the criteria could be "restaurants," "Sanur Beach," and "1 mile."Let us convert this into Python:
These "keywords" help us find places categorized as restaurants OR results that contain the term "restaurant." A comprehensive list of sites whose names and types both have the word "restaurant" is better than using "type" or "name" of places.
For example, we can make reservations at Se'i Sapi and Sushi Tei at the same time. If we use the term "name," we will only see places whose names contain the word "restaurant." If we use the word "type," we get areas whose type is "restaurant." However, using "keywords" has the disadvantage that data cleaning takes longer.
Step 2: Create some necessary libraries, like:
Create some necessary modules, such as:
The "files imported from google. colab" did you notice? Yes, to open or save data in Google Colab, we need to use google. colab library.
Step 3: Create a piece of code that generates data based on the first Step's variables.
With this code, we get the location's name, longitude, latitude, IDs, ratings, and area for each keyword and coordinate. Suppose there are 40 locales near Sanur; Google will output the results on two pages. If there are 55 results, there are three pages. Since Google only shows 20 entries per page, we need to specify the 'next page token' to retrieve the following page data.
The maximum number of data points we retrieve is 60, which is Google's policy. For example, within one kilometer of our starting point, there are 140 restaurants. This means that only 60 of the 140 restaurants will be created.
So, to avoid inconsistencies, we need to get both the radius and the coordinates right. Ensure that the diameter is not too large so that "only 60 points are created, although there are many of them". Also, ensure the radius is manageable, as this would result in a long list of coordinates. Neither can be efficient, so we need to capture the context of a location earlier.
Continue reading the blog to learn more how to extract data from Google Maps using Python.
Step 4: Store information on the user's computer
Final Step: To integrate all these procedures into a complete code:
You can now quickly download data from various Google Colab files. To download data, select "Files" after clicking the arrow button in the left pane!
Your data will be scraped and exported in CSV format, ready for visualization with all the tools you know! This can be Tableau, Python, R, etc. Here we used Kepler.gl for visualization, a powerful WebGL-enabled web tool for geographic diagnostic visualizations.
The data is displayed in the spreadsheet as follows:
In the Kepler.gl map, it is shown as follows:
From our location, lounging on Sanur beach, there are 59 nearby eateries. Now we can explore our neighborhood cuisine by adding names and reviews to a map!
Conclusion:
Food data extraction using Google Maps, Python, and Google Colab can be an efficient and cost-effective way to obtain necessary information for studies, analysis, or business purposes. However, it is important to follow Google Maps' terms of service and use the data ethically and legally. However, you should be aware of limitations and issues, such as managing web-based applications, dealing with CAPTCHA, and avoiding Google blocking.
Are you looking for an expert Food Data Scraping service provider? Contact us today! Visit the Food Data Scrape website and get more information about Food Data Scraping and Mobile Grocery App Scraping. Know more : https://www.fooddatascrape.com/how-to-extract-food-data-from-google-maps-with-google-colab-python.php
#Extract Food Data From Google Maps#extracting Google Maps data#Google Maps data scraping#Food data extraction using Google Maps
0 notes
Text
Zyneto Technologies: Leading Mobile App Development Companies in the US & India
In today’s mobile-first world, having a robust and feature-rich mobile application is key to staying ahead of the competition. Whether you’re a startup or an established enterprise, the right mobile app development partner can help elevate your business. Zyneto Technologies is recognized as one of the top mobile app development companies in the USA and India, offering innovative and scalable solutions that meet the diverse needs of businesses across the globe.
Why Zyneto Technologies Stands Out Among Mobile App Development Companies in the USA and India
Zyneto Technologies is known for delivering high-quality mobile app development solutions that are tailored to your business needs. With a team of highly skilled developers, they specialize in building responsive, scalable, and feature
website- zyneto.com
#devops automation tools#devops services and solutions#devops solutions and services#devops solution providers#devops solutions company#devops solutions and service provider company#devops services#devops development services#devops consulting service#devops services company#web scraping solutions#web scraping chrome extension free#web scraping using google colab#selenium web scraping#best web scraping tools#node js web scraping#artificial intelligence web scraping#beautiful soup web scraping#best web scraping software#node js for web scraping#web scraping software#web scraping ai#free web scraping tools#web scraping python beautifulsoup#selenium web scraping python#web scraping with selenium and python#web site development#website design company near me#website design companies near me#website developers near me
0 notes
Text
Java download file from url

Java download file from url how to#
Java download file from url pdf#
Java download file from url generator#
Java download file from url code#
Java download file from url zip#
While ((numBytesRead = bufferedInputStream.read(bucket, 0, bucket.
Node.InputStream inputStream = url.openStream() īufferedInputStream bufferedInputStream = new BufferedInputStream(inputStream) įileOutputStream fileOutputStream = new FileOutputStream(outputPath).
Java download file from url pdf#
Vue.js Mozilla PDF.js pdfvuer Module PDF Viewer Example to Display PDF Documents in Browser Using Javascript Full Project For Beginners when the user clicks on the link, it downloads a file from a server url.Python 3 Script to Download PDF Files From URL Using BeautifulSoup4 and Requests Library Full Tutorial For Beginners.Python Tkinter GUI Script to Download PDF Document From URL Desktop App Full Project For Beginners.In this example, we are creating three files: index. Example of downloading file from the server in servlet. But if there is any java file or jsp file etc, you need to create a program to download that file. So there is no need to write the program to download.
Java download file from url zip#
Vue.js PDF.js Library PDF Viewer Example to Display PDF Documents inside Browser Using Javascript Full Project Full Project For Beginners If there is any jar or zip file, you can direct provide a link to that file.
You use the BufferedInputStream class to read the contents of a.
Java download file from url how to#
Python 3 Selenium Web Scraping Script to Take Screenshot of Website URL and Download it as PNG Image File Full Project For Beginners In this tutorial, you learn how to download a file from a URL using the Java IO package.
How to Download File From URL to Google Drive Using Google Colab in Python Full Project For Beginners.
For convenient, script-driven extraction of the downloadable file URLs and.
Java download file from url code#
Javascript PDFMake Library to Export jQuery HTML DataTable to CSV,Excel and PDF Documents in Browser Full Project For Beginners website and especially the download page for source code and.Build a Sapui5 PDF Viewer With Custom Validation Error Messages and Download PDF Button in Browser Using Vanilla Javascript Full Project For Beginners.
Java download file from url generator#
Vue.js Dynamic JSON Data File Generator With Live Preview From Form Field Values and Download as JSON Blob File in Browser Using Javascript Full Project For Beginners.
Python Tkinter Script to Build Download Manger to Download Bulk Multiple Files From URL with Progressbar Animation Using PySmartDL Library Full Project For Beginners.
Using ansferFrom () method class in Java provides several methods for reading, writing, mapping, and manipulating a file. This post provides an overview of some of the available alternatives to accomplish this. Here is Spring boot example to download a file but this code can. It can also be used as the assignment target for a method reference or a lambda expression. StreamingResponseBody is a functional interface. Download File Using StreamingResponseBody.
Python 3 wkhtmltopdf Script to Convert HTML File to PDF or Website URL to PDF Document Using PDFKit Library Full Project For Beginners There are several ways to download a file from a URL in Java. Here we will see following three methods to download a file directly to the client easily: 1.
Sometimes we want to save a web file to our own computer.
Vue.js Mozilla PDF.js vue-pdf-app Library PDF Viewer to Display PDF Documents in Browser Using Javascript Full Tutorial For Beginners The curl tool lets us fetch a given URL from the command-line.
Now sync option will appear at the top right corner click on the sync now option. implementation ‘:android-pdf-viewer:2.8.2’. ReadableByteChannel readChannel Channels.newChannel ( new URL ( ' ).openStream ()) The ReadableByteChannel class creates a stream to read content from the URL.
Node.js Project to Encode Local PDF File or From URL to Base64 String Using pdf-to-base64 Library in Javascript Full Project For Beginners Step 2: Add dependency to adle (Module:app) Navigate to the Gradle Scripts > adle (Module:app) and add the below dependency in the dependencies section. In order to download the contents of a URL, we will use the ReadableByteChannel and the FileChannel classes.
Python 3 Script to Download Image or PDF File From URL Using Requests and Validators Library Full Project For Beginners.
This code will do for downloading a file from path, This code will also work if you have saved your path in database and to download from that path.
jsPDF Tutorial to Export and Print Div HTML Content to PDF Document and Download it as PDF File in Browser Using Javascript Full Project For Beginners In this article we are going to see how to download a file from a folder using Java, In addition to that we are also going to see how to change the format of that file and download it.
jQuery FullCalendar Integration Using PHP MySQL & AJAX.
Open a browser and navigate to the REST services endpoint of the USA map service (URL: http://<.>Image Crop and Upload using JQuery with PHP Ajax Test the SOE in the ArcGIS Server Services Directory.
jQuery NiceForm – Form Validation & AJAX Submit Plugin.

0 notes
Text
How to Scrape IMDb Top Box Office Movies Data using Python?

Different Libraries for Data Scrapping
We all understand that in Python, you have various libraries for various objectives. We will use the given libraries:
BeautifulSoup: It is utilized for web scraping objectives for pulling data out from XML and HTML files. It makes a parse tree using page source codes, which can be utilized to scrape data in a categorized and clearer manner.
Requests: It allows you to send HTTP/1.1 requests with Python. Using it, it is easy to add content including headers, multipart files, form data, as well as parameters through easy Python libraries. This also helps in accessing response data from Python in a similar way.
Pandas: It is a software library created for Python programming language to do data analysis and manipulation. Particularly, it provides data operations and structures to manipulate numerical tables as well as time series.
For scraping data using data extraction with Python, you have to follow some basic steps:
1: Finding the URL:
Here, we will extract IMDb website data to scrape the movie title, gross, weekly growth, as well as total weeks for the finest box office movies in the US. This URL for a page is https://www.imdb.com/chart/boxoffice/?ref_=nv_ch_cht
2: Reviewing the Page
Do right-click on that element as well as click on the “Inspect” option.
3: Get the Required Data to Scrape
Here, we will go to scrape data including movies title, weekly growth, and name, gross overall, and total weeks are taken for it that is in “div” tag correspondingly.
4: Writing the Code
For doing that, you can utilize Jupiter book or Google Colab. We are utilizing Google Colab here:
Import libraries:
import requests from bs4 import BeautifulSoup import pandas as pd
Make empty arrays and we would utilize them in the future to store data of a particular column.
TitleName=[] Gross=[] Weekend=[] Week=[]
Just open the URL as well as scrape data from a website.
url = "https://www.imdb.com/chart/boxoffice/?ref_=nv_ch_cht" r = requests.get(url).content
With the use of Find as well as Find All techniques in BeautifulSoup, we scrape data as well as store that in a variable.
soup = BeautifulSoup(r, "html.parser") list = soup.find("tbody", {"class":""}).find_all("tr") x = 1 for i in list: title = i.find("td",{"class":"titleColumn"}) gross = i.find("span",{"class":"secondaryInfo"}) weekend = i.find("td",{"class":"ratingColumn"}) week=i.find("td",{"class":"weeksColumn"}
With the append option, we store all the information in an Array, which we have made before.
TitleName.append(title.text) Gross.append(gross.text) Weekend.append(weekend.text) Week.append(week.text)
5. Storing Data in the Sheet. We Store Data in the CSV Format
df=pd.DataFrame({'Movie Title':TitleName, 'Weekend':Weekend, 'Gross':Gross, 'Week':Week}) df.to_csv('DS-PR1-18IT012.csv', index=False, encoding='utf-8')
6. It’s Time to Run the Entire Code
All the information is saved as IMDbRating.csv within the path of a Python file.
For more information, contact 3i Data Scraping or ask for a free quote about IMDb Top Box Office Movies Data Scraping services.
0 notes
Text

Do you want a complete list of restaurants with their ratings and addresses whenever you visit a place or go for holidays? Off-course yes as it makes your way much easier. The easiest way to do it is using data scraping.
Web scraping or data scraping imports data from a website to the local machine. The result is in the form of spreadsheets so that you can get an entire list of restaurants available around me having its address as well as ratings in the easy spreadsheet!
Here at Foodspark, we use Python 3 scripts to scrape restaurant and food data as installing Python could be very useful. For proofreading the script, we use Google Colab for running the script as it helps us running the Python scripts on the cloud.
As our objective is to find a complete listing of places, scraping Google Maps data is its answer. Using Google Maps scraping, we can extract a place’s name, coordinates, address, kind of place, ratings, phone number, as well as other important data. For a start, we can also use a Places Scraping API. By using the Places Scraping API, it’s easy to extract Places data.
0 notes
Text
Using Python to recover SEO site traffic (Part two)
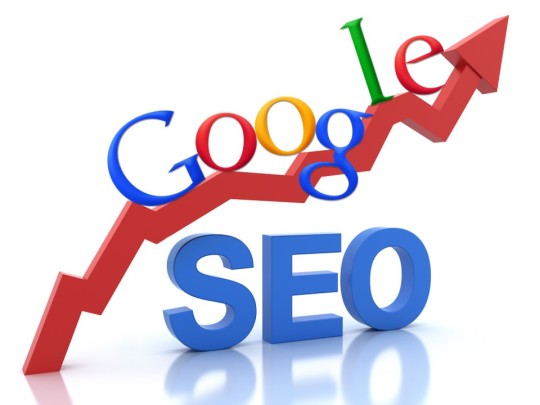
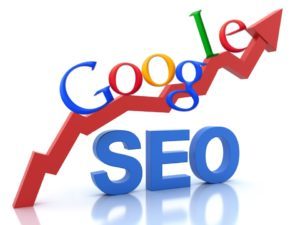
Automating the process of narrowing down site traffic issues with Python gives you the opportunity to help your clients recover fast. This is the second part of a three-part series. In part one, I introduced our approach to nail down the pages losing traffic. We call it the “winners vs losers” analysis. If you have a big site, reviewing individual pages losing traffic as we did on part one might not give you a good sense of what the problem is. So, in part two we will create manual page groups using regular expressions. If you stick around to read part three, I will show you how to group pages automatically using machine learning. You can find the code used in part one, two and three in this Google Colab notebook. Let’s walk over part two and learn some Python.
Incorporating redirects
As the site our analyzing moved from one platform to another, the URLs changed, and a decent number of redirects were put in place. In order to track winners and losers more accurately, we want to follow the redirects from the first set of pages. We were not really comparing apples to apples in part one. If we want to get a fully accurate look at the winners and losers, we’ll have to try to discover where the source pages are redirecting to, then repeat the comparison. 1. Python requests We’ll use the requests library which simplifies web scraping, to send an HTTP HEAD request to each URL in our Google Analytics data set, and if it returns a 3xx redirect, we’ll record the ultimate destination and re-run our winners and losers analysis with the correct, final URLs. HTTP HEAD requests speed up the process and save bandwidth as the web server only returns headers, not full HTML responses. Below are two functions we’ll use to do this. The first function takes in a single URL and returns the status code and any resulting redirect location (or None if there isn’t a redirect.) The second function takes in a list of URLs and runs the first function on each of them, saving all the results in a list. View the code on Gist. This process might take a while (depending on the number of URLs). Please note that we introduce a delay between requests because we don’t want to overload the server with our requests and potentially cause it to crash. We also only check for valid redirect status codes 301, 302, 307. It is not wise to check the full range as for example 304 means the page didn’t change. Once we have the redirects, however, we can repeat the winners and losers analysis exactly as before. 2. Using combine_first In part one we learned about different join types. We first need to do a left merge/join to append the redirect information to our original Google Analytics data frame while keeping the data for rows with no URLs in common. To make sure that we use either the original URL or the redirect URL if it exists, we use another data frame method called combine_first() to create a true_url column. For more information on exactly how this method works, see the combine_first documentation. We also extract the path from the URLs and format the dates to Python DateTime objects. View the code on Gist. 3. Computing totals before and after the switch View the code on Gist. 4. Recalculating winners vs losers View the code on Gist. 5. Sanity check View the code on Gist. This is what the output looks like.
Using regular expressions to group pages
Many websites have well-structured URLs that make their page types easy to parse. For example, a page with any one of the following paths given below is pretty clearly a paginated category page. /category/toys?page=1 /c/childrens-toys/3/ Meanwhile, a path structure like the paths given below might be a product page. /category/toys/basketball-product-1.html /category/toys/p/action-figure.html We need a way to categorize these pages based on the structure of the text contained in the URL. Luckily this type of problem (that is, examining structured text) can be tackled very easily with a “Domain Specific Language” known as Regular Expressions or “regex.” Regex expressions can be extremely complicated, or extremely simple. For example, the following regex query (written in python) would allow you to find the exact phrase “find me” in a string of text. regex = r"find me" Let’s try it out real quick. text = "If you can find me in this string of text, you win! But if you can't find me, you lose" regex = r"find me" print("Match index", "tMatch text") for match in re.finditer(regex, text): print(match.start(), "tt", match.group()) The output should be: Match index Match text 11 find me 69 find me Grouping by URL Now we make use of a slightly more advanced regex expression that contains a negative lookahead. Fully understanding the following regex expressions is left as an exercise for the reader, but suffice it to say we’re looking for “Collection” (aka “category”) pages and “Product” pages. We create a new column called “group” where we label any rows whose true_url match our regex string accordingly. Finally, we simply re-run our winners and losers’ analysis but instead of grouping by individual URLs like we did before, we group by the page type we found using regex. View the code on Gist. The output looks like this:
Plotting the results
Finally, we’ll plot the results of our regex-based analysis, to get a feel for which groups are doing better or worse. We’re going to use an open source plotting library called Plotly to do so. In our first set of charts, we’ll define 3 bar charts that will go on the same plot, corresponding to the traffic differences, data from before, and data from after our cutoff point respectively. We then tell Plotly to save an HTML file containing our interactive plot, and then we’ll display the HTML within the notebook environment. Notice that Plotly has grouped together our bar charts based on the “group” variable that we passed to all the bar charts on the x-axis, so now we can see that the “collections” group very clearly has had the biggest difference between our two time periods. View the code on Gist. We get this nice plot which you can interact within the Jupyter notebook!
Next up we’ll plot a line graph showing the traffic over time for all of our groups. Similar to the one above, we’ll create three separate lines that will go on the same chart. This time, however, we do it dynamically with a “for loop”. After we create the line graph, we can add some annotations using the Layout parameter when creating the Plotly figure. View the code on Gist. This produces this painful to see, but valuable chart.
Results
From the bar chart and our line graph, we can see two separate events occurred with the “Collections” type pages which caused a loss in traffic. Unlike the uncategorized pages or the product pages, something has gone wrong with collections pages in particular. From here we can take off our programmer hats, and put on our SEO hats and go digging for the cause of this traffic loss, now that we know that it’s the “Collections” pages which were affected the most. During further work with this client, we narrowed down the issue to massive consolidation of category pages during the move. We helped them recreate them from the old site and linked them from a new HTML sitemap with all the pages, as they didn’t want these old pages in the main navigation. Manually grouping pages is a valuable technique, but a lot of work if you need to work with many brands. In part three, the final part of the series, I will discuss a clever technique to group pages automatically using machine learning. Hamlet Batista is the CEO and founder of RankSense, an agile SEO platform for online retailers and manufacturers. He can be found on Twitter @hamletbatista. The post Using Python to recover SEO site traffic (Part two) appeared first on Search Engine Watch. Read the full article
0 notes
Text
Intro to Python - Whiteboard Friday
Posted by BritneyMuller
Python is a programming language that can help you uncover incredible SEO insights and save you time by automating time-consuming tasks. But for those who haven't explored this side of search, it can be intimidating. In this episode of Whiteboard Friday, Britney Muller and a true python expert named Pumpkin offer an intro into a helpful tool that's worth your time to learn.
Click on the whiteboard image above to open a high resolution version in a new tab!
Video Transcription
Hey, Moz fans. Welcome to another edition of Whiteboard Friday. Today we're talking all about introduction to Python, which is why I have a special co-host here. She is a ball python herself, total expert. Her name is Pumpkin, and she's the best.
What is Python?
So what is Python? This has been in the industry a lot lately. There's a lot of commotion that you should know how to use it or know how to talk about it. Python is an open source, object-oriented programming language that was created in 1991.
Simpler to use than R
Some fun facts about Python is it's often compared to R, but it's arguably more simple to use. The syntax just oftentimes feels more simple and common-sense, like when you're new to programming.
Big companies use it
Huge companies use it. NASA, Google, tons of companies out there use it because it's widely supported.
It's open source
It is open source. So pretty cool. While we're going through this Whiteboard Friday, I would love it if we would do a little Python programming today. So I'm just going to ask that you also visit this in another tab, python.org/downloads. Download the version for your computer and we'll get back to that.
Why does Python matter?
So why should you care?
Automates time-consuming tasks
Python is incredibly powerful because it helps you automate time-consuming tasks. It can do these things at scale so that you can free up your time to work on higher-level thinking, to work on more strategy. It's really, really exciting where these things are going.
Log file analysis
Some examples of that are things like log file analysis. Imagine if you could just set up an automated system with Python to alert you any time one of your primary pages wasn't being crawled as frequently as it typically is. You can do all sorts of things. Let's say Google crawls your robots.txt and it throws out a server error, which many of you know causes huge problems. It can alert you. You can set up scripts like that to do really comprehensive tasks.
Internal link analysis
Some other examples, internal link analysis, it can do a really great job of that.
Discover keyword opportunities
It can help you discover keyword opportunities by looking at bulk keyword data and identifying some really important indicators.
Image optimization
It's really great for things like image optimization. It can auto tag and alt text images. It can do really powerful things there.
Scrape websites
It can also scrape the websites that you're working with to do really high volume tasks.
Google Search Console data analysis
It can also pull Google Search Console data and do analysis on those types of things.
I do have a list of all of the individuals within SEO who are currently doing really, really powerful things with Python. I highly suggest you check out some of Hamlet Batista's recent scripts where he's using Python to do all sorts of really cool SEO tasks.
How do you run Python?
What does this even look like? So you've hopefully downloaded Python as a programming language on your computer. But now you need to run it somewhere. Where does that live?
Set up a virtual environment using Terminal
So first you should be setting up a virtual environment. But for the purpose of these examples, I'm just going to ask that you pull up your terminal application.
It looks like this. You could also be running Python within something like Jupyter Notebook or Google Colab. But just pull up your terminal and let's check and make sure that you've downloaded Python properly.
Check to make sure you've downloaded Python properly
So the first thing that you do is you open up the terminal and just type in "python --version." You should see a readout of the version that you downloaded for your computer. That's awesome.
Activate Python and perform basic tasks
So now we're just going to activate Python and do some really basic tasks. So just type in "python" and hit Enter. You should hopefully see these three arrow things within your terminal. From here, you can do something like print ("Hello, World!"). So you enter it exactly like you see it here, hit Enter, and it will say "Hello, World!" which is pretty cool.
You can also do fun things like just basic math. You can add two numbers together using something like this. So these are individual lines. After you complete the print (sum), you'll see the readout of the sum of those two numbers. You can randomly generate numbers. I realize these aren't direct SEO applications, but these are the silly things that give you confidence to run programs like what Hamlet talks about.
Have fun — try creating a random number generator
So I highly suggest you just have fun, create a little random number generator, which is really cool. Mine is pulling random numbers from 0 to 100. You can do 0 to 10 or whatever you'd like. A fun fact, after you hit Enter and you see that random number, if you want to continue, using your up arrow will pull up the last command within your terminal.
It even goes back to these other ones. So that's a really quick way to rerun something like a random number generator. You can just crank out a bunch of them if you want for some reason.
Automating different tasks
This is where you can start to get into really cool scripts as well for pulling URLs using Requests HTML. Then you can pull unique information from web pages.
You can pull at bulk tens of thousands of title tags within a URL list. You can pull things like H1s, canonicals, all sorts of things, and this makes it incredibly easy to do it at scale. One of my favorite ways to pull things from URLs is using xpath within Python.
This is a lot easier than it looks. So this might be an xpath for some websites, but websites are marked up differently. So when you're trying to pull something from a particular site, you can right-click into Chrome Developer Tools. Within Chrome Developer Tools, you can right-click what it is that you're trying to scrape with Python.
You just select "Copy xpath," and it will give you the exact xpath for that website, which is kind of a fun trick if you're getting into some of this stuff.
Libraries
What are libraries? How do we make this stuff more and more powerful? Python is really strong on its own, but what makes it even stronger are these libraries or packages which are add-ons that do incredible things.
This is just a small percentage of libraries that can do things like data collection, cleaning, visualization, processing, and deployment. One of my favorite ways to get some of the more popular packages is just to download Anaconda, because it comes with all of these commonly used, most popular packages.
So it's kind of a nice way to get all of it in one spot or at least most of them.
Learn more
So you've kind of dipped your toes and you kind of understand what Python is and what people are using it for. Where can you learn more? How can you start? Well, Codecademy has a really great Python course, as well as Google, Kaggle, and even the Python.org website have some really great resources that you can check out.
This is a list of individuals I really admire in the SEO space, who are doing incredible work with Python and have all inspired me in different ways. So definitely keep an eye on what they are up to:
Hamlet Batista
Ruth Everett
Tom Donahue
Kristin Tynski
Paul Shapiro
Tyler Reardon
JR Oakes
Hulya Coban
@Jessthebp
But yeah, Pumpkin and I have really enjoyed this, and we hope you did too. So thank you so much for joining us for this special edition of Whiteboard Friday. We will see you soon. Bye, guys.
Video transcription by Speechpad.com
Sign up for The Moz Top 10, a semimonthly mailer updating you on the top ten hottest pieces of SEO news, tips, and rad links uncovered by the Moz team. Think of it as your exclusive digest of stuff you don't have time to hunt down but want to read!
via Blogger https://ift.tt/35BzYsR #blogger #bloggingtips #bloggerlife #bloggersgetsocial #ontheblog #writersofinstagram #writingprompt #instapoetry #writerscommunity #writersofig #writersblock #writerlife #writtenword #instawriters #spilledink #wordgasm #creativewriting #poetsofinstagram #blackoutpoetry #poetsofig
0 notes
Text
Intro to Python - Whiteboard Friday
Posted by BritneyMuller
Python is a programming language that can help you uncover incredible SEO insights and save you time by automating time-consuming tasks. But for those who haven't explored this side of search, it can be intimidating. In this episode of Whiteboard Friday, Britney Muller and a true python expert named Pumpkin offer an intro into a helpful tool that's worth your time to learn.
Click on the whiteboard image above to open a high resolution version in a new tab!
Video Transcription
Hey, Moz fans. Welcome to another edition of Whiteboard Friday. Today we're talking all about introduction to Python, which is why I have a special co-host here. She is a ball python herself, total expert. Her name is Pumpkin, and she's the best.
What is Python?
So what is Python? This has been in the industry a lot lately. There's a lot of commotion that you should know how to use it or know how to talk about it. Python is an open source, object-oriented programming language that was created in 1991.
Simpler to use than R
Some fun facts about Python is it's often compared to R, but it's arguably more simple to use. The syntax just oftentimes feels more simple and common-sense, like when you're new to programming.
Big companies use it
Huge companies use it. NASA, Google, tons of companies out there use it because it's widely supported.
It's open source
It is open source. So pretty cool. While we're going through this Whiteboard Friday, I would love it if we would do a little Python programming today. So I'm just going to ask that you also visit this in another tab, python.org/downloads. Download the version for your computer and we'll get back to that.
Why does Python matter?
So why should you care?
Automates time-consuming tasks
Python is incredibly powerful because it helps you automate time-consuming tasks. It can do these things at scale so that you can free up your time to work on higher-level thinking, to work on more strategy. It's really, really exciting where these things are going.
Log file analysis
Some examples of that are things like log file analysis. Imagine if you could just set up an automated system with Python to alert you any time one of your primary pages wasn't being crawled as frequently as it typically is. You can do all sorts of things. Let's say Google crawls your robots.txt and it throws out a server error, which many of you know causes huge problems. It can alert you. You can set up scripts like that to do really comprehensive tasks.
Internal link analysis
Some other examples, internal link analysis, it can do a really great job of that.
Discover keyword opportunities
It can help you discover keyword opportunities by looking at bulk keyword data and identifying some really important indicators.
Image optimization
It's really great for things like image optimization. It can auto tag and alt text images. It can do really powerful things there.
Scrape websites
It can also scrape the websites that you're working with to do really high volume tasks.
Google Search Console data analysis
It can also pull Google Search Console data and do analysis on those types of things.
I do have a list of all of the individuals within SEO who are currently doing really, really powerful things with Python. I highly suggest you check out some of Hamlet Batista's recent scripts where he's using Python to do all sorts of really cool SEO tasks.
How do you run Python?
What does this even look like? So you've hopefully downloaded Python as a programming language on your computer. But now you need to run it somewhere. Where does that live?
Set up a virtual environment using Terminal
So first you should be setting up a virtual environment. But for the purpose of these examples, I'm just going to ask that you pull up your terminal application.
It looks like this. You could also be running Python within something like Jupyter Notebook or Google Colab. But just pull up your terminal and let's check and make sure that you've downloaded Python properly.
Check to make sure you've downloaded Python properly
So the first thing that you do is you open up the terminal and just type in "python --version." You should see a readout of the version that you downloaded for your computer. That's awesome.
Activate Python and perform basic tasks
So now we're just going to activate Python and do some really basic tasks. So just type in "python" and hit Enter. You should hopefully see these three arrow things within your terminal. From here, you can do something like print ("Hello, World!"). So you enter it exactly like you see it here, hit Enter, and it will say "Hello, World!" which is pretty cool.
You can also do fun things like just basic math. You can add two numbers together using something like this. So these are individual lines. After you complete the print (sum), you'll see the readout of the sum of those two numbers. You can randomly generate numbers. I realize these aren't direct SEO applications, but these are the silly things that give you confidence to run programs like what Hamlet talks about.
Have fun — try creating a random number generator
So I highly suggest you just have fun, create a little random number generator, which is really cool. Mine is pulling random numbers from 0 to 100. You can do 0 to 10 or whatever you'd like. A fun fact, after you hit Enter and you see that random number, if you want to continue, using your up arrow will pull up the last command within your terminal.
It even goes back to these other ones. So that's a really quick way to rerun something like a random number generator. You can just crank out a bunch of them if you want for some reason.
Automating different tasks
This is where you can start to get into really cool scripts as well for pulling URLs using Requests HTML. Then you can pull unique information from web pages.
You can pull at bulk tens of thousands of title tags within a URL list. You can pull things like H1s, canonicals, all sorts of things, and this makes it incredibly easy to do it at scale. One of my favorite ways to pull things from URLs is using xpath within Python.
This is a lot easier than it looks. So this might be an xpath for some websites, but websites are marked up differently. So when you're trying to pull something from a particular site, you can right-click into Chrome Developer Tools. Within Chrome Developer Tools, you can right-click what it is that you're trying to scrape with Python.
You just select "Copy xpath," and it will give you the exact xpath for that website, which is kind of a fun trick if you're getting into some of this stuff.
Libraries
What are libraries? How do we make this stuff more and more powerful? Python is really strong on its own, but what makes it even stronger are these libraries or packages which are add-ons that do incredible things.
This is just a small percentage of libraries that can do things like data collection, cleaning, visualization, processing, and deployment. One of my favorite ways to get some of the more popular packages is just to download Anaconda, because it comes with all of these commonly used, most popular packages.
So it's kind of a nice way to get all of it in one spot or at least most of them.
Learn more
So you've kind of dipped your toes and you kind of understand what Python is and what people are using it for. Where can you learn more? How can you start? Well, Codecademy has a really great Python course, as well as Google, Kaggle, and even the Python.org website have some really great resources that you can check out.
This is a list of individuals I really admire in the SEO space, who are doing incredible work with Python and have all inspired me in different ways. So definitely keep an eye on what they are up to:
Hamlet Batista
Ruth Everett
Tom Donahue
Kristin Tynski
Paul Shapiro
Tyler Reardon
JR Oakes
Hulya Coban
@Jessthebp
But yeah, Pumpkin and I have really enjoyed this, and we hope you did too. So thank you so much for joining us for this special edition of Whiteboard Friday. We will see you soon. Bye, guys.
Video transcription by Speechpad.com
Sign up for The Moz Top 10, a semimonthly mailer updating you on the top ten hottest pieces of SEO news, tips, and rad links uncovered by the Moz team. Think of it as your exclusive digest of stuff you don't have time to hunt down but want to read!
Intro to Python - Whiteboard Friday Theo dõi các thông tin khác tại: https://foogleseo.blogspot.com Intro to Python - Whiteboard Friday posted first on https://foogleseo.blogspot.com/ #FoogleSEO #luongthuyvy Nguồn: http://bit.ly/2QFTvnK #luongthuyvy
0 notes