#Applications of AI Sensors
Explore tagged Tumblr posts
Text
AI Sensors and Robotics: The Synergy of Automation
Artificial Intelligence (AI) Sensor technology is poised to transform the way we perceive and interact with the world around us. These intelligent sensors are ushering in a new era of automation, data-driven decision-making, and enhanced connectivity. In this blog, we will explore the incredible potential of AI sensors, their applications across various industries, and the challenges and…

View On WordPress
0 notes
Text
🚀🤖 The Future of Robotics is Here! 🤖🚀 👉 Meet the Unitree G1 Humanoid Robot 🤩💡 ✅ Walks at 2 m/s speed 🏃♂️ ✅ 360° Vision for Smart Navigation 👀🛰 ✅ Deep Learning for Real-World Tasks 📚💻 ✅ Handles Fragile Objects with Care 🥛🖐 ✅ Ideal for Healthcare 🏥, Manufacturing 🏭 & Space Exploration 🚀🌌 💵 Price starts at $116,000 💸 🔥 Game-changer for Industries! 💥 👉 Want to know more? Click here! 📲👇 🔗 #UnitreeG1 #HumanoidRobot #AI #FutureTech #Robotics #Innovation 🚀🛠
#advanced robot#affordable humanoid robot#Artificial Intelligence#construction robot#deep reinforcement learning#Future of Robotics#G1 EDU version#G1 robot#humanoid AI#humanoid robot#humanoid robot market#humanoid robot price#industrial automation#industrial robotics#manufacturing robot#research humanoid robot#robot for education#robot for industry#robot with 360-degree vision#robot with advanced sensors#robotics future#smart learning robot#space exploration robot#Unitree G1#Unitree G1 advanced technology#Unitree G1 applications#Unitree G1 features#Unitree G1 hand dexterity#Unitree G1 humanoid robot#Unitree G1 learning capability
1 note
·
View note
Text
There's a nuance to the Amazon AI checkout story that gets missed.
Because AI-assisted checkouts on its own isn't a bad thing:
This was a big story in 2022, about a bread-checkout system in Japan that turned out to be applicable in checking for cancer cells in sample slides.
But that bonus anti-cancer discovery isn't the subject here, the actual bread-checkout system is. That checkout system worked, because it wasn't designed with the intent of making the checkout cashier obsolete, rather, it was there to solve a real problem: it's hard to tell pastry apart at a glance, and the customers didn't like their bread with a plastic-wrapping and they didn't like the cashiers handling the bread to count loaves.
So they trained the system intentionally, under controlled circumstances, before testing and launching the tech. The robot does what it's good at, and it doesn't need to be omniscient because it's a tool, not a replacement worker.
Amazon, however, wanted to offload its training not just on an underpaid overseas staff, but on the customers themselves. And they wanted it out NOW so they could brag to shareholders about this new tech before the tech even worked. And they wanted it to replace a person, but not just the cashier. There were dreams of a world where you can't shoplift because you'd get billed anyway dancing in the investor's heads.
Only, it's one thing to make a robot that helps cooperative humans count bread, and it's another to try and make one that can thwart the ingenuity of hungry people.
The foreign workers performing the checkouts are actually supposed to be training the models. A lot of reports gloss over this in an effort to present the efforts as an outsourcing Mechanical Turk but that's really a side-effect. These models all work on datasets, and the only place you get a dataset of "this visual/sensor input=this purchase" is if someone is cataloging a dataset correlating the two...
Which Amazon could have done by simply putting the sensor system in place and correlating the purchase data from the cashiers with the sensor tracking of the customer. Just do that for as long as you need to build the dataset and test it by having it predict and compare in the background until you reach your preferred ratio. If it fails, you have a ton of market research data as a consolation prize.
But that could take months or years and you don't get to pump your stock until it works, and you don't get to outsource your cashiers while pretending you've made Westworld work.
This way, even though Amazon takes a little bit of a PR bloody nose, they still have the benefit of any stock increase this already produced, the shareholders got their dividends.
Which I suppose is a lot of words to say:
#amazon AI#ai discourse#amazon just walk out#just walk out#the only thing that grows forever is cancer#capitalism#amazon
147 notes
·
View notes
Text
Integration of AI in Driver Testing and Evaluation
Introduction: As technology continues to shape the future of transportation, Canada has taken a major leap in modernizing its driver testing procedures by integrating Artificial Intelligence (AI) into the evaluation process. This transition aims to enhance the objectivity, fairness, and efficiency of driving assessments, marking a significant advancement in how new drivers are tested and trained across the country.

Key Points:
Automated Test Scoring for Objectivity: Traditional driving test evaluations often relied heavily on human judgment, which could lead to inconsistencies or perceived bias. With AI-driven systems now analysing road test performance, scoring is based on standardized metrics such as speed control, reaction time, lane discipline, and compliance with traffic rules. These AI systems use sensor data, GPS tracking, and in-car cameras to deliver highly accurate, impartial evaluations, removing potential examiner subjectivity.
Real-Time Feedback Enhances Learning: One of the key benefits of AI integration is the ability to deliver immediate feedback to drivers once the test concludes. Drivers can now receive a breakdown of their performance in real time—highlighting both strengths and areas needing improvement. This timely feedback accelerates the learning process and helps individuals better prepare for future driving scenarios or retests, if required.
Enhanced Test Consistency Across Canada: With AI systems deployed uniformly across various testing centres, all applicants are assessed using the same performance parameters and technology. This ensures that no matter where in Canada a person takes their road test, the evaluation process remains consistent and fair. It also eliminates regional discrepancies and contributes to national standardization in driver competency.
Data-Driven Improvements to Driver Education: AI doesn’t just assess drivers—it collects and analyses test data over time. These insights are then used to refine driver education programs by identifying common mistakes, adjusting training focus areas, and developing better instructional materials. Platforms like licenseprep.ca integrate this AI-powered intelligence to update practice tools and learning modules based on real-world testing patterns.
Robust Privacy and Data Protection Measures: As personal driving data is collected during AI-monitored tests, strict privacy policies have been established to protect individual information. All recorded data is encrypted, securely stored, and only used for training and evaluation purposes. Compliance with national data protection laws ensures that drivers’ privacy is respected throughout the testing and feedback process.
Explore More with Digital Resources: For a closer look at how AI is transforming driver testing in Canada and to access AI-informed preparation materials, visit licenseprep.ca. The platform stays current with tech-enabled changes and offers resources tailored to the evolving standards in driver education.
#AIDrivingTests#SmartTesting#DriverEvaluation#TechInTransport#CanadaRoads#LicensePrepAI#FutureOfDriving
4 notes
·
View notes
Text

Researchers leverage inkjet printing to make a portable multispectral 3D camera
Researchers have used inkjet printing to create a compact multispectral version of a light field camera. The camera, which fits in the palm of the hand, could be useful for many applications including autonomous driving, classification of recycled materials and remote sensing. 3D spectral information can be useful for classifying objects and materials; however, capturing 3D spatial and spectral information from a scene typically requires multiple devices or time-intensive scanning processes. The new light field camera solves this challenge by simultaneously acquiring 3D information and spectral data in a single snapshot. "To our knowledge, this is the most advanced and integrated version of a multispectral light field camera," said research team leader Uli Lemmer from the Karlsruhe Institute of Technology in Germany. "We combined it with new AI methods for reconstructing the depth and spectral properties of the scene to create an advanced sensor system for acquiring 3D information."
Read more.
9 notes
·
View notes
Text
ARMxy Series Industrial Embeddedd Controller with Python for Industrial Automation
Case Details
1. Introduction
In modern industrial automation, embedded computing devices are widely used for production monitoring, equipment control, and data acquisition. ARM-based Industrial Embeddedd Controller, known for their low power consumption, high performance, and rich industrial interfaces, have become key components in smart manufacturing and Industrial IoT (IIoT). Python, as an efficient and easy-to-use programming language, provides a powerful ecosystem and extensive libraries, making industrial automation system development more convenient and efficient.
This article explores the typical applications of ARM Industrial Embeddedd Controller combined with Python in industrial automation, including device control, data acquisition, edge computing, and remote monitoring.
2. Advantages of ARM Industrial Embeddedd Controller in Industrial Automation
2.1 Low Power Consumption and High Reliability
Compared to x86-based industrial computers, ARM processors consume less power, making them ideal for long-term operation in industrial environments. Additionally, they support fanless designs, improving system stability.
2.2 Rich Industrial Interfaces
Industrial Embeddedd Controllerxy integrate GPIO, RS485/232, CAN, DIN/DO/AIN/AO/RTD/TC and other interfaces, allowing direct connection to various sensors, actuators, and industrial equipment without additional adapters.
2.3 Strong Compatibility with Linux and Python
Most ARM Industrial Embeddedd Controller run embedded Linux systems such as Ubuntu, Debian, or Yocto. Python has broad support in these environments, providing flexibility in development.
3. Python Applications in Industrial Automation
3.1 Device Control
On automated production lines, Python can be used to control relays, motors, conveyor belts, and other equipment, enabling precise logical control. For example, it can use GPIO to control industrial robotic arms or automation line actuators.
Example: Controlling a Relay-Driven Motor via GPIO
import RPi.GPIO as GPIO import time
# Set GPIO mode GPIO.setmode(GPIO.BCM) motor_pin = 18 GPIO.setup(motor_pin, GPIO.OUT)
# Control motor operation try: while True: GPIO.output(motor_pin, GPIO.HIGH) # Start motor time.sleep(5) # Run for 5 seconds GPIO.output(motor_pin, GPIO.LOW) # Stop motor time.sleep(5) except KeyboardInterrupt: GPIO.cleanup()
3.2 Sensor Data Acquisition and Processing
Python can acquire data from industrial sensors, such as temperature, humidity, pressure, and vibration, for local processing or uploading to a server for analysis.
Example: Reading Data from a Temperature and Humidity Sensor
import Adafruit_DHT
sensor = Adafruit_DHT.DHT22 pin = 4 # GPIO pin connected to the sensor
humidity, temperature = Adafruit_DHT.read_retry(sensor, pin) print(f"Temperature: {temperature:.2f}°C, Humidity: {humidity:.2f}%")
3.3 Edge Computing and AI Inference
In industrial automation, edge computing reduces reliance on cloud computing, lowers latency, and improves real-time response. ARM industrial computers can use Python with TensorFlow Lite or OpenCV for defect detection, object recognition, and other AI tasks.
Example: Real-Time Image Processing with OpenCV
import cv2
cap = cv2.VideoCapture(0) # Open camera
while True: ret, frame = cap.read() gray = cv2.cvtColor(frame, cv2.COLOR_BGR2GRAY) # Convert to grayscale cv2.imshow("Gray Frame", gray)
if cv2.waitKey(1) & 0xFF == ord('q'): break
cap.release() cv2.destroyAllWindows()
3.4 Remote Monitoring and Industrial IoT (IIoT)
ARM industrial computers can use Python for remote monitoring by leveraging MQTT, Modbus, HTTP, and other protocols to transmit real-time equipment status and production data to the cloud or build a private industrial IoT platform.
Example: Using MQTT to Send Sensor Data to the Cloud
import paho.mqtt.client as mqtt import json
def on_connect(client, userdata, flags, rc): print(f"Connected with result code {rc}")
client = mqtt.Client() client.on_connect = on_connect client.connect("broker.hivemq.com", 1883, 60) # Connect to public MQTT broker
data = {"temperature": 25.5, "humidity": 60} client.publish("industrial/data", json.dumps(data)) # Send data client.loop_forever()
3.5 Production Data Analysis and Visualization
Python can be used for industrial data analysis and visualization. With Pandas and Matplotlib, it can store data, perform trend analysis, detect anomalies, and improve production management efficiency.
Example: Using Matplotlib to Plot Sensor Data Trends
import matplotlib.pyplot as plt
# Simulated data time_stamps = list(range(10)) temperature_data = [22.5, 23.0, 22.8, 23.1, 23.3, 23.0, 22.7, 23.2, 23.4, 23.1]
plt.plot(time_stamps, temperature_data, marker='o', linestyle='-') plt.xlabel("Time (min)") plt.ylabel("Temperature (°C)") plt.title("Temperature Trend") plt.grid(True) plt.show()
4. Conclusion
The combination of ARM Industrial Embeddedd Controller and Python provides an efficient and flexible solution for industrial automation. From device control and data acquisition to edge computing and remote monitoring, Python's extensive library support and strong development capabilities enable industrial systems to become more intelligent and automated. As Industry 4.0 and IoT technologies continue to evolve, the ARMxy + Python combination will play an increasingly important role in industrial automation.
2 notes
·
View notes
Text


New diagnostic tool will help LIGO hunt gravitational waves
Machine learning tool developed by UCR researchers will help answer fundamental questions about the universe.
Finding patterns and reducing noise in large, complex datasets generated by the gravitational wave-detecting LIGO facility just got easier, thanks to the work of scientists at the University of California, Riverside.
The UCR researchers presented a paper at a recent IEEE big-data workshop, demonstrating a new, unsupervised machine learning approach to find new patterns in the auxiliary channel data of the Laser Interferometer Gravitational-Wave Observatory, or LIGO. The technology is also potentially applicable to large scale particle accelerator experiments and large complex industrial systems.
LIGO is a facility that detects gravitational waves — transient disturbances in the fabric of spacetime itself, generated by the acceleration of massive bodies. It was the first to detect such waves from merging black holes, confirming a key part of Einstein’s Theory of Relativity. LIGO has two widely-separated 4-km-long interferometers — in Hanford, Washington, and Livingston, Louisiana — that work together to detect gravitational waves by employing high-power laser beams. The discoveries these detectors make offer a new way to observe the universe and address questions about the nature of black holes, cosmology, and the densest states of matter in the universe.
Each of the two LIGO detectors records thousands of data streams, or channels, which make up the output of environmental sensors located at the detector sites.
“The machine learning approach we developed in close collaboration with LIGO commissioners and stakeholders identifies patterns in data entirely on its own,” said Jonathan Richardson, an assistant professor of physics and astronomy who leads the UCR LIGO group. “We find that it recovers the environmental ‘states’ known to the operators at the LIGO detector sites extremely well, with no human input at all. This opens the door to a powerful new experimental tool we can use to help localize noise couplings and directly guide future improvements to the detectors.”
Richardson explained that the LIGO detectors are extremely sensitive to any type of external disturbance. Ground motion and any type of vibrational motion — from the wind to ocean waves striking the coast of Greenland or the Pacific — can affect the sensitivity of the experiment and the data quality, resulting in “glitches” or periods of increased noise bursts, he said.
“Monitoring the environmental conditions is continuously done at the sites,” he said. “LIGO has more than 100,000 auxiliary channels with seismometers and accelerometers sensing the environment where the interferometers are located. The tool we developed can identify different environmental states of interest, such as earthquakes, microseisms, and anthropogenic noise, across a number of carefully selected and curated sensing channels.”
Vagelis Papalexakis, an associate professor of computer science and engineering who holds the Ross Family Chair in Computer Science, presented the team’s paper, titled “Multivariate Time Series Clustering for Environmental State Characterization of Ground-Based Gravitational-Wave Detectors,” at the IEEE's 5th International Workshop on Big Data & AI Tools, Models, and Use Cases for Innovative Scientific Discovery that took place last month in Washington, D.C.
“The way our machine learning approach works is that we take a model tasked with identifying patterns in a dataset and we let the model find patterns on its own,” Papalexakis said. “The tool was able to identify the same patterns that very closely correspond to the physically meaningful environmental states that are already known to human operators and commissioners at the LIGO sites.”
Papalexakis added that the team had worked with the LIGO Scientific Collaboration to secure the release of a very large dataset that pertains to the analysis reported in the research paper. This data release allows the research community to not only validate the team’s results but also develop new algorithms that seek to identify patterns in the data.
“We have identified a fascinating link between external environmental noise and the presence of certain types of glitches that corrupt the quality of the data,” Papalexakis said. “This discovery has the potential to help eliminate or prevent the occurrence of such noise.”
The team organized and worked through all the LIGO channels for about a year. Richardson noted that the data release was a major undertaking.
“Our team spearheaded this release on behalf of the whole LIGO Scientific Collaboration, which has about 3,200 members,” he said. “This is the first of these particular types of datasets and we think it’s going to have a large impact in the machine learning and the computer science community.”
Richardson explained that the tool the team developed can take information from signals from numerous heterogeneous sensors that are measuring different disturbances around the LIGO sites. The tool can distill the information into a single state, he said, that can then be used to search for time series associations of when noise problems occurred in the LIGO detectors and correlate them with the sites’ environmental states at those times.
“If you can identify the patterns, you can make physical changes to the detector — replace components, for example,” he said. “The hope is that our tool can shed light on physical noise coupling pathways that allow for actionable experimental changes to be made to the LIGO detectors. Our long-term goal is for this tool to be used to detect new associations and new forms of environmental states associated with unknown noise problems in the interferometers.”
Pooyan Goodarzi, a doctoral student working with Richardson and a coauthor on the paper, emphasized the importance of releasing the dataset publicly.
“Typically, such data tend to be proprietary,” he said. “We managed, nonetheless, to release a large-scale dataset that we hope results in more interdisciplinary research in data science and machine learning.”
The team’s research was supported by a grant from the National Science Foundation awarded through a special program, Advancing Discovery with AI-Powered Tools, focused on applying artificial intelligence/machine learning to address problems in the physical sciences.
5 notes
·
View notes
Text
Smart Switchgear in 2025: What Electrical Engineers Need to Know
In the fast-evolving world of electrical infrastructure, smart switchgear is no longer a futuristic concept — it’s the new standard. As we move through 2025, the integration of intelligent systems into traditional switchgear is redefining how engineers design, monitor, and maintain power distribution networks.
This shift is particularly crucial for electrical engineers, who are at the heart of innovation in sectors like manufacturing, utilities, data centers, commercial construction, and renewable energy.
In this article, we’ll break down what smart switchgear means in 2025, the technologies behind it, its benefits, and what every electrical engineer should keep in mind.
What is Smart Switchgear?
Smart switchgear refers to traditional switchgear (devices used for controlling, protecting, and isolating electrical equipment) enhanced with digital technologies, sensors, and communication modules that allow:
Real-time monitoring
Predictive maintenance
Remote operation and control
Data-driven diagnostics and performance analytics
This transformation is powered by IoT (Internet of Things), AI, cloud computing, and edge devices, which work together to improve reliability, safety, and efficiency in electrical networks.
Key Innovations in Smart Switchgear (2025 Edition)
1. IoT Integration
Smart switchgear is equipped with intelligent sensors that collect data on temperature, current, voltage, humidity, and insulation. These sensors communicate wirelessly with central systems to provide real-time status and alerts.
2. AI-Based Predictive Maintenance
Instead of traditional scheduled inspections, AI algorithms can now predict component failure based on usage trends and environmental data. This helps avoid downtime and reduces maintenance costs.
3. Cloud Connectivity
Cloud platforms allow engineers to remotely access switchgear data from any location. With user-friendly dashboards, they can visualize key metrics, monitor health conditions, and set thresholds for automated alerts.
4. Cybersecurity Enhancements
As devices get connected to networks, cybersecurity becomes crucial. In 2025, smart switchgear is embedded with secure communication protocols, access control layers, and encrypted data streams to prevent unauthorized access.
5. Digital Twin Technology
Some manufacturers now offer a digital twin of the switchgear — a virtual replica that updates in real-time. Engineers can simulate fault conditions, test load responses, and plan future expansions without touching the physical system.
Benefits for Electrical Engineers
1. Operational Efficiency
Smart switchgear reduces manual inspections and allows remote diagnostics, leading to faster response times and reduced human error.
2. Enhanced Safety
Early detection of overload, arc flash risks, or abnormal temperatures enhances on-site safety, especially in high-voltage environments.
3. Data-Driven Decisions
Real-time analytics help engineers understand load patterns and optimize distribution for efficiency and cost savings.
4. Seamless Scalability
Modular smart systems allow for quick expansion of power infrastructure, particularly useful in growing industrial or smart city projects.
Applications Across Industries
Manufacturing Plants — Monitor energy use per production line
Data Centers — Ensure uninterrupted uptime and cooling load balance
Commercial Buildings — Integrate with BMS (Building Management Systems)
Renewable Energy Projects — Balance grid load from solar or wind sources
Oil & Gas Facilities — Improve safety and compliance through monitoring
What Engineers Need to Know Moving Forward
1. Stay Updated with IEC & IEEE Standards
Smart switchgear must comply with global standards. Engineers need to be familiar with updates related to IEC 62271, IEC 61850, and IEEE C37 series.
2. Learn Communication Protocols
Proficiency in Modbus, DNP3, IEC 61850, and OPC UA is essential to integrating and troubleshooting intelligent systems.
3. Understand Lifecycle Costing
Smart switchgear might have a higher upfront cost but offers significant savings in maintenance, energy efficiency, and downtime over its lifespan.
4. Collaborate with IT Teams
The line between electrical and IT is blurring. Engineers should work closely with cybersecurity and cloud teams for seamless, secure integration.
Conclusion
Smart switchgear is reshaping the way electrical systems are built and managed in 2025. For electrical engineers, embracing this innovation isn’t just an option — it’s a career necessity.
At Blitz Bahrain, we specialize in providing cutting-edge switchgear solutions built for the smart, digital future. Whether you’re an engineer designing the next big project or a facility manager looking to upgrade existing systems, we’re here to power your progress.
#switchgear#panel#manufacturer#bahrain25#electrical supplies#electrical equipment#electrical engineers#electrical
5 notes
·
View notes
Text
Getting Started with Industrial Robotics Programming
Industrial robotics is a field where software engineering meets automation to drive manufacturing, assembly, and inspection processes. With the rise of Industry 4.0, the demand for skilled robotics programmers is rapidly increasing. This post introduces you to the fundamentals of industrial robotics programming and how you can get started in this exciting tech space.
What is Industrial Robotics Programming?
Industrial robotics programming involves creating software instructions for robots to perform tasks such as welding, picking and placing objects, painting, or quality inspection. These robots are typically used in factories and warehouses, and are often programmed using proprietary or standard languages tailored for automation tasks.
Popular Robotics Programming Languages
RAPID – Used for ABB robots.
KRL (KUKA Robot Language) – For KUKA industrial robots.
URScript – Used by Universal Robots.
Fanuc KAREL / Teach Pendant Programming
ROS (Robot Operating System) – Widely used open-source middleware for robotics.
Python and C++ – Common languages for simulation and integration with sensors and AI.
Key Components in Robotics Programming
Motion Control: Programming the path, speed, and precision of robot arms.
Sensor Integration: Use of cameras, force sensors, and proximity detectors for adaptive control.
PLC Communication: Integrating robots with Programmable Logic Controllers for factory automation.
Safety Protocols: Programming emergency stops, limit switches, and safe zones.
Human-Machine Interface (HMI): Designing interfaces for operators to control and monitor robots.
Sample URScript Code (Universal Robots)
# Move to position movej([1.0, -1.57, 1.57, -1.57, -1.57, 0.0], a=1.4, v=1.05) # Gripper control (example function call) set_digital_out(8, True) # Close gripper sleep(1) set_digital_out(8, False) # Open gripper
Software Tools You Can Use
RoboDK – Offline programming and simulation.
ROS + Gazebo – Open-source tools for simulation and robotic control.
ABB RobotStudio
Fanuc ROBOGUIDE
Siemens TIA Portal – For integration with industrial control systems.
Steps to Start Your Journey
Learn the basics of industrial robotics and automation.
Familiarize yourself with at least one brand of industrial robot (ABB, KUKA, UR, Fanuc).
Get comfortable with control systems and communication protocols (EtherCAT, PROFINET).
Practice with simulations before handling real robots.
Study safety standards (ISO 10218, ANSI/RIA R15.06).
Real-World Applications
Automated welding in car manufacturing.
High-speed pick and place in packaging.
Precision assembly of electronics.
Material handling and palletizing in warehouses.
Conclusion
Industrial robotics programming is a specialized yet rewarding field that bridges software with real-world mechanics. Whether you’re interested in working with physical robots or developing smart systems for factories, gaining skills in robotics programming can open up incredible career paths in manufacturing, automation, and AI-driven industries.
2 notes
·
View notes
Text
What is artificial intelligence (AI)?
Imagine asking Siri about the weather, receiving a personalized Netflix recommendation, or unlocking your phone with facial recognition. These everyday conveniences are powered by Artificial Intelligence (AI), a transformative technology reshaping our world. This post delves into AI, exploring its definition, history, mechanisms, applications, ethical dilemmas, and future potential.
What is Artificial Intelligence? Definition: AI refers to machines or software designed to mimic human intelligence, performing tasks like learning, problem-solving, and decision-making. Unlike basic automation, AI adapts and improves through experience.
Brief History:
1950: Alan Turing proposes the Turing Test, questioning if machines can think.
1956: The Dartmouth Conference coins the term "Artificial Intelligence," sparking early optimism.
1970s–80s: "AI winters" due to unmet expectations, followed by resurgence in the 2000s with advances in computing and data availability.
21st Century: Breakthroughs in machine learning and neural networks drive AI into mainstream use.
How Does AI Work? AI systems process vast data to identify patterns and make decisions. Key components include:
Machine Learning (ML): A subset where algorithms learn from data.
Supervised Learning: Uses labeled data (e.g., spam detection).
Unsupervised Learning: Finds patterns in unlabeled data (e.g., customer segmentation).
Reinforcement Learning: Learns via trial and error (e.g., AlphaGo).
Neural Networks & Deep Learning: Inspired by the human brain, these layered algorithms excel in tasks like image recognition.
Big Data & GPUs: Massive datasets and powerful processors enable training complex models.
Types of AI
Narrow AI: Specialized in one task (e.g., Alexa, chess engines).
General AI: Hypothetical, human-like adaptability (not yet realized).
Superintelligence: A speculative future AI surpassing human intellect.
Other Classifications:
Reactive Machines: Respond to inputs without memory (e.g., IBM’s Deep Blue).
Limited Memory: Uses past data (e.g., self-driving cars).
Theory of Mind: Understands emotions (in research).
Self-Aware: Conscious AI (purely theoretical).
Applications of AI
Healthcare: Diagnosing diseases via imaging, accelerating drug discovery.
Finance: Detecting fraud, algorithmic trading, and robo-advisors.
Retail: Personalized recommendations, inventory management.
Manufacturing: Predictive maintenance using IoT sensors.
Entertainment: AI-generated music, art, and deepfake technology.
Autonomous Systems: Self-driving cars (Tesla, Waymo), delivery drones.
Ethical Considerations
Bias & Fairness: Biased training data can lead to discriminatory outcomes (e.g., facial recognition errors in darker skin tones).
Privacy: Concerns over data collection by smart devices and surveillance systems.
Job Displacement: Automation risks certain roles but may create new industries.
Accountability: Determining liability for AI errors (e.g., autonomous vehicle accidents).
The Future of AI
Integration: Smarter personal assistants, seamless human-AI collaboration.
Advancements: Improved natural language processing (e.g., ChatGPT), climate change solutions (optimizing energy grids).
Regulation: Growing need for ethical guidelines and governance frameworks.
Conclusion AI holds immense potential to revolutionize industries, enhance efficiency, and solve global challenges. However, balancing innovation with ethical stewardship is crucial. By fostering responsible development, society can harness AI’s benefits while mitigating risks.
2 notes
·
View notes
Text
Lenovo Idea Tab Pro
The Lenovo Idea Tab Pro, unveiled in March 2025, is a versatile 12.7-inch tablet designed to cater to both students and everyday users. It combines robust performance with user-friendly features, making it a compelling choice in the tablet market.
Design and Display
The Idea Tab Pro boasts a sleek and lightweight design, measuring 291.8 x 189.1 x 6.9 mm and weighing approximately 620 grams. Its 12.7-inch IPS LCD screen offers a resolution of 2944 x 1840 pixels, delivering crisp and vibrant visuals. The display supports a 144Hz refresh rate and HDR10, enhancing the viewing experience with smoother transitions and richer colors. An optional anti-reflection coating is available to reduce glare, improving usability in various lighting conditions.
Performance
At its core, the Idea Tab Pro is powered by the MediaTek Dimensity 8300 chipset, featuring an octa-core CPU configuration: one Cortex-A715 core at 3.35 GHz, three Cortex-A715 cores at 3.2 GHz, and four Cortex-A510 cores at 2.2 GHz. This setup ensures efficient multitasking and smooth performance across applications. The tablet comes equipped with 8GB of LPDDR5X RAM and offers storage options of 128GB (UFS 3.1) or 256GB (UFS 4.0), providing ample space for apps, media, and documents.
Camera Capabilities
For photography and video calls, the Idea Tab Pro features a 13 MP rear camera with autofocus and LED flash, capable of recording 1080p videos. The front-facing 8 MP camera is suitable for selfies and virtual meetings, also supporting 1080p video recording.
Audio and Multimedia
Audio quality is a highlight, with the tablet housing four JBL stereo speakers that support 24-bit/192kHz Hi-Res audio. This setup ensures an immersive sound experience, whether you're watching movies, listening to music, or participating in video conferences.
Battery Life and Charging
The device is equipped with a substantial 10,200 mAh Li-Po battery, supporting 45W wired charging. This large battery capacity ensures extended usage, making it reliable for all-day activities without frequent recharging.
Operating System and AI Features
Running on Android 14, the Idea Tab Pro integrates advanced AI capabilities through Google Gemini and features like Circle to Search with Google. These tools enhance user interaction, providing intuitive and efficient ways to access information and perform tasks.
Connectivity and Additional Features
Connectivity options include Wi-Fi 802.11 a/b/g/n/ac/6e, Bluetooth 5.3, and USB Type-C 3.2 with DisplayPort support. The tablet also features a side-mounted fingerprint sensor integrated into the power button for secure and convenient access. Stylus support is available, catering to users interested in drawing or note-taking.
Pricing and Availability
The Lenovo Idea Tab Pro is available in various configurations:
8GB RAM with 128GB storage, including a pen, priced at
8GB RAM with 256GB storage, including a pen, priced at
12GB RAM with 256GB storage, without accessories, priced at
Additional bundles with accessories like a folio case are available at varying price points. Prospective buyers should verify the included accessories with retailers to ensure clarity.
Conclusion
The Lenovo Idea Tab Pro stands out as a well-rounded tablet, offering a blend of performance, display quality, and user-centric features. Its integration of AI capabilities and support for accessories like a stylus and keyboard pack make it a versatile tool for both educational and everyday use.
#Lenovo Idea Tab Pro#Lenovo Idea Tab Pro price#Lenovo Idea Tab Pro price in bamgladesh#Lenovo Idea Tab Pro bangladesh
3 notes
·
View notes
Text
The Automation Revolution: How Embedded Analytics is Leading the Way

Embedded analytics tools have emerged as game-changers, seamlessly integrating data-driven insights into business applications and enabling automation across various industries. By providing real-time analytics within existing workflows, these tools empower organizations to make informed decisions without switching between multiple platforms.
The Role of Embedded Analytics in Automation
Embedded analytics refers to the integration of analytical capabilities directly into business applications, eliminating the need for separate business intelligence (BI) tools. This integration enhances automation by:
Reducing Manual Data Analysis: Automated dashboards and real-time reporting eliminate the need for manual data extraction and processing.
Improving Decision-Making: AI-powered analytics provide predictive insights, helping businesses anticipate trends and make proactive decisions.
Enhancing Operational Efficiency: Automated alerts and anomaly detection streamline workflow management, reducing bottlenecks and inefficiencies.
Increasing User Accessibility: Non-technical users can easily access and interpret data within familiar applications, enabling data-driven culture across organizations.
Industry-Wide Impact of Embedded Analytics
1. Manufacturing: Predictive Maintenance & Process Optimization
By analyzing real-time sensor data, predictive maintenance reduces downtime, enhances production efficiency, and minimizes repair costs.
2. Healthcare: Enhancing Patient Outcomes & Resource Management
Healthcare providers use embedded analytics to track patient records, optimize treatment plans, and manage hospital resources effectively.
3. Retail: Personalized Customer Experiences & Inventory Optimization
Retailers integrate embedded analytics into e-commerce platforms to analyze customer preferences, optimize pricing, and manage inventory.
4. Finance: Fraud Detection & Risk Management
Financial institutions use embedded analytics to detect fraudulent activities, assess credit risks, and automate compliance monitoring.
5. Logistics: Supply Chain Optimization & Route Planning
Supply chain managers use embedded analytics to track shipments, optimize delivery routes, and manage inventory levels.
6. Education: Student Performance Analysis & Learning Personalization
Educational institutions utilize embedded analytics to track student performance, identify learning gaps, and personalize educational experiences.
The Future of Embedded Analytics in Automation
As AI and machine learning continue to evolve, embedded analytics will play an even greater role in automation. Future advancements may include:
Self-Service BI: Empowering users with more intuitive, AI-driven analytics tools that require minimal technical expertise.
Hyperautomation: Combining embedded analytics with robotic process automation (RPA) for end-to-end business process automation.
Advanced Predictive & Prescriptive Analytics: Leveraging AI for more accurate forecasting and decision-making support.
Greater Integration with IoT & Edge Computing: Enhancing real-time analytics capabilities for industries reliant on IoT sensors and connected devices.
Conclusion
By integrating analytics within existing workflows, businesses can improve efficiency, reduce operational costs, and enhance customer experiences. As technology continues to advance, the synergy between embedded analytics and automation will drive innovation and reshape the future of various industries.
To know more: data collection and insights
data analytics services
2 notes
·
View notes
Text
Satellite IoT Market Key Players Growth Strategies and Business Models to 2033
Introduction
The Satellite Internet of Things (IoT) market has been experiencing rapid growth in recent years, driven by increasing demand for global connectivity, advancements in satellite technology, and expanding IoT applications across various industries. As businesses and governments seek to leverage IoT for remote monitoring, asset tracking, and environmental sensing, satellite-based solutions have emerged as a crucial component of the global IoT ecosystem. This article explores the key trends, growth drivers, challenges, and future outlook of the satellite IoT market through 2032.
Market Overview
The satellite IoT market encompasses a range of services and solutions that enable IoT devices to communicate via satellite networks, bypassing terrestrial infrastructure constraints. This market is poised to grow significantly due to the increasing number of IoT devices, estimated to exceed 30 billion by 2030. The adoption of satellite IoT solutions is particularly prominent in industries such as agriculture, maritime, transportation, energy, and defense, where traditional connectivity options are limited.
Download a Free Sample Report:- https://tinyurl.com/5bx2u8ms
Key Market Drivers
Expanding IoT Applications
The proliferation of IoT devices across industries is fueling demand for satellite-based connectivity solutions. Sectors like agriculture, logistics, and environmental monitoring rely on satellite IoT for real-time data transmission from remote locations.
Advancements in Satellite Technology
The development of Low Earth Orbit (LEO) satellite constellations has significantly enhanced the capability and affordability of satellite IoT services. Companies like SpaceX (Starlink), OneWeb, and Amazon (Project Kuiper) are investing heavily in satellite networks to provide global coverage.
Rising Demand for Remote Connectivity
As industries expand operations into remote and rural areas, the need for uninterrupted IoT connectivity has increased. Satellite IoT solutions offer reliable alternatives to terrestrial networks, ensuring seamless data transmission.
Regulatory Support and Investments
Governments and space agencies worldwide are promoting satellite IoT initiatives through funding, policy frameworks, and public-private partnerships, further driving market growth.
Growing Need for Asset Tracking and Monitoring
Sectors such as logistics, oil and gas, and maritime heavily rely on satellite IoT for real-time asset tracking, predictive maintenance, and operational efficiency.
Market Challenges
High Initial Costs and Maintenance
Deploying and maintaining satellite IoT infrastructure involves significant investment, which may hinder adoption among small and medium enterprises.
Limited Bandwidth and Latency Issues
Despite advancements, satellite networks still face challenges related to bandwidth limitations and latency, which can impact real-time data transmission.
Cybersecurity Concerns
With the increasing number of connected devices, the risk of cyber threats and data breaches is a major concern for satellite IoT operators.
Industry Trends
Emergence of Hybrid Connectivity Solutions
Companies are integrating satellite IoT with terrestrial networks, including 5G and LPWAN, to provide seamless and cost-effective connectivity solutions.
Miniaturization of Satellites
The trend toward smaller, cost-efficient satellites (e.g., CubeSats) is making satellite IoT services more accessible and scalable.
AI and Edge Computing Integration
Artificial intelligence (AI) and edge computing are being incorporated into satellite IoT systems to enhance data processing capabilities, reduce latency, and improve decision-making.
Proliferation of Low-Cost Satellite IoT Devices
With declining costs of satellite IoT modules and sensors, adoption rates are increasing across industries.
Sustainable Space Practices
Efforts to minimize space debris and implement eco-friendly satellite technology are gaining traction, influencing the future of satellite IoT deployments.
Market Segmentation
By Service Type
Satellite Connectivity Services
Satellite IoT Platforms
Data Analytics & Management
By End-User Industry
Agriculture
Transportation & Logistics
Energy & Utilities
Maritime
Defense & Government
Healthcare
By Geography
North America
Europe
Asia-Pacific
Latin America
Middle East & Africa
Future Outlook (2024-2032)
The satellite IoT market is expected to grow at a compound annual growth rate (CAGR) of over 20% from 2024 to 2032. Key developments anticipated in the market include:
Expansion of LEO satellite constellations for enhanced global coverage.
Increased investment in space-based IoT startups and innovation hubs.
Strategic collaborations between telecom providers and satellite operators.
Adoption of AI-driven analytics for predictive monitoring and automation.
Conclusion
The satellite IoT market is on a trajectory of substantial growth, driven by technological advancements, increasing demand for remote connectivity, and expanding industrial applications. While challenges such as cost and security remain, innovations in satellite design, AI integration, and hybrid network solutions are expected to propel the industry forward. As we move toward 2032, satellite IoT will play an increasingly vital role in shaping the future of global connectivity and digital transformation across various sectors.Read Full Report:-https://www.uniprismmarketresearch.com/verticals/information-communication-technology/satellite-iot.html
2 notes
·
View notes
Text
What Is Generative Physical AI? Why It Is Important?
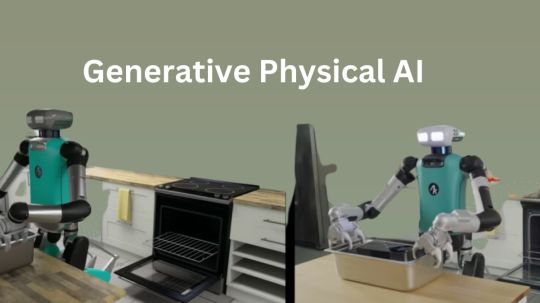
What is Physical AI?
Autonomous robots can see, comprehend, and carry out intricate tasks in the actual (physical) environment with to physical artificial intelligence. Because of its capacity to produce ideas and actions to carry out, it is also sometimes referred to as “Generative physical AI.”
How Does Physical AI Work?
Models of generative AI Massive volumes of text and picture data, mostly from the Internet, are used to train huge language models like GPT and Llama. Although these AIs are very good at creating human language and abstract ideas, their understanding of the physical world and its laws is still somewhat restricted.
Current generative AI is expanded by Generative physical AI, which comprehends the spatial linkages and physical behavior of the three-dimensional environment in which the all inhabit. During the AI training process, this is accomplished by supplying extra data that includes details about the spatial connections and physical laws of the actual world.
Highly realistic computer simulations are used to create the 3D training data, which doubles as an AI training ground and data source.
A digital doppelganger of a location, such a factory, is the first step in physically-based data creation. Sensors and self-governing devices, such as robots, are introduced into this virtual environment. The sensors record different interactions, such as rigid body dynamics like movement and collisions or how light interacts in an environment, and simulations that replicate real-world situations are run.
What Function Does Reinforcement Learning Serve in Physical AI?
Reinforcement learning trains autonomous robots to perform in the real world by teaching them skills in a simulated environment. Through hundreds or even millions of trial-and-error, it enables self-governing robots to acquire abilities in a safe and efficient manner.
By rewarding a physical AI model for doing desirable activities in the simulation, this learning approach helps the model continually adapt and become better. Autonomous robots gradually learn to respond correctly to novel circumstances and unanticipated obstacles via repeated reinforcement learning, readying them for real-world operations.
An autonomous machine may eventually acquire complex fine motor abilities required for practical tasks like packing boxes neatly, assisting in the construction of automobiles, or independently navigating settings.
Why is Physical AI Important?
Autonomous robots used to be unable to detect and comprehend their surroundings. However, Generative physical AI enables the construction and training of robots that can naturally interact with and adapt to their real-world environment.
Teams require strong, physics-based simulations that provide a secure, regulated setting for training autonomous machines in order to develop physical AI. This improves accessibility and utility in real-world applications by facilitating more natural interactions between people and machines, in addition to increasing the efficiency and accuracy of robots in carrying out complicated tasks.
Every business will undergo a transformation as Generative physical AI opens up new possibilities. For instance:
Robots: With physical AI, robots show notable improvements in their operating skills in a range of environments.
Using direct input from onboard sensors, autonomous mobile robots (AMRs) in warehouses are able to traverse complicated settings and avoid impediments, including people.
Depending on how an item is positioned on a conveyor belt, manipulators may modify their grabbing position and strength, demonstrating both fine and gross motor abilities according to the object type.
This method helps surgical robots learn complex activities like stitching and threading needles, demonstrating the accuracy and versatility of Generative physical AI in teaching robots for particular tasks.
Autonomous Vehicles (AVs): AVs can make wise judgments in a variety of settings, from wide highways to metropolitan cityscapes, by using sensors to sense and comprehend their environment. By exposing AVs to physical AI, they may better identify people, react to traffic or weather, and change lanes on their own, efficiently adjusting to a variety of unforeseen situations.
Smart Spaces: Large interior areas like factories and warehouses, where everyday operations include a constant flow of people, cars, and robots, are becoming safer and more functional with to physical artificial intelligence. By monitoring several things and actions inside these areas, teams may improve dynamic route planning and maximize operational efficiency with the use of fixed cameras and sophisticated computer vision models. Additionally, they effectively see and comprehend large-scale, complicated settings, putting human safety first.
How Can You Get Started With Physical AI?
Using Generative physical AI to create the next generation of autonomous devices requires a coordinated effort from many specialized computers:
Construct a virtual 3D environment: A high-fidelity, physically based virtual environment is needed to reflect the actual world and provide synthetic data essential for training physical AI. In order to create these 3D worlds, developers can simply include RTX rendering and Universal Scene Description (OpenUSD) into their current software tools and simulation processes using the NVIDIA Omniverse platform of APIs, SDKs, and services.
NVIDIA OVX systems support this environment: Large-scale sceneries or data that are required for simulation or model training are also captured in this stage. fVDB, an extension of PyTorch that enables deep learning operations on large-scale 3D data, is a significant technical advancement that has made it possible for effective AI model training and inference with rich 3D datasets. It effectively represents features.
Create synthetic data: Custom synthetic data generation (SDG) pipelines may be constructed using the Omniverse Replicator SDK. Domain randomization is one of Replicator’s built-in features that lets you change a lot of the physical aspects of a 3D simulation, including lighting, position, size, texture, materials, and much more. The resulting pictures may also be further enhanced by using diffusion models with ControlNet.
Train and validate: In addition to pretrained computer vision models available on NVIDIA NGC, the NVIDIA DGX platform, a fully integrated hardware and software AI platform, may be utilized with physically based data to train or fine-tune AI models using frameworks like TensorFlow, PyTorch, or NVIDIA TAO. After training, reference apps such as NVIDIA Isaac Sim may be used to test the model and its software stack in simulation. Additionally, developers may use open-source frameworks like Isaac Lab to use reinforcement learning to improve the robot’s abilities.
In order to power a physical autonomous machine, such a humanoid robot or industrial automation system, the optimized stack may now be installed on the NVIDIA Jetson Orin and, eventually, the next-generation Jetson Thor robotics supercomputer.
Read more on govindhtech.com
#GenerativePhysicalAI#generativeAI#languagemodels#PyTorch#NVIDIAOmniverse#AImodel#artificialintelligence#NVIDIADGX#TensorFlow#AI#technology#technews#news#govindhtech
3 notes
·
View notes
Text
How-To IT
Topic: Core areas of IT
1. Hardware
• Computers (Desktops, Laptops, Workstations)
• Servers and Data Centers
• Networking Devices (Routers, Switches, Modems)
• Storage Devices (HDDs, SSDs, NAS)
• Peripheral Devices (Printers, Scanners, Monitors)
2. Software
• Operating Systems (Windows, Linux, macOS)
• Application Software (Office Suites, ERP, CRM)
• Development Software (IDEs, Code Libraries, APIs)
• Middleware (Integration Tools)
• Security Software (Antivirus, Firewalls, SIEM)
3. Networking and Telecommunications
• LAN/WAN Infrastructure
• Wireless Networking (Wi-Fi, 5G)
• VPNs (Virtual Private Networks)
• Communication Systems (VoIP, Email Servers)
• Internet Services
4. Data Management
• Databases (SQL, NoSQL)
• Data Warehousing
• Big Data Technologies (Hadoop, Spark)
• Backup and Recovery Systems
• Data Integration Tools
5. Cybersecurity
• Network Security
• Endpoint Protection
• Identity and Access Management (IAM)
• Threat Detection and Incident Response
• Encryption and Data Privacy
6. Software Development
• Front-End Development (UI/UX Design)
• Back-End Development
• DevOps and CI/CD Pipelines
• Mobile App Development
• Cloud-Native Development
7. Cloud Computing
• Infrastructure as a Service (IaaS)
• Platform as a Service (PaaS)
• Software as a Service (SaaS)
• Serverless Computing
• Cloud Storage and Management
8. IT Support and Services
• Help Desk Support
• IT Service Management (ITSM)
• System Administration
• Hardware and Software Troubleshooting
• End-User Training
9. Artificial Intelligence and Machine Learning
• AI Algorithms and Frameworks
• Natural Language Processing (NLP)
• Computer Vision
• Robotics
• Predictive Analytics
10. Business Intelligence and Analytics
• Reporting Tools (Tableau, Power BI)
• Data Visualization
• Business Analytics Platforms
• Predictive Modeling
11. Internet of Things (IoT)
• IoT Devices and Sensors
• IoT Platforms
• Edge Computing
• Smart Systems (Homes, Cities, Vehicles)
12. Enterprise Systems
• Enterprise Resource Planning (ERP)
• Customer Relationship Management (CRM)
• Human Resource Management Systems (HRMS)
• Supply Chain Management Systems
13. IT Governance and Compliance
• ITIL (Information Technology Infrastructure Library)
• COBIT (Control Objectives for Information Technologies)
• ISO/IEC Standards
• Regulatory Compliance (GDPR, HIPAA, SOX)
14. Emerging Technologies
• Blockchain
• Quantum Computing
• Augmented Reality (AR) and Virtual Reality (VR)
• 3D Printing
• Digital Twins
15. IT Project Management
• Agile, Scrum, and Kanban
• Waterfall Methodology
• Resource Allocation
• Risk Management
16. IT Infrastructure
• Data Centers
• Virtualization (VMware, Hyper-V)
• Disaster Recovery Planning
• Load Balancing
17. IT Education and Certifications
• Vendor Certifications (Microsoft, Cisco, AWS)
• Training and Development Programs
• Online Learning Platforms
18. IT Operations and Monitoring
• Performance Monitoring (APM, Network Monitoring)
• IT Asset Management
• Event and Incident Management
19. Software Testing
• Manual Testing: Human testers evaluate software by executing test cases without using automation tools.
• Automated Testing: Use of testing tools (e.g., Selenium, JUnit) to run automated scripts and check software behavior.
• Functional Testing: Validating that the software performs its intended functions.
• Non-Functional Testing: Assessing non-functional aspects such as performance, usability, and security.
��� Unit Testing: Testing individual components or units of code for correctness.
• Integration Testing: Ensuring that different modules or systems work together as expected.
• System Testing: Verifying the complete software system’s behavior against requirements.
• Acceptance Testing: Conducting tests to confirm that the software meets business requirements (including UAT - User Acceptance Testing).
• Regression Testing: Ensuring that new changes or features do not negatively affect existing functionalities.
• Performance Testing: Testing software performance under various conditions (load, stress, scalability).
• Security Testing: Identifying vulnerabilities and assessing the software’s ability to protect data.
• Compatibility Testing: Ensuring the software works on different operating systems, browsers, or devices.
• Continuous Testing: Integrating testing into the development lifecycle to provide quick feedback and minimize bugs.
• Test Automation Frameworks: Tools and structures used to automate testing processes (e.g., TestNG, Appium).
19. VoIP (Voice over IP)
VoIP Protocols & Standards
• SIP (Session Initiation Protocol)
• H.323
• RTP (Real-Time Transport Protocol)
• MGCP (Media Gateway Control Protocol)
VoIP Hardware
• IP Phones (Desk Phones, Mobile Clients)
• VoIP Gateways
• Analog Telephone Adapters (ATAs)
• VoIP Servers
• Network Switches/ Routers for VoIP
VoIP Software
• Softphones (e.g., Zoiper, X-Lite)
• PBX (Private Branch Exchange) Systems
• VoIP Management Software
• Call Center Solutions (e.g., Asterisk, 3CX)
VoIP Network Infrastructure
• Quality of Service (QoS) Configuration
• VPNs (Virtual Private Networks) for VoIP
• VoIP Traffic Shaping & Bandwidth Management
• Firewall and Security Configurations for VoIP
• Network Monitoring & Optimization Tools
VoIP Security
• Encryption (SRTP, TLS)
• Authentication and Authorization
• Firewall & Intrusion Detection Systems
• VoIP Fraud DetectionVoIP Providers
• Hosted VoIP Services (e.g., RingCentral, Vonage)
• SIP Trunking Providers
• PBX Hosting & Managed Services
VoIP Quality and Testing
• Call Quality Monitoring
• Latency, Jitter, and Packet Loss Testing
• VoIP Performance Metrics and Reporting Tools
• User Acceptance Testing (UAT) for VoIP Systems
Integration with Other Systems
• CRM Integration (e.g., Salesforce with VoIP)
• Unified Communications (UC) Solutions
• Contact Center Integration
• Email, Chat, and Video Communication Integration
2 notes
·
View notes
Text
The Role of Photon Insights in Helps In Academic Research
In recent times, the integration of Artificial Intelligence (AI) with academic study has been gaining significant momentum that offers transformative opportunities across different areas. One area in which AI has a significant impact is in the field of photonics, the science of producing as well as manipulating and sensing photos that can be used in medical, telecommunications, and materials sciences. It also reveals its ability to enhance the analysis of data, encourage collaboration, and propel the development of new technologies.
Understanding the Landscape of Photonics
Photonics covers a broad range of technologies, ranging from fibre optics and lasers to sensors and imaging systems. As research in this field gets more complicated and complex, the need for sophisticated analytical tools becomes essential. The traditional methods of data processing and interpretation could be slow and inefficient and often slow the pace of discovery. This is where AI is emerging as a game changer with robust solutions that improve research processes and reveal new knowledge.
Researchers can, for instance, use deep learning methods to enhance image processing in applications such as biomedical imaging. AI-driven algorithms can improve the image’s resolution, cut down on noise, and even automate feature extraction, which leads to more precise diagnosis. Through automation of this process, experts are able to concentrate on understanding results, instead of getting caught up with managing data.
Accelerating Material Discovery
Research in the field of photonics often involves investigation of new materials, like photonic crystals, or metamaterials that can drastically alter the propagation of light. Methods of discovery for materials are time-consuming and laborious and often require extensive experiments and testing. AI can speed up the process through the use of predictive models and simulations.
Facilitating Collaboration
In a time when interdisciplinary collaboration is vital, AI tools are bridging the gap between researchers from various disciplines. The research conducted in the field of photonics typically connects with fields like engineering, computer science, and biology. AI-powered platforms aid in this collaboration by providing central databases and sharing information, making it easier for researchers to gain access to relevant data and tools.
Cloud-based AI solutions are able to provide shared datasets, which allows researchers to collaborate with no limitations of geographic limitations. Collaboration is essential in photonics, where the combination of diverse knowledge can result in revolutionary advances in technology and its applications.
Automating Experimental Procedures
Automation is a third area in which AI is becoming a major factor in the field of academic research in the field of photonics. The automated labs equipped with AI-driven technology can carry out experiments with no human involvement. The systems can alter parameters continuously based on feedback, adjusting conditions for experiments to produce the highest quality outcomes.
Furthermore, robotic systems that are integrated with AI can perform routine tasks like sampling preparation and measurement. This is not just more efficient but also decreases errors made by humans, which results in more accurate results. Through automation researchers can devote greater time for analysis as well as development which will speed up the overall research process.
Predictive Analytics for Research Trends
The predictive capabilities of AI are crucial for analyzing and predicting research trends in the field of photonics. By studying the literature that is already in use as well as research outputs, AI algorithms can pinpoint new themes and areas of research. This insight can assist researchers to prioritize their work and identify emerging trends that could be destined to be highly impactful.
For organizations and funding bodies These insights are essential to allocate resources as well as strategic plans. If they can understand where research is heading, they are able to help support research projects that are in line with future requirements, ultimately leading to improvements that benefit the entire society.
Ethical Considerations and Challenges
While the advantages of AI in speeding up research in photonics are evident however, ethical considerations need to be taken into consideration. Questions like privacy of data and bias in algorithmic computation, as well as the possibility of misuse by AI technology warrant careful consideration. Institutions and researchers must adopt responsible AI practices to ensure that the applications they use enhance human decision-making and not substitute it.
In addition, the incorporation in the use of AI into academic studies calls for the level of digital literacy which not every researcher are able to attain. Therefore, investing in education and education about AI methods and tools is vital to reap the maximum potential advantages.
Conclusion
The significance of AI in speeding up research at universities, especially in the field of photonics, is extensive and multifaceted. Through improving data analysis and speeding up the discovery of materials, encouraging collaboration, facilitating experimental procedures and providing insights that are predictive, AI is reshaping the research landscape. As the area of photonics continues to grow, the integration of AI technologies is certain to be a key factor in fostering innovation and expanding our knowledge of applications based on light.
Through embracing these developments scientists can open up new possibilities for research, which ultimately lead to significant scientific and technological advancements. As we move forward on this new frontier, interaction with AI as well as academic researchers will prove essential to address the challenges and opportunities ahead. The synergy between these two disciplines will not only speed up discovery in photonics, but also has the potential to change our understanding of and interaction with the world that surrounds us.
2 notes
·
View notes