#Benefits of AI and ML
Explore tagged Tumblr posts
Text
In today’s digital age, the buzz around Artificial Intelligence (AI) and Machine Learning (ML) is palpable, with businesses across industries.
In today’s digital age, the buzz around Artificial Intelligence (AI) and Machine Learning (ML) is palpable, with businesses across industries increasingly leveraging these technologies to drive innovation and gain competitive advantages.
#ai and ml development#ai development#ml development#ai development company#ROI in AI and ML#Benefits of AI and ML
0 notes
Text
I'm just saying maybe you shouldn't talk about the Luddites if you actually refuse to engage with their historical struggle and the reasons for it and instead just perpetuate the bourgeois propaganda about them and somehow act that's the materialist take. get fucking serious please.
#swear MLs on here really do love to be contrarian instead of having actual material analysis#like I'm not saying every anti-AI take is a coming from a rational and sober class analysis#but being against an anti-ai sentiment because there is a popular swell of it and so it must be stupid#and then defending that stance as politically justified in part by denouncing people as luddites#and then when ppl tell you about *the actual class character of the luddite movement* and why it's relevant to a marxist tech crit today#and how the modern definition is a bourgeois corruption to poison the well against a genuine threat to rising industrial capitalism#... your response is 'well that's how people understand it today. luddism is a step away from anti-civ reactionaries'#WHO IS REJECTING A HISTORICAL MATERIALIST ANALYSIS HERE?#sipping on the idealism of bourgeois propaganda against actual working class revolt and calling that a materialist political program?#grow up.#meanwhile WHO ARE YOU BENEFITING?#what infrastructure consolidation are you defending???#what energy grid privatisation and calcification are you cheering? do you think that's actually going to be good for us??? ever????#fucking unserious ass people - some technology is a systematic harm!#some technology was made by capitalists for capitalist ends! and will never benefit the working class bc it was created specifically not to#you have to be able to use your big brained material analysis to understand the class character of technology!#otherwise what even is the fucking point of you#sometimes it's not something that would be good just bc the workers are running it!#GROW UP
6 notes
·
View notes
Text
Boost E-commerce in Saudi Arabia with ML-Powered Apps
In today's digital era, the e-commerce industry in Saudi Arabia is rapidly expanding, fueled by increasing internet penetration and a tech-savvy population. To stay competitive, businesses are turning to advanced technologies, particularly Machine Learning (ML), to enhance user experiences, optimize operations, and drive growth. This article explores how ML is transforming the e-commerce landscape in Saudi Arabia and how businesses can leverage this technology to boost their success.
The Current E-commerce Landscape in Saudi Arabia
The e-commerce market in Saudi Arabia has seen exponential growth over the past few years. With a young population, widespread smartphone usage, and supportive government policies, the Kingdom is poised to become a leading e-commerce hub in the Middle East. Key players like Noon, Souq, and Jarir have set the stage, but the market is ripe for innovation, especially with the integration of Machine Learning.
The Role of Machine Learning in E-commerce
Machine Learning, a subset of Artificial Intelligence (AI), involves the use of algorithms to analyze data, learn from it, and make informed decisions. In e-commerce, ML enhances various aspects, from personalization to fraud detection. Machine Learning’s ability to analyze large datasets and identify trends is crucial for businesses aiming to stay ahead in a competitive market.
Personalized Shopping Experiences
Personalization is crucial in today’s e-commerce environment. ML algorithms analyze user data, such as browsing history and purchase behavior, to recommend products that align with individual preferences. This not only elevates the customer experience but also drives higher conversion rates. For example, platforms that leverage ML for personalization have seen significant boosts in sales, as users are more likely to purchase items that resonate with their interests.
Optimizing Inventory Management
Effective inventory management is critical for e-commerce success. ML-driven predictive analytics can forecast demand with high accuracy, helping businesses maintain optimal inventory levels. This minimizes the chances of overstocking or running out of products, ensuring timely availability for customers. E-commerce giants like Amazon have successfully implemented ML to streamline their inventory management processes, setting a benchmark for others to follow.
Dynamic Pricing Strategies
Price is a major factor influencing consumer decisions. Machine Learning enables real-time dynamic pricing by assessing market trends, competitor rates, and customer demand. This allows businesses to adjust their prices to maximize revenue while remaining competitive. Dynamic pricing, powered by ML, has proven effective in attracting price-sensitive customers and increasing overall profitability.
Enhanced Customer Support
Customer support is another area where ML shines. AI-powered chatbots and virtual assistants can handle a large volume of customer inquiries, providing instant responses and resolving issues efficiently. This not only improves customer satisfaction but also reduces the operational costs associated with maintaining a large support team. E-commerce businesses in Saudi Arabia can greatly benefit from incorporating ML into their customer service strategies.
Fraud Detection and Security
With the rise of online transactions, ensuring the security of customer data and payments is paramount. ML algorithms can detect fraudulent activities by analyzing transaction patterns and identifying anomalies. By implementing ML-driven security measures, e-commerce businesses can protect their customers and build trust, which is essential for long-term success.
Improving Marketing Campaigns
Effective marketing is key to driving e-commerce success. ML can analyze customer data to create targeted marketing campaigns that resonate with specific audiences. It enhances the impact of marketing efforts, leading to improved customer engagement and higher conversion rates. Successful e-commerce platforms use ML to fine-tune their marketing strategies, ensuring that their messages reach the right people at the right time.
Case Study: Successful E-commerce Companies in Saudi Arabia Using ML
Several e-commerce companies in Saudi Arabia have already begun leveraging ML to drive growth. For example, Noon uses ML to personalize the shopping experience and optimize its supply chain, leading to increased customer satisfaction and operational efficiency. These companies serve as examples of how ML can be a game-changer in the competitive e-commerce market.
Challenges of Implementing Machine Learning in E-commerce
While the benefits of ML are clear, implementing this technology in e-commerce is not without challenges. Technical hurdles, such as integrating ML with existing systems, can be daunting. Additionally, there are concerns about data privacy, particularly in handling sensitive customer information. Businesses must address these challenges to fully harness the power of ML.
Future Trends in Machine Learning and E-commerce
As ML continues to evolve, new trends are emerging that will shape the future of e-commerce. For instance, the integration of ML with augmented reality (AR) offers exciting possibilities, such as virtual try-ons for products. Businesses that stay ahead of these trends will be well-positioned to lead the market in the coming years.
Influence of Machine Learning on Consumer Behavior in Saudi Arabia
ML is already influencing consumer behavior in Saudi Arabia, with personalized experiences leading to increased customer loyalty. As more businesses adopt ML, consumers can expect even more tailored shopping experiences, further enhancing their satisfaction and engagement.
Government Support and Regulations
The Saudi government is proactively encouraging the integration of cutting-edge technologies, including ML, within the e-commerce industry. Through initiatives like Vision 2030, the government aims to transform the Kingdom into a global tech hub. However, businesses must also navigate regulations related to data privacy and AI to ensure compliance.
Conclusion
Machine Learning is revolutionizing e-commerce in Saudi Arabia, offering businesses new ways to enhance user experiences, optimize operations, and drive growth. By embracing ML, e-commerce companies can not only stay competitive but also set new standards in the industry. The future of e-commerce in Saudi Arabia is bright, and Machine Learning will undoubtedly play a pivotal role in shaping its success.
FAQs
How does Machine Learning contribute to the e-commerce sector? Machine Learning enhances e-commerce by improving personalization, optimizing inventory, enabling dynamic pricing, and enhancing security.
How can Machine Learning improve customer experiences in e-commerce? ML analyzes user data to provide personalized recommendations, faster customer support, and tailored marketing campaigns, improving overall satisfaction.
What are the challenges of integrating ML in e-commerce? Challenges include technical integration, data privacy concerns, and the need for skilled professionals to manage ML systems effectively.
Which Saudi e-commerce companies are successfully using ML? Companies like Noon and Souq are leveraging ML for personalized shopping experiences, inventory management, and customer support.
What is the future of e-commerce with ML in Saudi Arabia? The future looks promising with trends like ML-driven AR experiences and more personalized
#machine learning e-commerce#Saudi Arabia tech#ML-powered apps#e-commerce growth#AI in retail#customer experience Saudi Arabia#digital transformation Saudi#ML app benefits#AI-driven marketing#predictive analytics retail#Saudi digital economy#e-commerce innovation#smart retail solutions#AI tech adoption#machine learning in business
2 notes
·
View notes
Text
Why AI and ML Are the Future of Scalable MLOps Workflows?

In today’s fast-paced world of machine learning, speed and accuracy are paramount. But how can businesses ensure that their ML models are continuously improving, deployed efficiently, and constantly monitored for peak performance? Enter MLOps—a game-changing approach that combines the best of machine learning and operations to streamline the entire lifecycle of AI models. And now, with the infusion of AI and ML into MLOps itself, the possibilities are growing even more exciting.
Imagine a world where model deployment isn’t just automated but intelligently optimized, where model monitoring happens in real-time without human intervention, and where continuous learning is baked into every step of the process. This isn’t a far-off vision—it’s the future of MLOps, and AI/ML is at its heart. Let’s dive into how these powerful technologies are transforming MLOps and taking machine learning to the next level.
What is MLOps?
MLOps (Machine Learning Operations) combines machine learning and operations to streamline the end-to-end lifecycle of ML models. It ensures faster deployment, continuous improvement, and efficient management of models in production. MLOps is crucial for automating tasks, reducing manual intervention, and maintaining model performance over time.
Key Components of MLOps
Continuous Integration/Continuous Deployment (CI/CD): Automates testing, integration, and deployment of models, ensuring faster updates and minimal manual effort.
Model Versioning: Tracks different model versions for easy comparison, rollback, and collaboration.
Model Testing: Validates models against real-world data to ensure performance, accuracy, and reliability through automated tests.
Monitoring and Management: Continuously tracks model performance to detect issues like drift, ensuring timely updates and interventions.
Differences Between Traditional Software DevOps and MLOps
Focus: DevOps handles software code deployment, while MLOps focuses on managing evolving ML models.
Data Dependency: MLOps requires constant data handling and preprocessing, unlike DevOps, which primarily deals with software code.
Monitoring: MLOps monitors model behavior over time, while DevOps focuses on application performance.
Continuous Training: MLOps involves frequent model retraining, unlike traditional DevOps, which deploys software updates less often.
AI/ML in MLOps: A Powerful Partnership
As machine learning continues to evolve, AI and ML technologies are playing an increasingly vital role in enhancing MLOps workflows. Together, they bring intelligence, automation, and adaptability to the model lifecycle, making operations smarter, faster, and more efficient.
Enhancing MLOps with AI and ML: By embedding AI/ML capabilities into MLOps, teams can automate critical yet time-consuming tasks, reduce manual errors, and ensure models remain high-performing in production. These technologies don’t just support MLOps—they supercharge it.
Automating Repetitive Tasks: Machine learning algorithms are now used to handle tasks that once required extensive manual effort, such as:
Data Preprocessing: Automatically cleaning, transforming, and validating data.
Feature Engineering: Identifying the most relevant features for a model based on data patterns.
Model Selection and Hyperparameter Tuning: Using AutoML to test multiple algorithms and configurations, selecting the best-performing combination with minimal human input.
This level of automation accelerates model development and ensures consistent, scalable results.
Intelligent Monitoring and Self-Healing: AI also plays a key role in model monitoring and maintenance:
Predictive Monitoring: AI can detect early signs of model drift, performance degradation, or data anomalies before they impact business outcomes.
Self-Healing Systems: Advanced systems can trigger automatic retraining or rollback actions when issues are detected, keeping models accurate and reliable without waiting for manual intervention.
Key Applications of AI/ML in MLOps
AI and machine learning aren’t just being managed by MLOps—they’re actively enhancing it. From training models to scaling systems, AI/ML technologies are being used to automate, optimize, and future-proof the entire machine learning pipeline. Here are some of the key applications:
1. Automated Model Training and Tuning: Traditionally, choosing the right algorithm and tuning hyperparameters required expert knowledge and extensive trial and error. With AI/ML-powered tools like AutoML, this process is now largely automated. These tools can:
Test multiple models simultaneously
Optimize hyperparameters
Select the best-performing configuration
This not only speeds up experimentation but also improves model performance with less manual intervention.
2. Continuous Integration and Deployment (CI/CD): AI streamlines CI/CD pipelines by automating critical tasks in the deployment process. It can:
Validate data consistency and schema changes
Automatically test and promote new models
Reduce deployment risks through anomaly detection
By using AI, teams can achieve faster, safer, and more consistent model deployments at scale.
3. Model Monitoring and Management: Once a model is live, its job isn’t done—constant monitoring is essential. AI systems help by:
Detecting performance drift, data shifts, or anomalies
Sending alerts or triggering automated retraining when issues arise
Ensuring models remain accurate and reliable over time
This proactive approach keeps models aligned with real-world conditions, even as data changes.
4. Scaling and Performance Optimization: As ML workloads grow, resource management becomes critical. AI helps optimize performance by:
Dynamically allocating compute resources based on demand
Predicting system load and scaling infrastructure accordingly
Identifying bottlenecks and inefficiencies in real-time
These optimizations lead to cost savings and ensure high availability in large-scale ML deployments.
Benefits of Integrating AI/ML in MLOps
Bringing AI and ML into MLOps doesn’t just refine processes—it transforms them. By embedding intelligence and automation into every stage of the ML lifecycle, organizations can unlock significant operational and strategic advantages. Here are the key benefits:
1. Increased Efficiency and Faster Deployment Cycles: AI-driven automation accelerates everything from data preprocessing to model deployment. With fewer manual steps and smarter workflows, teams can build, test, and deploy models much faster, cutting down time-to-market and allowing quicker experimentation.
2. Enhanced Accuracy in Predictive Models: With ML algorithms optimizing model selection and tuning, the chances of deploying high-performing models increase. AI also ensures that models are continuously evaluated and updated, improving decision-making with more accurate, real-time predictions.
3. Reduced Human Intervention and Manual Errors: Automating repetitive tasks minimizes the risk of human errors, streamlines collaboration, and frees up data scientists and engineers to focus on higher-level strategy and innovation. This leads to more consistent outcomes and reduced operational overhead.
4. Continuous Improvement Through Feedback Loops: AI-powered MLOps systems enable continuous learning. By monitoring model performance and feeding insights back into training pipelines, the system evolves automatically, adjusting to new data and changing environments without manual retraining.
Integrating AI/ML into MLOps doesn’t just make operations smarter—it builds a foundation for scalable, self-improving systems that can keep pace with the demands of modern machine learning.
Future of AI/ML in MLOps
The future of MLOps is poised to become even more intelligent and autonomous, thanks to rapid advancements in AI and ML technologies. Trends like AutoML, reinforcement learning, and explainable AI (XAI) are already reshaping how machine learning workflows are built and managed. AutoML is streamlining the entire modeling process—from data preprocessing to model deployment—making it more accessible and efficient. Reinforcement learning is being explored for dynamic resource optimization and decision-making within pipelines, while explainable AI is becoming essential to ensure transparency, fairness, and trust in automated systems.
Looking ahead, AI/ML will drive the development of fully autonomous machine learning pipelines—systems capable of learning from performance metrics, retraining themselves, and adapting to new data with minimal human input. These self-sustaining workflows will not only improve speed and scalability but also ensure long-term model reliability in real-world environments. As organizations increasingly rely on AI for critical decisions, MLOps will evolve into a more strategic, intelligent framework—one that blends automation, adaptability, and accountability to meet the growing demands of AI-driven enterprises.
As AI and ML continue to evolve, their integration into MLOps is proving to be a game-changer, enabling smarter automation, faster deployments, and more resilient model management. From streamlining repetitive tasks to powering predictive monitoring and self-healing systems, AI/ML is transforming MLOps into a dynamic, intelligent backbone for machine learning at scale. Looking ahead, innovations like AutoML and explainable AI will further refine how we build, deploy, and maintain ML models. For organizations aiming to stay competitive in a data-driven world, embracing AI-powered MLOps isn’t just an option—it’s a necessity. By investing in this synergy today, businesses can future-proof their ML operations and unlock faster, smarter, and more reliable outcomes tomorrow.
#AI and ML#future of AI and ML#What is MLOps#Differences Between Traditional Software DevOps and MLOps#Benefits of Integrating AI/ML in MLOps
0 notes
Text
AI and ML are dominating the world of technology but maybe they will be a great blessing for society and bring us a lot of positive benefits. AI and ML have some of the advantages which solve a problem within any field, they can automate complicated processes and improve decision making. However the evolution of these technologies has their own hurdles which need to be considered such as issues such as, bias in the algorithms, privacy violations, employment due to automation, and what people do not know about them exist. Their appropriate solution lets us build AI and ML to be safe and effective to apply, bringing more value to people’s lives and minimising potential risks.
0 notes
Text
0 notes
Text
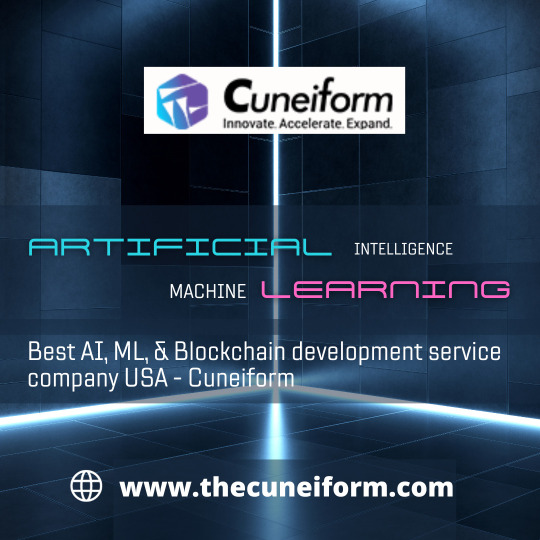
m have a best AI, ML & Block chain development service company USA. Our Artificial Intelligence technologies usher in a new era of intelligent breakthroughs, unlock boundless discoveries, and improve human potential.
#SERP Advertising with AI#AI or ML driven mobile app#AI/ML Development Company#AI in performance testing#Benefits of Ai in performance testing
0 notes
Text
I saw a post the other day calling criticism of generative AI a moral panic, and while I do think many proprietary AI technologies are being used in deeply unethical ways, I think there is a substantial body of reporting and research on the real-world impacts of the AI boom that would trouble the comparison to a moral panic: while there *are* older cultural fears tied to negative reactions to the perceived newness of AI, many of those warnings are Luddite with a capital L - that is, they're part of a tradition of materialist critique focused on the way the technology is being deployed in the political economy. So (1) starting with the acknowledgement that a variety of machine-learning technologies were being used by researchers before the current "AI" hype cycle, and that there's evidence for the benefit of targeted use of AI techs in settings where they can be used by trained readers - say, spotting patterns in radiology scans - and (2) setting aside the fact that current proprietary LLMs in particular are largely bullshit machines, in that they confidently generate errors, incorrect citations, and falsehoods in ways humans may be less likely to detect than conventional disinformation, and (3) setting aside as well the potential impact of frequent offloading on human cognition and of widespread AI slop on our understanding of human creativity...
What are some of the material effects of the "AI" boom?
Guzzling water and electricity
The data centers needed to support AI technologies require large quantities of water to cool the processors. A to-be-released paper from the University of California Riverside and the University of Texas Arlington finds, for example, that "ChatGPT needs to 'drink' [the equivalent of] a 500 ml bottle of water for a simple conversation of roughly 20-50 questions and answers." Many of these data centers pull water from already water-stressed areas, and the processing needs of big tech companies are expanding rapidly. Microsoft alone increased its water consumption from 4,196,461 cubic meters in 2020 to 7,843,744 cubic meters in 2023. AI applications are also 100 to 1,000 times more computationally intensive than regular search functions, and as a result the electricity needs of data centers are overwhelming local power grids, and many tech giants are abandoning or delaying their plans to become carbon neutral. Google’s greenhouse gas emissions alone have increased at least 48% since 2019. And a recent analysis from The Guardian suggests the actual AI-related increase in resource use by big tech companies may be up to 662%, or 7.62 times, higher than they've officially reported.
Exploiting labor to create its datasets
Like so many other forms of "automation," generative AI technologies actually require loads of human labor to do things like tag millions of images to train computer vision for ImageNet and to filter the texts used to train LLMs to make them less racist, sexist, and homophobic. This work is deeply casualized, underpaid, and often psychologically harmful. It profits from and re-entrenches a stratified global labor market: many of the data workers used to maintain training sets are from the Global South, and one of the platforms used to buy their work is literally called the Mechanical Turk, owned by Amazon.
From an open letter written by content moderators and AI workers in Kenya to Biden: "US Big Tech companies are systemically abusing and exploiting African workers. In Kenya, these US companies are undermining the local labor laws, the country’s justice system and violating international labor standards. Our working conditions amount to modern day slavery."
Deskilling labor and demoralizing workers
The companies, hospitals, production studios, and academic institutions that have signed contracts with providers of proprietary AI have used those technologies to erode labor protections and worsen working conditions for their employees. Even when AI is not used directly to replace human workers, it is deployed as a tool for disciplining labor by deskilling the work humans perform: in other words, employers use AI tech to reduce the value of human labor (labor like grading student papers, providing customer service, consulting with patients, etc.) in order to enable the automation of previously skilled tasks. Deskilling makes it easier for companies and institutions to casualize and gigify what were previously more secure positions. It reduces pay and bargaining power for workers, forcing them into new gigs as adjuncts for its own technologies.
I can't say anything better than Tressie McMillan Cottom, so let me quote her recent piece at length: "A.I. may be a mid technology with limited use cases to justify its financial and environmental costs. But it is a stellar tool for demoralizing workers who can, in the blink of a digital eye, be categorized as waste. Whatever A.I. has the potential to become, in this political environment it is most powerful when it is aimed at demoralizing workers. This sort of mid tech would, in a perfect world, go the way of classroom TVs and MOOCs. It would find its niche, mildly reshape the way white-collar workers work and Americans would mostly forget about its promise to transform our lives. But we now live in a world where political might makes right. DOGE’s monthslong infomercial for A.I. reveals the difference that power can make to a mid technology. It does not have to be transformative to change how we live and work. In the wrong hands, mid tech is an antilabor hammer."
Enclosing knowledge production and destroying open access
OpenAI started as a non-profit, but it has now become one of the most aggressive for-profit companies in Silicon Valley. Alongside the new proprietary AIs developed by Google, Microsoft, Amazon, Meta, X, etc., OpenAI is extracting personal data and scraping copyrighted works to amass the data it needs to train their bots - even offering one-time payouts to authors to buy the rights to frack their work for AI grist - and then (or so they tell investors) they plan to sell the products back at a profit. As many critics have pointed out, proprietary AI thus works on a model of political economy similar to the 15th-19th-century capitalist project of enclosing what was formerly "the commons," or public land, to turn it into private property for the bourgeois class, who then owned the means of agricultural and industrial production. "Open"AI is built on and requires access to collective knowledge and public archives to run, but its promise to investors (the one they use to attract capital) is that it will enclose the profits generated from that knowledge for private gain.
AI companies hungry for good data to train their Large Language Models (LLMs) have also unleashed a new wave of bots that are stretching the digital infrastructure of open-access sites like Wikipedia, Project Gutenberg, and Internet Archive past capacity. As Eric Hellman writes in a recent blog post, these bots "use as many connections as you have room for. If you add capacity, they just ramp up their requests." In the process of scraping the intellectual commons, they're also trampling and trashing its benefits for truly public use.
Enriching tech oligarchs and fueling military imperialism
The names of many of the people and groups who get richer by generating speculative buzz for generative AI - Elon Musk, Mark Zuckerberg, Sam Altman, Larry Ellison - are familiar to the public because those people are currently using their wealth to purchase political influence and to win access to public resources. And it's looking increasingly likely that this political interference is motivated by the probability that the AI hype is a bubble - that the tech can never be made profitable or useful - and that tech oligarchs are hoping to keep it afloat as a speculation scheme through an infusion of public money - a.k.a. an AIG-style bailout.
In the meantime, these companies have found a growing interest from military buyers for their tech, as AI becomes a new front for "national security" imperialist growth wars. From an email written by Microsoft employee Ibtihal Aboussad, who interrupted Microsoft AI CEO Mustafa Suleyman at a live event to call him a war profiteer: "When I moved to AI Platform, I was excited to contribute to cutting-edge AI technology and its applications for the good of humanity: accessibility products, translation services, and tools to 'empower every human and organization to achieve more.' I was not informed that Microsoft would sell my work to the Israeli military and government, with the purpose of spying on and murdering journalists, doctors, aid workers, and entire civilian families. If I knew my work on transcription scenarios would help spy on and transcribe phone calls to better target Palestinians, I would not have joined this organization and contributed to genocide. I did not sign up to write code that violates human rights."
So there's a brief, non-exhaustive digest of some vectors for a critique of proprietary AI's role in the political economy. tl;dr: the first questions of material analysis are "who labors?" and "who profits/to whom does the value of that labor accrue?"
For further (and longer) reading, check out Justin Joque's Revolutionary Mathematics: Artificial Intelligence, Statistics and the Logic of Capitalism and Karen Hao's forthcoming Empire of AI.
24 notes
·
View notes
Text
Coming to fountain pens after being into fiber crafts so far long is comical in its size difference. Like yes hello I'm a ball of 30 meters of yarn and I won't even make a scarf. Meanwhile the humble 15 ml of ink in the bottle will easily write 600+ pages using a fine or medium nib. You get people going like "oh god . . . I bought too much ink, I'll never finish it all in my lifetime" about a box smaller than a suitcase, while a crafter is going to the local craft store for the nth time and has a closet overflowing with yarn (the wrong kind)
And like, I think this reflects how we undervalue some aspects of our lives under capitalism because once you actually stop to consider things long term, a shirt that gets torn in less than a year is worth less than a shirt that's $50+ in materials and 20+ hours of unpaid labor. Because that shirt will last forever!! It will last so long you'll be like "ffs I wish I could get rid of this the style is outdated and it doesn't fit me properly anymore." And yet. The short term one IS more expensive when you divide the cost over the years it lasts. That's not just cope, that's Vimes boots theory right there. The fun part where you knit/sew/etc it all yourself is an additional benefit that obscures how much value a handmade shirt (no matter how shittily) has in material life.
With things that are optional, where you can do it the more efficient but not necessarily less costly way, you no longer have to rely on a company to produce the things you need for it. If your gel pen dies an untimely death by its ink running out, and you find you they don't make those refills anymore, you have a plastic casing with nothing new to put in it and no pen to write with. With fountain pens you have a converter option that frees you from proprietary products. It's basically open source handwriting. You can even hand make your own ink (professionals course, do not attempt) so even if you're stuck in the woods you can still write with it if you had the foresight to write down the recipe.
And uhhh what are some other "luxury" hobbies that are just doing things without a subscription model . . . physical journalling/mail doesn't require electricity, film photography doesn't require software updates, typewriters can make things legible without relying on a computer, physical art doesn't rely on software either, hand sewing can work without electricity and you can do more complicated/stronger techniques, etc etc. Basically: electricity isn't bad, but being beholden to a corporation (as we know with AI) who can put a stranglehold on it and remove your capacity to do that task independently is bad. Acoustic hobbies- even if they don't amount to anything from a survivalist or independence from society sense- give people greater autonomy in important areas of their lives that they can leverage when people put in price raising measures. And that's ✨ priceless ✨
Important edit: I don't think "investment pieces" are valuable though. The value of an acoustic hobby is not if you can sell it, it's if companies can't sell to YOU. A mistake people get tripped up on us the $10,000 purses and shit that's just markers of wealth. Hobby value bottoms out low and you're immediately destroying any resell value it has by using it so like. If it isn't materially worth the money you spend on it then it's a ripoff. (Example: fancy decals you don't want? Rip off. Unnecessary gold accents? Rip off. Etc)
22 notes
·
View notes
Text
Okay, I have my own opinions about AI, especially AI art, but this is actually a very cool application!
So when you think about it, we can quantify vision/sight using the actual wavelengths of light, and we can quantify hearing using frequency, but there really isn't a way to quantify smell. So scientists at the University of Reading set out to create an AI to do just that.
The AI was trained on a dataset of 5000 known odor-causing molecules. It was given their structures, and a list of various scent descriptors (such as "floral sweet" or "musty" or "buttery") and how well those descriptors fit on a scale of 1-5. After being trained on this data, the AI was able to be shown a new molecule and predict what its scent would be, using the various descriptors.
The AI's prediction abilities were compared against a panel of humans, who would smell the compound of interest and assign the descriptors. The AI's predictions were actually just as good as the human descriptions. Professor Jane Parker, who worked on the project, explained the following.
"We don't currently have a way to measure or accurately predict the odor of a molecule, based on its molecular structure. You can get so far with current knowledge of the molecular structure, but eventually you are faced with numerous exceptions where the odor and structure don't match. This is what has stumped previous models of olfaction. The fantastic thing about this new ML generated model is that it correctly predicts the odor of those exceptions"
Now what can we do with this "AI Nose", you might ask? Well, it may have benefits in the food and fragrance industries, for one. A machine that is able to quickly filter through compounds to find one with specific odor qualities could be a good way to find new, sustainable sources of fragrance in foods or perfumes. The team also believes that this "scent map" that the AI model builds could be linked to metabolism. In other words, odors that are close to each other on the map, or smell similar, are also more likely to be metabolically related
#science#stem#science side of tumblr#stemblr#biology#biochemistry#chemistry#machine learning#technology#innovation
338 notes
·
View notes
Text
i keep developing skills and talents that are not terribly ethical. the primary subfield of physics i worked on in undergrad is really only useful for natsec jobs, which im not eligible for or willing to do. the primary skillset im developing in grad school is... well, "full stack" AI/ML. which i probably wont have a hard time finding a job doing but most of the jobs i can find will not exactly be making the world a better place! high chances if i take the first offer i get im either spying on people to more efficiently sell them products or im finding ways to automate people's jobs away for only marginal benefit to the overall economy. if im lucky maybe i'll get something in advanced manufacturing or knowledge management or something not totally horrible. or just like a generic software dev job i guess
but uhhhhh i hate how little this feels like a coincidence? jobs pay well because not a lot of people can do them but also because they fuck over a lot of people and thats what results in a sufficient amount of wealth to pay the salaries that highly-skilled™ workers like me will demand. but maybe i can land something meaningless and inconsequential enough that i can sleep easier knowing im not making the world not too much worse. if i play my cards right i'll probably "semi-retire" at 40 and go back to grad school for something i actually care about. maybe i can spend the second half(?) of it doing something i truly think is worthwhile even if it doesnt pay all that much. i guess that's far luckier than most people...
11 notes
·
View notes
Note
Do you have any explanation for why so many Tumblr communists (MLs in particular) are so heavily “pro-AI”? I’ve tried to understand where they are coming from, but their arguments are all very contrarian, so I can’t really follow them.
ML have always been very pro-capital, going back to Lenin:
If the words we have quoted provoke a smile, the following discovery made by the “Left Communists” will provoke nothing short of Homeric laughter. According to them, under the “Bolshevik deviation to the right” the Soviet Republic is threatened with “evolution towards state capitalism”. They have really frightened us this time! And with what gusto these “Left Communists” repeat this threatening revelation in their theses and articles. . . .
It has not occurred to them that state capitalism would be a step forward as compared with the present state of affairs in our Soviet Republic. If in approximately six months’ time state capitalism became established in our Republic, this would be a great success and a sure guarantee that within a year socialism will have gained a permanently firm hold and will have become invincible in our country.
They mistake the progressive nature of capitalism for a reason to support it. They do not understand that in doing so they put themselves in an antagonist position toward proletarians.
For the AI thing specifically, there's also the fact that anti-AI people on the internet are often being idiots about it. They do fall into legalistic, copyright-fundamentalist attitudes, or make arguments about the soullesness of the machine, or say wrong things about the technology.
But that's understandable, we shouldn't expect otherwise. It's up to would be marxists to be better and articulate what, in my opinion, is the actual problem. Namely that what's going on is the conversion of labor into value without compensation (again, capital).
As for what should be done about it I think artists and writers having the right to withold their work from being used for training is a perfectly reasonable compromise and also a tangible, achievable goal. People will say it's not because IP exists to benefit corporations, but by that logic we never would have had things like the minimum wage. The capitalist system is biased toward capitalists but they do have to pretend to respect their own rules, and you can use this to extract concessions.
2 notes
·
View notes
Text
The good AI.
I want my computer to be a scrungly little guy. I want my computer to be GERTY (The friendly AI from the movie Moon).
I want to stagger in, of a morning with my coffee and say "Hey there little buddy, whaaaa?" and my little silicone brain in a box goes "Oh hey. You got a couple of emails - most are boring but there's a coupon for frozen pizza. Your friends had a big chat on Discord. Groggers wanted to talk to you but I told them you were asleep. Want the news?"
And then it loads up Google news.
It tells me about thing I wanted to do that I set calendar stuff for, or reminders. It lets me know if there's any deals on my wishlist and shopping list items. It has a Wiki of stuff I've saved (Recipes, manuals, how-tos, interesting articles, notes like "Don't buy that brand of frozen pizza. Ugh!") and does agent stuff: If I tell it I want a holiday it goes through my list of destinations while the computer's idle and lets me know if it found a good deal.
If I want to cook I can tell it what I'm cooking and it checks my Wiki for a recipe then answers questions about it and does unit conversion, sets timers...
I want it to run on my phone so while I'm out and I get messages I can tell the AI to respond by typing a response like:
🤖"Assistant here: The boss is wandering around and has his hands full. They said to tell you they'll talk later when they're home"
.. or let the AI used advanced spellcheck trained on my style to do speech to text or fix my typing fumbles.
Y'know. Useful stuff.
Specifically stuff that'd mean you didn't necessarily have to look at a screen. You know who'd benefit from that? Everyone.
But especially people who can't see well, have motor skill issues or got left behind by technology. Boomers. Kids who weren't taught computer skills. Blind or partially sighted people.
You know how valuable a phone or a tablet would be if you could prop it up and talk to it about recipes you saved? Where you could go through your freezer, fridge, and cupboards and let it keep track of your food and make suggestions to use fresh food up before it expires?
What I do have is an LLM that sits in a window can't interact except by outputting text, and consistently gets every answer wrong.
It'll even run on an iPad. Hilariously when I asked what it thought it was running on it assume dit was 2065 and it was running on a quantum supercomputer that was simulating a fully intelligent mind.
Like no, it's an iPad mini with 4GB of ram, and the first persona I tried building kept trying to typefuck me.
It's not even good at proof reading or spell checking. It definitely can't do math, or correctly generate BASH scripts (Or ZSH). But it's fine for taking an input and talking about it in a reasonably affable manner.
So I feel that eventually systems will be two parts: A ML system that extracts information from videos, web pages and system notifications, and small fast LLM that uses a lore document and a persona prompt that turns the information into conversational output.
2 notes
·
View notes
Text
Intelligent Data Management in Life Sciences: A Game Changer for the Pharmaceutical Industry
In the fast-paced world of life sciences and pharmaceuticals, data management is crucial for driving innovation, enhancing compliance, and ensuring patient safety. With an ever-growing volume of data being generated across clinical trials, drug development, and regulatory compliance, pharmaceutical companies face the challenge of managing and analyzing this vast amount of data efficiently. Intelligent data management offers a solution to these challenges, ensuring that organizations in the life sciences industry can harness the full potential of their data.
Mastech InfoTrellis is a leader in implementing AI-first data management solutions, enabling pharmaceutical companies to streamline their operations, improve decision-making, and accelerate their research and development efforts. This blog explores the critical role of intelligent data management in the pharmaceutical industry, focusing on how Mastech InfoTrellis helps companies navigate data complexity to enhance business outcomes.
What Is Intelligent Data Management in Life Sciences?
Intelligent data management refers to the use of advanced technologies, such as artificial intelligence (AI), machine learning (ML), and automation, to manage, analyze, and leverage data in a way that improves operational efficiency and decision-making. In the life sciences industry, data is generated from various sources, including clinical trials, electronic health records (EHR), genomic research, and regulatory filings. Intelligent data management solutions help pharmaceutical companies streamline the collection, organization, and analysis of this data, making it easier to extract actionable insights and comply with stringent regulatory requirements.
Mastech InfoTrellis applies cutting-edge data management solutions tailored to the pharmaceutical industry, focusing on improving data accessibility, enhancing data governance, and enabling real-time analytics for better decision-making.
Join - ReimAIgined Intelligence at Informatica World 2025
The Importance of Data Management in the Pharmaceutical Industry
Effective data management is the backbone of the pharmaceutical industry. With the increasing volume of data generated in drug discovery, clinical trials, and regulatory compliance, pharmaceutical companies need intelligent systems to handle this data efficiently. Poor data management can lead to significant challenges, such as:
Regulatory non-compliance: In the pharmaceutical industry, compliance with global regulations, including those from the FDA and EMA, is paramount. Mishandling data or failing to track changes in regulations can lead to severe penalties and delays in product approvals.
Data silos: In many organizations, data is stored in different departments or systems, making it difficult to access and analyze holistically. This leads to inefficiencies and delays in decision-making.
Inaccurate data insights: Inaccurate or incomplete data can hinder the development of new drugs or the identification of critical health trends, affecting the overall success of research and development projects.
Intelligent data management solutions, such as those offered by Mastech InfoTrellis, address these issues by ensuring that data is accurate, accessible, and actionable, helping pharmaceutical companies optimize their workflows and drive better business outcomes.
Key Benefits of Intelligent Data Management in Life Sciences
1. Improved Data Governance and Compliance
In the pharmaceutical industry, data governance is a critical function, particularly when it comes to regulatory compliance. Intelligent data management solutions automate the processes of data validation, audit trails, and reporting, ensuring that all data handling processes comply with industry regulations.
Mastech InfoTrellis provides Informatica CDGC (Cloud Data Governance and Compliance), which ensures that data management processes align with industry standards such as Good Clinical Practice (GCP), Good Manufacturing Practice (GMP), and 21 CFR Part 11. This integration enhances data traceability and ensures that pharmaceutical companies can provide accurate and timely reports to regulatory bodies.
2. Enhanced Data Access and Collaboration
In a complex, multi-departmental organization like a pharmaceutical company, it is essential to have data that is easily accessible to the right stakeholders at the right time. Intelligent data management systems ensure that data from clinical trials, research teams, and regulatory departments is integrated into a unified platform.
With Mastech InfoTrellis's AI-powered Reltio MDM (Master Data Management) solution, pharmaceutical companies can break down data silos and provide a 360-degree view of their operations. This enables seamless collaboration between teams and faster decision-making across departments.
3. Faster Drug Development and Innovation
Pharmaceutical companies must make data-driven decisions quickly to bring new drugs to market efficiently. Intelligent data management accelerates the process by enabling faster access to real-time data, reducing the time spent on data gathering and analysis.
By leveraging AI and machine learning algorithms, Mastech InfoTrellis can automate data analysis, providing real-time insights into clinical trial results and research data. This accelerates the identification of promising drug candidates and speeds up the development process.
4. Real-Time Analytics for Better Decision-Making
In life sciences, every minute counts, especially during clinical trials and regulatory submissions. Intelligent data management systems provide pharmaceutical companies with real-time analytics that can help them make informed decisions faster.
By applying AI-powered analytics, pharmaceutical companies can quickly identify trends, predict outcomes, and optimize clinical trial strategies. This allows them to make data-backed decisions that improve drug efficacy, reduce adverse reactions, and ensure patient safety.
Mastech InfoTrellis: Transforming Data Management in the Pharmaceutical Industry
Mastech InfoTrellis is at the forefront of intelligent data management in the life sciences sector. The company's AI-first approach combines the power of Reltio MDM, Informatica CDGC, and AI-driven analytics to help pharmaceutical companies streamline their data management processes, improve data quality, and accelerate decision-making.
By leveraging Master Data Management (MDM) and Cloud Data Governance solutions, Mastech InfoTrellis empowers pharmaceutical companies to:
Integrate data from multiple sources for a unified view
Enhance data accuracy and integrity for better decision-making
Ensure compliance with global regulatory standards
Optimize the drug development process and improve time-to-market
Real-World Use Case: Improving Clinical Trial Efficiency
One real-world example of how intelligent data management is revolutionizing the pharmaceutical industry is the use of Mastech InfoTrellis's Reltio MDM solution in clinical trials. By integrating data from multiple trial sites, research teams, and regulatory bodies, Mastech InfoTrellis helped a major pharmaceutical company reduce the time spent on data gathering and processing by over 30%, enabling them to focus on analyzing results and making quicker decisions. This improvement led to a faster drug approval process and better patient outcomes.
People Also Ask
How does data management benefit the pharmaceutical industry?
Data management in the pharmaceutical industry ensures that all data, from clinical trials to regulatory filings, is accurate, accessible, and compliant with industry regulations. It helps streamline operations, improve decision-making, and speed up drug development.
What is the role of AI in pharmaceutical data management?
AI enhances pharmaceutical data management by automating data analysis, improving data accuracy, and providing real-time insights. AI-driven analytics allow pharmaceutical companies to identify trends, predict outcomes, and optimize clinical trials.
What are the challenges of data management in the pharmaceutical industry?
The pharmaceutical industry faces challenges such as data silos, regulatory compliance, and the sheer volume of data generated. Intelligent data management solutions help address these challenges by integrating data, automating governance, and providing real-time analytics.
Conclusion: The Future of Data Management in Life Sciences
Intelligent data management is no longer just an option for pharmaceutical companies—it's a necessity. With the power of AI, machine learning, and advanced data integration tools, Mastech InfoTrellis is helping pharmaceutical companies improve efficiency, compliance, and decision-making. By adopting these solutions, life sciences organizations can not only enhance their current operations but also position themselves for future growth and innovation.
As the pharmaceutical industry continues to evolve, intelligent data management will play a critical role in transforming how companies develop and deliver life-changing therapies to the market.
2 notes
·
View notes
Text
What is artificial intelligence (AI)?
Imagine asking Siri about the weather, receiving a personalized Netflix recommendation, or unlocking your phone with facial recognition. These everyday conveniences are powered by Artificial Intelligence (AI), a transformative technology reshaping our world. This post delves into AI, exploring its definition, history, mechanisms, applications, ethical dilemmas, and future potential.
What is Artificial Intelligence? Definition: AI refers to machines or software designed to mimic human intelligence, performing tasks like learning, problem-solving, and decision-making. Unlike basic automation, AI adapts and improves through experience.
Brief History:
1950: Alan Turing proposes the Turing Test, questioning if machines can think.
1956: The Dartmouth Conference coins the term "Artificial Intelligence," sparking early optimism.
1970s–80s: "AI winters" due to unmet expectations, followed by resurgence in the 2000s with advances in computing and data availability.
21st Century: Breakthroughs in machine learning and neural networks drive AI into mainstream use.
How Does AI Work? AI systems process vast data to identify patterns and make decisions. Key components include:
Machine Learning (ML): A subset where algorithms learn from data.
Supervised Learning: Uses labeled data (e.g., spam detection).
Unsupervised Learning: Finds patterns in unlabeled data (e.g., customer segmentation).
Reinforcement Learning: Learns via trial and error (e.g., AlphaGo).
Neural Networks & Deep Learning: Inspired by the human brain, these layered algorithms excel in tasks like image recognition.
Big Data & GPUs: Massive datasets and powerful processors enable training complex models.
Types of AI
Narrow AI: Specialized in one task (e.g., Alexa, chess engines).
General AI: Hypothetical, human-like adaptability (not yet realized).
Superintelligence: A speculative future AI surpassing human intellect.
Other Classifications:
Reactive Machines: Respond to inputs without memory (e.g., IBM’s Deep Blue).
Limited Memory: Uses past data (e.g., self-driving cars).
Theory of Mind: Understands emotions (in research).
Self-Aware: Conscious AI (purely theoretical).
Applications of AI
Healthcare: Diagnosing diseases via imaging, accelerating drug discovery.
Finance: Detecting fraud, algorithmic trading, and robo-advisors.
Retail: Personalized recommendations, inventory management.
Manufacturing: Predictive maintenance using IoT sensors.
Entertainment: AI-generated music, art, and deepfake technology.
Autonomous Systems: Self-driving cars (Tesla, Waymo), delivery drones.
Ethical Considerations
Bias & Fairness: Biased training data can lead to discriminatory outcomes (e.g., facial recognition errors in darker skin tones).
Privacy: Concerns over data collection by smart devices and surveillance systems.
Job Displacement: Automation risks certain roles but may create new industries.
Accountability: Determining liability for AI errors (e.g., autonomous vehicle accidents).
The Future of AI
Integration: Smarter personal assistants, seamless human-AI collaboration.
Advancements: Improved natural language processing (e.g., ChatGPT), climate change solutions (optimizing energy grids).
Regulation: Growing need for ethical guidelines and governance frameworks.
Conclusion AI holds immense potential to revolutionize industries, enhance efficiency, and solve global challenges. However, balancing innovation with ethical stewardship is crucial. By fostering responsible development, society can harness AI’s benefits while mitigating risks.
2 notes
·
View notes
Text
How to create Dynamic and Adaptive AI for Mobile Games
In the competitive world of mobile gaming, creating an experience that keeps players coming back requires more than just stunning graphics and intuitive controls. Today's gamers demand intelligent, responsive opponents and allies that adapt to their play style and provide consistent challenges. Let's dive into how dynamic and adaptive AI can transform your mobile game development process and create more engaging experiences for your players.

Why AI Matters in Mobile Game Development
When we talk about mobile games, we're dealing with a unique set of constraints and opportunities. Players engage in shorter sessions, often in distracting environments, and expect experiences that can be both casual and deeply engaging. Traditional scripted behaviors simply don't cut it anymore.
Dynamic AI systems that learn and evolve provide several key benefits:
They create unpredictable experiences that increase replayability
They adjust difficulty in real-time to maintain the perfect challenge level
They create the illusion of intelligence without requiring massive computational resources
They help personalize the gaming experience for each player
Building Blocks of Adaptive Game AI
Behavior Trees and Decision Making
At the foundation of most game AI systems are behavior trees - hierarchical structures that organize decision-making processes. For mobile games, lightweight behavior trees can be incredibly effective. They allow NPCs (non-player characters) to evaluate situations and select appropriate responses based on current game states.
The beauty of behavior trees in mobile development is that they're relatively simple to implement and don't require excessive processing power. A well-designed behavior tree can give the impression of complex decision-making while actually running efficiently on limited mobile hardware.
Machine Learning for Pattern Recognition
While traditional AI techniques still dominate mobile game development, machine learning is making inroads where appropriate. Simple ML models can be trained to recognize player patterns and adapt accordingly:
"We implemented a basic ML model that tracks how aggressive players are during combat sequences," says indie developer Sarah Chen. "What surprised us was how little data we needed to create meaningful adaptations. Even with just a few gameplay sessions, our enemies began responding differently to cautious versus aggressive players."
For mobile games, the key is implementing lightweight ML solutions that don't drain battery or require constant server connections.
Dynamic Difficulty Adjustment
Perhaps the most immediately valuable application of adaptive AI is in difficulty balancing. Games that are too easy become boring; games that are too hard lead to frustration and abandonment.
By monitoring player success rates, completion times, and even physiological indicators like input force or timing patterns, games can subtly adjust challenge levels. For example:
If a player fails a level multiple times, enemy spawn rates might decrease slightly
If a player breezes through challenges, puzzle complexity might increase
If a player shows mastery of one game mechanic, the AI can introduce variations that require new strategies
The trick is making these adjustments invisible to the player. Nobody wants to feel like the game is "letting them win," but everyone appreciates a well-balanced challenge.
Implementation Strategies for Mobile Platforms
Distributed Computing Approaches
Mobile devices have limits, but that doesn't mean your AI needs to be simple. Consider a hybrid approach:
Handle immediate reactions and simple behaviors on-device
Offload more complex learning and adaptation to occasional server communications
Update AI behavior parameters during normal content updates
This approach keeps gameplay smooth while still allowing for sophisticated adaptation over time.
Optimizing for Battery and Performance
When designing AI systems for mobile games, efficiency isn't optional - it's essential. Some practical tips:
Limit AI updates to fixed intervals rather than every frame
Use approximation algorithms when exact calculations aren't necessary
Consider "fake" AI that gives the impression of intelligence through clever design rather than complex computations
Batch AI calculations during loading screens or other natural pauses
"Our most sophisticated enemy AI actually uses less processing power than our earliest attempts," notes mobile game developer Marcus Kim. "We realized that perceived intelligence matters more than actual computational complexity."
Case Studies: Adaptive AI Success Stories
Roguelike Mobile Games
Games like "Dead Cells Mobile" and "Slay the Spire" have shown how procedural generation paired with adaptive difficulty can create nearly infinite replayability. These games analyze player performance and subtly adjust enemy compositions, item drops, and challenge levels to maintain engagement.
Casual Puzzle Games
Even simple puzzle games benefit from adaptive AI. Games like "Two Dots" adapt difficulty curves based on player performance, ensuring that casual players aren't frustrated while still challenging veterans.
Ethical Considerations in Game AI
As we develop more sophisticated AI systems, ethical questions emerge:
How transparent should we be about adaptation mechanisms?
Is it fair to create different experiences for different players?
How do we ensure AI systems don't manipulate vulnerable players?
The mobile game community is still working through these questions, but most developers agree that the player experience should come first, with adaptations designed to maximize enjoyment rather than exploitation.
Looking Forward: The Future of Mobile Game AI
As mobile devices continue to become more powerful, the possibilities for on-device AI expand dramatically. We're already seeing games that incorporate:
Natural language processing for more realistic NPC interactions
Computer vision techniques for AR games that understand the player's environment
Transfer learning that allows AI behaviors to evolve across multiple play sessions
The most exciting developments may come from combining these approaches with traditional game design wisdom.
Getting Started with Adaptive AI
If you're new to implementing adaptive AI in your mobile games, start small:
Identify one aspect of your game that could benefit from adaptation (enemy difficulty, resource scarcity, puzzle complexity)
Implement simple tracking of relevant player metrics
Create modest adjustments based on those metrics
Test extensively with different player types
Iterate based on player feedback
Remember that the goal isn't to create the most technically impressive AI system, but to enhance player experience through thoughtful adaptation.
Conclusion
Dynamic and adaptive AI represents one of the most exciting frontiers in mobile game development. By creating opponents and systems that respond intelligently to player behavior, we can deliver more engaging, personalized experiences that keep players coming back. Whether you're developing a casual puzzle game or an ambitious mobile RPG, incorporating adaptive elements can elevate your game to new heights of player satisfaction.
The most successful mobile games of tomorrow won't just have the flashiest graphics or the most innovative mechanics – they'll be the ones that seem to understand their players, providing just the right challenge at just the right moment through intelligent, adaptive AI systems.
#game#mobile game development#metaverse#nft#blockchain#multiplayer games#unity game development#vr games#gaming
2 notes
·
View notes