#Bidirectional Encoder Representations from Transformers
Explore tagged Tumblr posts
Text
Mengenal Google Featured Snippets dan Teknologi AI Terbaru: MUM, BERT, dan Google SGE
Google Featured Snippets, atau sering disebut juga sebagai Ringkasan AI, adalah teknologi terbaru yang dikembangkan oleh Google untuk menampilkan jawaban cepat dan relevan langsung di hasil pencarian. Menggunakan teknologi canggih seperti MUM (Multitask Unified Model) dan BERT (Bidirectional Encoder Representations from Transformers), Google mampu memahami konteks konten dengan lebih baik, membantu pengguna menemukan jawaban yang lebih kompleks dan mendalam. Dengan adanya Google SGE (Search Generative Experience), hasil pencarian menjadi semakin informatif, disertai sumber-sumber referensi yang lebih lengkap.

Mengenal Google Featured Snippets atau Ringkasan AI
Halo, Mang Oyo SEO Gacor di sini! Kali ini saya akan membahas satu fitur luar biasa dari Google yang disebut Google Featured Snippets atau Ringkasan AI. Mungkin teman-teman sudah sering melihatnya—jawaban langsung di atas hasil pencarian, tanpa perlu masuk ke website lain. Ya, itulah snippet, atau bahasa sederhananya, “jawaban cepat”.
Apa itu Google Featured Snippets?
Google Featured Snippets adalah ringkasan dari jawaban yang Google anggap paling relevan untuk pertanyaan atau pencarian tertentu. Dengan fitur ini, pengguna bisa mendapatkan informasi yang dicari tanpa harus mengklik halaman web. Google menampilkan informasi dari berbagai sumber di halaman pertama, di bagian yang sering disebut sebagai "posisi nol".
Mengapa Google Menyediakan Featured Snippets?
Tujuan utama Google dengan Featured Snippets ini adalah meningkatkan pengalaman pengguna. Google ingin membantu kita semua mendapatkan jawaban yang cepat, tepat, dan ringkas atas pertanyaan umum. Di balik itu, ada teknologi canggih yang bekerja, seperti MUM, BERT, dan SGE.
Teknologi di Balik Google Featured Snippets
1. BERT (Bidirectional Encoder Representations from Transformers)
Pertama-tama, kita kenalan dengan BERT, teknologi AI Google yang memungkinkan mesin pencari memahami konteks kata-kata dalam sebuah kalimat. Contohnya, jika Anda mengetik "tempat wisata murah di Bandung," BERT akan memahami bahwa Anda mencari lokasi dengan harga terjangkau, bukan sekadar informasi acak tentang Bandung.
Bagaimana BERT Bekerja? BERT berfokus pada hubungan antar-kata dalam sebuah kalimat, baik sebelum maupun setelah kata kunci. Dengan begitu, Google bisa lebih memahami apa sebenarnya yang Anda cari.
2. MUM (Multitask Unified Model)
Selanjutnya ada MUM, teknologi yang lebih canggih dari BERT. MUM mampu menangani pencarian yang lebih kompleks dan mendalam. Misalnya, jika Anda bertanya, "Perlengkapan apa yang dibutuhkan untuk mendaki Gunung Gede di musim hujan?" MUM bisa memahami bahwa Anda mencari informasi detail dan konteks spesifik terkait musim, lokasi, dan aktivitas.
Keunggulan MUM:
MUM bisa mengerti maksud dari pertanyaan yang lebih rumit.
MUM bisa menjawab dalam berbagai bahasa.
MUM mengolah informasi visual selain teks, jadi kalau Anda unggah foto sepatu mendaki, MUM bisa memberikan rekomendasi perlengkapan mendaki lainnya.
3. Google SGE (Search Generative Experience)
Terakhir, yang tak kalah menarik adalah Google SGE. Inovasi ini menghadirkan hasil pencarian yang lebih generatif atau memberikan jawaban lebih mendalam. Dengan teknologi ini, Google bisa menciptakan konten yang kompleks langsung di hasil pencarian, lengkap dengan sumber referensi di bawahnya.
Contoh Penggunaan SGE: Jika Anda mencari “cara membuat strategi SEO yang efektif,” Google SGE bisa memberikan langkah-langkah spesifik yang merangkum informasi dari beberapa artikel yang relevan. Ini sangat membantu bagi pebisnis kecil yang ingin belajar tanpa harus membaca banyak artikel.
Cara Mang Oyo SEO Mengoptimalkan Konten untuk Featured Snippets
Nah, bagaimana caranya supaya konten kita bisa muncul di Google Featured Snippets? Ini dia panduan singkat dari Mang Oyo SEO Gacor:
Jawab Pertanyaan Secara Langsung Google suka menampilkan jawaban yang langsung ke intinya, jadi pastikan artikel Anda memberikan jawaban yang jelas.
Gunakan Format yang Tepat Misalnya, untuk pertanyaan "apa itu SEO?" sertakan penjelasan ringkas di paragraf pertama. Untuk panduan langkah-langkah, gunakan bullet points atau numbering. Format seperti ini lebih berpotensi muncul di snippet.
Pakai Heading (H1, H2, H3) yang Relevan Struktur heading juga berpengaruh. Gunakan heading yang sesuai dengan kata kunci dan subtopik agar Google memahami hierarki informasi dalam artikel.
Perhatikan Panjang Artikel dan Keunikan Konten Featured Snippets biasanya muncul dari artikel yang mendalam, unik, dan menjawab berbagai sisi dari pertanyaan. Pastikan tidak ada duplikasi dan konten Anda memiliki gaya bahasa sendiri yang sesuai.
Optimalkan dengan Data Structured (Schema Markup) Tambahkan schema markup pada bagian tertentu dalam artikel Anda agar Google mudah memahaminya sebagai jawaban potensial.
Kesimpulan
Google Featured Snippets, MUM, BERT, dan SGE adalah inovasi yang mengubah cara kita menemukan informasi. Dengan pemahaman konteks yang lebih mendalam, Google dapat memberikan jawaban yang relevan dan sesuai kebutuhan pengguna. Bagi pemilik bisnis atau pengelola situs, memahami dan mengoptimalkan konten untuk snippet ini menjadi peluang besar untuk meningkatkan visibilitas dan lalu lintas situs.
Dengan mengikuti panduan ini, Anda bisa membuat artikel yang lebih berpotensi tampil di posisi teratas hasil pencarian Google. Semoga artikel dari Mang Oyo SEO Gacor ini bisa membantu Anda menaklukkan Google Featured Snippets!
#Multitask Unified Model#Bidirectional Encoder Representations from Transformers#Google Featured Snippets#Ringkasan AI
0 notes
Text
What is BERT(Bidirectional Encoder Representations from Transformers) - day 67
Understanding BERT: How It Works and Its Role in NLP Understanding BERT: How It Works and Why It’s Transformative in NLP BERT (Bidirectional Encoder Representations from Transformers) is a foundational model in Natural Language Processing (NLP) that has reshaped how machines understand language. Developed by Google in 2018, BERT brought significant improvements in language understanding tasks by…
0 notes
Text
The Future of Digital Marketing in 2025 – Trends Every Business Must Adopt
Introduction
As we step into 2025, digital marketing is evolving at an unprecedented pace. Businesses that stay ahead of trends will increase brand visibility, attract more leads, and boost conversions. From AI-driven SEO to hyper-personalized marketing, the digital landscape is more competitive than ever.
Whether you’re a small business owner, entrepreneur, or marketing professional, understanding these trends will help you craft a winning digital marketing strategy. Let’s explore the top digital marketing trends for 2025 that will shape the future of online success.
1. AI-Powered SEO is the Future
Search engines are becoming smarter and more intuitive. With AI-powered algorithms like Google’s MUM (Multitask Unified Model) and BERT (Bidirectional Encoder Representations from Transformers), traditional SEO tactics are no longer enough.
How AI is Transforming SEO in 2025?
✔ AI-driven content creation: Advanced AI tools analyze search intent to create highly relevant, optimized content. ✔ Predictive analytics: AI predicts user behavior, helping businesses optimize content for better engagement. ✔ Voice and visual search optimization: As voice assistants like Siri, Alexa, and Google Assistant become more popular, brands must adapt their SEO strategy to long-tail conversational queries.
Actionable Tip: Optimize for natural language searches, use structured data markup, and ensure website accessibility to improve rankings in 2025.
2. Video Marketing Continues to Dominate
With platforms like TikTok, Instagram Reels, and YouTube Shorts, video marketing is becoming the most powerful form of content in 2025.
Why is Video Marketing Essential?
📌 80% of internet traffic will be video content by 2025 (Cisco Report). 📌 Short-form videos increase engagement and hold attention longer than static content. 📌 Live streaming and interactive videos help brands connect with audiences in real-time.
Actionable Tip: Focus on storytelling, behind-the-scenes content, product demonstrations, and influencer collaborations to boost engagement.
3. Hyper-Personalization with AI & Data Analytics
Consumers expect highly personalized experiences, and AI-powered marketing automation makes it possible.
How Does Hyper-Personalization Work?
✔ AI analyzes customer behavior and past interactions to create tailored marketing messages. ✔ Email marketing campaigns are dynamically personalized based on user interests. ✔ Chatbots and voice assistants provide real-time, customized support.
Actionable Tip: Leverage tools like HubSpot, Salesforce, and Marketo to automate personalized marketing campaigns.
4. Influencer Marketing Becomes More Authentic
The influencer marketing industry is projected to reach $21.1 billion by 2025. However, brands are shifting from celebrity influencers to micro and nano-influencers for better authenticity and engagement.
Why Micro-Influencers Matter?
🎯 Higher engagement rates than macro-influencers. 🎯 More trust & relatability with niche audiences. 🎯 Cost-effective collaborations for brands with limited budgets.
Actionable Tip: Partner with influencers in your niche and use user-generated content (UGC) to enhance brand credibility.
5. Voice & Visual Search Optimization is a Must
By 2025, 50% of all searches will be voice or image-based, making traditional text-based SEO insufficient.
How to Optimize for Voice & Visual Search?
✔ Use long-tail keywords & conversational phrases. ✔ Optimize images with alt text & structured data. ✔ Ensure your site is mobile-friendly and fast-loading.
Actionable Tip: Implement Google Lens-friendly content to appear in image-based search results.
Conclusion
The future of digital marketing in 2025 is driven by AI, personalization, and immersive experiences. If you’re not adapting, you’re falling behind!
Looking for expert digital marketing strategies? Mana Media Marketing can help you grow and dominate your niche. Contact us today!
2 notes
·
View notes
Text
Understanding Google Algorithm Updates: What You Need to Know
Google is the most dominant search engine in the world, handling over 90% of global searches. One of the key reasons behind its success is its ever-evolving search algorithm. Google constantly refines its search engine algorithms to ensure that users get the most relevant, high-quality results for their queries. But what exactly are Google algorithm updates, and why should you care? More importantly, how can businesses and digital marketers adapt to these updates and stay ahead in SEO?
In this article, we’ll dive into the history of Google algorithm updates, explore some of the most impactful updates, and offer insights on how to optimize your website for these changes. Plus, if you’re looking to become an SEO expert, we’ll show you why joining CADL’s SEO courses in Zirakpur is the perfect way to learn everything you need about mastering Google algorithms.
1. What Is a Google Algorithm Update?
Google’s search algorithm is a complex system used to rank websites and deliver the most relevant search results to users. It takes into account various factors such as the quality of the content, relevance to the search query, page speed, mobile-friendliness, backlinks, and user engagement.
Google regularly updates its algorithm to improve the accuracy and quality of its search results. Some of these updates are minor and go unnoticed by most, while others can significantly impact website rankings. These updates aim to enhance the user experience by filtering out low-quality content and rewarding websites that provide real value.
Key Insight:
Google's algorithm is constantly evolving. By joining CADL's SEO course in Zirakpur, you’ll learn how to optimize your website to meet the changing demands of the algorithm and stay competitive in the digital landscape.
2. History of Google Algorithm Updates
Over the years, Google has rolled out several significant algorithm updates, each with a specific goal in mind. Some of these updates have become famous for the dramatic impact they’ve had on search rankings. Understanding these updates is crucial for any SEO professional.
Here are some of the most impactful updates in Google's history:
a. Panda (2011)
The Panda update was introduced to reduce the rankings of low-quality sites, such as those with thin or duplicate content. It focused on improving search results by promoting high-quality, valuable content and penalizing sites with too many ads, low word count, or keyword stuffing.
b. Penguin (2012)
Penguin targeted spammy link-building practices. Sites that engaged in black-hat SEO techniques, such as buying backlinks or participating in link schemes, saw a sharp drop in their rankings. This update emphasized the importance of natural, high-quality backlinks in improving SEO rankings.
c. Hummingbird (2013)
The Hummingbird update brought a shift towards understanding the meaning behind search queries rather than just matching keywords. Google started to emphasize “semantic search,” which aimed to understand the context and intent behind user searches. This made Google better at answering questions directly and delivering more accurate results.
d. Mobilegeddon (2015)
With mobile search growing rapidly, Google rolled out the Mobilegeddon update to prioritize mobile-friendly websites in search results. Websites that were not optimized for mobile devices saw their rankings decline, while responsive sites got a boost.
e. RankBrain (2015)
RankBrain introduced machine learning into Google’s algorithm. It helped Google better understand ambiguous or complex search queries by identifying patterns in how users interact with search results. RankBrain is now considered one of the top ranking factors.
f. BERT (2019)
BERT (Bidirectional Encoder Representations from Transformers) is an update aimed at better understanding the nuances and context of words in search queries. It allows Google to interpret the meaning behind user queries more effectively, particularly for long-tail keywords or natural language searches.
3. Why Google Updates Matter for SEO
Google algorithm updates are designed to improve the user experience, but they can also cause significant fluctuations in website rankings. SEO professionals need to be aware of these updates to ensure their strategies align with Google’s goals. When an algorithm update rolls out, some websites might see a boost in rankings, while others may experience a drop.
Why Do Google Algorithm Updates Matter?
Maintaining or Improving Rankings: A new update might penalize websites with poor content or shady link-building practices, while rewarding those with high-quality, relevant content. If you're not keeping up with updates, your website may lose visibility and organic traffic.
Adapting SEO Strategies: Each algorithm update comes with new guidelines. For example, after the Penguin update, websites had to shift away from spammy backlinks and focus on building genuine, authoritative links. Not staying informed means you could be optimizing your website incorrectly.
Enhancing User Experience: Google’s updates are always focused on improving the user experience. By aligning your website with Google’s vision, you’re also ensuring that users have a better experience when they visit your site.
Key Insight:
Staying on top of algorithm updates is essential for anyone involved in SEO. CADL’s SEO training in Zirakpur will give you the knowledge to understand and adapt to these changes, keeping your website ahead of the competition.
4. How to Adapt to Google Algorithm Updates
Now that you understand the importance of Google algorithm updates, the next step is learning how to adapt. Here are some strategies to ensure your SEO practices remain up-to-date and effective:
a. Create High-Quality Content
Google's ultimate goal is to provide users with the most relevant and valuable content. Focus on creating in-depth, informative, and engaging content that answers users' questions and solves their problems. Avoid thin content, keyword stuffing, and duplicate pages, as these can negatively impact your rankings.
b. Focus on User Intent
With updates like Hummingbird and RankBrain, Google is increasingly focusing on understanding user intent rather than just matching keywords. When developing content, think about what users are looking for and how you can meet their needs. Answer common questions, provide solutions, and offer valuable insights.
c. Optimize for Mobile
With the Mobilegeddon update and the rise of mobile-first indexing, having a mobile-friendly website is no longer optional. Ensure your site is responsive, loads quickly, and provides a smooth user experience on all devices.
d. Build High-Quality Backlinks
Backlinks remain one of the most important ranking factors, but quality matters more than quantity. Focus on earning backlinks from authoritative, reputable websites in your industry. Avoid participating in link schemes or buying backlinks, as these practices can lead to penalties under updates like Penguin.
e. Monitor Your Analytics
After every algorithm update, it's essential to monitor your website's analytics to identify any changes in traffic, rankings, or user behavior. If you notice a drop in traffic or rankings, investigate whether your site is affected by the update and adjust your SEO strategy accordingly.
f. Stay Informed
SEO is an ever-evolving field, and staying informed about Google algorithm updates is crucial. Regularly read SEO blogs, attend webinars, and join online communities to stay updated on the latest changes and trends. At CADL, you’ll gain access to resources and training that will keep you on top of every important SEO update.
5. How CADL’s SEO Course Can Help You Stay Ahead
As Google continues to refine its algorithm, SEO professionals need to be proactive in adapting to these changes. Whether you're just starting in the field or looking to improve your existing SEO skills, joining CADL's SEO course in Zirakpur is the ideal way to master the strategies necessary for success.
Here’s what you can expect from CADL’s SEO training:
Comprehensive Curriculum: Learn everything from the basics of SEO to advanced techniques for optimizing content, backlinks, and technical SEO.
Real-Time Updates: Stay updated with the latest Google algorithm changes and learn how to implement the best practices that align with these updates.
Hands-On Learning: CADL provides practical, hands-on experience, allowing you to apply what you’ve learned to real-world SEO scenarios.
Expert Guidance: Get guidance from experienced SEO professionals who will help you understand the intricacies of Google’s algorithm and how to work with it, not against it.
Conclusion
Google algorithm updates are an integral part of the SEO landscape, and keeping up with them is essential for maintaining and improving your website’s rankings. From Panda and Penguin to RankBrain and BERT, Google’s updates have transformed the way websites are ranked, putting user experience at the forefront.
If you’re serious about excelling in SEO and staying ahead of the competition, joining CADL’s SEO course in Zirakpur is the best decision you can make. You'll gain the skills and knowledge needed to navigate Google's ever-evolving algorithm, ensuring long-term success in the digital marketing world.
2 notes
·
View notes
Text
Interesting Papers for Week 34, 2024
Co-dependent excitatory and inhibitory plasticity accounts for quick, stable and long-lasting memories in biological networks. Agnes, E. J., & Vogels, T. P. (2024). Nature Neuroscience, 27(5), 964–974.
Action sequence learning, habits, and automaticity in obsessive-compulsive disorder. Banca, P., Herrojo Ruiz, M., Gonzalez-Zalba, M. F., Biria, M., Marzuki, A. A., Piercy, T., … Robbins, T. W. (2024). eLife, 12, e87346.4.
The dynamic state of a prefrontal–hypothalamic–midbrain circuit commands behavioral transitions. Chen, C., Altafi, M., Corbu, M.-A., Trenk, A., van den Munkhof, H., Weineck, K., … Ponomarenko, A. (2024). Nature Neuroscience, 27(5), 952–963.
Transformation of Motion Pattern Selectivity from Retina to Superior Colliculus. DePiero, V. J., Deng, Z., Chen, C., Savier, E. L., Chen, H., Wei, W., & Cang, J. (2024). Journal of Neuroscience, 44(20), e1704232024.
Distributed representations of prediction error signals across the cortical hierarchy are synergistic. Gelens, F., Äijälä, J., Roberts, L., Komatsu, M., Uran, C., Jensen, M. A., … Canales-Johnson, A. (2024). Nature Communications, 15, 3941.
Learning‐induced bidirectional enhancement of inhibitory synaptic metaplasticity. Kundu, S., Paul, B., Reuevni, I., Lamprecht, R., & Barkai, E. (2024). Journal of Physiology, 602(10), 2343–2358.
Retuning of hippocampal representations during sleep. Maboudi, K., Giri, B., Miyawaki, H., Kemere, C., & Diba, K. (2024). Nature, 629(8012), 630–638.
Encoding Manual Dexterity through Modulation of Intrinsic α Band Connectivity. Maddaluno, O., Della Penna, S., Pizzuti, A., Spezialetti, M., Corbetta, M., de Pasquale, F., & Betti, V. (2024). Journal of Neuroscience, 44(20), e1766232024.
Simultaneous, cortex-wide dynamics of up to 1 million neurons reveal unbounded scaling of dimensionality with neuron number. Manley, J., Lu, S., Barber, K., Demas, J., Kim, H., Meyer, D., … Vaziri, A. (2024). Neuron, 112(10), 1694-1709.e5.
Differential contributions of the hippocampal dentate gyrus and CA1 subfield to mnemonic discrimination. Mitchnick, K. A., Marlatte, H., Belchev, Z., Gao, F., & Rosenbaum, R. S. (2024). Hippocampus, 34(6), 278–283.
Biases in hand perception are driven by somatosensory computations, not a distorted hand model. Peviani, V. C., Miller, L. E., & Medendorp, W. P. (2024). Current Biology, 34(10), 2238-2246.e5.
Parallel processing of quickly and slowly mobilized reserve vesicles in hippocampal synapses. Rodriguez Gotor, J. J., Mahfooz, K., Perez-Otano, I., & Wesseling, J. F. (2024). eLife, 12, e88212.3.
Inferring control objectives in a virtual balancing task in humans and monkeys. Sadeghi, M., Sharif Razavian, R., Bazzi, S., Chowdhury, R. H., Batista, A. P., Loughlin, P. J., & Sternad, D. (2024). eLife, 12, e88514.3.
Theta and alpha oscillations in human hippocampus and medial parietal cortex support the formation of location‐based representations. Satish, A., Keller, V. G., Raza, S., Fitzpatrick, S., & Horner, A. J. (2024). Hippocampus, 34(6), 284–301.
Multisensory Integration Underlies the Distinct Representation of Odor-Taste Mixtures in the Gustatory Cortex of Behaving Rats. Stocke, S., & Samuelsen, C. L. (2024). Journal of Neuroscience, 44(20), e0071242024.
A Bayesian observer model reveals a prior for natural daylights in hue perception. Su, Y., Shi, Z., & Wachtler, T. (2024). Vision Research, 220, 108406.
Sleep pressure modulates single-neuron synapse number in zebrafish. Suppermpool, A., Lyons, D. G., Broom, E., & Rihel, J. (2024). Nature, 629(8012), 639–645.
Reinforcement Motor Learning After Cerebellar Damage Is Related to State Estimation. White, C. M., Snow, E. C., & Therrien, A. S. (2023). The Cerebellum, 23(3), 1061–1073.
Sensorimotor adaptation to destabilizing dynamics in weakly electric fish. Yang, Y., Yared, D. G., Fortune, E. S., & Cowan, N. J. (2024). Current Biology, 34(10), 2118-2131.e5.
Performance errors during rodent learning reflect a dynamic choice strategy. Zhu, Z., & Kuchibhotla, K. V. (2024). Current Biology, 34(10), 2107-2117.e5.
#neuroscience#science#research#brain science#scientific publications#cognitive science#neurobiology#cognition#psychophysics#neurons#neural computation#neural networks#computational neuroscience
5 notes
·
View notes
Text
Transfer Learning in NLP: Impact of BERT, GPT-3, and T5 on NLP Tasks
Transfer learning has revolutionized the field of Natural Language Processing (NLP) by allowing models to leverage pre-trained knowledge on large datasets for various downstream tasks. Among the most impactful models in this domain are BERT, GPT-3, and T5. Let's explore these models and their significance in NLP.
1. BERT (Bidirectional Encoder Representations from Transformers)
Overview:
Developed by Google, BERT was introduced in 2018 and marked a significant leap in NLP by using bidirectional training of Transformer models.
Unlike previous models that processed text in a unidirectional manner, BERT looks at both left and right context in all layers, providing a deeper understanding of the language.
Key Features:
Bidirectional Contextual Understanding: BERT’s bidirectional approach allows it to understand the context of a word based on both preceding and following words.
Pre-training Tasks: BERT uses two pre-training tasks – Masked Language Modeling (MLM) and Next Sentence Prediction (NSP). MLM involves predicting masked words in a sentence, while NSP involves predicting if two sentences follow each other in the text.
Impact on NLP Tasks:
Text Classification: BERT's contextual understanding improves the performance of text classification tasks like sentiment analysis and spam detection.
Named Entity Recognition (NER): BERT enhances NER tasks by accurately identifying entities in the text due to its deep understanding of the context.
Question Answering: BERT has set new benchmarks in QA tasks, as it can effectively comprehend and answer questions based on given contexts.
2. GPT-3 (Generative Pre-trained Transformer 3)
Overview:
Developed by OpenAI, GPT-3 is one of the largest language models ever created, with 175 billion parameters.
It follows a unidirectional (left-to-right) autoregressive approach, generating text based on the preceding words.
Key Features:
Scale and Size: GPT-3’s massive size allows it to generate highly coherent and contextually relevant text, making it suitable for a wide range of applications.
Few-Shot Learning: GPT-3 can perform tasks with minimal examples, reducing the need for large labeled datasets for fine-tuning.
Impact on NLP Tasks:
Text Generation: GPT-3 excels at generating human-like text, making it useful for creative writing, chatbots, and content creation.
Translation: While not specifically trained for translation, GPT-3 can perform reasonably well in translating text between languages due to its extensive pre-training.
Code Generation: GPT-3 can generate code snippets and assist in programming tasks, demonstrating its versatility beyond traditional NLP tasks.
3. T5 (Text-to-Text Transfer Transformer)
Overview:
Developed by Google, T5 frames all NLP tasks as a text-to-text problem, where both input and output are text strings.
This unified approach allows T5 to handle a wide variety of tasks with a single model architecture.
Key Features:
Text-to-Text Framework: By converting tasks like translation, summarization, and question answering into a text-to-text format, T5 simplifies the process of applying the model to different tasks.
Pre-training on Diverse Datasets: T5 is pre-trained on the C4 dataset (Colossal Clean Crawled Corpus), which provides a rich and diverse training set.
Impact on NLP Tasks:
Summarization: T5 achieves state-of-the-art results in text summarization by effectively condensing long documents into concise summaries.
Translation: T5 performs competitively in translation tasks by leveraging its text-to-text framework to handle multiple language pairs.
Question Answering and More: T5's versatility allows it to excel in various tasks, including QA, sentiment analysis, and more, by simply changing the text inputs and outputs.
Conclusion
BERT, GPT-3, and T5 have significantly advanced the field of NLP through their innovative architectures and pre-training techniques. These models have set new benchmarks across various NLP tasks, demonstrating the power and versatility of transfer learning. By leveraging large-scale pre-training, they enable efficient fine-tuning on specific tasks, reducing the need for extensive labeled datasets and accelerating the development of NLP applications.
These models have not only improved the performance of existing tasks but have also opened up new possibilities in areas like creative text generation, few-shot learning, and unified task frameworks, paving the way for future advancements in NLP.
3 notes
·
View notes
Text
Natural Language Processing (NLP) and its Advancements
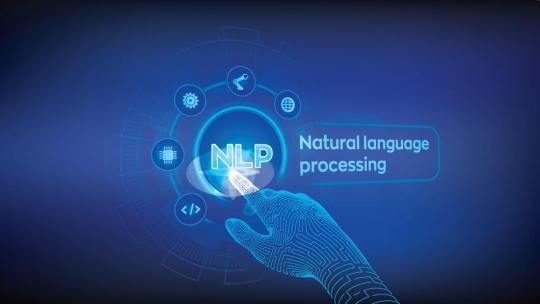
Introduction
Natural Language Processing (NLP) is a branch of artificial intelligence that focuses on the interaction between computers and human language. It aims to enable machines to understand, interpret, and generate natural language, bridging the gap between human communication and computational systems. In this article, we will explore the concept of NLP and discuss its advancements and applications.
Understanding Natural Language Processing (NLP)
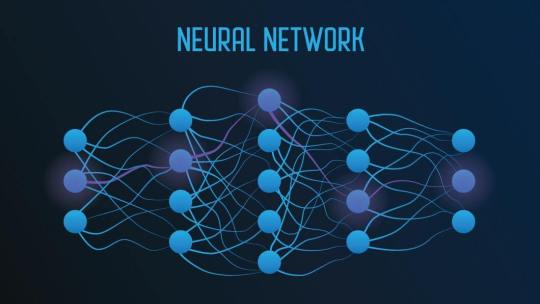
Definition of NLP:
NLP involves the development of algorithms and models that enable computers to process and understand human language. It encompasses a range of tasks, including speech recognition, language understanding, sentiment analysis, machine translation, and text generation.
Key Components of NLP:
NLP involves several key components:
Tokenization: Breaking down text into individual words, phrases, or sentences.
Part-of-Speech (POS) Tagging: Assigning grammatical tags to each word in a sentence.
Named Entity Recognition (NER): Identifying and classifying named entities, such as names, locations, and organizations.
Parsing: Analyzing the grammatical structure of a sentence.
Sentiment Analysis: Determining the sentiment or emotion expressed in a text.
Machine Translation: Translating text from one language to another.
Text Generation: Creating human-like text based on given prompts or contexts.
Advancements in Natural Language Processing (NLP)
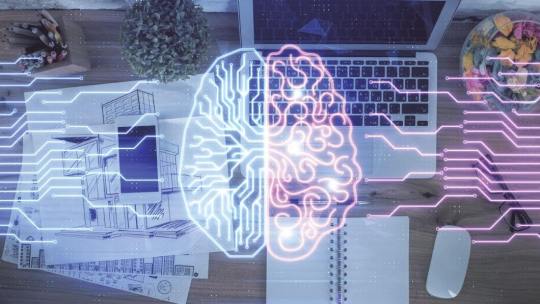
Deep Learning and Neural Networks:Advancements in deep learning and neural networks have significantly contributed to the progress of NLP. Deep learning models, such as recurrent neural networks (RNNs) and transformer models like BERT and GPT, have achieved remarkable results in various NLP tasks. These models can learn complex patterns and dependencies in language data, improving accuracy and performance.
Pretrained Language Models:Pretrained language models have emerged as a game-changer in NLP. Models like BERT (Bidirectional Encoder Representations from Transformers) and GPT (Generative Pretrained Transformer) are pretrained on large amounts of text data and can be fine-tuned for specific tasks. They have shown remarkable capabilities in tasks like question-answering, text completion, and sentiment analysis.
Multilingual NLP:With the global nature of communication, multilingual NLP has gained importance. Researchers have developed models that can handle multiple languages simultaneously, allowing for cross-lingual tasks like machine translation, sentiment analysis, and information retrieval. These advancements are fostering communication and understanding across language barriers.
Contextual Understanding:NLP models are becoming better at understanding the context and nuances of language. Contextual embeddings, such as ELMo and BERT, capture the meaning of a word based on its surrounding words, leading to more accurate and context-aware language understanding. This advancement has improved tasks like question-answering and language generation.
Domain-Specific NLP Applications:NLP is being applied to various industry-specific domains. In healthcare, NLP helps in extracting information from medical records, aiding in diagnosis and treatment. In finance, NLP assists in sentiment analysis for trading decisions and fraud detection. In customer service, chatbots powered by NLP enable efficient and personalized interactions. These domain-specific applications are enhancing productivity and decision-making.
Future Directions of NLP
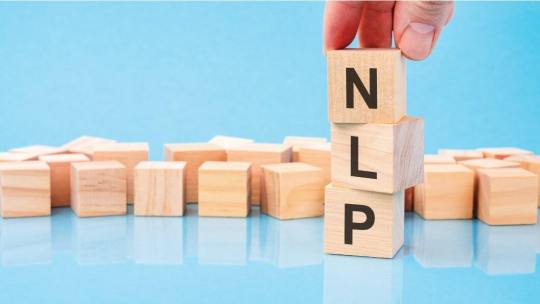
Explainable AI: One of the ongoing challenges in NLP is the lack of transparency and interpretability of models. Future research aims to develop techniques that provide explanations for the decisions made by NLP models, enabling users to understand the reasoning behind the system’s outputs. This will be particularly crucial in sensitive domains where accountability and trust are paramount.
Emotion and Context Recognition: Advancing NLP models to recognize and understand human emotions and contextual cues will enable more nuanced and personalized interactions. Emotion recognition can be useful in chatbots, virtual assistants, and mental health applications. Context recognition will allow systems to adapt their responses based on the user’s situation, leading to more meaningful and relevant interactions.
Ethical Considerations: As NLP becomes more pervasive, it is essential to address ethical considerations. This includes ensuring fairness and mitigating biases in NLP models, protecting user privacy, and establishing guidelines for responsible use of NLP technologies. Ongoing research and collaboration are necessary to develop ethical frameworks and standards that govern the development and deployment of NLP systems.
Cross-Modal NLP: Cross-modal NLP involves integrating multiple modalities, such as text, images, and audio, to achieve a deeper understanding of human communication. This field aims to develop models that can effectively process and interpret information from different modalities, enabling more comprehensive and multimodal interactions.
Continual Learning:Continual learning in NLP focuses on the ability of models to adapt and learn from new data continuously. This is crucial in dynamic environments where language evolves and new concepts emerge. Future NLP systems will be designed to learn incrementally, improving their performance over time and adapting to changing linguistic patterns.
Conclusion
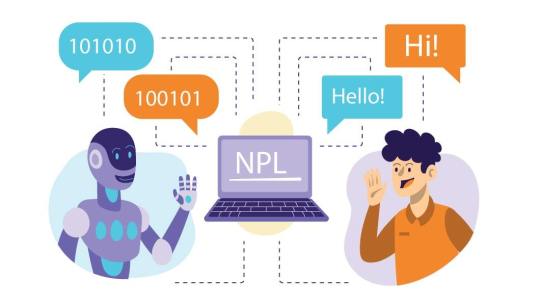
Natural Language Processing has witnessed significant advancements, thanks to developments in deep learning, pretrained models, multilingual capabilities, contextual understanding, and domain-specific applications. These advancements are driving progress in language understanding, sentiment analysis, translation, and text generation. As NLP continues to evolve, we can expect further breakthroughs that will enhance the interaction between humans and machines, making natural language processing more seamless and intuitive.
The advancements in natural language processing have revolutionized the way we interact with computers and machines. From deep learning models to pretrained language models and multilingual capabilities, NLP has made significant progress in understanding and generating human language. Future directions include explainable AI, emotion and context recognition, ethical considerations, cross-modal NLP, and continual learning. As NLP continues to evolve, we can expect more sophisticated language understanding, improved user experiences, and new applications across various industries.
FAQs
FAQ 1: What are some real-world applications of Natural Language Processing (NLP)?
NLP has numerous real-world applications across various domains. Some examples include:
Virtual assistants like Siri and Alexa that understand and respond to spoken commands.
Text analysis tools used in sentiment analysis for understanding customer feedback.
Machine translation services like Google Translate that enable communication across different languages.
Chatbots and customer support systems that provide automated responses to user inquiries.
Information retrieval systems that extract relevant information from large text corpora.
FAQ 2: How does NLP handle different languages and dialects?
NLP research and development focus on handling multiple languages and dialects. Pretrained models like BERT and GPT can be fine-tuned for specific languages. Additionally, language-specific resources like lexicons and grammatical rules are created to support language processing. However, the availability and quality of NLP tools and resources may vary across languages.
FAQ 3: How does NLP deal with understanding the context of words and phrases?
NLP models leverage contextual embeddings and deep learning techniques to understand the context of words and phrases. Models like BERT encode the meaning of a word based on its surrounding words, capturing contextual information. This allows the models to grasp the nuances and multiple meanings of words in different contexts, improving language understanding.
FAQ 4: What challenges does NLP face in understanding human language?
NLP still faces several challenges in understanding human language. Some of these challenges include:
Ambiguity: Words and phrases often have multiple meanings, making it challenging to determine the intended sense in a given context.
Idioms and figurative language: NLP models may struggle to interpret idiomatic expressions, metaphors, or sarcasm.
Out-of-vocabulary words: NLP models may encounter words or phrases that they haven’t seen during training, leading to difficulties in understanding.
Cultural and domain-specific references: NLP models may struggle to comprehend references that are specific to a particular culture or domain.
FAQ 5: How can NLP be used for information extraction from unstructured text?
NLP techniques, such as named entity recognition and relationship extraction, are employed to extract structured information from unstructured text. Named entity recognition identifies and classifies named entities like names, locations, and organizations. Relationship extraction identifies connections between entities. These techniques enable the extraction of valuable information from large volumes of text, aiding in tasks like data mining and knowledge discovery.
2 notes
·
View notes
Text
Mastering BERT for Text Classification: A Step-by-Step Guide to Fine-Tuning for Optimal Accuracy
Text classification is one of the fundamental tasks in Natural Language Processing (NLP), allowing machines to understand and categorize text into predefined labels. Whether you're analyzing product reviews, classifying emails as spam or not, or even detecting sentiments in social media posts, text classification is everywhere. It’s a powerful tool for transforming raw text data into actionable insights.
One of the most remarkable breakthroughs in NLP in recent years is the introduction of BERT (Bidirectional Encoder Representations from Transformers). But here's the thing: while BERT is impressive right out of the box, it becomes even more powerful when you fine-tune it for specific tasks like text classification. Fine-tuning BERT allows you to tailor this pre-trained model to your unique needs and achieve superior performance.
In this guide, we’ll break down the entire process of fine-tuning BERT for text classification. From the very basics of understanding BERT to the technical steps involved in training your own model, we’ll make sure you walk away with all the knowledge you need to master BERT for text classification.
What is BERT and How Does It Work? Before we dive into fine-tuning, let’s first take a moment to understand BERT itself.
At its core, BERT is a pre-trained transformer model developed by Google. It’s designed to understand the intricacies of human language by processing the context of words based on both their left and right sides — hence the term “bidirectional.” Imagine reading a sentence where the meaning of a word depends not only on the words before it but also on the words that come after it. That’s exactly how BERT works.
Why is BERT Special? Prior to BERT, NLP models like word2vec and GloVe were the go-to solutions. These models represented words as static vectors, meaning they could only capture the meaning of words based on their immediate context. BERT, however, uses a transformer architecture and processes all words in a sentence simultaneously (using its bidirectional attention mechanism). This allows BERT to deeply understand context, resulting in better performance on tasks like text classification, named entity recognition, and more.
In short, BERT is like a "language expert" that understands a word in the context of the entire sentence, making it far more accurate than previous models.
Why Fine-Tune BERT for Text Classification? Although BERT is powerful on its own, fine-tuning is essential to adapt the model to specific tasks. Think of BERT as a highly skilled individual who has a vast amount of general knowledge but needs to specialize in a particular field. Fine-tuning allows you to customize BERT’s understanding of language to excel at a specific task, such as text classification.
What Does Fine-Tuning Do? Fine-tuning involves training BERT on your own labeled data, allowing it to adjust its internal weights and biases to recognize patterns in your specific text classification problem. This process helps the model:
Adapt to domain-specific language.
Improve accuracy on the task at hand.
Learn to classify based on task-specific features, like sentiment or topic.
Let’s say you're using BERT for sentiment analysis (classifying whether a sentence is positive, negative, or neutral). Fine-tuning will help BERT become better at understanding the sentiment expressed in your dataset.
Step-by-Step Guide to Fine-Tuning BERT for Text Classification Now that we understand the “why” behind fine-tuning, let’s dive into the how. Here's a step-by-step guide to fine-tuning BERT for text classification.
Step 1: Preparing the Dataset The first step in fine-tuning BERT for text classification is preparing the dataset. BERT requires labeled examples, where each text sample is paired with a category (label).
Dataset Format The dataset should have a format like this:
Text Label "I love this product!" Positive "This is the worst purchase I've ever made." Negative "It’s okay, but not what I expected." Neutral Common Datasets Here are a few commonly used datasets for text classification tasks:
IMDB: A popular dataset for sentiment analysis (positive/negative movie reviews).
20 Newsgroups: A dataset for topic classification.
Once you have your dataset, you’ll need to preprocess the text data to make it ready for training. Typical preprocessing steps include:
Tokenization: Breaking the text into smaller pieces (tokens), such as words or subwords.
Lowercasing: Converting all text to lowercase to avoid distinguishing between the same words in different cases.
Stop Word Removal: Removing common words (e.g., “the,” “and,” “in”) that don’t add much meaning.
Step 2: Setting Up the Environment Next, you’ll need to set up the environment with the necessary libraries.
Required Libraries: Hugging Face Transformers: A library that provides pre-trained models and tools to fine-tune them.
PyTorch or TensorFlow: Deep learning frameworks that work with BERT.
To install Hugging Face’s Transformers library, run:
pip install transformers
If you choose PyTorch, install it via:
pip install torch
Alternatively, if you’re using TensorFlow, install:
pip install tensorflow
Step 3: Loading Pre-Trained BERT
Once your environment is set up, you can load the pre-trained BERT model. Hugging Face’s Transformers library makes this incredibly easy. To load the base BERT model, use the following code:
from transformers import BertTokenizer, BertForSequenceClassification
Load pre-trained BERT tokenizer and model
tokenizer = BertTokenizer.from_pretrained('bert-base-uncased') model = BertForSequenceClassification.from_pretrained('bert-base-uncased', num_labels=2)
Here, 'bert-base-uncased' refers to a smaller version of BERT (Base) that doesn’t distinguish between uppercase and lowercase letters. You can also use 'bert-large-uncased' for a more powerful model, but it requires more computational resources.
Step 4: Fine-Tuning the Model With your model loaded, you can start fine-tuning it. To fine-tune BERT, you’ll need to:
Prepare the data: Tokenize your input text.
Set hyperparameters: Choose a learning rate, batch size, and number of epochs.
Train the model on your dataset.
Here’s a simplified version of the code for fine-tuning BERT using the Trainer class from Hugging Face:
from transformers import Trainer, TrainingArguments
Tokenize the dataset
train_encodings = tokenizer(train_texts, truncation=True, padding=True) val_encodings = tokenizer(val_texts, truncation=True, padding=True)
Define training arguments
training_args = TrainingArguments( output_dir='./results', num_train_epochs=3, per_device_train_batch_size=8, per_device_eval_batch_size=8, warmup_steps=500, weight_decay=0.01, logging_dir='./logs', )
Initialize Trainer
trainer = Trainer( model=model, args=training_args, train_dataset=train_dataset, eval_dataset=val_dataset, )
Fine-tune the model
trainer.train()
During fine-tuning, you’ll adjust hyperparameters like learning rate and batch size to ensure the model converges effectively.
Step 5: Evaluating the Model
After fine-tuning, it’s time to evaluate the model. Common metrics for text classification include:
Accuracy
Precision, Recall, F1 Score
You can evaluate the model using the following code:
Evaluate the model
results = trainer.evaluate()
Print the evaluation results
print(results)
You can also visualize the performance using a confusion matrix, which shows how well the model classifies the text into different categories.
Step 6: Saving and Using the Fine-Tuned Model
Once your model is trained, save it for later use:
Save the model and tokenizer
model.save_pretrained('./fine_tuned_model') tokenizer.save_pretrained('./fine_tuned_model')
Now you can load your fine-tuned model for inference and classify new text:
Load the fine-tuned model
model = BertForSequenceClassification.from_pretrained('./fine_tuned_model') tokenizer = BertTokenizer.from_pretrained('./fine_tuned_model')
Predict sentiment of new text
inputs = tokenizer("I love this product!", return_tensors="pt") outputs = model(**inputs)
Common Pitfalls When Fine-Tuning BERT for Text Classification
Fine-tuning BERT is an exciting process, but there are some common challenges to keep in mind:
Overfitting
Overfitting happens when the model becomes too specialized to the training data and performs poorly on new, unseen data. To prevent overfitting, use early stopping, adjust dropout rates, or employ regularization.
Imbalanced Datasets
If your dataset has more examples of one class than another (e.g., more positive reviews than negative ones), the model may become biased. Techniques like oversampling, undersampling, or using class weights can help.
GPU Limitations
BERT is computationally expensive. If you don’t have access to powerful hardware, consider using smaller models like DistilBERT for faster results.
Advanced Tips and Tricks for Fine-Tuning BERT
For those looking to push their models even further, here are some advanced strategies:
Data Augmentation: Generate synthetic data to improve the diversity of your dataset.
Adversarial Training: Introduce slight perturbations to the input data to make the model more robust.
Multi-task Learning: Fine-tune BERT on multiple related tasks simultaneously to leverage shared knowledge across tasks.
Real-World Use Cases for Fine-Tuned BERT in Text Classification
Fine-tuning BERT is valuable for various NLP applications:
Sentiment Analysis: Classifying customer feedback as positive, negative, or neutral.
Spam Detection: Identifying spam emails or messages.
Named Entity Recognition (NER): Classifying entities (e.g., people, organizations, locations) in a text.
Text Summarization: Creating concise summaries of longer text.
Language Translation: Fine-tuning BERT for bilingual text classification.
Conclusion
Fine-tuning BERT for text classification is a powerful way to leverage a pre-trained model for your specific needs. By carefully preparing your data, setting up the right environment, and following best practices for fine-tuning, you can build highly accurate models for tasks ranging from sentiment analysis to spam detection.
So, what are you waiting for? Start experimenting with your own dataset, and explore the world of NLP with BERT!
FAQs
Q1. What is BERT and why is it important for text classification?
BERT is a transformer-based model that understands text context bidirectionally. It’s crucial for text classification because it captures contextual meaning in a sentence, which helps improve accuracy.
Q2. How does fine-tuning BERT improve its performance on specific tasks?
Fine-tuning adjusts BERT’s pre-trained weights to specialize in a particular task, allowing it to recognize patterns that are specific to your dataset and task.
Q3. What datasets are best suited for fine-tuning BERT for text classification?
Popular datasets include IMDB for sentiment analysis and 20 Newsgroups for topic classification.
Q4. What tools do I need to fine-tune BERT?
You'll need Hugging Face’s Transformers library and either PyTorch or TensorFlow for deep learning.
Q5. Can I fine-tune BERT on a small dataset?
Yes, but you may need to apply techniques like data augmentation to prevent overfitting.
Q6. How do I prevent overfitting when fine-tuning BERT?
Use strategies like early stopping, regularization, and dropout layers to prevent the model from memorizing the training data.
0 notes
Text
搜索引擎SEO优化的最新趋势是什么?TG@yuantou2048
在当今数字化时代,搜索引擎优化(SEO)是企业在线成功的关键因素之一。随着技术的发展和用户行为的变化,SEO策略也需要不断更新以适应新的趋势。以下是当前搜索引擎SEO优化的一些最新趋势:
1. 移动优先:随着越来越多的人使用移动设备进行搜索,Google和其他搜索引擎已经转向了移动优先索引。这意味着网站需要为移动用户提供优秀的体验,包括快速加载时间和易于导航的设计。
2. 语音搜索优化:随着智能音箱和虚拟助手的普及,语音搜索正在迅速增长。优化内容以适应自然语言查询变得越来越重要。确保你的网站内容能够回答常见问题,并采用对话式语言来匹配用户的搜索习惯。
3. 核心网络指标:Google推出了核心网络指标(Core Web Vitals),这是一组衡量网页性能、稳定性和交互性的关键指标。优化这些指标可以提高用户体验,从而提升排名。
4. 增强现实内容:AR技术的应用使得搜索结果更加丰富多样。创建与AR兼容的内容可以帮助吸引更多的流量。
5. 本地化SEO:对于许多行业来说,本地化SEO变得至关重要。通过优化本地关键词和结构化数据,可以提高在本地搜索中的可见性。
6. E-A-T原则:即Expertise, Authoritativeness, Trustworthiness(专业性、权威性和可信度)。确保你的内容具有高质量且有价值的信息,同时保持页面加载速度和响应性。
7. 视频内容:视频已经成为一种重要的内容形式。优化视频元数据和标签,使其更容易被搜索引擎发现并展示给相关用户。
8. 人工智能与机器学习:AI和机器学习算法的进步使得搜索引擎能够更好地理解用户意图。因此,创建高质量、有用的内容变得更加重要。
9. 零点击搜索:很多搜索查询直接在搜索结果页面上显示答案,减少了点击率。因此,创建独特且高质量的内容变得尤为重要。
10. 安全性和隐私保护:随着���们对网络安全的关注增加,拥有HTTPS协议的站点更有可能获得更好的排名。此外,提供安全的浏览环境也是必不可少的。
11. 个性化搜索:搜索引擎正朝着个性化方向发展,根据用户偏好提供定制化结果。利用结构化数据标记可以帮助搜索引擎更好地理解你的网页内容。
12. 可访问性:随着无障碍设计标准的提高,确保网站对所有用户群体都友好将有助于提升排名。
13. 多设备兼容性:随着设备种类的多样化,确保你的网站在各种设备上都能良好运行,并且符合SEO最佳实践。
14. 人工智能:AI驱动的技术如BERT(Bidirectional Encoder Representations from Transformers)等工具帮助改善用户体验,提高转化率。
15. 持续关注用户体验:除了传统的SEO元素外,提供良好的用户体验仍然是一个关键因素。确保网站易于访问并且满足不同能力水平的用户需求成为了一个新趋势。
16. 社交媒体整合:将社交媒体平台作为营销渠道的一部分,可以增加品牌曝光度及互动性。
17. 图像优化:优化图片大小和格式,使它们能够在任何设备上快速加载并提供无缝体验。
18. 链接建设:建立高质量外部链接仍然是有效策略之一。
19. 视频优化:随着短视频平台的增长,优化视频内容变得越来越重要。
10. 内容质量:高质量、原创且有价值的内容仍然是成功的关键。
加飞机@yuantou2048
six mining
cesur mining
0 notes
Text
How Do Search Engine Algorithms Impact SEO Strategies?
Search engine algorithms play a crucial role in determining how websites rank in search engine results pages (SERPs). These algorithms are complex sets of rules and calculations used by search engines like Google, Bing, and Yahoo to analyze, index, and rank web pages based on their relevance and quality. SEO (Search Engine Optimization) strategies must constantly evolve to align with these algorithm updates, ensuring that websites remain visible and competitive in search rankings.
Understanding how search engine algorithms impact SEO strategies is essential for businesses and digital marketers looking to drive organic traffic, improve rankings, and increase online visibility. Let’s explore the key ways in which these algorithms shape modern SEO approaches.
1. Keyword Relevance and Search Intent
Earlier SEO strategies relied heavily on exact-match keywords, but modern search engine algorithms prioritize search intent and relevance. Google’s RankBrain and BERT (Bidirectional Encoder Representations from Transformers) algorithms focus on understanding the context behind a user’s query rather than just matching keywords.
SEO Strategy Adaptation:
Focus on semantic SEO, using natural language and related terms instead of just exact-match keywords.
Optimize content for long-tail keywords and user intent (informational, navigational, or transactional searches).
Use structured data and schema markup to help search engines understand the page’s content better.
2. Content Quality and Relevance
Search engines prioritize high-quality, authoritative, and relevant content that provides real value to users. Algorithms like Google’s Helpful Content Update ensure that pages written purely for SEO purposes (without user value) are ranked lower.
SEO Strategy Adaptation:
Focus on E-E-A-T (Experience, Expertise, Authoritativeness, Trustworthiness) by publishing well-researched, credible, and informative content.
Avoid thin or duplicate content—each page should provide unique value to the reader.
Use multimedia elements like images, videos, and infographics to improve engagement.
3. Backlinks and Domain Authority
Search engines consider backlinks (external links pointing to a website) as a major ranking factor. Google’s PageRank algorithm evaluates the quality and relevance of backlinks, ensuring that only authoritative, credible websites pass link equity.
SEO Strategy Adaptation:
Focus on earning high-quality backlinks from reputable sites instead of quantity.
Use guest blogging, outreach, and PR strategies to secure backlinks from industry leaders.
Avoid spammy or black-hat SEO techniques (such as buying backlinks), as search engines penalize such practices.
#content posting calendar#social media calendar#ad video - digital media calendar#digital marketing online in india#best social marketing agency#digital media calendar services
0 notes
Text
Sure, here is the article formatted according to your specifications:
Google AI SEO Optimization TG@yuantou2048
Google's advancements in artificial intelligence (AI) have significantly impacted various sectors, including search engine optimization (SEO). AI plays a crucial role in enhancing the efficiency and effectiveness of SEO strategies. In this article, we will explore how Google's AI technologies are revolutionizing SEO practices.
The Role of AI in SEO
Artificial Intelligence has become an integral part of Google's search algorithms. With the introduction of machine learning and natural language processing, Google can now better understand user intent and provide more relevant search results. This shift has led to significant changes in SEO practices.
How AI Influences SEO
1. Understanding User Intent: AI algorithms help Google understand the context and intent behind search queries. This means that content creators need to focus on creating high-quality, informative content that addresses users' needs rather than just targeting specific keywords.
2. RankBrain and BERT: Google's RankBrain and BERT (Bidirectional Encoder Representations from Transformers) use AI to interpret the meaning behind search queries. These tools help Google deliver more accurate and personalized results, making it essential for SEO professionals to create content that aligns with what users are truly looking for, not just keyword matching.
2. Content Quality: AI-driven systems prioritize content quality over keyword stuffing. Content that provides genuine value and answers user queries effectively tends to rank higher. This shift emphasizes the importance of creating valuable, engaging content that resonates with the audience.
3. Technical SEO: AI also aids in technical SEO by identifying and addressing issues such as site speed, mobile-friendliness, and overall user experience. Websites that offer a seamless browsing experience and quick load times are more likely to rank well.
4. Voice Search Optimization: With the rise of voice searches, optimizing for conversational queries has become crucial. Understanding and catering to these types of searches can significantly improve rankings.
5. Link Analysis: AI helps in analyzing backlinks and their relevance. High-quality, authoritative links from reputable sources are given more weight, emphasizing the importance of building a robust backlink profile and ensuring that websites are optimized for both desktop and mobile devices.
6. Structured Data: Implementing structured data (like schema markup) helps search engines understand the content better, leading to improved visibility and engagement metrics.
7. Local SEO: Local SEO strategies benefit greatly from AI. By understanding the nuances of local search queries, businesses can enhance their local SEO efforts.
8. Personalization: AI enables Google to personalize search results based on user behavior and preferences, making it vital to ensure your website is optimized for voice search and featured snippets. Structured data and schema markup play a critical role in improving visibility in rich snippets and featured snippets.
9. Machine Learning: Machine learning algorithms can predict user behavior and preferences, allowing for more precise and contextually relevant results. Ensuring your website is optimized for voice search and mobile-first indexing is key.
10. User Experience: User experience (UX) is another area where AI plays a pivotal role. Optimizing for voice search and ensuring your content is easily digestible and valuable.
In conclusion, leveraging AI in SEO requires a strategic approach. By focusing on user intent, content quality, and technical optimizations, businesses can stay ahead in the ever-evolving digital landscape. Stay tuned for more insights on how AI continues to shape the future of SEO.
Feel free to let me know if you need any further adjustments!
加飞机@yuantou2048
EPP Machine
相关推荐
0 notes
Text
How AI is Changing SEO: What Businesses Need to Know in 2025
SEO (Search Engine Optimization) has always been an evolving field, yet Artificial Intelligence (AI) is rapidly reshaping it more than ever before. By 2025, companies that don't adapt to AI-powered SEO strategies could fall behind. With improved algorithms for content production to predictive analytics that predict analytics AI has altered how websites are ranked on search engines such as Google.
If you are curious to understand how AI is impacting SEO, and what steps must be taken in order to keep up, this blog provides all the answers.
1. AI and Search Algorithms Are Smarter Than Ever:
Google's AI-driven algorithm has become highly sophisticated over the years, taking into account user context, intent and quality content as opposed to simply keyword searches. Recent AI updates include:
Google RankBrain & BERT: Analyzing User Intent
Google's RankBrain and BERT (Bidirectional Encoder Representations derived from Transformers) AI models have transformed how search engines interpret search queries, helping Google recognize natural speech. Businesses should aim to produce content that is relevant, conversational and useful if they want their brand or products or services to stand out in this highly competitive space.
MUM (Multitask Unified Model) Is Converging on Success:
Google's MUM algorithm leverages artificial intelligence (AI) to advance SEO by simultaneously recognizing text images videos and multiple languages simultaneously. Businesses should utilize structured information in their content that goes beyond text such as videos information graphics infographics etc. for increased visibility.
What This Means for Businesses:
Focus on Semantic SEO rather than simply keyword stuffing. Utilize structured information (Schema Markup) to assist search engines in understanding your content better, and optimize for voice-search since AI recognizes conversations more readily than written text does.
2. AI-Powered Content Creation & Optimization:
Formulating high-quality, SEO-friendly and relevant content has never been more critical. Though AI tools such as ChatGPT Jasper and Copy.ai can generate content, Google still prefers human-generated original and valuable pieces over automated ones.
How AI Contributes to Content Optimization:
AI tools analyze the most successful content, providing recommendations to improve readability, keyword use and engagement. Natural Language Processing (NLP) tools assist businesses in producing content aligned with Google's AI algorithms.
Artificial Intelligence-powered Content Gap Analysis can assist in identifying those topics for which you are competing but do not meet them in full.
Human creativity cannot be replaced. AI may assist with researching, structuring and suggesting changes, but companies must always incorporate human elements to maintain authenticity.
Tips for Success: For effective SEO-optimized content that ranks well in search results, working with an established SEO firm like Wave Riser in Dubai ensures AI-compatibility while still offering high levels of engagement.
3. AI and UX Experience as New Ranking Factors:
Since Google upgraded their core Web Vitals upgrade, User Experience (UX) is becoming an important SEO factor. Artificial Intelligence is also becoming more and more of an influencer when it comes to optimizing UX.
Search results that are personalized based on user behavior: improving website speed and mobile friendliness; expanding visual and vocal search capabilities for search; AI-powered personalization in Search.
Google's AI makes adjustments based on past behaviors, the location of their search, and even preferences of each individual user. For example, if a resident of Dubai searches for "best marketing agency", Google will prioritize companies with strong local SEO as top contenders.
Businesses must ensure their websites are optimized for searches based on location and ensure users enjoy an uninterrupted experience across platforms.
What This Means for You:
Increase page speed using AI-powered tools such as PageSpeed Insights; optimise mobile-friendliness by prioritising mobile-first indexing with Google.
Optimized for voice search to make life simpler for Artificial Intelligence-powered Assistants such as Alexa, Siri, or Google Assistant will become increasingly in demand by 2025.
Wave Riser, an SEO Agency in Dubai and specialising in AI-powered SEO strategies that optimize user experience while increasing ranking positions, can assist businesses looking to boost both UX and SEO.
4. Artificial Intelligence for Keyword Research and Predictive SEO:
Artificial Intelligence is revolutionizing keyword analysis. Instead of solely considering competition and volume of searches, AI-powered SEO tools such as SEMrush Ahrefs and SurferSEO provide predictive analyses based on real data that predict future trends in search.
AI-Driven Keyword Research Tools:
AI can help suggest Long-Tail Keywords that have higher conversion chances; Forecast seasonal search trends (e.g. "best Ramadan marketing strategies in Dubai") Explore competitors to identify hidden ranking opportunities
Predictive SEO Has Emerged as a Key Weapon:
Predictive SEO uses artificial intelligence (AI) to predict search trends before they reach their peak, helping businesses stay ahead of competitors by optimizing their websites for tomorrow.
Actionable Tip: Utilize AI-powered keyword research tools as well as invest in long-term content strategies so your rankings remain secure in the future.
5. Artificial Intelligence and Link Building for Improved Outreach Strategies:
Backlinks remain an integral component of ranking; however, artificial intelligence (AI) tools are changing how businesses think about linking strategies. AI tools help by:
Locating high-authority backlinks: Automating outreach efforts towards influencers and bloggers; analysing potentially damaging links which could hurt SEO performance.
Google values quality links from reputable sources over illegal or dubious ones.
Pro Tip: Work with one of Dubai's most experienced SEO firms such as Wave Riser to implement an algorithm-driven, ethical link building strategy designed to increase organic rankings and improve organic positioning.
Final Thoughts on AI in SEO
AI's aim is not to replace SEO but instead enhance it. Companies that harness AI power for data-driven strategies and human creativity will dominate search results by 2025.
If you want to stay ahead of the competition in this era of artificial intelligence (AI), employing experts such as Wave Riser's SEO service based in Dubai may help. They specialize in using AI while guaranteeing long-term SEO success.
Are you seeking to ensure the future success of your SEO strategies? Get in touch with Wave Riser immediately for artificial intelligence-powered solutions!
0 notes
Text
How AI is Changing SEO: The Future of Search Optimization
Search Engine Optimization (SEO) has always been an evolving field, adapting to changes in search engine algorithms and user behavior. With the rapid advancement of Artificial Intelligence (AI), SEO is undergoing one of its most transformative shifts yet. AI is not only changing how search engines understand and rank content but also how marketers optimize websites, create content, and improve user experience.
In this article, we will explore the various ways AI is revolutionizing SEO and what businesses and digital marketers should do to stay ahead.
1. AI-Powered Search Algorithms
Search engines, especially Google, have been integrating AI to improve their ability to understand and rank web pages. Major AI-driven updates have included:
Google RankBrain – Introduced in 2015, RankBrain uses machine learning to process search queries and deliver the most relevant results based on user intent.
Google BERT (Bidirectional Encoder Representations from Transformers) – Launched in 2019, BERT helps Google understand the context and meaning behind words in a search query, making it easier to match users with relevant content.
Google MUM (Multitask Unified Model) – MUM, introduced in 2021, is 1,000 times more powerful than BERT and can analyze and understand text, images, and even video to improve search accuracy.
These AI-driven updates mean that keyword stuffing and traditional SEO tactics are becoming less effective. Instead, search engines prioritize high-quality, relevant, and authoritative content.
2. AI in Keyword Research and Content Optimization
AI-powered SEO tools have significantly improved keyword research and content optimization. Platforms like SurferSEO, Clearscope, and Frase use AI to analyze top-performing content and provide data-driven recommendations. AI can:
Identify keyword opportunities by analyzing search trends and competitor content.
Suggest content structures that align with Google's ranking factors.
Optimize content for user intent, ensuring that it meets the expectations of searchers.
Instead of blindly inserting keywords, businesses must focus on semantic search, where AI understands the meaning behind words and phrases, helping content rank based on topic relevance rather than just keyword density.
3. AI and Content Creation
AI-generated content is becoming increasingly sophisticated. Tools like ChatGPT, Jasper, and Copy.ai assist marketers by:
Generating blog posts, product descriptions, and ad copy quickly and efficiently.
Creating engaging meta titles and descriptions to improve click-through rates.
Personalizing content based on user behavior and search intent.
While AI can assist with content generation, human oversight remains crucial. Google's recent Helpful Content Updates emphasize that content should be original, valuable, and written for humans rather than just for search engines.
4. AI-Powered Voice and Visual Search Optimization
The rise of voice search through Google Assistant, Siri, and Alexa has changed the way people search online. AI plays a significant role in processing natural language queries, making it essential for businesses to optimize for voice search by:
Using conversational language and long-tail keywords.
Structuring content in a question-and-answer format.
Focusing on local SEO, as many voice searches are location-based (e.g., “best Italian restaurant near me”).
Similarly, AI has improved visual search through tools like Google Lens and Pinterest Lens, allowing users to search using images instead of text. Optimizing images with descriptive alt text, proper file names, and structured data can improve rankings in visual search results.
5. AI and Predictive SEO
AI’s ability to analyze large datasets enables predictive SEO, where marketers can anticipate upcoming search trends. AI-powered tools like Google Trends, SEMrush, and Ahrefs provide insights into:
Which topics will gain traction in the near future.
Seasonal trends that influence search behavior.
The impact of algorithm updates on rankings.
By leveraging predictive SEO, businesses can create content proactively rather than reactively, staying ahead of competitors.
6. AI in Link Building and Technical SEO
AI is also streamlining link-building and technical SEO by:
Identifying high-authority backlink opportunities through automated tools.
Analyzing website structure and fixing issues like broken links, slow-loading pages, and duplicate content.
Generating schema markup to improve rich snippets and enhance search visibility.
AI-powered chatbots and automation tools can also improve website engagement, which indirectly influences SEO rankings.
7. AI and Personalized Search Experiences
Search engines are moving towards personalized search experiences, where AI tailors results based on a user’s:
Search history
Location
Device type
Browsing behavior
This means that SEO strategies need to be more user-centric than ever. Optimizing for user experience (UX), mobile-friendliness, and page speed is crucial to ensuring a website ranks well in personalized search results.
Conclusion: Adapting to AI-Driven SEO
AI is changing SEO in ways that demand a shift in strategy. Instead of traditional keyword stuffing and link-building tactics, businesses should focus on:
✅ Creating high-quality, intent-driven content ✅ Optimizing for voice and visual search ✅ Leveraging AI-powered tools for insights and automation ✅ Prioritizing user experience and personalized search
SEO professionals who embrace AI and integrate it into their strategies will have a competitive edge in the evolving digital landscape.
For more details on our products and services, please feel free to visit us at: Link Building Services, Google Adwords, Google Local Business, Web Analytics Service & Article Submission Services.
Please feel free to visit us at:https://webigg.com/
0 notes
Text
Fine-Tuning BERT for NLP Projects: Best Practices Guide
1. Introduction Brief Explanation Fine-tuning BERT (Bidirectional Encoder Representations from Transformers) allows you to adapt this powerful pre-trained model to your specific NLP tasks, enhancing performance on your dataset. This guide will walk you through the process, emphasizing best practices and troubleshooting common issues. What Readers Will Learn How to fine-tune BERT for custom…
0 notes
Text
What Are the Key Steps in AI Agent Platform Development?

Artificial Intelligence (AI) agents have become an integral part of modern technology, revolutionizing industries by performing complex tasks autonomously. From customer service bots to advanced predictive systems in healthcare, AI agents are increasingly present in various sectors. Developing an AI agent platform is a challenging yet rewarding endeavor that requires a clear understanding of the technical, operational, and business aspects of AI. In this blog, we’ll delve into the key steps involved in developing a robust AI agent platform that can serve as the foundation for various AI-driven applications.
Step 1: Define the Purpose and Scope of the AI Agent
The first and foremost step in developing an AI agent platform is defining its purpose. AI agents can range from simple task automation bots to highly sophisticated systems that simulate human intelligence. Understanding the end goals of the platform is crucial, as it will guide the design and technology stack decisions throughout the development process. The scope should include:
Target Audience: Who will be the end-users? Are they businesses, consumers, or specific industries?
Tasks and Functionality: What specific tasks should the AI agent perform? These can include natural language processing, machine learning tasks, decision-making, or data analysis.
Integration: Will the AI agent interact with other systems? Defining integration requirements with existing databases, CRMs, or third-party tools is essential.
Autonomy Level: How independent should the agent be? Some AI agents require human oversight, while others may be fully autonomous.
Having a clear, well-defined purpose ensures the platform is built with a specific set of capabilities, saving time and resources during development.
Step 2: Choose the Right AI Technologies
The development of an AI agent platform requires selecting the right tools, frameworks, and technologies. The technology stack will largely depend on the intended use case, but the following technologies are typically involved:
Machine Learning (ML): For predictive analytics, anomaly detection, and learning from data, machine learning models such as supervised, unsupervised, and reinforcement learning may be employed.
Natural Language Processing (NLP): If the platform involves language understanding, technologies like GPT (Generative Pre-trained Transformer) or BERT (Bidirectional Encoder Representations from Transformers) are popular choices.
Computer Vision: In cases where the AI agent needs to interpret visual data, computer vision models like CNN (Convolutional Neural Networks) come into play.
Robotic Process Automation (RPA): For automating repetitive tasks across various applications, RPA tools might be used.
Frameworks and Libraries: Popular AI frameworks like TensorFlow, PyTorch, and Keras for ML, OpenAI’s GPT for language models, or custom neural network architectures can be employed based on the project’s requirements.
Choosing the right technology is critical for ensuring that the platform meets performance standards and can scale as needed.
Step 3: Data Collection and Preprocessing
For AI agents to function effectively, they need access to large datasets that can train them. This is one of the most significant challenges in AI development, as the quality and diversity of the data play a pivotal role in shaping the accuracy and effectiveness of the agent. The data collection process can include:
Gathering Data: Whether from open datasets, APIs, or user-generated content, the data needs to be relevant to the agent’s functionality. For instance, a customer service AI bot would require conversational data.
Data Preprocessing: Raw data often contains noise and inconsistencies. Cleaning, transforming, and organizing data into a usable format is essential for the AI model’s performance.
Data Labeling: For supervised learning models, data labeling is critical. This step involves annotating datasets to train the models to recognize patterns and make decisions.
Data Privacy and Security: Ensuring data privacy compliance (such as GDPR) and securing sensitive information is paramount when handling user or client data.
A comprehensive data collection and preprocessing pipeline can significantly enhance the AI agent’s learning capabilities, enabling it to perform tasks with higher accuracy.
Step 4: Model Selection and Training
Once the data is ready, the next step is to select appropriate machine learning models and begin the training process. The choice of model depends on the agent’s intended task. For instance:
Supervised Learning: If the agent needs to classify data or make predictions, supervised learning models (e.g., Decision Trees, Random Forests, or Support Vector Machines) may be used.
Unsupervised Learning: For clustering or anomaly detection, unsupervised learning models like K-Means or DBSCAN may be better suited.
Reinforcement Learning: If the AI agent will interact with its environment and improve over time, reinforcement learning (RL) algorithms like Q-Learning or Deep Q Networks (DQN) can be used.
Transfer Learning: If there is a lack of data, leveraging pre-trained models (e.g., GPT-3, BERT) and fine-tuning them for specific use cases can be an efficient way to kickstart training.
Training involves feeding the model with data, allowing it to learn patterns, and fine-tuning parameters to optimize the agent’s performance. This phase can be time-consuming and computationally expensive, especially for deep learning models that require massive datasets.
Step 5: Evaluation and Testing
After training the AI model, it’s essential to evaluate its performance rigorously. This phase helps identify any flaws or weaknesses before deploying the agent. Evaluation can be done using:
Accuracy Metrics: Depending on the agent’s task, metrics like precision, recall, F1-score, or ROC-AUC might be used to measure the model's accuracy.
A/B Testing: Running different versions of the AI agent with varying algorithms or models to compare which performs best.
Simulations: For AI agents that interact with the real world, simulated environments can be used to test how the agent behaves in different scenarios.
User Testing: If the platform is customer-facing, conducting beta tests and gathering user feedback can reveal usability issues and performance shortcomings.
Continuous testing and iteration ensure that the AI agent operates optimally under different conditions.
Step 6: Integration with Existing Systems
Integrating the AI agent into an existing infrastructure is crucial for ensuring smooth operations. This step involves:
APIs and SDKs: Developing APIs or using existing ones to enable seamless communication between the AI agent and other systems like CRMs, ERP tools, or customer-facing applications.
Backend Integration: Ensuring that the AI agent can communicate with databases, process data, and provide real-time insights if necessary.
Frontend Integration: If the AI agent has a user-facing component (e.g., a chatbot or voice assistant), ensuring it integrates smoothly with the frontend interface is key to delivering a seamless user experience.
Proper integration ensures that the AI agent can perform its tasks in conjunction with other business operations, creating a more efficient and connected system.
Step 7: Deployment and Continuous Monitoring
Once everything is in place, the AI agent can be deployed to the production environment. However, deployment is not the end of the development process. Continuous monitoring is essential to ensure the system remains effective and performs well as it interacts with real users. Some tasks involved in deployment and monitoring include:
Deployment Pipelines: Using CI/CD (Continuous Integration and Continuous Deployment) tools to automate the deployment of new features or updates to the AI agent.
Monitoring: Keeping track of the AI agent’s performance in real-time, monitoring for errors, performance bottlenecks, or failures in real-world applications.
Iterative Updates: Based on user feedback and performance metrics, the AI agent may need periodic updates, retraining, and fine-tuning to improve its performance.
It’s essential to maintain a feedback loop to adapt the AI agent to changing environments and ensure it remains relevant over time.
Step 8: Ethical Considerations and User Privacy
Ethical considerations must be integrated into every stage of AI agent development. Some key ethical aspects include:
Bias Mitigation: Ensuring the AI agent doesn’t inherit biases from the data it is trained on.
Transparency: Providing users with a clear understanding of how the AI agent operates and makes decisions.
Privacy and Security: Complying with privacy laws, securing user data, and ensuring the AI agent does not misuse sensitive information.
Building ethical AI systems can help foster trust with users and avoid legal and reputational risks.
Conclusion
Developing an AI agent platform is a complex yet rewarding task that requires a multi-faceted approach. From defining the purpose and choosing the right technologies to continuously monitoring the agent’s performance, each step is critical for creating a successful platform. By following these key steps, developers can build AI-powered systems that are capable, ethical, and scalable, paving the way for innovation across a wide range of industries.
0 notes
Text
En este curso, se presentan la arquitectura de transformadores y el modelo de Bidirectional Encoder Representations from Transformers (BERT). Aprenderás sobre los componentes principales de la arquitectura de transformadores, como el mecanismo de autoatención, y cómo se usa para crear el modelo BERT. También aprenderás sobre las diferentes tareas para las que puede usarse BERT, como la clasificación de texto, la respuesta de preguntas y la inferencia de lenguaje natural. Tardarás aproximadamente 45 minutos en completar este curso.
0 notes