#HPCApplications
Explore tagged Tumblr posts
Text
FPGA vs Microcontroller: The Ultimate Programmable Showdown

FPGA vs Microcontroller
Two types of integrated circuits (ICs) that are frequently contrasted are field programmable gate arrays (FPGAs) and microcontroller units (MCUs). Embedded systems and digital design are two typical applications for these ICs. It is possible to think of FPGA vs microcontroller as “small computers” that may be included into smaller gadgets and bigger systems.
Programmability and processing power are the main distinctions between FPGA and microcontroller as processors. FPGAs are more costly even though they have greater power and versatility. Microcontrollers are less expensive, but they also offer less customisation. Microcontrollers are quite powerful and affordable in many applications. Nonetheless, FPGAs are required for some demanding or evolving applications, such as those that need parallel processing.
FPGAs have hardware reprogrammability, in contrast to microcontrollers. Because of their distinctive design, users are able to alter the chip’s architecture to suit the needs of the application. Microcontrollers can only read one line of code, but FPGAs can handle many inputs. An FPGA can be programmed like a microcontroller, but not vice versa.
The FPGA is field-programmable gate array
FPGAs from Xilinx debuted in 1985. Processing power and adaptability are their hallmarks. Therefore, they are recommended for many DSP, prototyping, and HPC applications.
FPGAs, unlike ASICs, can be customised and reconfigured “in the field,” after production. FPGAs’ primary feature is customisation, but they also require programmability. FPGAs must be configured in verilog or VHDL, unlike ASICs. Programming an FPGA requires expertise, which increases costs and delays adoption. Generally, FPGAs need to be set upon startup, however some do have non-volatile memory that can save programming instructions after the device is turned down.
FPGA advantages
FPGAs are nonetheless helpful in applications that demand high performance, low latency, and real-time adaptability in spite of these difficulties. FPGAs work especially effectively in applications that need the following:
Quick prototyping
FPGAs may be readily configured into a variety of customised digital circuit types, avoiding the need for expensive and time-consuming fabrication processes and enabling faster deployments, evaluations, and modifications.
Hardware-based accelerated
The FPGA’s parallel processing capabilities are advantageous for demanding applications. For computationally demanding applications like machine learning algorithms, cryptography, and signal processing, FPGAs may provide considerable performance gains.
Personalisation
FPGAs are a versatile hardware option that are simple to customise to fit the demands of a given project.
Durability
Given that FPGAs may be updated and modified to meet changing project demands and technology standards, FPGA-based designs may have a longer hardware lifecycle.
FPGA parts
FPGAs are made up of a variety of programmable logic units connected by a programmable routing fabric in order to provide reconfigurability. The following are the key parts of a standard FPGA:
Blocks of configurable logic (CLBs)
In addition to providing computation capabilities, CLBs may have a limited number of simple logic components, including flip-flops for data storage, multiplexors, logic gates, and small look-up tables (LUTs).
Interconnects with programming capabilities
These linkages, which consist of wire segments connected by electrically programmable switches, offer routing channels between the various FPGA resources, enabling the development of unique digital circuits and a variety of topologies.
Blocks for I/O (IOBs)
Input output (I/O) blocks facilitate the interaction between an FPGA and other external devices by enabling the FPGA to receive data from and operate peripherals.
FPGA applications
Due to its versatility, FPGAs are used in many industries.
Aerospace and defence
FPGAs are the ideal option for image processing, secure communications, radar systems, and radar systems because they provide high-speed parallel processing that is useful for data collecting.
Systems of industrial control (ICS)
Power grids, oil refineries, and water treatment plants are just a few examples of the industrial control systems that use FPGAs, which are easily optimised to match the specific requirements of different industries. FPGAs can be utilised to create several automations and hardware-based encryption features for effective cybersecurity in these vital industries.
ASIC creation
New ASIC chips are frequently prototyped using FPGAs.
Automotive
FPGAs are ideally suited for advanced driving assistance systems (ADAS), sensor fusion, and GPS due to their sophisticated signal processing capabilities.
Information hubs
By optimising high-bandwidth, low-latency servers, networking, and storage infrastructure, FPGAs enhance the value of data centres.
Features of FPGAs
Processor core: Logic blocks that can be configured
Memory: Interface for external memory
auxiliary parts: Modifiable input/output blocks
Programming: Hardware description language (VHDL, Verilog) is used in programming.
Reconfigurability: Extremely reprogrammable and reconfigurable logic
What is a microcontroller?
Microcontrollers are a kind of small, pre-assembled ASIC that have an erasable programmable read-only memory (EPROM) for storing bespoke programmes, memory (RAM), and a processor core (or cores). Microcontrollers, sometimes referred to as “system-on-a-chip (SoC)” solutions, are essentially tiny computers combined into a single piece of hardware that may be utilised separately or in larger embedded systems.
Because of their affordable accessibility, hobbyists and educators prefer consumer-grade microcontrollers, including the Arduino Starter Kit and Microchip Technology PIC, which can be customised using assembly language or mainstream programming languages (C, C++). Microcontrollers are frequently used in industrial applications and are also capable of managing increasingly difficult and important jobs. However, in more demanding applications, a microcontroller’s effectiveness may be limited by reduced processing power and memory resources.
Benefits of microcontrollers
Microcontrollers have numerous benefits despite their drawbacks, such as the following:
Small-scale layout
Microcontrollers combine all required parts onto a single, compact chip, making them useful in applications where weight and size are important considerations.
Energy effectiveness
Because they utilise little power, microcontrollers are perfect for battery-powered gadgets and other power-constrained applications.
Economical
By delivering a full SoC solution, microcontrollers reduce peripheral needs.All-purpose, low-cost microcontrollers can significantly cut project costs.
Adaptability
While less flexible than FPGA and microcontroller can be programmed for many applications. Software can change, update, and tune microcontrollers, but hardware cannot.
Parts of microcontrollers
Compact and capable, self-contained microcontrollers are an excellent option when reprogrammability is not a top concern. The essential parts of a microcontroller are as follows:
CPU, or central processing unit
The CPU, sometimes known as the “brain,” executes commands and manages processes.
Recall
Non-volatile memory (ROM, FLASH) stores the microcontroller’s programming code, while volatile memory (RAM) stores temporary data that could be lost if the system loses power.
Auxiliary
Depending on the application, a microcontroller may have communication protocols (UART, SPI, I2C) and I/O interfaces like timers, counters, and ADCs.
Use cases for microcontrollers
Small, inexpensive, and non-volatile microcontrollers, in contrast to FPGAs, are widely used in contemporary electronics and are typically employed for certain purposes, such as the following:
Vehicle systems
Airbag deployment, engine control, and in-car infotainment systems all require microcontrollers.
End-user devices
Smartphones, smart TVs, and other household appliances especially IoT-connected ones use microcontrollers.
Automation in industry
Industrial applications include process automation, machinery control, and system monitoring are ideal uses for microcontrollers.
Medical equipment
Microcontrollers are frequently used in life-saving equipment including blood glucose monitors, pacemakers, and diagnostic instruments.
Features of a microcontroller
Central processing unit: Unchanged CPU Memory: ROM/Flash and integrated RAM Auxiliary parts: Integrated I/O interfaces for Software (C, Assembly) Programming Limited reconfigurability; firmware upgrades
Important distinctions between microcontrollers and FPGAs
A number of significant distinctions between FPGA and microcontroller should be taken into account when comparing them, including developer requirements, hardware architecture, processing power, and capabilities.
Hardware configuration
FPGA: Easy-to-customize programmable logic blocks and interconnects for digital circuits. Microcontroller: A fixed-architecture microcontroller contains a CPU, memory, and peripherals.
Capabilities for processing
FPGA: Multiple simultaneous processes are made possible by advanced parallel processing. Microcontroller: Capable of handling only one instruction at a time, microcontrollers are made for sequential processing.
Power usage
FPGA: Power consumption is usually higher than that of microcontrollers. Microcontroller: Designed to use less power, ideal for applications that run on batteries.
Coding
FPGA: Configuring and debugging this device requires specific understanding of hardware description languages. Microcontroller: Software development languages such as Javascript, Python, C, C++, and assembly languages can be used to programming microcontrollers.
Price
FPGA: FPGA hardware offers more power but comes with a higher price tag due to its higher power consumption and need for specialised programming abilities. It also requires advanced expertise. Microcontroller: Typically, a less expensive option that is readily available off the shelf, uses less power, and supports more widely used programming languages.
Flexibility
FPGA: Compared to microcontrollers, FPGAs are much more flexible and enable hardware customisation. Microcontroller: Compared to FPGAs, microcontrollers only provide surface-level customisation, despite being well-suited for a wide range of applications.
Examine the infrastructure solutions offered by IBM
Whether you’re searching for a small, affordable microcontroller or a flexible, potent FPGA processor, think about how IBM’s cutting-edge infrastructure solutions may help you grow your company. The new IBM FlashSystem 5300 offers enhanced cyber-resilience and performance. New IBM Storage Assurance makes storage ownership easier and supports you in resolving IT lifecycle issues.
Read more on Govindhtech.com
#microcontroller#digitaldesign#hardware#HPCapplications#cryptography#datastorage#cybersecurity#cpu#smartphones#Softwaredevelopment#news#technews#technology#technologynews#technologytrends#govindhtech
0 notes
Text
Aurora Supercomputer Sets a New Record for AI Tragic Speed!
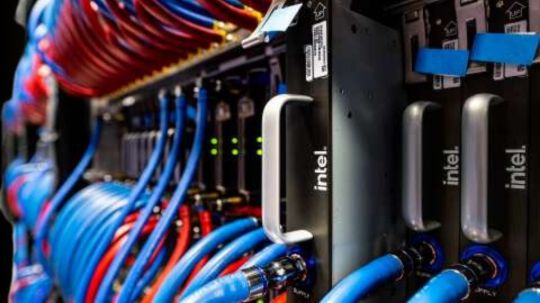
Intel Aurora Supercomputer
Together with Argonne National Laboratory and Hewlett Packard Enterprise (HPE), Intel announced at ISC High Performance 2024 that the Aurora supercomputer has broken the exascale barrier at 1.012 exaflops and is now the fastest AI system in the world for AI for open science, achieving 10.6 AI exaflops. Additionally, Intel will discuss how open ecosystems are essential to the advancement of AI-accelerated high performance computing (HPC).
Why This Is Important:
From the beginning, Aurora was intended to be an AI-centric system that would enable scientists to use generative AI models to hasten scientific discoveries. Early AI-driven research at Argonne has advanced significantly. Among the many achievements are the mapping of the 80 billion neurons in the human brain, the improvement of high-energy particle physics by deep learning, and the acceleration of drug discovery and design using machine learning.
Analysis
The Aurora supercomputer has 166 racks, 10,624 compute blades, 21,248 Intel Xeon CPU Max Series processors, and 63,744 Intel Data Centre GPU Max Series units, making it one of the world’s largest GPU clusters. 84,992 HPE slingshot fabric endpoints make up Aurora’s largest open, Ethernet-based supercomputing connection on a single system.
The Aurora supercomputer crossed the exascale barrier at 1.012 exaflops using 9,234 nodes, or just 87% of the system, yet it came in second on the high-performance LINPACK (HPL) benchmark. Aurora supercomputer placed third on the HPCG benchmark at 5,612 TF/s with 39% of the machine. The goal of this benchmark is to evaluate more realistic situations that offer insights into memory access and communication patterns two crucial components of real-world HPC systems. It provides a full perspective of a system’s capabilities, complementing benchmarks such as LINPACK.
How AI is Optimized
The Intel Data Centre GPU Max Series is the brains behind the Aurora supercomputer. The core of the Max Series is the Intel X GPU architecture, which includes specialised hardware including matrix and vector computing blocks that are ideal for AI and HPC applications. Because of the unmatched computational performance provided by the Intel X architecture, the Aurora supercomputer won the high-performance LINPACK-mixed precision (HPL-MxP) benchmark, which best illustrates the significance of AI workloads in HPC.
The parallel processing power of the X architecture excels at handling the complex matrix-vector operations that are a necessary part of neural network AI computing. Deep learning models rely heavily on matrix operations, which these compute cores are essential for speeding up. In addition to the rich collection of performance libraries, optimised AI frameworks, and Intel’s suite of software tools, which includes the Intel oneAPI DPC++/C++ Compiler, the X architecture supports an open ecosystem for developers that is distinguished by adaptability and scalability across a range of devices and form factors.
Enhancing Accelerated Computing with Open Software and Capacity
He will stress the value of oneAPI, which provides a consistent programming model for a variety of architectures. OneAPI, which is based on open standards, gives developers the freedom to write code that works flawlessly across a variety of hardware platforms without requiring significant changes or vendor lock-in. In order to overcome proprietary lock-in, Arm, Google, Intel, Qualcomm, and others are working towards this objective through the Linux Foundation’s Unified Acceleration Foundation (UXL), which is creating an open environment for all accelerators and unified heterogeneous compute on open standards. The UXL Foundation is expanding its coalition by adding new members.
As this is going on, Intel Tiber Developer Cloud is growing its compute capacity by adding new, cutting-edge hardware platforms and new service features that enable developers and businesses to assess the newest Intel architecture, innovate and optimise workloads and models of artificial intelligence rapidly, and then implement AI models at scale. Large-scale Intel Gaudi 2-based and Intel Data Centre GPU Max Series-based clusters, as well as previews of Intel Xeon 6 E-core and P-core systems for certain customers, are among the new hardware offerings. Intel Kubernetes Service for multiuser accounts and cloud-native AI training and inference workloads is one of the new features.
Next Up
Intel’s objective to enhance HPC and AI is demonstrated by the new supercomputers that are being implemented with Intel Xeon CPU Max Series and Intel Data Centre GPU Max Series technologies. The Italian National Agency for New Technologies, Energy and Sustainable Economic Development (ENEA) CRESCO 8 system will help advance fusion energy; the Texas Advanced Computing Centre (TACC) is fully operational and will enable data analysis in biology to supersonic turbulence flows and atomistic simulations on a wide range of materials; and the United Kingdom Atomic Energy Authority (UKAEA) will solve memory-bound problems that underpin the design of future fusion powerplants. These systems include the Euro-Mediterranean Centre on Climate Change (CMCC) Cassandra climate change modelling system.
The outcome of the mixed-precision AI benchmark will serve as the basis for Intel’s Falcon Shores next-generation GPU for AI and HPC. Falcon Shores will make use of Intel Gaudi’s greatest features along with the next-generation Intel X architecture. A single programming interface is made possible by this integration.
In comparison to the previous generation, early performance results on the Intel Xeon 6 with P-cores and Multiplexer Combined Ranks (MCR) memory at 8800 megatransfers per second (MT/s) deliver up to 2.3x performance improvement for real-world HPC applications, such as Nucleus for European Modelling of the Ocean (NEMO). This solidifies the chip’s position as the host CPU of choice for HPC solutions.
Read more on govindhtech.com
#aurorasupercomputer#AISystem#AIaccelerated#highperformancecomputing#aimodels#machinelearning#inteldatacentre#intelxeoncpu#HPC#MemoryAccess#HPCApplications#AIComputing#softwaretools#intelgaudi2#IntelXeon#aibenchmark#news#technews#technology#technologynews#technologytrends#govindhtech
0 notes
Text
Azure high performance computing enhances Surface products

Azure HPC
For HPC applications, Azure high performance computing (HPC) offers a full range of networking, storage, and processing capabilities along with workload orchestration services. In comparison to on-premises alternatives, Azure delivers comparable price/performance thanks to its purpose-built HPC infrastructure, solutions, and optimized application services. provides further advantages for high-performance computing. Azure also comes with state-of-the-art machine-learning algorithms to facilitate more intelligent decision making and intelligent simulations.
Cost-controlled performance that is optimized
Utilise every bit of your CPU, GPU, FPGA, and fast connection capacity to cut down on the time it takes to complete tasks from days to minutes.
Platform of production calibre
Azure is the cloud that simply works, with strong HPC stability, data security, and worldwide regulatory compliance.
Complete workflow flexibility
Create and maintain HPC clusters for your own personal usage alone, allowing cloud-based end-to-end application lifecycle management.
Including intelligence
With integrated DevOps, autoscaling cloud computing, and automated machine learning, you can create and train new AI models more quickly.
Examine Azure high performance computing options according to industry and application
Azure high performance computing can help developers, engineers, scientists, and researchers achieve new heights in their domains.
Automobile
With a highly secure infrastructure, it is possible to simulate every facet of vehicle engineering at a reasonable cost and scale.
Banking operations
Meet regulatory obligations with assurance thanks to a sophisticated and adaptable risk modelling infrastructure. As required, increase your capacity, and only pay for what you really need.
Vitality
Optimise the exploration, appraisal, completion, and production phases of the upstream oil and gas sector.
Life sciences and health
With an almost limitless high-performance bioinformatics infrastructure, you can accelerate discoveries in genomes, precision medicine, and clinical studies.
Silicon
High-performance, scalable, safe, and available infrastructure that is optimised for electrical design automation (EDA) workloads in terms of networking, computation, and storage.
Producing
Utilise scalable and highly secure on-demand HPC infrastructure to quickly iterate product design in order to shorten time to market and enhance product quality.
Applications
Machine learning
With Azure-powered advanced analytics, machine learning, and artificial intelligence workloads, you may operate clusters at almost limitless scalability, get strong remote workstations, and improve your insights.
Display
With Azure’s more than 50 global datacenter locations, you can run any graphic-intensive job in the cloud and provide amazing experiences to any device, anywhere, at any time.
Creating
Using a scalable, non-competing, MPAA-certified platform that is trusted by 95% of the Fortune 500, render with confidence.
Platform Services for HPC
With a cloud platform designed for global reach and equipped with services tailored to HPC applications, you may access enormous computational resources.
Interaction
With Azure ExpressRoute, create secure, private tunnels for hybrid cloud communication. Use InfiniBand to leverage Linux remote direct memory access (RDMA) for message passing interface (MPI) applications in your datacenter.
Services for Applications
Using Azure Batch, create, manage, and schedule tasks. Use Azure CycleCloud to dynamically deploy Azure high performance computing clusters.
Calculate
On Azure, find the ideal high-performance computing resources at an almost limitless scale. For applications requiring a lot of memory, use H-series virtual machines; for applications requiring graphics and CUDA/OpenCL, use N-series virtual machines; and for a fully managed supercomputer, use Cray.
Sensible Services
Utilising Data Lake Analytics, create predictive analysis-based apps for the next generation of users. Utilise your HPC data to create and execute machine learning models to get insights that help you make smarter choices.
Keepsake
Use data from on-site NAS devices equipped with HPC Cache to launch your HPC apps on Azure. Azure NetApp Files, provided as an Azure service directly inside an Azure datacenter, provide access to massive volumes of I/O with a sub-millisecond latency. Use Cray ClusterSor, a bare-metal, Lustre-based HPC storage system that is completely connected with Azure, for high throughput storage.Image credit to Azure
The mission of the Microsoft Surface organization is to provide renowned end-to-end experiences in hardware, software, and services that consumers adore using on a daily basis. Microsoft think that individuals who create a product represent that person, and that talented and passionate engineers and designers can create unique things when supported by the correct infrastructure and tools. Daily decisions on product features, dependability, and design are often made using simulation models at the product level.
The company is also embarking on a multi-year plan to produce unique items with exceptional efficiency. Microsoft Azure high performance computing is essential to making this goal possible. This is a description of how they used Azure high performance computing and simulation to do more with less.Image credit to Azure
Product development: laptop Surface
Azure high performance computing’s accessibility for Abaqus-based structural simulations contributed to its status as the main tool for product design development. Detailed translations of design ideas from digital computer-aided design (CAD) systems are made into FEA models.
These comprise all of the device’s primary subsystems and are actual digital prototypes. The analyst may assess viability by applying various test and reliability conditions in a virtual environment using FEA models. Hundreds of simulations are run over the course of a few days in order to assess different design concepts and methods for strengthening the device. The chosen design is then turned into a prototype and put through extensive testing to ensure it can withstand real-world circumstances. Azure’s engineering method includes many feedback loops for comparing FEA findings with real testing to validate the model.
The dynamic simulation’s starting condition is the impact velocity for a certain height. Using the Abaqus solver, the dynamic drop simulation is run on hundreds of cores of an Azure high performance computing cluster. Azure is used the Explicit and Abaqus solver, which is renowned for providing a reliable and accurate solution for fast-moving, nonlinear dynamic events like automobile crashworthiness and consumer electronics drop testing.
These solvers allow scalability to thousands of cores for high throughputs and are specifically tuned for Azure high performance computing clusters. On these optimized Azure HPC machines, simulation workloads finish in a few hours as opposed to the days they used to take. The analysts evaluate the data and compare the stress levels to the material limitations. After reviewing the findings, analysts and design teams change the designs. Because the Azure HPC servers allow very short turnaround times for evaluations, this cycle keeps repeating in rapid cycles.
The impact-induced motion and stress levels of the hinge’s interior components could be seen by the team thanks to the simulation. Azure was able to identify the primary problem and implement the necessary design changes as a result. This realization aided in the hinge assembly’s development to reduce stress levels. Since success required just one iteration, a significant amount of time was saved in the design process. Costs for testing, physical prototyping, and tooling were also reduced.
Currently, digital prototypes (FEA models) running on Azure high performance computing clusters are used to validate designs across the whole Microsoft Surface product range. In only a few weeks, thousands of simulation projects are regularly completed to allow state-of-the-art designs with very high dependability and customer satisfaction.
Next up
The group is now concentrating on implementing more scalable simulation and Azure HPC resources for multiphysics modelling and interdisciplinary collaborations. Enabling AI and machine learning for the purpose of creating new products is a big potential. The utilization of Azure HPC and the collaborations across Microsoft entities will be used to propel extensive advancements at a quick pace. Alongside the V4 Institute, Azure is advancing this digital transformation journey by using model-based systems engineering (MBSE). Working with Azure will be very beneficial for top-tier companies trying to scale digital simulations and accomplish more with fewer resources.
Read more on govindhtech.com
#azure#azurehpc#hpc#cloudcomputing#datasecurity#machinelearning#microsoft#hpcapplication#silicon#automobile#technology#technews#news#govindhtech
0 notes