#LanguageModeling
Explore tagged Tumblr posts
Text
Discover Samba, Microsoft’s innovative model designed for efficient unlimited Context Language Modeling. Its unique blend of State Space Model and Sliding Window Attention sets new standards in AI. Let us explore this fascinating model and learn more insightful info.
#AI#Microsoft#LanguageModeling#artificial intelligence#machinelearning#software engineering#programming#python#machine learning
0 notes
Text
#poll#naturallanguageprocessing#AI#chattingwithgemini#GoogleAI#machinelearning#peacejaway#AIethics#generativeLLM#googlegemini#@momentsofawareness#chatbot#languagemodeling#@PeaceJaway
0 notes
Text
What Is Generative Physical AI? Why It Is Important?
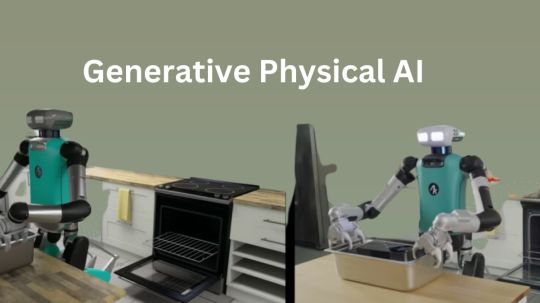
What is Physical AI?
Autonomous robots can see, comprehend, and carry out intricate tasks in the actual (physical) environment with to physical artificial intelligence. Because of its capacity to produce ideas and actions to carry out, it is also sometimes referred to as “Generative physical AI.”
How Does Physical AI Work?
Models of generative AI Massive volumes of text and picture data, mostly from the Internet, are used to train huge language models like GPT and Llama. Although these AIs are very good at creating human language and abstract ideas, their understanding of the physical world and its laws is still somewhat restricted.
Current generative AI is expanded by Generative physical AI, which comprehends the spatial linkages and physical behavior of the three-dimensional environment in which the all inhabit. During the AI training process, this is accomplished by supplying extra data that includes details about the spatial connections and physical laws of the actual world.
Highly realistic computer simulations are used to create the 3D training data, which doubles as an AI training ground and data source.
A digital doppelganger of a location, such a factory, is the first step in physically-based data creation. Sensors and self-governing devices, such as robots, are introduced into this virtual environment. The sensors record different interactions, such as rigid body dynamics like movement and collisions or how light interacts in an environment, and simulations that replicate real-world situations are run.
What Function Does Reinforcement Learning Serve in Physical AI?
Reinforcement learning trains autonomous robots to perform in the real world by teaching them skills in a simulated environment. Through hundreds or even millions of trial-and-error, it enables self-governing robots to acquire abilities in a safe and efficient manner.
By rewarding a physical AI model for doing desirable activities in the simulation, this learning approach helps the model continually adapt and become better. Autonomous robots gradually learn to respond correctly to novel circumstances and unanticipated obstacles via repeated reinforcement learning, readying them for real-world operations.
An autonomous machine may eventually acquire complex fine motor abilities required for practical tasks like packing boxes neatly, assisting in the construction of automobiles, or independently navigating settings.
Why is Physical AI Important?
Autonomous robots used to be unable to detect and comprehend their surroundings. However, Generative physical AI enables the construction and training of robots that can naturally interact with and adapt to their real-world environment.
Teams require strong, physics-based simulations that provide a secure, regulated setting for training autonomous machines in order to develop physical AI. This improves accessibility and utility in real-world applications by facilitating more natural interactions between people and machines, in addition to increasing the efficiency and accuracy of robots in carrying out complicated tasks.
Every business will undergo a transformation as Generative physical AI opens up new possibilities. For instance:
Robots: With physical AI, robots show notable improvements in their operating skills in a range of environments.
Using direct input from onboard sensors, autonomous mobile robots (AMRs) in warehouses are able to traverse complicated settings and avoid impediments, including people.
Depending on how an item is positioned on a conveyor belt, manipulators may modify their grabbing position and strength, demonstrating both fine and gross motor abilities according to the object type.
This method helps surgical robots learn complex activities like stitching and threading needles, demonstrating the accuracy and versatility of Generative physical AI in teaching robots for particular tasks.
Autonomous Vehicles (AVs): AVs can make wise judgments in a variety of settings, from wide highways to metropolitan cityscapes, by using sensors to sense and comprehend their environment. By exposing AVs to physical AI, they may better identify people, react to traffic or weather, and change lanes on their own, efficiently adjusting to a variety of unforeseen situations.
Smart Spaces: Large interior areas like factories and warehouses, where everyday operations include a constant flow of people, cars, and robots, are becoming safer and more functional with to physical artificial intelligence. By monitoring several things and actions inside these areas, teams may improve dynamic route planning and maximize operational efficiency with the use of fixed cameras and sophisticated computer vision models. Additionally, they effectively see and comprehend large-scale, complicated settings, putting human safety first.
How Can You Get Started With Physical AI?
Using Generative physical AI to create the next generation of autonomous devices requires a coordinated effort from many specialized computers:
Construct a virtual 3D environment: A high-fidelity, physically based virtual environment is needed to reflect the actual world and provide synthetic data essential for training physical AI. In order to create these 3D worlds, developers can simply include RTX rendering and Universal Scene Description (OpenUSD) into their current software tools and simulation processes using the NVIDIA Omniverse platform of APIs, SDKs, and services.
NVIDIA OVX systems support this environment: Large-scale sceneries or data that are required for simulation or model training are also captured in this stage. fVDB, an extension of PyTorch that enables deep learning operations on large-scale 3D data, is a significant technical advancement that has made it possible for effective AI model training and inference with rich 3D datasets. It effectively represents features.
Create synthetic data: Custom synthetic data generation (SDG) pipelines may be constructed using the Omniverse Replicator SDK. Domain randomization is one of Replicator’s built-in features that lets you change a lot of the physical aspects of a 3D simulation, including lighting, position, size, texture, materials, and much more. The resulting pictures may also be further enhanced by using diffusion models with ControlNet.
Train and validate: In addition to pretrained computer vision models available on NVIDIA NGC, the NVIDIA DGX platform, a fully integrated hardware and software AI platform, may be utilized with physically based data to train or fine-tune AI models using frameworks like TensorFlow, PyTorch, or NVIDIA TAO. After training, reference apps such as NVIDIA Isaac Sim may be used to test the model and its software stack in simulation. Additionally, developers may use open-source frameworks like Isaac Lab to use reinforcement learning to improve the robot’s abilities.
In order to power a physical autonomous machine, such a humanoid robot or industrial automation system, the optimized stack may now be installed on the NVIDIA Jetson Orin and, eventually, the next-generation Jetson Thor robotics supercomputer.
Read more on govindhtech.com
#GenerativePhysicalAI#generativeAI#languagemodels#PyTorch#NVIDIAOmniverse#AImodel#artificialintelligence#NVIDIADGX#TensorFlow#AI#technology#technews#news#govindhtech
3 notes
·
View notes
Video
youtube
🎥 RAG vs Fine-Tuning – Practical Comparison with Voice Prompts on Mobile...
#youtube#RAG FineTuning RetrievalAugmentedGeneration LLMs LanguageModels AIComparison VoicePromptAI MobileAI MachineLearning DeepLearning PromptEngin
0 notes
Text
The Rise of Small Language Models: Are They the Future of NLP?
In recent years, large language models like GPT-4 and PaLM have dominated the field of NLP (Natural Language Processing). However, in 2025, we are witnessing a major shift: the rise of small language models (SLMs). Models like LLaMA 3, Mistral, and Gemma are proving that bigger isn't always better for NLP tasks.
Unlike their massive counterparts, small models are designed to be lightweight, faster, and cost-effective, making them ideal for a variety of NLP applications such as real-time translation, chatbots, and voice assistants. They require significantly less computing power, making them perfect for edge computing, mobile devices, and private deployments where traditional NLP systems were too heavy to operate.
Moreover, small language models offer better customization, privacy, and control over NLP systems, allowing businesses to fine-tune models for specific needs without relying on external cloud services.
While large models still dominate in highly complex tasks, small language models are shaping the future of NLP — bringing powerful language capabilities to every device and business, big or small.
#NLP#SmallLanguageModels#AI#MachineLearning#EdgeComputing#NaturalLanguageProcessing#AIFuture#TechInnovation#AIEfficiency#NLPTrends#FutureOfAI#ArtificialIntelligence#LanguageModels#AIin2025#TechForBusiness#AIandPrivacy#SustainableAI#ModelOptimization
0 notes
Text
TopoGraphic Language Model is a cutting-edge AI technology designed to understand and generate human-like language by organizing data in a topographical structure. This model enhances semantic understanding by mapping concepts in a spatial, interconnected way, offering a deeper, more intuitive grasp of language. Perfect for those interested in how AI can merge linguistic patterns with geometric principles, TopoGraphic Language Models are shaping the future of natural language processing.
#AI#LanguageModels#TechInnovation#NLP#MachineLearning#TopoGraphicLanguageModel#BrainlikeAI#ArtificialBrain#NeuralNetworkArchitecture#AdvancedAIModels#AIandNeuroscience#NextGenLanguageModels#MachineLearningInnovation#AIResearch2025#TopoLM#ConsciousAI#AIMimickingtheBrain#CognitiveComputing#LanguageModelEvolution#FutureofAI#ailatestupdate#latestaiupdate#latestaitrends#ainews
0 notes
Text
Gemini 2.0 Expands Availability with New Updates and Models
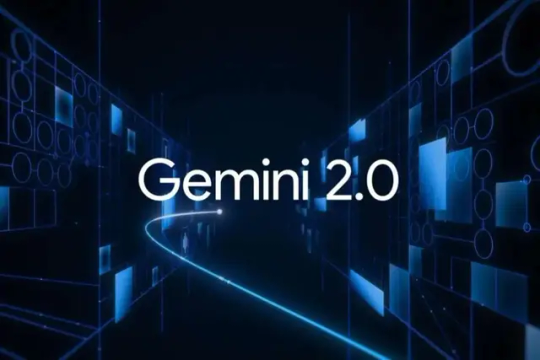
Source: gadgets360.com
Share Post:
LinkedIn
Twitter
Facebook
Reddit
Pinterest
Gemini 2.0 Flash Now Available to All Users
Google has announced the broader availability of Gemini 2.0 Flash, its high-efficiency AI model designed for developers. Initially introduced as an experimental version, the model has undergone updates to enhance its performance, particularly in complex problem-solving. The latest version, now accessible to all users of the Gemini app on both desktop and mobile, is set to improve creative, interactive, and collaborative experiences. Additionally, the updated model is now available via the Gemini API in Google AI Studio and Vertex AI, allowing developers to integrate it into production applications.
In addition to this, Google has introduced Gemini 2.0 Pro, an experimental model designed to deliver improved coding performance and handle complex prompts with enhanced reasoning capabilities. This advanced version is now available in Google AI Studio, Vertex AI, and the Gemini app for those using the Gemini Advanced tier. Furthermore, Google has launched Gemini 2.0 Flash-Lite, a cost-efficient variant of the model, currently available for public preview in Google AI Studio and Vertex AI.
Gemini 2.0 Flash: A Robust Model for Developers
Originally introduced at the I/O 2024 event, the Flash series has gained popularity among developers for its efficiency in handling large-scale, high-frequency tasks. With a 1 million-token context window, the model excels in multimodal reasoning across extensive datasets. The latest update of Gemini 2.0 Flash, now made generally available, enhances its capabilities in key performance benchmarks, with upcoming support for image generation and text-to-speech.
Users can access the updated model via the Gemini app or through the Gemini API in Google AI Studio and Vertex AI. Developers looking for details regarding pricing can refer to the Google for Developers blog, where more insights into cost structures and potential applications are provided.
New Experimental and Cost-Effective AI Models
Google continues to refine its AI offerings by launching experimental and cost-efficient models tailored to specific use cases. The latest experimental release, Gemini 2.0 Pro, is designed to deliver superior coding performance and effectively process complex prompts. With a context window of 2 million tokens, this model enables a more comprehensive analysis of vast datasets while also supporting advanced tools such as Google Search integration and code execution. Available to developers through Google AI Studio and Vertex AI, it can also be accessed by Gemini Advanced users via the model dropdown on desktop and mobile devices.
Additionally, Google has unveiled Gemini 2.0 Flash-Lite, an optimized and cost-effective model that builds on the success of its predecessor, 1.5 Flash. While maintaining the same speed and cost efficiency, Flash-Lite offers enhanced performance and improved benchmark results. With a 1 million-token context window and multimodal input capabilities, it enables high-volume processing at an affordable price. Currently available for public preview in Google AI Studio and Vertex AI, this model aims to provide developers with a budget-friendly yet powerful AI tool.
As AI technology evolves, Google remains committed to enhancing the safety and security of its models. The Gemini 2.0 lineup incorporates advanced reinforcement learning techniques to refine responses and mitigate risks associated with AI-generated content. Additionally, automated safety assessments help identify and address potential security threats, ensuring a secure and reliable experience for users.
#Gemini2_0#GeminiNextGen#GoogleAI#LLM#LanguageModel#AI#ArtificialIntelligence#Innovation#Technology#FutureOfAI#NextGenAI
0 notes
Text
Explore the potential of Platypus, the state-of-the-art framework for refining large language models. Platypus can improve the quality and diversity of the LLM’s outputs, as well as reduce the human effort and time required for the refinement process. Learn how it improves LLM performance on specific tasks using a small amount of data using human feedback and model merging techniques.
#Platypus#LLM#NLP#AI#MachineLearning#ModelMerging#TextGeneration#LanguageModeling#NaturalLanguageProcessing#ArtificialIntelligence#artificial intelligence#open source#machine learning#programming#software engineering
1 note
·
View note
Text
#naturallanguageprocessing#chattingwithbard#googlebard#AI#moa#GoogleAI#bard#machinelearning#peacejaway#AIethics#generativeLLM#momentsofawareness#chatbot#languagemodeling
0 notes
Text
Evolution Hints at Emerging Artificial General Intelligence

Recent developments in artificial intelligence (AI) have fueled speculation that the field may be inching toward the elusive goal of Artificial General Intelligence (AGI). This level of intelligence, which allows a machine to understand, learn, and apply reasoning across diverse domains with human-like adaptability, has long been considered a benchmark in AI research. However, researchers from the Massachusetts Institute of Technology (MIT) have introduced a new technique, termed Test-Time Training (TTT), which may represent a significant step toward AGI. The findings, published in a recent paper, showcase TTT's unexpected efficacy in enhancing AI’s abstract reasoning capabilities, a core component in AGI’s development. White Paper Summary Original White Paper What Makes Test-Time Training Revolutionary? Test-Time Training offers an innovative approach where AI models update their parameters dynamically during testing, allowing them to adapt to novel tasks beyond their pre-training. Traditional AI systems, even with extensive pre-training, struggle with tasks that require advanced reasoning, pattern recognition, or manipulation of unfamiliar information. TTT circumvents these limitations by enabling AI to “learn” from a minimal number of examples during inference, updating the model temporarily for that specific task. This real-time adaptability is essential for models to tackle unexpected challenges autonomously, making TTT a promising technique for AGI research. The MIT study tested TTT on the Abstraction and Reasoning Corpus (ARC), a benchmark composed of complex reasoning tasks involving diverse visual and logical challenges. The researchers demonstrated that TTT, combined with initial fine-tuning and augmentation, led to a six-fold improvement in accuracy over traditional fine-tuned models. When applied to an 8-billion parameter language model, TTT achieved 53% accuracy on ARC’s public validation set, exceeding the performance of previous methods by nearly 25% and matching the performance level of humans on many tasks. Pushing the Boundaries of Reasoning in AI The study’s findings suggest that achieving AGI may not solely depend on complex symbolic reasoning but could also be reached through enhanced neural network adaptations, as demonstrated by TTT. By dynamically adjusting its understanding and approach at the test phase, the AI system closely mimics human-like learning behaviors, enhancing both flexibility and accuracy. MIT’s study shows that TTT can push neural language models to excel in domains traditionally dominated by symbolic or rule-based systems. This could represent a paradigm shift in AI development strategies, bringing AGI within closer reach. Implications and Future Directions The implications of TTT are vast. By enabling AI models to adapt dynamically during testing, this approach could revolutionize applications across sectors that demand real-time decision-making, from autonomous vehicles to healthcare diagnostics. The findings encourage a reassessment of AGI’s feasibility, as TTT shows that AI systems might achieve sophisticated problem-solving capabilities without exclusively relying on highly structured symbolic AI. Despite these advances, researchers caution that AGI remains a complex goal with many unknowns. However, the ability of AI models to adjust parameters in real-time to solve new tasks signals a promising trajectory. The breakthrough hints at an imminent era where AI can not only perform specialized tasks but adapt across domains, a hallmark of AGI. In Summary: The research from MIT showcases the potential of Test-Time Training to bring AI models closer to Artificial General Intelligence. As these adaptable and reasoning capabilities are refined, the future of AI may not be limited to predefined tasks, but open to broad, versatile applications that mimic human cognitive flexibility. Read the full article
#abstractreasoning#AbstractionandReasoningCorpus#AGI#AIreasoning#ARCbenchmark#artificialgeneralintelligence#computationalreasoning#few-shotlearning#inferencetraining#languagemodels#LoRA#low-rankadaptation#machinelearningadaptation#neuralmodels#programsynthesis#real-timelearning#symbolicAI#test-timetraining#TTT
0 notes
Text
What Is Ministral? And Its Features Of Edge Language Model

High-performance, low-resource language models for edge computing are being revolutionized by Ministral, the best edge language model in the world.
What is Ministral?
On October 16, 2024, the Mistral AI team unveiled two new cutting-edge models, Ministral 3B and Ministral 8B, which their developers refer to as “the world’s best edge models” for on-device computing and edge use cases. This came on the first anniversary of the release of Mistral 7B, a model that revolutionized autonomous frontier AI innovation for millions of people.
With 3 billion and 8 billion parameters, respectively much less than the majority of their peers these two models are establishing a new benchmark in the sub-10B parameter area by providing unparalleled efficiency, commonsense reasoning, knowledge integration, and function-calling capabilities.
Features Of Ministral
With a number of cutting-edge capabilities, the Ministral 3B and 8B versions are perfect for a wide range of edge computing applications. Let’s examine what makes these models unique in more detail:
Versatility and Efficiency
From managing intricate workflows to carrying out extremely specialized operations, Ministral 3B and 8B are built to handle a broad range of applications. They are appropriate for both daily consumers and enterprise-level applications due to their adaptability, which allows them to be readily tailored to different industrial demands. Because of their adaptability, they can power solutions in a variety of areas, such as task-specific models, automation, and natural language processing, all while delivering efficient performance without requiring big, resource-intensive servers.
Extended Context Lengths
Compared to many other models in their class, these models can handle and process much longer inputs since they offer context lengths of up to 128k tokens. Applications such as document analysis, lengthy discussions, and any situation where preserving prolonged context is essential will find this functionality especially helpful.
At the moment, vLLM, an optimized library for effectively executing large language models (LLMs), offers this functionality up to 32k. Ministral 3B and 8B give consumers more comprehension and continuity during longer encounters by utilizing vLLM, which is crucial for sophisticated natural language creation and interpretation.
Innovative Interleaved Sliding-Window Attention (Ministral 8B)
Ministral 8B’s interleaved sliding-window attention method is one of its best features. By splitting the input sequence into overlapping windows that are processed repeatedly, this method maximizes memory consumption and processing performance.
The model can therefore carry out inference more effectively, which makes it perfect for edge AI applications with constrained processing resources. This capability makes Ministral 8B an ideal option for real-time applications by ensuring that it strikes a balance between high-speed processing and the capacity to manage huge contexts without consuming excessive amounts of memory.
Local, Privacy-First Inference
Ministral 3B and 8B are designed with local, privacy-first inference capabilities in response to the increasing demand from clients for privacy-conscious AI solutions. They are therefore perfect for uses like on-device translation, internet-less smart assistants, local analytics, and autonomous robots where data confidentiality is crucial. Users may lower the risks involved in sending data to cloud servers and improve overall security by running models directly on local devices, ensuring that sensitive data stays on-site.
Compute-Efficient and Low-Latency Solutions
Les Ministraux’s capacity to provide low-latency, compute-efficient AI solutions is one of their main advantages. This implies that even on devices with constrained hardware capabilities, they may respond quickly and continue to operate at high levels. Ministral 3B and 8B provide a scalable solution that can satisfy a broad range of applications without the strain of significant computational resources, whether they are being used by large-scale manufacturing teams optimizing automated processes or by independent hobbyists working on personal projects.
Efficient Intermediaries for Function-Calling
Ministral 3B and 8B are effective bridges for function-calling in intricate, multi-step processes when combined with bigger language models such as Mistral Large. To ensure that multi-step processes are carried out smoothly, they may be adjusted to handle certain duties like input processing, task routing, and API calls based on user intent. When sophisticated agentic processes are needed, their low latency and cheap cost allow for quicker interactions and better resource management.
These characteristics work together to make Ministral 3B and Ministral 8B effective instruments in the rapidly developing field of edge AI. They meet the many demands of users, ranging from people wanting useful on-device AI solutions to companies seeking scalable, privacy-first AI deployments, by providing a combination of adaptability, efficiency, and sophisticated context management.
Read more on Govindhtech.com
#languagemodels#Ministral#Ministral3B#Ministral8B#MistralAI#AI#News#Technews#Technology#Technologynews#Technologytrends#Govindhtech
0 notes
Text
LLM Developers & Development Company | Why Choose Feathersoft Info Solutions for Your AI Needs
In the ever-evolving landscape of artificial intelligence, Large Language Models (LLMs) are at the forefront of technological advancement. These sophisticated models, designed to understand and generate human-like text, are revolutionizing industries from healthcare to finance. As businesses strive to leverage LLMs to gain a competitive edge, partnering with expert LLM developers and development companies becomes crucial. Feathersoft Info Solutions stands out as a leader in this transformative field, offering unparalleled expertise in LLM development.
What Are Large Language Models?
Large Language Models are a type of AI designed to process and generate natural language with remarkable accuracy. Unlike traditional models, LLMs are trained on vast amounts of text data, enabling them to understand context, nuances, and even generate coherent and contextually relevant responses. This capability makes them invaluable for a range of applications, including chatbots, content creation, and advanced data analysis.
The Role of LLM Developers
Developing an effective LLM requires a deep understanding of both the technology and its applications. LLM developers are specialists in creating and fine-tuning these models to meet specific business needs. Their expertise encompasses:
Model Training and Fine-Tuning: Developers train LLMs on diverse datasets, adjusting parameters to improve performance and relevance.
Integration with Existing Systems: They ensure seamless integration of LLMs into existing business systems, optimizing functionality and user experience.
Customization for Specific Needs: Developers tailor LLMs to address unique industry requirements, enhancing their utility and effectiveness.
Why Choose Feathersoft Info Solutions Company for LLM Development?
Feathersoft Info Solutions excels in providing comprehensive LLM development services, bringing a wealth of experience and a proven track record to the table. Here’s why Feathersoft Info Solutions is the go-to choice for businesses looking to harness the power of LLMs:
Expertise and Experience: Feathersoft Info Solutions team comprises seasoned experts in AI and machine learning, ensuring top-notch development and implementation of LLM solutions.
Customized Solutions: Understanding that each business has unique needs, Feathersoft Info Solutionsoffers customized LLM solutions tailored to specific industry requirements.
Cutting-Edge Technology: Utilizing the latest advancements in AI, Feathersoft Info Solutions ensures that their LLMs are at the forefront of innovation and performance.
End-to-End Support: From initial consultation and development to deployment and ongoing support, Feathersoft Info Solutions provides comprehensive services to ensure the success of your LLM projects.
Applications of LLMs in Various Industries
The versatility of LLMs allows them to be applied across a multitude of industries:
Healthcare: Enhancing patient interactions, aiding in diagnostic processes, and streamlining medical documentation.
Finance: Automating customer support, generating financial reports, and analyzing market trends.
Retail: Personalizing customer experiences, managing inventory, and optimizing supply chain logistics.
Education: Creating intelligent tutoring systems, generating educational content, and analyzing student performance.
Conclusion
As LLM technology continues to advance, partnering with a skilled LLM development company like Feathersoft Info Solutions can provide your business with a significant advantage. Their expertise in developing and implementing cutting-edge LLM solutions ensures that you can fully leverage this technology to drive innovation and achieve your business goals.
For businesses ready to explore the potential of Large Language Models, Feathersoft Info Solutions offers the expertise and support needed to turn cutting-edge technology into actionable results. Contact Feathersoft Info Solutions today to start your journey toward AI-powered success.
#LLM#LargeLanguageModels#AI#ArtificialIntelligence#MachineLearning#TechInnovation#AIDevelopment#LanguageModels#DataScience#TechTrends#AIExperts#BusinessTech#AIConsulting#SoftwareDevelopment
0 notes
Text
GPT-4o Arrives! How to Unlock OpenAI's Latest Language Model
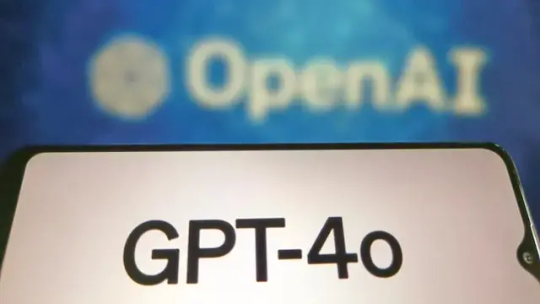
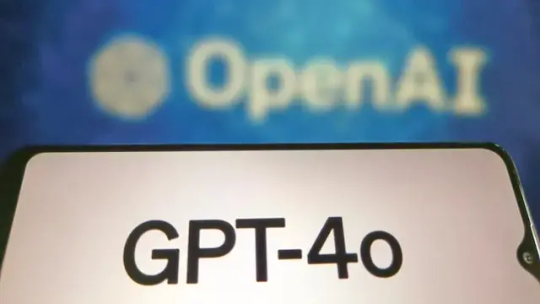
OpenAI GPT-4o is Now Rolling Out — Here's How to Get Access
OpenAI has announced the rollout of GPT-4o, the latest iteration of its groundbreaking language model. Designed to enhance both performance and accessibility, GPT-4o brings a host of new features and improvements over its predecessors. Here's everything you need to know about GPT-4o and how to gain access to this cutting-edge technology.
What is GPT-4o?
GPT-4o (Generative Pre-trained Transformer 4 optimized) is the newest version of OpenAI's language model, building on the foundation of GPT-4. This version focuses on optimization for a wider range of applications, including more efficient processing, improved accuracy, and better handling of complex queries. GPT-4o aims to provide users with a more robust and versatile tool for various tasks, from generating creative content to assisting with technical problem-solving. Also Read:How To Redirect Old Domain To New Domain
Key Features and Improvements
- Enhanced Performance: GPT-4o has been fine-tuned to deliver faster response times and more accurate outputs. This is achieved through advancements in both the model architecture and the underlying algorithms. - Improved Accuracy: With a larger dataset and more sophisticated training techniques, GPT-4o offers higher accuracy in understanding and generating human-like text. This means fewer errors and more relevant responses. - Broader Applications: GPT-4o is designed to be more versatile, making it suitable for a wider array of tasks. Whether you need help with writing, coding, research, or customer service, GPT-4o can handle it. - User-Friendly Interface: OpenAI has worked on improving the user interface, making it easier for users to interact with GPT-4o. The interface is more intuitive, allowing for smoother and more efficient use.
How to Get Access to GPT-4o
Getting access to GPT-4o is straightforward, but it depends on your current relationship with OpenAI and how you intend to use the model. Here are the steps to follow: https://www.youtube.com/watch?v=WzUnEfiIqP4 A demo For Chatgpt 4o - OpenAI API Access: If you already have an OpenAI API key, you might be eligible for an upgrade to GPT-4o. Check your OpenAI dashboard or contact support to see if you qualify for the new model. - Sign Up for OpenAI Services: For new users, signing up for OpenAI's API services is the first step. Visit the OpenAI website and follow the instructions to create an account. Once you have an account, you can request access to GPT-4o. - Subscription Plans: OpenAI offers various subscription plans that provide different levels of access to their models. Review the available plans and select one that suits your needs. Some plans might offer immediate access to GPT-4o, while others could have a waiting period. - Enterprise Solutions: For businesses and organizations, OpenAI provides tailored solutions. Contact OpenAI’s sales team to discuss enterprise access and custom integrations of GPT-4o into your workflows. - Educational and Research Access: OpenAI often collaborates with educational institutions and researchers. If you are part of such an institution, you might be able to get access to GPT-4o through academic partnerships.
Conclusion
GPT-4o represents a significant step forward in AI-driven language models, offering enhanced performance, improved accuracy, and broader applicability. Whether you're a developer, researcher, business professional, or just an enthusiast, accessing GPT-4o can open up new possibilities for your projects. Follow the steps outlined above to start using this powerful tool today. Stay tuned for more updates from OpenAI as they continue to innovate and expand the capabilities of artificial intelligence. Frequently Asked QuestionAnswer1. What is GPT-4o and how does it differ from previous versions?GPT-4o, or Generative Pre-trained Transformer 4 optimized, is the latest version of OpenAI's language model. It builds on the foundation of GPT-4 with enhanced performance, improved accuracy, and better handling of complex queries.2. What are the key features and improvements of GPT-4o?GPT-4o features faster response times, more accurate outputs, broader application versatility, and a more user-friendly interface. These improvements are due to advancements in model architecture, larger datasets, and sophisticated training techniques.3. How can GPT-4o enhance performance in various applications?GPT-4o can enhance performance by providing faster and more accurate responses, which is beneficial for applications such as creative content generation, technical problem-solving, coding, research, and customer service.4. What steps do I need to take to get access to GPT-4o?To access GPT-4o, you need to either have an OpenAI API key, sign up for OpenAI services on their website, choose a suitable subscription plan, or contact OpenAI’s sales team for enterprise solutions. Educational and research institutions may also have specific access options.5. Are there any specific subscription plans for accessing GPT-4o?Yes, OpenAI offers various subscription plans that provide different levels of access to their models. These plans can be reviewed on OpenAI's website to select one that best fits your needs, with some offering immediate access to GPT-4o and others having a waiting period.6. How can businesses integrate GPT-4o into their workflows?Businesses can integrate GPT-4o into their workflows by contacting OpenAI’s sales team to discuss tailored solutions and custom integrations. This allows businesses to leverage GPT-4o’s capabilities for specific applications and improve their processes.7. Is there a user-friendly interface for interacting with GPT-4o?Yes, OpenAI has improved the user interface for GPT-4o, making it more intuitive and easier to use. This helps users interact with the model more efficiently and effectively.8. Can educational institutions and researchers get access to GPT-4o?Yes, OpenAI often collaborates with educational institutions and researchers. These institutions can get access to GPT-4o through academic partnerships and specific programs designed to support research and educational use.9. What types of tasks is GPT-4o particularly suited for?GPT-4o is particularly suited for a wide range of tasks, including writing and content generation, coding assistance, research, customer service, and technical problem-solving. Its versatility makes it applicable across various fields and industries.10. How can I check if I am eligible for an upgrade to GPT-4o?If you already have an OpenAI API key, you can check your OpenAI dashboard or contact support to see if you qualify for an upgrade to GPT-4o. OpenAI may provide information on eligibility and the upgrade process directly on their platform. Read the full article
#AIInnovation#AIResearch#AITrends#AIUpdates#ArtificialIntelligence#GPT4oFeatures#GPT4oRollout#LanguageModel#MachineLearning#NextGenAI#OpenAIGPT4o#OpenAITech#TechLaunch#TechNews
0 notes
Text

🚀 Master Prompt Engineering with Visualpath! 🧠✨
🎯 Visualpath offers the best Prompt Engineering Training, diving deep into: 🔹 Crafting effective AI prompts 🤖 🔹 Advanced NLP techniques 📚 🔹 Real-world AI applications 🌟
💡 Learn to master AI model interactions and create innovative solutions with our online Prompt Engineering courses! 🌐
🔥 Enroll today and take the first step toward becoming an AI innovator! 💼
📞 Call: +91-9989971070 📲 WhatsApp: https://www.whatsapp.com/catalog/919989971070/ 📖 Blog: https://bestpromptengineeringcourse.blogspot.com/
🌐 Visit us: https://www.visualpath.in/prompt-engineering-course.html
#MachineLearning#AI#ArtificialIntelligence#NLP#LanguageModels#GenerativeAI#ChatGPT#AIResearch#DataScience#DeepLearning#PromptDesign#AITraining#TechInnovation#artificialintelligence#CareerGrowth#students#education#software#onlinetraining#ITskills#newtechnology#traininginstitutes#Visualpath
1 note
·
View note
Text
Discover the power of OpenOrca-Preview1-13B, a cost-effective language model developed by the OpenOrca team. Our latest article explores its capabilities and performance, showing how it achieved 60% of the improvement with only 6% of the data.
0 notes