#ML algorithms
Explore tagged Tumblr posts
Text
0 notes
Text
Choose AI/ML Algorithms Very Efficiently
The world is getting smarter every day, to keep up to date and satisfy consumer expectation tech companies adapting machine learning algorithms to make things easy but choosing a machine learning algorithm is always a tedious job for techies, there are lots of algorithms present for different kind of problems and we can use this for tackling things in different ways.
The machine learning algorithm’s main goal is to inspect the data and find similar patterns between them, and with that, make detailed predictions. As the name implies, ML algorithms are basically calculations prepared in different ways.
We are creating data every day; we are just surrounded by data in different formats. It comes from a variety of sources: business data, personal social media activity, sensors in the IoT, etc. Machine learning algorithms are used to extract data and turn it into something useful that can serve to automate processes, personalize experiences, and make difficult forecasts that human brains cannot do on their own.
Choosing algorithms solely depends on your project requirements. Given the type of tasks that ML algorithms answer, each type trains in absolute tasks, taking into consideration the limitations of the knowledge that you have and the necessities of your project.
Types of AI/ML Algorithms
Different types of machine learning algorithms are:
Supervised learning
Unsupervised learning
Semi-Supervised learning
Reinforcement learning
Supervised ML algorithm:
This is the most popular ML algorithm because of its flexibility and comprehensiveness, and it is mostly used to do the most common ML tasks. It requires labeled data.
Supervised knowledge depends on supervision; we train the machines utilizing the branded dataset and establish the training; bureaucracy thinks about the output. It allows you to collect data from previous experiences. Helps you improve performance tests using occurrence.
Unsupervised ML algorithm:
Unsupervised learning is typically achieved by using unsupervised machine learning techniques. Using unsupervised algorithms, you can handle problems differently than with supervised algorithms and operate in more complicated ways. Unsupervised learning, however, could be more irregular than the subsequent deep learning and support learning patterns based on natural input.
There are three main tasks in Unsupervised learning, such as:
Clustering: It is a data mining technique used for grouping unlabeled data based on similarities between them.
Association: It uses different rules to find relationships between variables in a given dataset. These plans are frequently secondhand for advertising basket studies and recommendation transformers.
Dimensionality Reduction: It is used when the number of features in a dataset is too high. It reduces the number of data inputs to a controllable size while more maintaining the dossier honor. Often, this technique is secondhand in the preprocessing dossier stage, in the way that when autoencoders erase noise from being able to be seen with eyes dossier to boost picture quality.
Semi-Supervised ML algorithm:
When you are using a training dataset with both labeled and unlabeled data or you can’t decide on whether to use supervised or unsupervised algorithms, Semi-Supervised is the best choice in that case.
Reinforcement ML algorithm:
Reinforcement knowledge algorithms are mostly based on dynamic compute methods. The idea behind this type of ML treasure is to compare investigation and exploitation. Other machine learning algorithms used mapping middle from two points of recommendation and productivity, Unlike directed supervised placement, where the feedback supported by the power is correct set of conduct for performing a task, support education uses rewards and penalties as signals for helpful and negative behavior.
Conclusion
Choosing an ML algorithm is apparently a complex task, particularly if you don’t have a far-reaching background in this field. However, knowledge about the types of algorithms and the tasks that they were created to resolve and solving a set of questions might help you resolve this complication. Learning more about machine learning algorithms, their types, and answering these questions might lead you to an algorithm that’ll be a perfect match for your goal.
Click the link below to learn more about the blog Choose AI/ML Algorithms Very Efficiently:
1 note
·
View note
Text
0 notes
Text

If you happen to have a conversation about technology trends with a business executive, founder, or software engineer, you definitely hear them talk about Machine Intelligence (Artificial Intelligence or AI), Machine Learning (ML), and automation. And they will also most probably tell you about how these technologies are revolutionizing the traditional business scenarios. It is gaining such prominence, that the total funding assigned to ML, globally during the first quarter of 2019 was close to $28.5 billion. With these statistics in mind, organizations have no choice but to dive deeper into AI and ML and learn how these technologies can help them stay relevant.
0 notes
Text
Machine Learning in Finance: Opportunities and Challenges
(Images made by author with MS Bing Image Creator ) Machine learning (ML), a branch of artificial intelligence (AI), is reshaping the finance industry, empowering investment professionals to unlock hidden insights, improve trading processes, and optimize portfolios. While ML holds great promise for revolutionizing decision-making, it presents challenges as well. This post explores current…
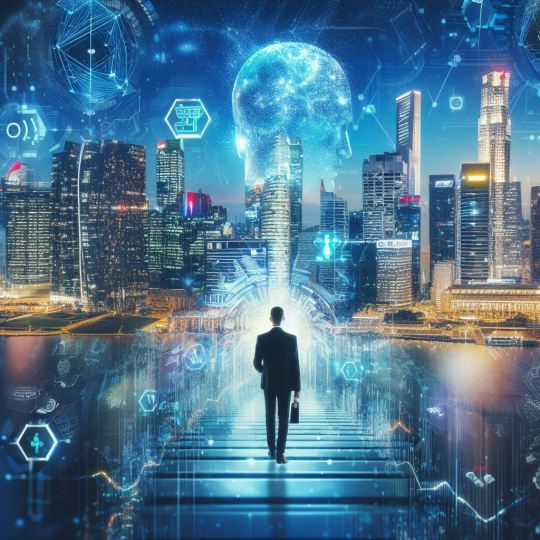
View On WordPress
#clustering#Finance#financial data analysis#kkn#machine learning#ml algorithms#nlp#overfitting#pca#portfolio optimization#random forests#svm
0 notes
Text
Transforming Predictive Maintenance with CIMCON Digital’s IoT Edge Platform: Unlocking Proactive Asset Management
Introduction
In today’s fast-paced and technologically advanced world, the need for efficient and proactive asset management is paramount for businesses to stay competitive. CIMCON Digital’s IoT Edge Platform emerges as a game-changer in the realm of Predictive Maintenance, empowering organizations to detect anomalies in advance using ML algorithms. This capability not only enables customers to plan schedules well in advance and avoid costly downtime but also provides real-time visibility into the remaining useful life of assets. In this article, we delve into how CIMCON Digital’s IoT Edge Platform revolutionizes Predictive Maintenance with practical examples of proactive asset management.
1. The Challenge of Reactive Maintenance
Traditionally, companies have been plagued by reactive maintenance practices, where assets are repaired or replaced only after failures occur. This reactive approach leads to unexpected downtime, reduced productivity, and increased maintenance costs. Predicting asset failures and planning maintenance schedules in advance is critical to ensure smooth operations, optimize resource allocation, and minimize overall downtime.
2. Empowering Proactive Maintenance with ML Algorithms
CIMCON Digital’s IoT Edge Platform is equipped with advanced Machine Learning algorithms that analyze real-time data from connected assets and machines. By continuously monitoring sensor data and historical performance trends, the platform can accurately detect anomalies and deviations from normal operating patterns. This proactive approach allows businesses to predict potential asset failures well in advance, providing ample time to schedule maintenance activities before any critical failures occur.
3. Planning Ahead to Avoid Downtime
Imagine a scenario in a manufacturing facility where a critical piece of equipment experiences an unexpected failure. The consequences could be disastrous, leading to costly downtime and missed production targets. With CIMCON Digital’s IoT Edge Platform in place, the same equipment would be continuously monitored in real-time. As soon as the platform detects any unusual behavior or signs of potential failure, it triggers an alert to the maintenance team.
Armed with this early warning, the maintenance team can plan the necessary repairs or replacements well in advance, avoiding unplanned downtime and minimizing disruption to production schedules. This capability not only ensures smooth operations but also optimizes maintenance resources and lowers the overall maintenance costs.
4. Real-Time Visibility into Asset Health
The IoT Edge Platform goes beyond detecting anomalies; it also provides real-time insights into the remaining useful life of assets. By analyzing historical performance data and asset health indicators, the platform estimates the remaining operational life of an asset with high accuracy.
Consider a scenario in a utility company managing a fleet of aging turbines. The maintenance team needs to know the remaining useful life of each turbine to plan proactive maintenance and avoid sudden breakdowns. With CIMCON Digital’s IoT Edge Platform, the team can access real-time information on the health of each turbine, enabling them to make data-driven decisions about maintenance schedules, parts replacement, and resource allocation.
5. Benefits of CIMCON Digital's IoT Edge Platform
CIMCON Digital’s IoT Edge Platform offers a host of benefits to businesses seeking to enhance their Predictive Maintenance capabilities:
a) Proactive Decision-making: By detecting anomalies in advance, the platform enables proactive decision-making, reducing reactive responses and enhancing overall operational efficiency.
b) Minimized Downtime: With the ability to schedule maintenance activities in advance, businesses can avoid costly downtime, leading to increased productivity and higher customer satisfaction.
c) Optimal Resource Allocation: The platform’s real-time visibility into asset health allows for better resource allocation, ensuring that maintenance efforts are targeted where they are most needed.
d) Cost Savings: By avoiding unexpected failures and optimizing maintenance schedules, businesses can significantly reduce maintenance costs and improve their bottom line.
Conclusion:
CIMCON Digital’s IoT Edge Platform empowers businesses to transcend traditional reactive maintenance practices and embrace a proactive approach to asset management. With the platform’s advanced ML algorithms, businesses can detect anomalies in advance, plan maintenance schedules proactively, and gain real-time visibility into asset health. This transformative capability results in minimized downtime, optimized resource allocation, and substantial cost savings. As CIMCON Digital’s IoT Edge Platform continues to revolutionize Predictive Maintenance, businesses can embark on a journey towards greater efficiency, productivity, and long-term sustainability.
#iot#Predictive Maintenance#Asset Management#IoT Edge Platform#Proactive Maintenance#ML Algorithms#Anomaly Detection#Resource Allocation#Real-time Visibility#Downtime Reduction#Cost Savings#Asset Health#CIMCON Digital#Reactive Maintenance#Operational Efficiency#Business Sustainability#Maintenance Scheduling#Data-driven Decisions#Production Optimization#Customer Satisfaction#Utility Company
0 notes
Text
How to Paraphrase Text Using ML Algorithms in Python?
"Delve into the world of text paraphrasing using ML algorithms with this engaging Medium article. Learn how to effectively rephrase text using Python, exploring the innovative techniques and algorithms that make it possible. Enhance your understanding of natural language processing and elevate your paraphrasing skills through this informative read on Nerd For Tech."
0 notes
Text
The surprising truth about data-driven dictatorships
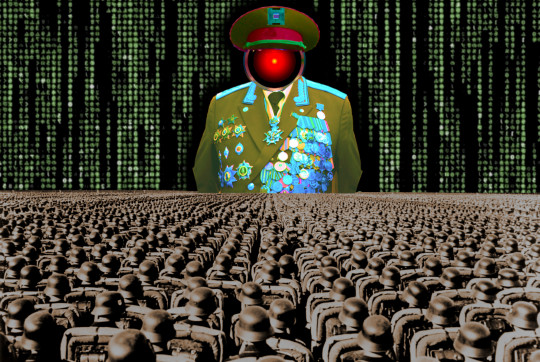
Here’s the “dictator’s dilemma”: they want to block their country’s frustrated elites from mobilizing against them, so they censor public communications; but they also want to know what their people truly believe, so they can head off simmering resentments before they boil over into regime-toppling revolutions.
These two strategies are in tension: the more you censor, the less you know about the true feelings of your citizens and the easier it will be to miss serious problems until they spill over into the streets (think: the fall of the Berlin Wall or Tunisia before the Arab Spring). Dictators try to square this circle with things like private opinion polling or petition systems, but these capture a small slice of the potentially destabiziling moods circulating in the body politic.
Enter AI: back in 2018, Yuval Harari proposed that AI would supercharge dictatorships by mining and summarizing the public mood — as captured on social media — allowing dictators to tack into serious discontent and diffuse it before it erupted into unequenchable wildfire:
https://www.theatlantic.com/magazine/archive/2018/10/yuval-noah-harari-technology-tyranny/568330/
Harari wrote that “the desire to concentrate all information and power in one place may become [dictators] decisive advantage in the 21st century.” But other political scientists sharply disagreed. Last year, Henry Farrell, Jeremy Wallace and Abraham Newman published a thoroughgoing rebuttal to Harari in Foreign Affairs:
https://www.foreignaffairs.com/world/spirals-delusion-artificial-intelligence-decision-making
They argued that — like everyone who gets excited about AI, only to have their hopes dashed — dictators seeking to use AI to understand the public mood would run into serious training data bias problems. After all, people living under dictatorships know that spouting off about their discontent and desire for change is a risky business, so they will self-censor on social media. That’s true even if a person isn’t afraid of retaliation: if you know that using certain words or phrases in a post will get it autoblocked by a censorbot, what’s the point of trying to use those words?
The phrase “Garbage In, Garbage Out” dates back to 1957. That’s how long we’ve known that a computer that operates on bad data will barf up bad conclusions. But this is a very inconvenient truth for AI weirdos: having given up on manually assembling training data based on careful human judgment with multiple review steps, the AI industry “pivoted” to mass ingestion of scraped data from the whole internet.
But adding more unreliable data to an unreliable dataset doesn’t improve its reliability. GIGO is the iron law of computing, and you can’t repeal it by shoveling more garbage into the top of the training funnel:
https://memex.craphound.com/2018/05/29/garbage-in-garbage-out-machine-learning-has-not-repealed-the-iron-law-of-computer-science/
When it comes to “AI” that’s used for decision support — that is, when an algorithm tells humans what to do and they do it — then you get something worse than Garbage In, Garbage Out — you get Garbage In, Garbage Out, Garbage Back In Again. That’s when the AI spits out something wrong, and then another AI sucks up that wrong conclusion and uses it to generate more conclusions.
To see this in action, consider the deeply flawed predictive policing systems that cities around the world rely on. These systems suck up crime data from the cops, then predict where crime is going to be, and send cops to those “hotspots” to do things like throw Black kids up against a wall and make them turn out their pockets, or pull over drivers and search their cars after pretending to have smelled cannabis.
The problem here is that “crime the police detected” isn’t the same as “crime.” You only find crime where you look for it. For example, there are far more incidents of domestic abuse reported in apartment buildings than in fully detached homes. That’s not because apartment dwellers are more likely to be wife-beaters: it’s because domestic abuse is most often reported by a neighbor who hears it through the walls.
So if your cops practice racially biased policing (I know, this is hard to imagine, but stay with me /s), then the crime they detect will already be a function of bias. If you only ever throw Black kids up against a wall and turn out their pockets, then every knife and dime-bag you find in someone’s pockets will come from some Black kid the cops decided to harass.
That’s life without AI. But now let’s throw in predictive policing: feed your “knives found in pockets” data to an algorithm and ask it to predict where there are more knives in pockets, and it will send you back to that Black neighborhood and tell you do throw even more Black kids up against a wall and search their pockets. The more you do this, the more knives you’ll find, and the more you’ll go back and do it again.
This is what Patrick Ball from the Human Rights Data Analysis Group calls “empiricism washing”: take a biased procedure and feed it to an algorithm, and then you get to go and do more biased procedures, and whenever anyone accuses you of bias, you can insist that you’re just following an empirical conclusion of a neutral algorithm, because “math can’t be racist.”
HRDAG has done excellent work on this, finding a natural experiment that makes the problem of GIGOGBI crystal clear. The National Survey On Drug Use and Health produces the gold standard snapshot of drug use in America. Kristian Lum and William Isaac took Oakland’s drug arrest data from 2010 and asked Predpol, a leading predictive policing product, to predict where Oakland’s 2011 drug use would take place.
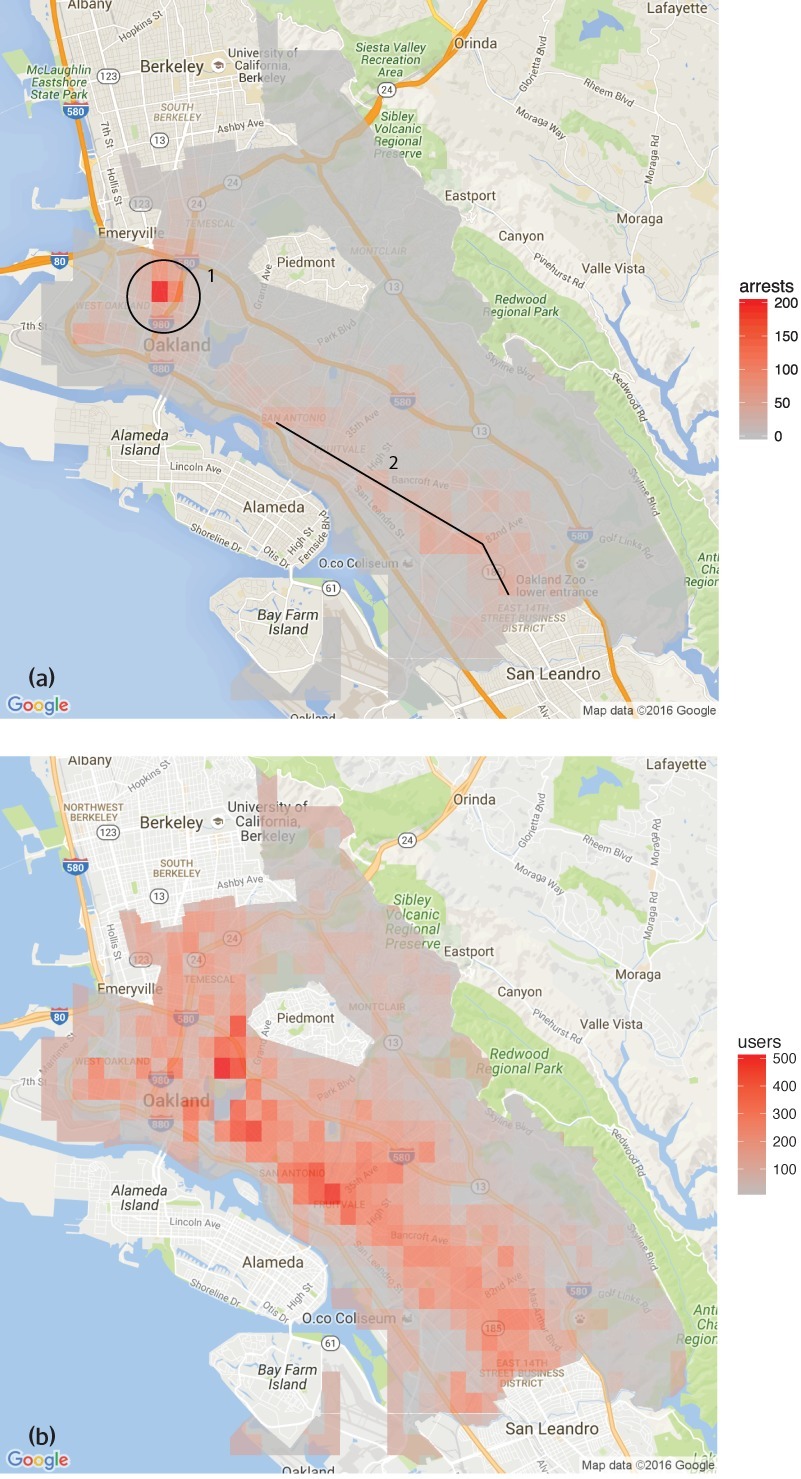
[Image ID: (a) Number of drug arrests made by Oakland police department, 2010. (1) West Oakland, (2) International Boulevard. (b) Estimated number of drug users, based on 2011 National Survey on Drug Use and Health]
Then, they compared those predictions to the outcomes of the 2011 survey, which shows where actual drug use took place. The two maps couldn’t be more different:
https://rss.onlinelibrary.wiley.com/doi/full/10.1111/j.1740-9713.2016.00960.x
Predpol told cops to go and look for drug use in a predominantly Black, working class neighborhood. Meanwhile the NSDUH survey showed the actual drug use took place all over Oakland, with a higher concentration in the Berkeley-neighboring student neighborhood.
What’s even more vivid is what happens when you simulate running Predpol on the new arrest data that would be generated by cops following its recommendations. If the cops went to that Black neighborhood and found more drugs there and told Predpol about it, the recommendation gets stronger and more confident.
In other words, GIGOGBI is a system for concentrating bias. Even trace amounts of bias in the original training data get refined and magnified when they are output though a decision support system that directs humans to go an act on that output. Algorithms are to bias what centrifuges are to radioactive ore: a way to turn minute amounts of bias into pluripotent, indestructible toxic waste.
There’s a great name for an AI that’s trained on an AI’s output, courtesy of Jathan Sadowski: “Habsburg AI.”
And that brings me back to the Dictator’s Dilemma. If your citizens are self-censoring in order to avoid retaliation or algorithmic shadowbanning, then the AI you train on their posts in order to find out what they’re really thinking will steer you in the opposite direction, so you make bad policies that make people angrier and destabilize things more.
Or at least, that was Farrell(et al)’s theory. And for many years, that’s where the debate over AI and dictatorship has stalled: theory vs theory. But now, there’s some empirical data on this, thanks to the “The Digital Dictator’s Dilemma,” a new paper from UCSD PhD candidate Eddie Yang:
https://www.eddieyang.net/research/DDD.pdf
Yang figured out a way to test these dueling hypotheses. He got 10 million Chinese social media posts from the start of the pandemic, before companies like Weibo were required to censor certain pandemic-related posts as politically sensitive. Yang treats these posts as a robust snapshot of public opinion: because there was no censorship of pandemic-related chatter, Chinese users were free to post anything they wanted without having to self-censor for fear of retaliation or deletion.
Next, Yang acquired the censorship model used by a real Chinese social media company to decide which posts should be blocked. Using this, he was able to determine which of the posts in the original set would be censored today in China.
That means that Yang knows that the “real” sentiment in the Chinese social media snapshot is, and what Chinese authorities would believe it to be if Chinese users were self-censoring all the posts that would be flagged by censorware today.
From here, Yang was able to play with the knobs, and determine how “preference-falsification” (when users lie about their feelings) and self-censorship would give a dictatorship a misleading view of public sentiment. What he finds is that the more repressive a regime is — the more people are incentivized to falsify or censor their views — the worse the system gets at uncovering the true public mood.
What’s more, adding additional (bad) data to the system doesn’t fix this “missing data” problem. GIGO remains an iron law of computing in this context, too.
But it gets better (or worse, I guess): Yang models a “crisis” scenario in which users stop self-censoring and start articulating their true views (because they’ve run out of fucks to give). This is the most dangerous moment for a dictator, and depending on the dictatorship handles it, they either get another decade or rule, or they wake up with guillotines on their lawns.
But “crisis” is where AI performs the worst. Trained on the “status quo” data where users are continuously self-censoring and preference-falsifying, AI has no clue how to handle the unvarnished truth. Both its recommendations about what to censor and its summaries of public sentiment are the least accurate when crisis erupts.
But here’s an interesting wrinkle: Yang scraped a bunch of Chinese users’ posts from Twitter — which the Chinese government doesn’t get to censor (yet) or spy on (yet) — and fed them to the model. He hypothesized that when Chinese users post to American social media, they don’t self-censor or preference-falsify, so this data should help the model improve its accuracy.
He was right — the model got significantly better once it ingested data from Twitter than when it was working solely from Weibo posts. And Yang notes that dictatorships all over the world are widely understood to be scraping western/northern social media.
But even though Twitter data improved the model’s accuracy, it was still wildly inaccurate, compared to the same model trained on a full set of un-self-censored, un-falsified data. GIGO is not an option, it’s the law (of computing).
Writing about the study on Crooked Timber, Farrell notes that as the world fills up with “garbage and noise” (he invokes Philip K Dick’s delighted coinage “gubbish”), “approximately correct knowledge becomes the scarce and valuable resource.”
https://crookedtimber.org/2023/07/25/51610/
This “probably approximately correct knowledge” comes from humans, not LLMs or AI, and so “the social applications of machine learning in non-authoritarian societies are just as parasitic on these forms of human knowledge production as authoritarian governments.”
The Clarion Science Fiction and Fantasy Writers’ Workshop summer fundraiser is almost over! I am an alum, instructor and volunteer board member for this nonprofit workshop whose alums include Octavia Butler, Kim Stanley Robinson, Bruce Sterling, Nalo Hopkinson, Kameron Hurley, Nnedi Okorafor, Lucius Shepard, and Ted Chiang! Your donations will help us subsidize tuition for students, making Clarion — and sf/f — more accessible for all kinds of writers.
Libro.fm is the indie-bookstore-friendly, DRM-free audiobook alternative to Audible, the Amazon-owned monopolist that locks every book you buy to Amazon forever. When you buy a book on Libro, they share some of the purchase price with a local indie bookstore of your choosing (Libro is the best partner I have in selling my own DRM-free audiobooks!). As of today, Libro is even better, because it’s available in five new territories and currencies: Canada, the UK, the EU, Australia and New Zealand!
[Image ID: An altered image of the Nuremberg rally, with ranked lines of soldiers facing a towering figure in a many-ribboned soldier's coat. He wears a high-peaked cap with a microchip in place of insignia. His head has been replaced with the menacing red eye of HAL9000 from Stanley Kubrick's '2001: A Space Odyssey.' The sky behind him is filled with a 'code waterfall' from 'The Matrix.']
Image: Cryteria (modified) https://commons.wikimedia.org/wiki/File:HAL9000.svg
CC BY 3.0 https://creativecommons.org/licenses/by/3.0/deed.en
—
Raimond Spekking (modified) https://commons.wikimedia.org/wiki/File:Acer_Extensa_5220_-_Columbia_MB_06236-1N_-_Intel_Celeron_M_530_-_SLA2G_-_in_Socket_479-5029.jpg
CC BY-SA 4.0 https://creativecommons.org/licenses/by-sa/4.0/deed.en
—
Russian Airborne Troops (modified) https://commons.wikimedia.org/wiki/File:Vladislav_Achalov_at_the_Airborne_Troops_Day_in_Moscow_%E2%80%93_August_2,_2008.jpg
“Soldiers of Russia” Cultural Center (modified) https://commons.wikimedia.org/wiki/File:Col._Leonid_Khabarov_in_an_everyday_service_uniform.JPG
CC BY-SA 3.0 https://creativecommons.org/licenses/by-sa/3.0/deed.en
#pluralistic#habsburg ai#self censorship#henry farrell#digital dictatorships#machine learning#dictator's dilemma#eddie yang#preference falsification#political science#training bias#scholarship#spirals of delusion#algorithmic bias#ml#Fully automated data driven authoritarianism#authoritarianism#gigo#garbage in garbage out garbage back in#gigogbi#yuval noah harari#gubbish#pkd#philip k dick#phildickian
833 notes
·
View notes
Text
Anyone else deeply disturbed that at middle school teacher in a kids show was named after a type of underwear? A type of underwear known to enhance the bust I might add
(Mme Caline Bustier)
A bustier:
…yikes
#I hope this doesn’t get flagged by the algorithm gods#but how did anyone on the production team think this was appropriate??#we get it she’s attractive#but maybe don’t give characters names based on them being sexy guys#it’s a show for little kids#idk maybe I’m overreacting?#it’s basically a corset#but I think even that name would be very weird#and its emphasis is literally on the bust#miraculous#mlb#miraculous ladybug#caline bustier#madame bustier#zombizou#ml salt#ml critical#ml criticism
27 notes
·
View notes
Note
Hey, quick non-CK question - what do you mean you got in trouble for hoarding URLs?? I didn't even know that was a THING?
Yeah haha. Not sure how long you’ve been on tumblr, but back in the day (like 10-12+ years ago) hoarding URLs was a big thing, especially in fandom spaces. Basically the idea was that you create a bunch of inactive sideblogs to save a URL that you might want to use in the future. At this point in time, switching back and forth between URLs was also a lot more common. I think some people even used to sell their URLs, or trade other URLs for them which is crazy. There was an entire URL hoarding real estate. It was actually a huge problem lol, I remember wanting to enter certain fandom spaces but being unable to get a URL related to it. And back then I feel like fandoms were a little harder to breach into, especially if you didn’t have a blog that looked like it should belong there—or maybe I was just younger and didn’t know how to interact with people lol, but either way it was still frustrating.
However, Tumblr has since (not sure when exactly) banned URL hoarding. Idk what exactly they quantify as hoarding, but I did have at least a few dozen URLs saved from over a decade ago that I honestly didn’t even remember I had. Most of them were related to emo bands lmao. I think what happened in my case is that this sideblog got caught in the tumblr spam filter somehow, which is why for months I wasn’t able to reply to people and was bot having posts appear in the tags. A lot of people have been having problems with being incorrectly flagged as spam in the last couple years, due to the algorithms they use to detect potential spam accounts. The real problem is that once your account has been flagged tumblr doesn’t notify you or communicate the problem to you, so if you don’t know what to look for (which I didn’t) you don’t know how to properly report the problem. I contacted support a few times but because I reported it as a bug, I suspect it didn’t go to the right place. Eventually this led to my account being terminated without warning or notification. I reached out to tumblr support on multiple platforms, and I think when they looked into the issue they saw that I had a bunch of URLs hoarded and suspended my main account (but restored access to this one). I deleted all the saved URLs, emailed support back, and they released my main account back. All in all I’m just happy with how quickly they resolved the issue tbh, I know a lot of people have spent months trying to get a terminated/suspended account back so I feel pretty grateful. And honestly I think it’s good that tumblr enforces the ban on URL hoarding, bc I remember how upsetting it was being like 12 years old and unable to get a URL related to the thing I really cared about.
#in terms of the algorithms if anyone cares—usually in order to be productionized ML algorithms need to have an accuracy rate of at least 70#this varies by industry and country#but lets even give the benefit of the doubt and say their algorithm has an accuracy rate of 90%#that still means 10% of the blogs they flag are false positives i.e real blogs run by real#people#(this is an oversimplification of model metrics hut you get the point)#in my case its possible this blog was flagged in the first case because of all the urls i had saved#but anyway! thats what happened lol#if youre worried about it id say that if you have more than 10 urls that are not active and have never been active you should delete#half of them#not ck#asks
6 notes
·
View notes
Text
How do you do Machine Learning research from scratch?
#machinelearning#artificialintelligence#art#digitalart#mlart#datascience#ai#algorithm#bigdata#research#ml research 2025
2 notes
·
View notes
Text
#multi-label classification#machine learning#classification techniques#AI#ML algorithms#quick insights#data science
0 notes
Text
relating things youve learned in other classes to current classes is so fun and gives you an ego trip like no other i absolutely recommend it
2 notes
·
View notes
Text
Statistical Tools
Daily writing promptWhat was the last thing you searched for online? Why were you looking for it?View all responses Checking which has been my most recent search on Google, I found that I asked for papers, published in the last 5 years, that used a Montecarlo method to check the reliability of a mathematical method to calculate a team’s efficacy. Photo by Andrea Piacquadio on Pexels.com I was…
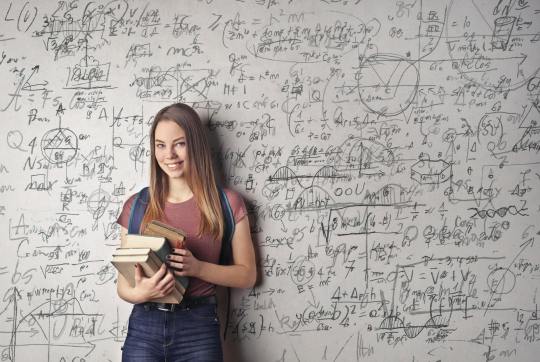
View On WordPress
#Adjusted R-Squared#Agile#AI#AIC#Akaike Information Criterion#Akaike Information Criterion (AIC)#Algorithm#algorithm design#Analysis#Artificial Intelligence#Bayesian Information Criterion#Bayesian Information Criterion (BIC)#BIC#Business#Coaching#consulting#Cross-Validation#dailyprompt#dailyprompt-2043#Goodness of Fit#Hypothesis Testing#inputs#Machine Learning#Mathematical Algorithm#Mathematics#Mean Squared Error#ML#Model Selection#Monte Carlo#Monte Carlo Methods
2 notes
·
View notes
Text
#machinelearning#artificialintelligence#art#digitalart#mlart#ai#datascience#algorithm#vr#bigdata#ml so good#ai artist
6 notes
·
View notes
Text
B.Tech CSE Specialization in AI Techniques & ML Algorithms Powered by L&T
B.Tech CSE (AI & ML) powered by L&T equips students with cutting-edge AI techniques & ML algorithms, preparing them for future-ready tech careers.
#b.tech CSE specialization in AI techniques & ML algorithms powered by L&T#b.tech CSE (AI & ML) powered by L&T course#admissions open in b.tech cse in AI & ML
1 note
·
View note