#RAG LLM Service Providers
Explore tagged Tumblr posts
Text
Think Smarter, Not Harder: Meet RAG

How do RAG make machines think like you?
Imagine a world where your AI assistant doesn't only talk like a human but understands your needs, explores the latest data, and gives you answers you can trust—every single time. Sounds like science fiction? It's not.
We're at the tipping point of an AI revolution, where large language models (LLMs) like OpenAI's GPT are rewriting the rules of engagement in everything from customer service to creative writing. here's the catch: all that eloquence means nothing if it can't deliver the goods—if the answers aren't just smooth, spot-on, accurate, and deeply relevant to your reality.
The question is: Are today's AI models genuinely equipped to keep up with the complexities of real-world applications, where context, precision, and truth aren't just desirable but essential? The answer lies in pushing the boundaries further—with Retrieval-Augmented Generation (RAG).
While LLMs generate human-sounding copies, they often fail to deliver reliable answers based on real facts. How do we ensure that an AI-powered assistant doesn't confidently deliver outdated or incorrect information? How do we strike a balance between fluency and factuality? The answer is in a brand new powerful approach: Retrieval-Augmented Generation (RAG).
What is Retrieval-Augmented Generation (RAG)?
RAG is a game-changing technique to increase the basic abilities of traditional language models by integrating them with information retrieval mechanisms. RAG does not only rely on pre-acquired knowledge but actively seek external information to create up-to-date and accurate answers, rich in context. Imagine for a second what could happen if you had a customer support chatbot able to engage in a conversation and draw its answers from the latest research, news, or your internal documents to provide accurate, context-specific answers.
RAG has the immense potential to guarantee informed, responsive and versatile AI. But why is this necessary? Traditional LLMs are trained on vast datasets but are static by nature. They cannot access real-time information or specialized knowledge, which can lead to "hallucinations"—confidently incorrect responses. RAG addresses this by equipping LLMs to query external knowledge bases, grounding their outputs in factual data.
How Does Retrieval-Augmented Generation (RAG) Work?
RAG brings a dynamic new layer to traditional AI workflows. Let's break down its components:
Embedding Model
Think of this as the system's "translator." It converts text documents into vector formats, making it easier to manage and compare large volumes of data.
Retriever
It's the AI's internal search engine. It scans the vectorized data to locate the most relevant documents that align with the user's query.
Reranker (Opt.)
It assesses the submitted documents and score their relevance to guarantee that the most pertinent data will pass along.
Language Model
The language model combines the original query with the top documents the retriever provides, crafting a precise and contextually aware response. Embedding these components enables RAG to enhance the factual accuracy of outputs and allows for continuous updates from external data sources, eliminating the need for costly model retraining.
How does RAG achieve this integration?
It begins with a query. When a user asks a question, the retriever sifts through a curated knowledge base using vector embeddings to find relevant documents. These documents are then fed into the language model, which generates an answer informed by the latest and most accurate information. This approach dramatically reduces the risk of hallucinations and ensures that the AI remains current and context-aware.
RAG for Content Creation: A Game Changer or just a IT thing?
Content creation is one of the most exciting areas where RAG is making waves. Imagine an AI writer who crafts engaging articles and pulls in the latest data, trends, and insights from credible sources, ensuring that every piece of content is compelling and accurate isn't a futuristic dream or the product of your imagination. RAG makes it happen.
Why is this so revolutionary?
Engaging and factually sound content is rare, especially in today's digital landscape, where misinformation can spread like wildfire. RAG offers a solution by combining the creative fluency of LLMs with the grounding precision of information retrieval. Consider a marketing team launching a campaign based on emerging trends. Instead of manually scouring the web for the latest statistics or customer insights, an RAG-enabled tool could instantly pull in relevant data, allowing the team to craft content that resonates with current market conditions.
The same goes for various industries from finance to healthcare, and law, where accuracy is fundamental. RAG-powered content creation tools promise that every output aligns with the most recent regulations, the latest research and market trends, contributing to boosting the organization's credibility and impact.
Applying RAG in day-to-day business
How can we effectively tap into the power of RAG? Here's a step-by-step guide:
Identify High-Impact Use Cases
Start by pinpointing areas where accurate, context-aware information is critical. Think customer service, marketing, content creation, and compliance—wherever real-time knowledge can provide a competitive edge.
Curate a robust knowledge base
RAG relies on the quality of the data it collects and finds. Build or connect to a comprehensive knowledge repository with up-to-date, reliable information—internal documents, proprietary data, or trusted external sources.
Select the right tools and technologies
Leverage platforms that support RAG architecture or integrate retrieval mechanisms with existing LLMs. Many AI vendors now offer solutions combining these capabilities, so choose one that fits your needs.
Train your team
Successful implementation requires understanding how RAG works and its potential impact. Ensure your team is well-trained in deploying RAG&aapos;s technical and strategic aspects.
Monitor and optimize
Like any technology, RAG benefits from continuous monitoring and optimization. Track key performance indicators (KPIs) like accuracy, response time, and user satisfaction to refine and enhance its application.
Applying these steps will help organizations like yours unlock RAG's full potential, transform their operations, and enhance their competitive edge.
The Business Value of RAG
Why should businesses consider integrating RAG into their operations? The value proposition is clear:
Trust and accuracy
RAG significantly enhances the accuracy of responses, which is crucial for maintaining customer trust, especially in sectors like finance, healthcare, and law.
Efficiency
Ultimately, RAG reduces the workload on human employees, freeing them to focus on higher-value tasks.
Knowledge management
RAG ensures that information is always up-to-date and relevant, helping businesses maintain a high standard of knowledge dissemination and reducing the risk of costly errors.
Scalability and change
As an organization grows and evolves, so does the complexity of information management. RAG offers a scalable solution that can adapt to increasing data volumes and diverse information needs.
RAG vs. Fine-Tuning: What's the Difference?
Both RAG and fine-tuning are powerful techniques for optimizing LLM performance, but they serve different purposes:
Fine-Tuning
This approach involves additional training on specific datasets to make a model more adept at particular tasks. While effective for niche applications, it can limit the model's flexibility and adaptability.
RAG
In contrast, RAG dynamically retrieves information from external sources, allowing for continuous updates without extensive retraining, which makes it ideal for applications where real-time data and accuracy are critical.
The choice between RAG and fine-tuning entirely depends on your unique needs. For example, RAG is the way to go if your priority is real-time accuracy and contextual relevance.
Concluding Thoughts
As AI evolves, the demand for RAG AI Service Providers systems that are not only intelligent but also accurate, reliable, and adaptable will only grow. Retrieval-Augmented generation stands at the forefront of this evolution, promising to make AI more useful and trustworthy across various applications.
Whether it's a content creation revolution, enhancing customer support, or driving smarter business decisions, RAG represents a fundamental shift in how we interact with AI. It bridges the gap between what AI knows and needs to know, making it the tool of reference to grow a real competitive edge.
Let's explore the infinite possibilities of RAG together
We would love to know; how do you intend to optimize the power of RAG in your business? There are plenty of opportunities that we can bring together to life. Contact our team of AI experts for a chat about RAG and let's see if we can build game-changing models together.
#RAG#Fine-tuning LLM for RAG#RAG System Development Companies#RAG LLM Service Providers#RAG Model Implementation#RAG-Enabled AI Platforms#RAG AI Service Providers#Custom RAG Model Development
0 notes
Text
Benefits Of Conversational AI & How It Works With Examples
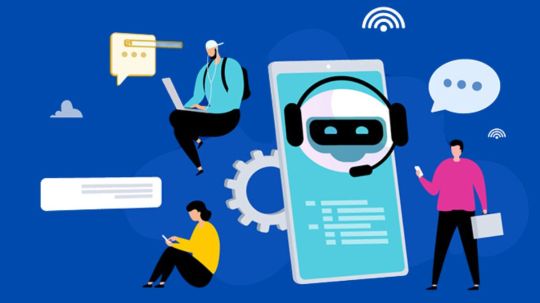
What Is Conversational AI?
Conversational AI mimics human speech. It’s made possible by Google’s foundation models, which underlie new generative AI capabilities, and NLP, which helps computers understand and interpret human language.
How Conversational AI works
Natural language processing (NLP), foundation models, and machine learning (ML) are all used in conversational AI.
Large volumes of speech and text data are used to train conversational AI systems. The machine is trained to comprehend and analyze human language using this data. The machine then engages in normal human interaction using this information. Over time, it improves the quality of its responses by continuously learning from its interactions.
Conversational AI For Customer Service
With IBM Watsonx Assistant, a next-generation conversational AI solution, anyone in your company can easily create generative AI assistants that provide customers with frictionless self-service experiences across all devices and channels, increase employee productivity, and expand your company.
User-friendly: Easy-to-use UI including pre-made themes and a drag-and-drop chat builder.
Out-of-the-box: Unconventional To better comprehend the context of each natural language communication, use large language models, large speech models, intelligent context gathering, and natural language processing and understanding (NLP, NLU).
Retrieval-augmented generation (RAG): It based on your company’s knowledge base, provides conversational responses that are correct, relevant, and current at all times.
Use cases
Watsonx Assistant may be easily set up to accommodate your department’s unique requirements.
Customer service
Strong client support With quick and precise responses, chatbots boost sales while saving contact center funds.
Human resources
All of your employees may save time and have a better work experience with HR automation. Questions can be answered by staff members at any time.
Marketing
With quick, individualized customer service, powerful AI chatbot marketing software lets you increase lead generation and enhance client experiences.
Features
Examine ways to increase production, enhance customer communications, and increase your bottom line.
Artificial Intelligence
Strong Watsonx Large Language Models (LLMs) that are tailored for specific commercial applications.
The Visual Builder
Building generative AI assistants using to user-friendly interface doesn’t require any coding knowledge.
Integrations
Pre-established links with a large number of channels, third-party apps, and corporate systems.
Security
Additional protection to prevent hackers and improper use of consumer information.
Analytics
Comprehensive reports and a strong analytics dashboard to monitor the effectiveness of conversations.
Self-service accessibility
For a consistent client experience, intelligent virtual assistants offer self-service responses and activities during off-peak hours.
Benfits of Conversational AI
Automation may save expenses while boosting output and operational effectiveness.
Conversational AI, for instance, may minimize human error and expenses by automating operations that are presently completed by people. Increase client happiness and engagement by providing a better customer experience.
Conversational AI, for instance, may offer a more engaging and customized experience by remembering client preferences and assisting consumers around-the-clock when human agents are not present.
Conversational AI Examples
Here are some instances of conversational AI technology in action:
Virtual agents that employ generative AI to support voice or text conversations are known as generative AI agents.
Chatbots are frequently utilized in customer care applications to respond to inquiries and offer assistance.
Virtual assistants are frequently voice-activated and compatible with smart speakers and mobile devices.
Software that converts text to speech is used to produce spoken instructions or audiobooks.
Software for speech recognition is used to transcribe phone conversations, lectures, subtitles, and more.
Applications Of Conversational AI
Customer service: Virtual assistants and chatbots may solve problems, respond to frequently asked questions, and offer product details.
E-commerce: Chatbots driven by AI can help customers make judgments about what to buy and propose products.
Healthcare: Virtual health assistants are able to make appointments, check patient health, and offer medical advice.
Education: AI-powered tutors may respond to student inquiries and offer individualized learning experiences.
In summary
The way to communicate with robots might be completely changed by the formidable technology known as conversational AI. Also can use its potential to produce more effective, interesting, and customized experiences if it comprehend its essential elements, advantages, and uses.
Read more on Govindhech.com
#ConversationalAI#AI#NLP#machinelearning#generativeAI#LLM#AIchatbot#News#Technews#Technology#Technologynews#Technologytrends#Govindhtech
3 notes
·
View notes
Text
Generative AI in Customer Service Explained: The Technology, Tools, and Trends Powering the Future of Customer Support?
Customer service is undergoing a radical transformation, fueled by the rise of Generative AI. Gone are the days when customer queries relied solely on static FAQs or long wait times for human agents. With the emergence of large language models and AI-driven automation, businesses are now delivering faster, smarter, and more personalized support experiences.
But how exactly does generative AI work in customer service? What tools are leading the change? And what trends should you watch for?
Let’s explore the technology, tools, and trends that are powering the future of customer support through generative AI.
1. What Is Generative AI in Customer Service?
Generative AI refers to AI systems that can generate human-like responses, ideas, or content based on trained data. In customer service, it means AI tools that can:
Understand and respond to customer queries in real time
Provide contextual, conversational assistance
Summarize long interactions
Personalize responses based on customer history
Unlike traditional rule-based chatbots, generative AI adapts dynamically, making interactions feel more human and engaging.
2. Core Technologies Powering Generative AI in Support
A. Large Language Models (LLMs)
LLMs like GPT-4, Claude, and Gemini are the foundation of generative AI. Trained on massive datasets, they understand language context, tone, and nuances, enabling natural interactions with customers.
B. Natural Language Processing (NLP)
NLP allows machines to comprehend and interpret human language. It's what enables AI tools to read tickets, interpret intent, extract sentiment, and generate suitable responses.
C. Machine Learning (ML) Algorithms
ML helps customer service AI to learn from past interactions, identify trends in support tickets, and improve performance over time.
D. Knowledge Graphs and RAG (Retrieval-Augmented Generation)
These enhance the factual accuracy of AI outputs by allowing them to pull relevant data from enterprise databases, manuals, or FAQs before generating responses.
3. Popular Generative AI Tools in Customer Service
Here are some of the leading tools helping companies implement generative AI in their support workflows:
1. Zendesk AI
Integrates generative AI to assist agents with reply suggestions, automatic ticket summarization, and knowledge article recommendations.
2. Freshdesk Copilot
Freshworks’ AI copilot helps agents resolve issues by summarizing customer conversations and recommending next steps in real-time.
3. Salesforce Einstein GPT
Einstein GPT offers generative AI-powered replies across CRM workflows, including customer support, with real-time data from Salesforce’s ecosystem.
4. Intercom Fin AI Agent
Designed to fully automate common customer queries using generative AI, Fin delivers highly accurate answers and passes complex tickets to agents when necessary.
5. Ada
An automation platform that uses generative AI to build customer flows without coding, Ada enables instant support that feels personal.
4. Top Use Cases of Generative AI in Customer Support
✅ 24/7 Automated Support
Generative AI enables round-the-clock support without human intervention, reducing reliance on night shift teams.
✅ Ticket Summarization
AI can summarize lengthy email or chat threads, saving agents time and enabling faster resolution.
✅ Response Drafting
AI can instantly draft professional replies that agents can review and send, speeding up response times.
✅ Knowledge Article Creation
Generative models can help generate and update help articles based on customer queries and ticket data.
✅ Intent Detection and Routing
AI detects the user's intent and routes the query to the right department or agent, reducing miscommunication and wait times.
5. Business Benefits of Generative AI in Customer Service
Increased Efficiency: AI reduces the time spent on repetitive queries and ticket categorization.
Cost Savings: Fewer agents are required to manage high ticket volumes.
Improved CX: Customers get faster, more accurate answers—often without needing to escalate.
Scalability: AI handles volume spikes without service dips.
Continuous Learning: AI models improve over time with every new interaction.
6. Emerging Trends Shaping the Future
1. AI-Human Hybrid Support
Companies are combining generative AI with human oversight. AI handles simple queries while humans address emotional or complex issues.
2. Multilingual Support
LLMs are becoming fluent in multiple languages, enabling instant global customer support without translation delays.
3. Emotionally Intelligent AI
AI is beginning to detect customer tone and sentiment, allowing it to adjust responses accordingly—being empathetic when needed.
4. Voice-Powered AI Agents
Voice bots powered by generative AI are emerging as a new frontier, delivering seamless spoken interactions.
5. Privacy-Compliant AI
With regulations like GDPR, companies are deploying AI models with built-in privacy filters and localized deployments (e.g., Private LLMs).
7. Challenges and Considerations
Despite the advantages, generative AI in customer service comes with some challenges:
Hallucinations (Inaccurate Responses): LLMs can sometimes fabricate answers if not grounded in verified knowledge sources.
Data Security Risks: Sharing sensitive customer data with third-party models can raise compliance issues.
Need for Continuous Training: AI systems must be regularly updated to stay relevant and accurate.
Enterprises must monitor, fine-tune, and regulate AI systems carefully to maintain brand trust and service quality.
8. The Road Ahead: What to Expect
The future of customer service is AI-augmented, not AI-replaced. As generative AI tools mature, they’ll shift from assisting to proactively resolving customer needs—automating complex workflows like returns, disputes, and onboarding. Businesses that embrace this evolution today will lead in both cost-efficiency and customer satisfaction tomorrow.
Conclusion
Generative AI in customer service is redefining what excellent customer service looks like—making it faster, more personalized, and increasingly autonomous. Whether you're a startup or a global brand, adopting these tools early can offer a serious competitive edge.
0 notes
Text
The Ultimate Guide to AI Agent Development for Enterprise Automation in 2025
In the fast-evolving landscape of enterprise technology, AI agents have emerged as powerful tools driving automation, efficiency, and innovation. As we step into 2025, organizations are no longer asking if they should adopt AI agents—but how fast they can build and scale them across workflows.
This comprehensive guide unpacks everything you need to know about AI agent development for enterprise automation—from definitions and benefits to architecture, tools, and best practices.

🚀 What Are AI Agents?
AI agents are intelligent software entities that can autonomously perceive their environment, make decisions, and act on behalf of users or systems to achieve specific goals. Unlike traditional bots, AI agents can reason, learn, and interact contextually, enabling them to handle complex, dynamic enterprise tasks.
Think of them as your enterprise’s digital co-workers—automating tasks, communicating across systems, and continuously improving through feedback.
🧠 Why AI Agents Are Key to Enterprise Automation in 2025
1. Hyperautomation Demands Intelligence
Gartner predicts that by 2025, 70% of organizations will implement structured automation frameworks, where intelligent agents play a central role in managing workflows across HR, finance, customer service, IT, and supply chain.
2. Cost Reduction & Productivity Gains
Enterprises using AI agents report up to 40% reduction in operational costs and 50% faster task completion rates, especially in repetitive and decision-heavy processes.
3. 24/7 Autonomy and Scalability
Unlike human teams, AI agents work round-the-clock, handle large volumes of data, and scale effortlessly across cloud-based environments.
🏗️ Core Components of an Enterprise AI Agent
To develop powerful AI agents, understanding their architecture is key. The modern enterprise AI agent typically includes:
Perception Layer: Integrates with sensors, databases, APIs, or user input to observe its environment.
Reasoning Engine: Uses logic, rules, and LLMs (Large Language Models) to make decisions.
Planning Module: Generates action steps to achieve goals.
Action Layer: Executes commands via APIs, RPA bots, or enterprise applications.
Learning Module: Continuously improves via feedback loops and historical data.
🧰 Tools and Technologies for AI Agent Development in 2025
Developers and enterprises now have access to an expansive toolkit. Key technologies include:
🤖 LLMs (Large Language Models)
OpenAI GPT-4+, Anthropic Claude, Meta Llama 3
Used for task understanding, conversational interaction, summarization
🛠️ Agent Frameworks
LangChain, AutoGen, CrewAI, MetaGPT
Enable multi-agent systems, memory handling, tool integration
🧩 Integration Platforms
Zapier, Make, Microsoft Power Automate
Used for task automation and API-level integrations
🧠 RAG (Retrieval-Augmented Generation)
Enables agents to access external knowledge sources, ensuring context-aware and up-to-date responses
🔄 Vector Databases & Memory
Pinecone, Weaviate, Chroma
Let agents retain long-term memory and user-specific knowledge
🛠️ Steps to Build an Enterprise AI Agent in 2025
Here’s a streamlined process to develop robust AI agents tailored to your enterprise needs:
1. Define the Use Case
Start with a clear objective. Popular enterprise use cases include:
IT support automation
HR onboarding and management
Sales enablement
Invoice processing
Customer service response
2. Choose Your Agent Architecture
Decide between:
Single-agent systems (for simple tasks)
Multi-agent orchestration (for collaborative, goal-driven tasks)
3. Select the Right Tools
LLM provider (OpenAI, Anthropic)
Agent framework (LangChain, AutoGen)
Vector database for memory
APIs or RPA tools for action execution
4. Develop & Train
Build prompts or workflows
Integrate APIs and data sources
Train agents to adapt and improve from user feedback
5. Test and Deploy
Run real-world scenarios
Monitor behavior and adjust reasoning logic
Ensure enterprise-grade security, compliance, and scalability
🛡️ Security, Privacy, and Governance
As agents operate across enterprise systems, security and compliance must be integral to your development process:
Enforce role-based access control (RBAC)
Use private LLMs or secure APIs for sensitive data
Implement audit trails and logging for transparency
Regularly update models to prevent hallucinations or bias
📊 KPIs to Measure AI Agent Performance
To ensure ongoing improvement and ROI, track:
Task Completion Rate
Average Handling Time
Agent Escalation Rate
User Satisfaction (CSAT)
Cost Savings Per Workflow
🧩 Agentic AI: The Future of Enterprise Workflows
2025 marks the beginning of agentic enterprises, where AI agents become core building blocks of decision-making and operations. From autonomous procurement to dynamic scheduling, businesses are building systems where humans collaborate with agents, not just deploy them.
In the near future, we’ll see:
Goal-based agents with autonomy
Multi-agent systems negotiating outcomes
Cross-department agents driving insights
🏁 Final Thoughts: Start Building Now
AI agents are not just another automation trend—they are the new operating layer of enterprises. If you're looking to stay competitive in 2025 and beyond, investing in AI agent development is not optional. It’s strategic.
Start small, scale fast, and always design with your users and business outcomes in mind.
📣 Ready to Develop Your AI Agent?
Whether you're automating workflows, enhancing productivity, or creating next-gen customer experiences, building an AI agent tailored to your enterprise is within reach.
Partner with experienced AI agent developers to move from concept to implementation with speed, security, and scale.
0 notes
Text
{RAG vs.Fine-Tuning}: Which Approach Delivers Better Results for LLMs?
Imagine you’re building your dream home. You could either renovate an old house, making changes to the layout, adding new features, and fixing up what’s already there (Fine-Tuning), or you could start from scratch, using brand-new materials and designs to create something totally unique (RAG). In AI, Fine-Tuning means improving an existing model to work better for your specific needs, while Retrieval-Augmented Generation (RAG) adds external information to make the model smarter and more flexible. Just like with a home, which option {RAG vs.Fine-Tuning} you choose depends on what you want to achieve. Today, we’ll check out both the approaches to help you decide which one is right for your goals.
What Is LLM?
Large Language Models (LLMs) have taken the AI world by storm, capable of generating different types of content, answering queries, and even translating languages. As they are trained on extensive datasets, LLM showcase incredible versatility but they often struggle with outdated or context-specific information, limiting their effectiveness.
Key Challenges with LLMs:
LLMs can sometimes provide incorrect answers, even when sounding confident.
They may give responses that are off-target or irrelevant to the user's question.
LLMs rely on fixed datasets, leading to outdated or vague information that misses user specifics.
They can pull information from unreliable sources, risking the spread of misinformation.
Without understanding the context of a user’s question, LLMs might generate generic responses that are not helpful.
Different fields may use the same terms in various ways, causing misunderstandings in responses.
LLUMO AI's Eval LM makes it easy to test and compare different Large Language Models (LLMs). You can quickly view hundreds of outputs side by side to see which model performs best, and deliver accurate answers quickly, without losing quality.
How RAG Works?
Retrieval-augmented generation (RAG) is used to merge the strengths of generative models with retrieval-based systems. It retrieves relevant documents or data from an external database,websites or from any reliable source to enhance its responses and produce outputs not only accurate but also contextually latest and relevant.
A customer support chatbot that uses RAG, suppose a user asks about a specific product feature or service, the chatbot can quickly look up related FAQs, product manuals, and recent user reviews in its database. Combining this information creates a response that is latest, relevant, and helpful.
How RAG tackle LLM Challenges?
Retrieval-Augmented Generation (RAG) steps in to enhance LLMs and tackle these challenges:
Smart Retrieval: RAG first looks for the most relevant and up-to-date information from reliable sources, ensuring that responses are accurate.
Relevant Context: By giving the LLM specific, contextual data, RAG helps generate answers that are not only correct but also tailored to the user’s question.
Accuracy: With access to trustworthy sources, RAG greatly reduces the chances of giving false or misleading information, improving user trust.
Clarified Terminology: RAG uses diverse sources to help the LLM understand different meanings of terms, and minimizes the chances of confusion.
RAG turns LLMs into powerful tools that deliver precise, latest, and context-aware answers. This leads to better accuracy and consistency in LLM outputs. Think of it as a magic wand for today’s world, providing quick, relevant, and accurate answers right when you need them most.
How Fine-tuning Works?
Fine-tuning is a process where a pre-trained language model is adapted to a dataset relevant to a particular domain. It is particularly effective when you have a large amount of domain-specific data, allowing the model to perform exceptionally on that particular task. This process not only reduces computational costs but also allows users to tackle advanced models without starting from scratch.
A medical diagnosis tool designed for healthcare professionals. By fine-tuning a LLM on a dataset of patient records and medical literature, the model can learn that particular medical terminology and generate insights based on specific symptoms. For example, when a physician inputs symptoms, the fine-tuned model can offer potential diagnoses and treatment options tailored to that specific context.
How Fine-Tuning Makes a Difference in LLM
Fine-tuning is a powerful way to enhance LLMs and tackle these challenges effectively:
Tailored Training: Fine-tuning allows LLMs to be trained on specific datasets that reflect the specific information they’ll need to provide. This means they can learn the most relevant knowledge of the particular.
Improved Accuracy: By focusing on the right data, fine-tuning helps LLMs to deliver more precise answers that directly address user questions, and reduces the chances of misinformation.
Context Awareness: Fine-tuning helps LLMs to understand the context better, so they can generate most relevant and appropriate responses.
Clarified Terminology: With targeted training, LLMs can learn the nuances of different terms and phrases, helping them avoid confusion and provide clearer answers.
Fine-tuning works like a spell, transforming LLMs into powerful allies that provide answers that are not just accurate, but also deeply relevant and finely attuned to context. This enchanting enhancement elevates the user experience to new heights, creating a seamless interaction that feels almost magical.
How can LLumo AI help you?
In {RAG vs.Fine-Tuning}, LLUMO can help you gain complete insights on your LLM outputs and customer success using proprietary framework- Eval LM. To use LLumo Eval LM and evaluate your prompt output to generate insights needs follow these steps:
Step 1: Create a New Playground
Go to the Eval LM platform.
Click on the option to create a new playground. This is your workspace for generating and evaluating experiments.
Step 2: Choose How to Upload Your Data
In your new playground, you have three options for uploading your data:
Upload Your Data:
Simply drag and drop your file into the designated area. This is the quickest way to get your data in.
Choose a Template:
Select a template that fits your project. Once you've chosen one, upload your data file to use it with that template.
Customize Your Template:
If you want to tailor the template to your needs, you can add or remove columns. After customizing, upload your data file.
Step 3: Generate Responses
After uploading your data, click the button to run the process. This will generate responses based on your input.
Step 4: Evaluate Your Responses
Once the responses are generated, you can evaluate them using over 50 customizable Key Performance Indicators (KPIs).
You can define what each KPI means to you, ensuring it fits your evaluation criteria.
Step 5: Set Your Metrics
Choose the evaluation metrics you want to use. You can also select the language model (LLM) for generating responses.
After setting everything, you'll receive an evaluation score that indicates whether the responses pass or fail based on your criteria.
Step 6: Finalize and Run
Once you’ve completed all the setup, simply click on “Run.”
Your tailored responses are now ready for your specific niche!
Step 6: Evaluate you Accuracy Score
After generating responses, you can easily check how accurate they are. You can set your own rules to decide what counts as a good response, giving you full control over accuracy.
Why Choose Retrieval-Augmented Generation (RAG) in {RAG vs.Fine-Tuning}?
On a frequent basis, AI developers used to face challenges like data privacy, managing costs, and delivering accurate outputs. RAG effectively addresses these by offering a secure environment for data handling, reducing resource requirements, and enhancing the reliability of results. By choosing RAG over fine-tuning in {RAG vs.Fine-Tuning},companies can not only improve their operational efficiency but also build trust with their users through secure and accurate AI solutions.
While choosing {RAG vs.Fine-Tuning}, Retrieval-Augmented Generation (RAG) often outshines fine-tuning. This is primarily due to its security, scalability, reliability, and efficiency. Let's explore each of these with real-world use cases.
Data Security and Data Privacy
One of the biggest concerns for AI developers is data security. With fine-tuning, the proprietary data used to train the model becomes part of the model’s training set. This means there’s a risk of that data being exposed, potentially leading to security breaches or unauthorized access. In contrast, RAG keeps your data within a secured database environment.
Imagine a healthcare company using AI to analyze patient records. By using RAG, the company can pull relevant information securely without exposing sensitive patient data. This means they can generate insights or recommendations while ensuring patient confidentiality, thus complying with regulations like HIPAA.
Cost-Efficient and Scalable
Fine-tuning a large AI model takes a lot of time and resources because it needs labeled data and a lot of work to set up. RAG, however, can use the data you already have to give answers without needing a long training process. For example, an e-commerce company that wants to personalize customer experiences doesn’t have to spend weeks fine-tuning a model with customer data. Instead, they can use RAG to pull information from their existing product and customer data. This helps them provide personalized recommendations faster and at a lower cost, making things more efficient.
Reliable Response
The effectiveness of AI is judged by its ability to provide accurate and reliable responses. RAG excels in this aspect by consistently referencing the latest curated datasets to generate outputs. If an error occurs, it’s easier for the data team to trace the source of the response back to the original data, helping them understand what went wrong.
Take a financial advisory firm that uses AI to provide investment recommendations. By employing RAG, the firm can pull real-time market data and financial news to inform its advice. If a recommendation turns out to be inaccurate, the team can quickly identify whether the error stemmed from outdated information or a misinterpretation of the data, allowing for swift corrective action.
Let’s Check Out the Key Points to Evaluate {RAG vs.Fine-Tuning}
Here’s a simple tabular comparison between Retrieval-Augmented Generation (RAG) and Fine-Tuning:
Feature
Retrieval-Augmented Generation (RAG)
Fine-Tuning
Data Security
Keeps proprietary data within a secured database.
Data becomes part of the model, risking exposure.
Cost Efficiency
Lower costs by leveraging existing data; no training required.
Resource-intensive, requiring time and compute power.
Scalability
Easily scalable as it uses first-party data dynamically.
Scaling requires additional training and resources.
Speed of Implementation
Faster to implement; no lengthy training process.
Slower due to the need for extensive data preparation.
Accuracy of Responses
Pulls from the latest data for accurate outputs.
Performance may vary based on training data quality.
Error Tracking
Easier to trace errors back to specific data sources.
Harder to identify where things went wrong.
Use Case Flexibility
Adapts quickly to different tasks without retraining.
Best for specific tasks but less adaptable.
Development Effort
Requires less human effort and time.
High human effort needed for labeling and training.
Summing Up
Choosing between {RAG vs.Fine-Tuning},ultimately depends on your specific needs and resources. RAG is time and again the better option because it keeps your data safe, is more cost-effective, and can quickly adapt the latest information. This means it can provide accurate and relevant answers based on the latest data, which keeps you update.
On the other hand, Fine-Tuning is great for specific tasks but can be resource-heavy and less flexible. It shines in niche areas, but it doesn't handle changes as well as RAG does. Overall, RAG usually offers more capabilities for a wider range of needs. With LLUMO AI’s Eval LM, you can easily evaluate and compare model performance, helping you optimize both approaches. LLUMO’s tools ensure your AI delivers accurate, relevant results while saving time and resources, regardless of the method you choose
0 notes
Text
[ad_1] L&T Finance Limited (LTF), a leading Non-Banking Financial Company (NBFC), is transforming the home loan experience with the launch of Knowledgeable AI (KAI), an AI-powered virtual advisor, on its newly redesigned corporate website (www.ltfinance.com/home-loan). KAI, initially unveiled during LTF’s RAISE’ 24 event, represents a significant leap in leveraging AI to streamline and personalize the home loan journey. This innovation underscores LTF's commitment to providing cutting-edge solutions that empower customers and simplify the often-complex process of securing a home loan. L&T Finance's Knowledgeable AI (KAI) mascot Designed to tackle challenges such as complex terminology, intricate calculations, and lengthy application processes, KAI specifically addresses the needs of first-time buyers, making the journey to homeownership smoother and more accessible. It offers prospective homebuyers an intuitive, efficient, and user-friendly experience, delivering instant support and expert guidance at their fingertips. KAI utilizes advanced AI technology, including a specialized Large Language Model (LLM), to understand the unique needs of each user. This allows KAI to provide instant EMI calculations and loan estimates, expert answers to home loan questions, and to provide context and guidance, acting as a home loan guide rather than a mere chatbot. Mr. Sudipta Roy, Managing Director & CEO at LTF said, "We are pleased to launch KAI, a testament to our dedication to improving customer engagement and streamlining financial processes. With KAI, we are not just launching a chatbot; we are offering a personalized, 24/7 guide to help potential home buyers navigate the often-confusing home loan process. Our goal is to make the home buying journey simple, efficient, and accessible. What makes KAI truly unique is its ability to not only provide immediate responses to specific queries related to LTF's home loans but also offer guidance on a spectrum of related home loan topics.” KAI goes beyond basic chatbot functionality by drawing information from LTF documents (using latest RAG technology) and providing smooth EMI calculations using interactive sliders. Users can conveniently download EMI schedules and bookmark preferred options. KAI provides conversational style answers making it easy to understand for a broad base of users, and it can also handle follow-up questions seamlessly ensuring a comprehensive and user-friendly experience. About L&T Finance Ltd. (LTF) L&T Finance Ltd. (LTF) (www.ltfs.com), formerly known as L&T Finance Holdings Ltd., is a leading Non-Banking Financial Company (NBFC), offering a range of financial products and services. Headquartered in Mumbai, the Company has been rated ‘AAA’ — the highest credit rating for NBFCs — by four leading rating agencies. It has also received leadership scores and ratings by global and national Environmental, Social, and Governance (ESG) rating providers for its sustainability performance. The Company has been certified as a Great Place To Work® and has also won many prestigious awards for its flagship CSR project – “Digital Sakhi”- which focuses on women's empowerment and digital and financial inclusion. Under Right to Win, being in the ‘right businesses’ has helped the Company become one of the leading financiers in key Retail products. The Company is focused on creating a top-class, digitally enabled, Retail finance company as part of the Lakshya 2026 plan. The goal is to move the emphasis from product focus to customer focus and establish a robust Retail portfolio with quality assets, thus creating a Fintech@Scale while keeping ESG at the core. Fintech@Scale is one of the pillars of the Company’s strategic roadmap - Lakshya 2026. The Company has approximately 2.5 Crore customer database, which is being leveraged to cross-sell, up-sell, and identify new customers.Facebook: LnTFS Twitter: LnTFinance YouTube: Ltfinance
LinkedIn: L&TFinance Instagram: Lntfinance !function(f,b,e,v,n,t,s) if(f.fbq)return;n=f.fbq=function()n.callMethod? n.callMethod.apply(n,arguments):n.queue.push(arguments); if(!f._fbq)f._fbq=n;n.push=n;n.loaded=!0;n.version='2.0'; n.queue=[];t=b.createElement(e);t.async=!0; t.src=v;s=b.getElementsByTagName(e)[0]; s.parentNode.insertBefore(t,s)(window,document,'script', 'https://connect.facebook.net/en_US/fbevents.js'); fbq('init', '311356416665414'); fbq('track', 'PageView'); [ad_2] Source link
0 notes
Text
[ad_1] L&T Finance Limited (LTF), a leading Non-Banking Financial Company (NBFC), is transforming the home loan experience with the launch of Knowledgeable AI (KAI), an AI-powered virtual advisor, on its newly redesigned corporate website (www.ltfinance.com/home-loan). KAI, initially unveiled during LTF’s RAISE’ 24 event, represents a significant leap in leveraging AI to streamline and personalize the home loan journey. This innovation underscores LTF's commitment to providing cutting-edge solutions that empower customers and simplify the often-complex process of securing a home loan. L&T Finance's Knowledgeable AI (KAI) mascot Designed to tackle challenges such as complex terminology, intricate calculations, and lengthy application processes, KAI specifically addresses the needs of first-time buyers, making the journey to homeownership smoother and more accessible. It offers prospective homebuyers an intuitive, efficient, and user-friendly experience, delivering instant support and expert guidance at their fingertips. KAI utilizes advanced AI technology, including a specialized Large Language Model (LLM), to understand the unique needs of each user. This allows KAI to provide instant EMI calculations and loan estimates, expert answers to home loan questions, and to provide context and guidance, acting as a home loan guide rather than a mere chatbot. Mr. Sudipta Roy, Managing Director & CEO at LTF said, "We are pleased to launch KAI, a testament to our dedication to improving customer engagement and streamlining financial processes. With KAI, we are not just launching a chatbot; we are offering a personalized, 24/7 guide to help potential home buyers navigate the often-confusing home loan process. Our goal is to make the home buying journey simple, efficient, and accessible. What makes KAI truly unique is its ability to not only provide immediate responses to specific queries related to LTF's home loans but also offer guidance on a spectrum of related home loan topics.” KAI goes beyond basic chatbot functionality by drawing information from LTF documents (using latest RAG technology) and providing smooth EMI calculations using interactive sliders. Users can conveniently download EMI schedules and bookmark preferred options. KAI provides conversational style answers making it easy to understand for a broad base of users, and it can also handle follow-up questions seamlessly ensuring a comprehensive and user-friendly experience. About L&T Finance Ltd. (LTF) L&T Finance Ltd. (LTF) (www.ltfs.com), formerly known as L&T Finance Holdings Ltd., is a leading Non-Banking Financial Company (NBFC), offering a range of financial products and services. Headquartered in Mumbai, the Company has been rated ‘AAA’ — the highest credit rating for NBFCs — by four leading rating agencies. It has also received leadership scores and ratings by global and national Environmental, Social, and Governance (ESG) rating providers for its sustainability performance. The Company has been certified as a Great Place To Work® and has also won many prestigious awards for its flagship CSR project – “Digital Sakhi”- which focuses on women's empowerment and digital and financial inclusion. Under Right to Win, being in the ‘right businesses’ has helped the Company become one of the leading financiers in key Retail products. The Company is focused on creating a top-class, digitally enabled, Retail finance company as part of the Lakshya 2026 plan. The goal is to move the emphasis from product focus to customer focus and establish a robust Retail portfolio with quality assets, thus creating a Fintech@Scale while keeping ESG at the core. Fintech@Scale is one of the pillars of the Company’s strategic roadmap - Lakshya 2026. The Company has approximately 2.5 Crore customer database, which is being leveraged to cross-sell, up-sell, and identify new customers.Facebook: LnTFS Twitter: LnTFinance YouTube: Ltfinance
LinkedIn: L&TFinance Instagram: Lntfinance !function(f,b,e,v,n,t,s) if(f.fbq)return;n=f.fbq=function()n.callMethod? n.callMethod.apply(n,arguments):n.queue.push(arguments); if(!f._fbq)f._fbq=n;n.push=n;n.loaded=!0;n.version='2.0'; n.queue=[];t=b.createElement(e);t.async=!0; t.src=v;s=b.getElementsByTagName(e)[0]; s.parentNode.insertBefore(t,s)(window,document,'script', 'https://connect.facebook.net/en_US/fbevents.js'); fbq('init', '311356416665414'); fbq('track', 'PageView'); [ad_2] Source link
0 notes
Text
AI in eCommerce: Top Use Cases, Challenges, and Benefits

Artificial Intelligence and eCommerce are a match made in heaven. AI in eCommerce has been prevalent for years, with giants like Amazon leveraging it to the fullest. In 2024, it has evolved to an extent where its feats seemed impossible just a few years ago.
Integrating AI in eCommerce empowers you to deliver a truly optimized customer experience. The possibilities for what it can achieve are limitless.
The Pandemic Shift
The pandemic was a turning point for the eCommerce industry. It made online shopping the only viable option. The eCommerce sales increased by a whopping 43% in 2020. Even though the pandemic is over, people have adopted this new way of shopping. The convenience, variety, and safety offered by online shopping have captivated a vast audience.
As we navigate the post-pandemic era, AI has emerged as a serious upgrade. It has the potential to solve many persisting eCommerce problems.
Challenges in the eCommerce Industry
While the eCommerce industry has witnessed rapid growth and widespread adoption, it’s not without its challenges. Some of the most pressing issues include:
1) Customer Acquisition and Retention
Acquiring customers in the eCommerce industry requires significant marketing investments, making it a costly endeavour. At the same time, retaining existing customers and turning them into loyal brand advocates can be difficult, especially in a highly competitive market.
2) Personalization and Relevance
A vast catalog and ever-changing customer expectations make it difficult to provide personalized customer experiences. Delivering content that resonates with individual customers can be time-consuming.
3) Inventory Management
Demand forecasting is critical to avoid stockouts and overstocking. It’s a feat to balance inventory levels to meet customer needs while minimizing holding costs.
4) Fraud Detection
Online shopping can bring with it shenanigans such as identity theft and credit card fraud, which can be hard to detect manually or with traditional protocols.
5) Supply Chain Optimization
Optimizing supply chain operations, including transportation, warehousing, and delivery, is crucial to ensuring timely and cost-effective delivery.
AI in eCommerce: The Much-Needed Upgrade
AI has emerged as a powerful tool for businesses, and it’s going to be the differentiator. It’s not about AI transforming eCommerce, but about businesses adopting the AI disruption happening right now.
AI offers a plethora of features that benefit each and every process of the eCommerce sector. From customer service to supply chain management, all sectors can amplify their operations.
Here are the different types of AI technologies that will benefit eCommerce businesses:
Natural Language Processing (NLP)
Large Language Model (LLM)
Machine Learning
Data Engineering
Retrieval Augmented Generation (RAG)
Why Integrate AI in eCommerce?
As an eCommerce business, integrating AI is the best decision you can make at this point in time. AI algorithms can automate many tasks, such as product recommendations, sales processes, advertising, and more. Results: Reduced time and expenses.
As we’ll discuss soon, AI can help you top your top line, increase customer retention, and enhance efficiency when deployed in the right places. eCommerce businesses like Amazon and eBay have been using AI for years to keep customers engaged. Now’s the time to leverage AI and transform the way you do business online.
In this blog, we’ll look at the five best use cases of AI for eCommerce.
Top 12 Use Cases of AI in eCommerce
eCommerce was the next step after retail, and AI-powered eCommerce is the step further. It offers new opportunities to enhance CX, automate repetitive tasks, and spike revenue. Let’s have a look at the top 12 use cases of Artificial Intelligence in e-commerce.
1) Personalized Product Recommendations
eCommerce giants have been using AI to personalize product recommendations for ages. The compounded advancements in technology have taken it to a whole new level. It’s the era of Hype personalization.
Companies that implement personalization correctly generate 40% more revenue than those that don’t. Let’s understand how AI helps you achieve that number.
AI integration in eCommerce can track even the smallest customer interactions, such as clicks, searches, and purchases. Based on this, it predicts exactly what the customer likes and wants next. It recommends the exact product, price, and time to pitch so that the customer can’t resist buying.
Hyper personalization helps customers find products easily while recommending other products they are interested in. This results in increased sales and customer loyalty.
2) Demand Forecasting
The market can be very unpredictable at times. Integrating AI in eCommerce can help you navigate the uncertainties.
Predictive AI algorithms analyze vast amounts of transactional and behavioral data in real-time to forecast demand. You’ll know exactly the product and quantity to stock up on to maximize revenue. Stockouts and stockpiling are things of the past.
All of this is possible, as machine learning can identify patterns and trends that humans might miss.
3) Optimized Marketing Approach
In traditional retail, shoppers observe customers, make deductions like Sherlock Holmes, and recommend the right products. AI in the eCommerce industry does the same.
AI can analyze every micro-interaction and predict the customer’s next step. Crack the code to pricing and promotions leveraging predictive analytics. AI tells you exactly which product to pitch at what price so that the customer will surely buy it.
Did someone leave a cart stranded in the hallway? AI can tell you what to pitch to bring the customer back.
AI can also help you make your existing happy customers happier with smart cross-selling and upselling.
AI is a powerful tool that can empower your sales team and save them a ton of time. Only 33% of a sales rep’s time is spent selling. The rest is spent finding the right prospects. AI can change the script.
4) Refined Customer Service
A major roadblock in optimizing customer service in eCommerce is the sheer volume of queries you get. It’s next to impossible for a support team to be available all the time. But AI can.
Generative AI-powered agents are accessible 24/7 and provide personalized responses to user queries. They can handle complex queries that involve product specifications and guidance. Your support team can focus on handling more important matters that require human intervention.
The best part? Agents are very effective in upselling, as they can recommend other products the user might be interested in.
To read full article, visit this link: Top Use Cases of AI in eCommerce
1 note
·
View note
Text
Revolutionizing Business with Custom Language Models
The business world has been transformed by the advent of Large Language Models (LLMs), powerful AI tools that have redefined how companies interact with data and natural language. While general-purpose LLMs like GPT-3 have garnered significant attention, they come with limitations that can hinder their effectiveness in specialized business contexts.
The Challenge of General-Purpose LLMs
Despite their impressive capabilities, general LLMs face two primary challenges in business applications:
Hallucinations: These models can sometimes generate false or misleading information, which is particularly problematic in business settings where accuracy is crucial.
Lack of Specialization: The "one-size-fits-all" approach often fails to address the unique needs and terminology of specific industries.
Retrieval-Augmented Generation: A Game-Changer
To overcome these limitations, innovative AI consultants are turning to Retrieval-Augmented Generation (RAG). This technique enhances LLMs by providing them with context from relevant document corpuses. Here's how it works:
Utilizes vector databases and word embeddings
Translates complex textual information into mathematical space
Enables LLMs to leverage domain-specific knowledge with increased accuracy
Transforming Internal Documentation into Actionable Intelligence
By incorporating tailored LLMs with retrieval systems, businesses can unlock the potential of their internal documentation. This approach can lead to significant improvements in various areas:
Legal document analysis
Technical support
Personalized customer service
Enhanced decision-making processes
These custom models understand a company's unique language, goals, and nuances, turning vast repositories of information into valuable, actionable intelligence.
The Future of AI in Business
As Large Language Models continue to evolve and gain traction, they are becoming a crucial focus for companies aiming to innovate and streamline operations through AI adoption. However, to truly harness the power of these technologies, businesses need to look beyond off-the-shelf solutions.
Developing effective LLM and AI strategies requires expertise in tailoring these models to individual business needs. By working with experienced AI consultants, companies can create custom language models that not only avoid the pitfalls of general-purpose LLMs but also provide a significant competitive advantage in their respective industries.
In conclusion, while general LLMs have opened new possibilities, it's the customized, retrieval-augmented models that will truly revolutionize how businesses operate, make decisions, and serve their customers in the AI-driven future.
0 notes
Text
The Mistral AI New Model Large-Instruct-2411 On Vertex AI
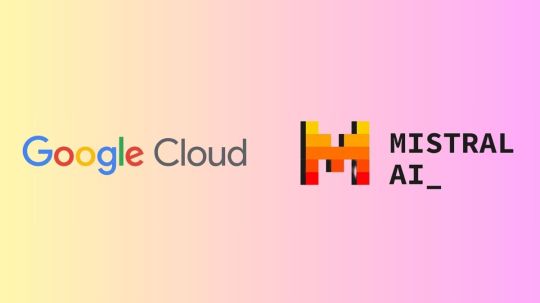
Introducing the Mistral AI New Model Large-Instruct-2411 on Vertex AI from Mistral AI
Mistral AI’s models, Codestral for code generation jobs, Mistral Large 2 for high-complexity tasks, and the lightweight Mistral Nemo for reasoning tasks like creative writing, were made available on Vertex AI in July. Google Cloud is announcing that the Mistral AI new model is now accessible on Vertex AI Model Garden: Mistral-Large-Instruct-2411 is currently accessible to the public.
Large-Instruct-2411 is a sophisticated dense large language model (LLM) with 123B parameters that extends its predecessor with improved long context, function calling, and system prompt. It has powerful reasoning, knowledge, and coding skills. The approach is perfect for use scenarios such as big context applications that need strict adherence for code generation and retrieval-augmented generation (RAG), or sophisticated agentic workflows with exact instruction following and JSON outputs.
The new Mistral AI Large-Instruct-2411 model is available for deployment on Vertex AI via its Model-as-a-Service (MaaS) or self-service offering right now.
With the new Mistral AI models on Vertex AI, what are your options?
Using Mistral’s models to build atop Vertex AI, you can:
Choose the model that best suits your use case: A variety of Mistral AI models are available, including effective models for low-latency requirements and strong models for intricate tasks like agentic processes. Vertex AI simplifies the process of assessing and choosing the best model.
Try things with assurance: Vertex AI offers fully managed Model-as-a-Service for Mistral AI models. Through straightforward API calls and thorough side-by-side evaluations in its user-friendly environment, you may investigate Mistral AI models.
Control models without incurring extra costs: With pay-as-you-go pricing flexibility and fully managed infrastructure built for AI workloads, you can streamline the large-scale deployment of the new Mistral AI models.
Adjust the models to your requirements: With your distinct data and subject expertise, you will be able to refine Mistral AI’s models to produce custom solutions in the upcoming weeks.
Create intelligent agents: Using Vertex AI’s extensive toolkit, which includes LangChain on Vertex AI, create and coordinate agents driven by Mistral AI models. To integrate Mistral AI models into your production-ready AI experiences, use Genkit’s Vertex AI plugin.
Construct with enterprise-level compliance and security: Make use of Google Cloud’s integrated privacy, security, and compliance features. Enterprise controls, like the new organization policy for Vertex AI Model Garden, offer the proper access controls to guarantee that only authorized models are accessible.
Start using Google Cloud’s Mistral AI models
Google Cloud’s dedication to open and adaptable AI ecosystems that assist you in creating solutions that best meet your needs is demonstrated by these additions. Its partnership with Mistral AI demonstrates its open strategy in a cohesive, enterprise-ready setting. Many of the first-party, open-source, and third-party models offered by Vertex AI, including the recently released Mistral AI models, can be provided as a fully managed Model-as-a-service (MaaS) offering, giving you enterprise-grade security on its fully managed infrastructure and the ease of a single bill.
Mistral Large (24.11)
The most recent iteration of the Mistral Large model, known as Mistral Large (24.11), has enhanced reasoning and function calling capabilities.
Mistral Large is a sophisticated Large Language Model (LLM) that possesses cutting-edge knowledge, reasoning, and coding skills.
Intentionally multilingual: English, French, German, Spanish, Italian, Chinese, Japanese, Korean, Portuguese, Dutch, Polish, Arabic, and Hindi are among the dozens of languages that are supported.
Multi-model capability: Mistral Large 24.11 maintains cutting-edge performance on text tasks while excelling at visual comprehension.
Competent in coding: Taught more than 80 coding languages, including Java, Python, C, C++, JavaScript, and Bash. Additionally, more specialized languages like Swift and Fortran were taught.
Agent-focused: Top-notch agentic features, including native function calls and JSON output.
Sophisticated reasoning: Cutting-edge reasoning and mathematical skills.
Context length: 128K is the most that Mistral Large can support.
Use cases
Agents: Made possible by strict adherence to instructions, JSON output mode, and robust safety measures
Text: Creation, comprehension, and modification of synthetic text
RAG: Important data is preserved across lengthy context windows (up to 128K tokens).
Coding includes creating, finishing, reviewing, and commenting on code. All popular coding languages are supported.
Read more on govindhtech.com
#MistralAI#ModelLarge#VertexAI#MistralLarge2#Codestral#retrievalaugmentedgeneration#RAG#VertexAIModelGarden#LargeLanguageModel#LLM#technology#technews#news#govindhtech
0 notes
Text
AI Agent Development: A Complete Guide to Building Smart, Autonomous Systems in 2025
Artificial Intelligence (AI) has undergone an extraordinary transformation in recent years, and 2025 is shaping up to be a defining year for AI agent development. The rise of smart, autonomous systems is no longer confined to research labs or science fiction — it's happening in real-world businesses, homes, and even your smartphone.
In this guide, we’ll walk you through everything you need to know about AI Agent Development in 2025 — what AI agents are, how they’re built, their capabilities, the tools you need, and why your business should consider adopting them today.
What Are AI Agents?
AI agents are software entities that perceive their environment, reason over data, and take autonomous actions to achieve specific goals. These agents can range from simple chatbots to advanced multi-agent systems coordinating supply chains, running simulations, or managing financial portfolios.
In 2025, AI agents are powered by large language models (LLMs), multi-modal inputs, agentic memory, and real-time decision-making, making them far more intelligent and adaptive than their predecessors.
Key Components of a Smart AI Agent
To build a robust AI agent, the following components are essential:
1. Perception Layer
This layer enables the agent to gather data from various sources — text, voice, images, sensors, or APIs.
NLP for understanding commands
Computer vision for visual data
Voice recognition for spoken inputs
2. Cognitive Core (Reasoning Engine)
The brain of the agent where LLMs like GPT-4, Claude, or custom-trained models are used to:
Interpret data
Plan tasks
Generate responses
Make decisions
3. Memory and Context
Modern AI agents need to remember past actions, preferences, and interactions to offer continuity.
Vector databases
Long-term memory graphs
Episodic and semantic memory layers
4. Action Layer
Once decisions are made, the agent must act. This could be sending an email, triggering workflows, updating databases, or even controlling hardware.
5. Autonomy Layer
This defines the level of independence. Agents can be:
Reactive: Respond to stimuli
Proactive: Take initiative based on context
Collaborative: Work with other agents or humans
Use Cases of AI Agents in 2025
From automating tasks to delivering personalized user experiences, here’s where AI agents are creating impact:
1. Customer Support
AI agents act as 24/7 intelligent service reps that resolve queries, escalate issues, and learn from every interaction.
2. Sales & Marketing
Agents autonomously nurture leads, run A/B tests, and generate tailored outreach campaigns.
3. Healthcare
Smart agents monitor patient vitals, provide virtual consultations, and ensure timely medication reminders.
4. Finance & Trading
Autonomous agents perform real-time trading, risk analysis, and fraud detection without human intervention.
5. Enterprise Operations
Internal copilots assist employees in booking meetings, generating reports, and automating workflows.
Step-by-Step Process to Build an AI Agent in 2025
Step 1: Define Purpose and Scope
Identify the goals your agent must accomplish. This defines the data it needs, actions it should take, and performance metrics.
Step 2: Choose the Right Model
Leverage:
GPT-4 Turbo or Claude for text-based agents
Gemini or multimodal models for agents requiring image, video, or audio processing
Step 3: Design the Agent Architecture
Include layers for:
Input (API, voice, etc.)
LLM reasoning
External tool integration
Feedback loop and memory
Step 4: Train with Domain-Specific Knowledge
Integrate private datasets, knowledge bases, and policies relevant to your industry.
Step 5: Integrate with APIs and Tools
Use plugins or tools like LangChain, AutoGen, CrewAI, and RAG pipelines to connect agents with real-world applications and knowledge.
Step 6: Test and Simulate
Simulate environments where your agent will operate. Test how it handles corner cases, errors, and long-term memory retention.
Step 7: Deploy and Monitor
Run your agent in production, track KPIs, gather user feedback, and fine-tune the agent continuously.
Top Tools and Frameworks for AI Agent Development in 2025
LangChain – Chain multiple LLM calls and actions
AutoGen by Microsoft – For multi-agent collaboration
CrewAI – Team-based autonomous agent frameworks
OpenAgents – Prebuilt agents for productivity
Vector Databases – Pinecone, Weaviate, Chroma for long-term memory
LLMs – OpenAI, Anthropic, Mistral, Google Gemini
RAG Pipelines – Retrieval-Augmented Generation for knowledge integration
Challenges in Building AI Agents
Even with all this progress, there are hurdles to be aware of:
Hallucination: Agents may generate inaccurate information.
Context loss: Long conversations may lose relevancy without strong memory.
Security: Agents with action privileges must be protected from misuse.
Ethical boundaries: Agents must be aligned with company values and legal standards.
The Future of AI Agents: What’s Coming Next?
2025 marks a turning point where AI agents move from experimental to mission-critical systems. Expect to see:
Personalized AI Assistants for every employee
Decentralized Agent Networks (Autonomous DAOs)
AI Agents with Emotional Intelligence
Cross-agent Collaboration in real-time enterprise ecosystems
Final Thoughts
AI agent development in 2025 isn’t just about automating tasks — it’s about designing intelligent entities that can think, act, and grow autonomously in dynamic environments. As tools mature and real-time data becomes more accessible, your organization can harness AI agents to unlock unprecedented productivity and innovation.
Whether you’re building an internal operations copilot, a trading agent, or a personalized shopping assistant, the key lies in choosing the right architecture, grounding the agent in reliable data, and ensuring it evolves with your needs.
1 note
·
View note
Text
Data Analytics Strategy
Our Data Analytics Services are designed to help organizations extract valuable insights from their data, leveraging a combination of computer skills, mathematics, statistics, descriptive techniques, and predictive models. These insights are then used to recommend actions or guide decision-making processes within a business context. Data Analytics Strategy
Key Components and Execution
1. Advanced Analytics and Insights
Descriptive Analytics: We utilize descriptive techniques to summarize historical data and identify patterns. This provides a clear picture of what has happened in the past, helping businesses understand trends and behaviors.
Predictive Analytics: Implementing predictive models, we analyze current and historical data to make forecasts about future events. Techniques such as machine learning, regression analysis, and time series forecasting are used to predict outcomes and trends.
Prescriptive Analytics: We provide actionable recommendations based on data analysis. By using optimization and simulation algorithms, we help businesses determine the best course of action among various options.
2. Machine Learning and Artificial Intelligence (AI)
Machine Learning Models: Leveraging machine learning techniques, we build models that can learn from data and improve over time. These models are used for tasks such as classification, regression, clustering, and anomaly detection.
AI Integration: Implementing AI solutions to automate processes, enhance decision-making, and drive innovation. AI technologies are used for natural language processing (NLP), image recognition, and other advanced applications.
Large Language Models (LLM): Utilizing advanced NLP models like GPT-4 for tasks such as text generation, summarization, and sentiment analysis. These models help in understanding and generating human language, providing deeper insights from textual data.
3. Data Science
Statistical Analysis: Utilizing statistical methods to interpret complex data and uncover hidden patterns. Our data scientists apply techniques such as hypothesis testing, variance analysis, and multivariate analysis to gain deeper insights.
Data Visualization: Creating interactive and intuitive data visualizations to help stakeholders understand data insights quickly. Tools like Tableau, Power BI, and D3.js are used to build dashboards and visual reports.
4. Real-time Analytics
Stream Processing: Leveraging technologies such as Apache Kafka, Apache Flink, and Spark Streaming, we handle real-time data ingestion and processing. This enables immediate analysis and insights, crucial for time-sensitive decisions.
Event-driven Architectures: Implementing event-driven systems to process and analyze data in real-time. This supports the development of responsive applications and systems that can react to changes as they occur.
5. Big Data and Analytics Platforms
Big Data Technologies: Utilizing Hadoop ecosystems and Spark for big data processing and analytics. These technologies allow us to handle and analyze large volumes of data efficiently.
Analytics Platforms: Deploying platforms like Databricks, Snowflake, and Google BigQuery for advanced analytics and machine learning integration. These platforms provide robust environments for executing complex data analytics tasks.
6. Recent Techniques in Analytics
Retrieval-Augmented Generation (RAG): Integrating RAG models to improve information retrieval and provide accurate, context-aware responses. This technique combines large language models with document retrieval systems to enhance the quality and relevance of generated outputs.
Edge Analytics: Performing data processing at the edge of the network, near the data source, to reduce latency and bandwidth usage. This technique is especially useful for IoT applications and real-time decision-making.
AutoML: Leveraging automated machine learning tools to streamline the model development process, making it more accessible and efficient. AutoML helps in selecting the best algorithms and hyperparameters, reducing the time and expertise required for building machine learning models.
Execution Approach
Assessment and Planning: Conducting a thorough assessment of your current data analytics capabilities and identifying key areas for improvement. We develop a tailored analytics strategy that aligns with your business goals. Data Strategy and Analytics
Implementation and Integration: Implementing advanced analytics solutions and integrating them into your existing systems. This includes setting up data pipelines, building predictive models, and developing visualization tools.
Optimization and Monitoring: Continuously monitoring and optimizing analytics processes to ensure they deliver the expected value. We use performance metrics and feedback loops to refine models and techniques.
Training and Support: Providing training to your team on the new analytics tools and methodologies. We offer ongoing support to ensure the effective use and continuous improvement of your data analytics capabilities.
0 notes
Text
Supercharging Generative AI: The Power of NVIDIA RTX AI PCs and Cloud Workstations

Introduction
Generative AI is revolutionizing the world of Windows applications and gaming. It’s enabling dynamic NPCs, helping creators generate new art, and boosting gamers’ frame rates by up to 4x. But this is just the beginning. As the capabilities and use cases for generative AI grow, so does the demand for robust compute resources. Enter NVIDIA RTX AI PCs and workstations that tap into the cloud to supercharge these AI-driven experiences. Let’s dive into how hybrid AI solutions combine local and cloud-based computing to meet the evolving demands of AI workloads.
Hybrid AI: A Match Made in Tech Heaven
As AI adoption continues to rise, developers need versatile deployment options. Running AI locally on NVIDIA RTX GPUs offers high performance, low latency, and constant availability, even without internet connectivity. On the other hand, cloud-based AI can handle larger models and scale across multiple GPUs, serving many clients simultaneously. Often, a single application will leverage both approaches.
Hybrid AI harmonizes local PC and workstation compute power with cloud scalability, providing the flexibility to optimize AI workloads based on specific use cases, cost, and performance. This setup ensures that AI tasks run efficiently, whether they are local or cloud-based, all accelerated by NVIDIA GPUs and the comprehensive NVIDIA AI stack, including TensorRT and TensorRT-LLM.
Tools and Technologies Supporting Hybrid AI
NVIDIA offers a range of tools and technologies to support hybrid AI workflows for creators, gamers, and developers. Let’s explore how these innovations are transforming various industries.
Dream in the Cloud, Create Locally on RTX
Generative AI is a game-changer for artists, enabling them to ideate, prototype, and brainstorm new creations. One such solution, Generative AI by iStock — powered by NVIDIA Edify — provides a generative photography service built for artists. It trains on licensed content and compensates contributing artists.
Generative AI by iStock offers tools for exploring styles, modifying parts of an image, and expanding the canvas, allowing artists to quickly bring their ideas to life. Once the creative concept is ready, artists can switch to their local RTX-powered PCs and workstations. These systems provide AI acceleration in over 125 top creative apps, allowing artists to realize their full vision, whether they are using Photoshop, DaVinci Resolve, or Blender.
Bringing NPCs to Life with Hybrid ACE
Hybrid AI is also revolutionizing interactive PC gaming. NVIDIA ACE enables game developers to integrate state-of-the-art generative AI models into digital avatars on RTX AI PCs. Powered by AI neural networks, NVIDIA ACE allows developers to create NPCs that understand and respond to human player text and speech in real-time, enhancing the gaming experience.
Hybrid Developer Tools for Versatile AI Model Building
Hybrid AI also facilitates the development and fine-tuning of new AI models. NVIDIA AI Workbench allows developers to quickly create, test, and customize pretrained generative AI models and LLMs on RTX GPUs. With streamlined access to popular repositories like Hugging Face, GitHub, and NVIDIA NGC, AI Workbench simplifies the development process, enabling data scientists and developers to collaborate and migrate projects seamlessly.
When additional performance is needed, projects can scale to data centers, public clouds, or NVIDIA DGX Cloud. They can then be brought back to local RTX systems for inference and light customization. Pre-built Workbench projects support tasks such as document chat using retrieval-augmented generation (RAG) and customizing LLMs using fine-tuning.
The Hybrid RAG Workbench Project
The Hybrid RAG Workbench project provides a customizable application that developers can run locally or in the cloud. It allows developers to embed documents locally and run inference either on a local RTX system or a cloud endpoint hosted on NVIDIA’s API catalog. This flexibility supports various models, endpoints, and containers, ensuring developers can optimize performance based on their GPU of choice.
Conclusion
NVIDIA RTX AI PCs and workstations, combined with cloud-based solutions, offer a powerful platform for creators, gamers, and developers. By leveraging hybrid AI workflows, users can take advantage of the best of both worlds, achieving high performance, scalability, and flexibility in their AI-driven projects.
Generative AI is transforming gaming, videoconferencing, and interactive experiences of all kinds. Stay informed about the latest developments and innovations by subscribing to the AI Decoded newsletter. And if you found this article helpful, consider supporting us! Your support can make a significant difference in our progress and innovation!
Muhammad Hussnain Facebook | Instagram | Twitter | Linkedin | Youtube
1 note
·
View note
Text
Partnership with NVIDIA for Generative AI
SAP has recently partnered with NVIDIA to enhance the adoption of generative AI across its enterprise applications, a move that signifies a significant advancement in AI capabilities for business solutions.
Key Highlights of the Partnership
SAP Business AI:
The collaboration aims to build and deliver SAP Business AI, which includes scalable, business-specific generative AI capabilities integrated into the Joule copilot and across SAP's cloud solutions. The generative AI hub will underpin these capabilities, providing access to a broad range of large language models.
Generative AI in SAP Solutions:
The partnership will integrate generative AI into SAP's latest solutions such as SAP Datasphere, SAP Business Technology Platform (BTP), and RISE with SAP. This integration is expected to enhance AI capabilities within these platforms, enabling better data insights, process automation, and intelligent decision-making.
Technological Integration:
SAP will leverage NVIDIA's generative AI foundry services to fine-tune LLMs for domain-specific applications. Additionally, new NVIDIA NIM microservices will be deployed to maximize inference performance, and NVIDIA Nem Retriever microservices will enhance data accuracy and insights using retrieval-augmented generation (RAG) capabilities.
Use Cases and Applications:
The partnership explores over 20 use cases where generative AI can simplify and enhance digital transformation. These include intelligent invoice matching in SAP S/4HANA Cloud, improved HR functions in SAP SuccessFactors, and better business process recommendations in SAP Signaigo.
Strategic Goals:
The strategic goal of this partnership is to help enterprises leverage AI to unlock the potential of their data, streamline operations, and drive innovation. This aligns with SAP's broader strategy to invest in technologies that deliver tangible business value and empower enterprises to stay competitive.
Overall, the SAP-NVIDIA partnership marks a significant step in making advanced AI capabilities accessible and practical for businesses, facilitating the integration of cutting-edge AI technologies into everyday business processes. This collaboration aims to bring about substantial efficiency and innovation in the enterprise sector.
0 notes