#Reinforcement learning in semiconductor processes
Explore tagged Tumblr posts
Text
Machine learning applications in semiconductor manufacturing
Machine Learning Applications in Semiconductor Manufacturing: Revolutionizing the Industry
The semiconductor industry is the backbone of modern technology, powering everything from smartphones and computers to autonomous vehicles and IoT devices. As the demand for faster, smaller, and more efficient chips grows, semiconductor manufacturers face increasing challenges in maintaining precision, reducing costs, and improving yields. Enter machine learning (ML)—a transformative technology that is revolutionizing semiconductor manufacturing. By leveraging ML, manufacturers can optimize processes, enhance quality control, and accelerate innovation. In this blog post, we’ll explore the key applications of machine learning in semiconductor manufacturing and how it is shaping the future of the industry.
Predictive Maintenance
Semiconductor manufacturing involves highly complex and expensive equipment, such as lithography machines and etchers. Unplanned downtime due to equipment failure can cost millions of dollars and disrupt production schedules. Machine learning enables predictive maintenance by analyzing sensor data from equipment to predict potential failures before they occur.
How It Works: ML algorithms process real-time data from sensors, such as temperature, vibration, and pressure, to identify patterns indicative of wear and tear. By predicting when a component is likely to fail, manufacturers can schedule maintenance proactively, minimizing downtime.
Impact: Predictive maintenance reduces equipment downtime, extends the lifespan of machinery, and lowers maintenance costs.
Defect Detection and Quality Control
Defects in semiconductor wafers can lead to significant yield losses. Traditional defect detection methods rely on manual inspection or rule-based systems, which are time-consuming and prone to errors. Machine learning, particularly computer vision, is transforming defect detection by automating and enhancing the process.
How It Works: ML models are trained on vast datasets of wafer images to identify defects such as scratches, particles, and pattern irregularities. Deep learning algorithms, such as convolutional neural networks (CNNs), excel at detecting even the smallest defects with high accuracy.
Impact: Automated defect detection improves yield rates, reduces waste, and ensures consistent product quality.
Process Optimization
Semiconductor manufacturing involves hundreds of intricate steps, each requiring precise control of parameters such as temperature, pressure, and chemical concentrations. Machine learning optimizes these processes by identifying the optimal settings for maximum efficiency and yield.
How It Works: ML algorithms analyze historical process data to identify correlations between input parameters and output quality. Techniques like reinforcement learning can dynamically adjust process parameters in real-time to achieve the desired outcomes.
Impact: Process optimization reduces material waste, improves yield, and enhances overall production efficiency.
Yield Prediction and Improvement
Yield—the percentage of functional chips produced from a wafer—is a critical metric in semiconductor manufacturing. Low yields can result from various factors, including process variations, equipment malfunctions, and environmental conditions. Machine learning helps predict and improve yields by analyzing complex datasets.
How It Works: ML models analyze data from multiple sources, including process parameters, equipment performance, and environmental conditions, to predict yield outcomes. By identifying the root causes of yield loss, manufacturers can implement targeted improvements.
Impact: Yield prediction enables proactive interventions, leading to higher productivity and profitability.
Supply Chain Optimization
The semiconductor supply chain is highly complex, involving multiple suppliers, manufacturers, and distributors. Delays or disruptions in the supply chain can have a cascading effect on production schedules. Machine learning optimizes supply chain operations by forecasting demand, managing inventory, and identifying potential bottlenecks.
How It Works: ML algorithms analyze historical sales data, market trends, and external factors (e.g., geopolitical events) to predict demand and optimize inventory levels. Predictive analytics also helps identify risks and mitigate disruptions.
Impact: Supply chain optimization reduces costs, minimizes delays, and ensures timely delivery of materials.
Advanced Process Control (APC)
Advanced Process Control (APC) is critical for maintaining consistency and precision in semiconductor manufacturing. Machine learning enhances APC by enabling real-time monitoring and control of manufacturing processes.
How It Works: ML models analyze real-time data from sensors and equipment to detect deviations from desired process parameters. They can automatically adjust settings to maintain optimal conditions, ensuring consistent product quality.
Impact: APC improves process stability, reduces variability, and enhances overall product quality.
Design Optimization
The design of semiconductor devices is becoming increasingly complex as manufacturers strive to pack more functionality into smaller chips. Machine learning accelerates the design process by optimizing chip layouts and predicting performance outcomes.
How It Works: ML algorithms analyze design data to identify patterns and optimize layouts for performance, power efficiency, and manufacturability. Generative design techniques can even create novel chip architectures that meet specific requirements.
Impact: Design optimization reduces time-to-market, lowers development costs, and enables the creation of more advanced chips.
Fault Diagnosis and Root Cause Analysis
When defects or failures occur, identifying the root cause can be challenging due to the complexity of semiconductor manufacturing processes. Machine learning simplifies fault diagnosis by analyzing vast amounts of data to pinpoint the source of problems.
How It Works: ML models analyze data from multiple stages of the manufacturing process to identify correlations between process parameters and defects. Techniques like decision trees and clustering help isolate the root cause of issues.
Impact: Faster fault diagnosis reduces downtime, improves yield, and enhances process reliability.
Energy Efficiency and Sustainability
Semiconductor manufacturing is energy-intensive, with significant environmental impacts. Machine learning helps reduce energy consumption and improve sustainability by optimizing resource usage.
How It Works: ML algorithms analyze energy consumption data to identify inefficiencies and recommend energy-saving measures. For example, they can optimize the operation of HVAC systems and reduce idle time for equipment.
Impact: Energy optimization lowers operational costs and reduces the environmental footprint of semiconductor manufacturing.
Accelerating Research and Development
The semiconductor industry is driven by continuous innovation, with new materials, processes, and technologies being developed regularly. Machine learning accelerates R&D by analyzing experimental data and predicting outcomes.
How It Works: ML models analyze data from experiments to identify promising materials, processes, or designs. They can also simulate the performance of new technologies, reducing the need for physical prototypes.
Impact: Faster R&D cycles enable manufacturers to bring cutting-edge technologies to market more quickly.
Challenges and Future Directions
While machine learning offers immense potential for semiconductor manufacturing, there are challenges to overcome. These include the need for high-quality data, the complexity of integrating ML into existing workflows, and the shortage of skilled professionals. However, as ML technologies continue to evolve, these challenges are being addressed through advancements in data collection, model interpretability, and workforce training.
Looking ahead, the integration of machine learning with other emerging technologies, such as the Internet of Things (IoT) and digital twins, will further enhance its impact on semiconductor manufacturing. By embracing ML, manufacturers can stay competitive in an increasingly demanding and fast-paced industry.
Conclusion
Machine learning is transforming semiconductor manufacturing by enabling predictive maintenance, defect detection, process optimization, and more. As the industry continues to evolve, ML will play an increasingly critical role in driving innovation, improving efficiency, and ensuring sustainability. By harnessing the power of machine learning, semiconductor manufacturers can overcome challenges, reduce costs, and deliver cutting-edge technologies that power the future.
This blog post provides a comprehensive overview of machine learning applications in semiconductor manufacturing. Let me know if you’d like to expand on any specific section or add more details!
#semiconductor manufacturing#Machine learning in semiconductor manufacturing#AI in semiconductor industry#Predictive maintenance in chip manufacturing#Defect detection in semiconductor wafers#Semiconductor process optimization#Yield prediction in semiconductor manufacturing#Advanced Process Control (APC) in semiconductors#Semiconductor supply chain optimization#Fault diagnosis in chip manufacturing#Energy efficiency in semiconductor production#Deep learning for semiconductor defects#Computer vision in wafer inspection#Reinforcement learning in semiconductor processes#Semiconductor yield improvement using AI#Smart manufacturing in semiconductors#AI-driven semiconductor design#Root cause analysis in chip manufacturing#Sustainable semiconductor manufacturing#IoT in semiconductor production#Digital twins in semiconductor manufacturing
0 notes
Text
Are NASDAQ Technology Stocks Aligned with the Next Phase of Digital Innovation?
The technology sector continues to be a cornerstone of market activity, drawing consistent attention amid ongoing advancements in artificial intelligence, cloud computing, and cybersecurity. As digital transformation accelerates, the performance and positioning of publicly traded technology companies on the NASDAQ exchange offer insights into broader sectoral trends. This blog explores the current market behavior of NASDAQ Technology Stocks, with emphasis on structural growth factors, institutional movements, and the implications of innovation-led business models.
Ongoing Momentum in NASDAQ Technology Stocks
NASDAQ Technology Stocks reflect a dynamic intersection of hardware, software, and digital service providers. Companies in this segment span sub-sectors such as semiconductor production, data analytics, cloud infrastructure, and artificial intelligence platforms. As the global economy undergoes digital shifts, technology firms have seen expanded roles across both commercial and consumer landscapes.
Over recent quarters, several of these companies have shown resilience despite economic headwinds. While not uniformly performing, many large-cap and mid-cap firms within NASDAQ Technology Stocks continue to allocate capital toward research and development, strategic acquisitions, and enhanced computing capabilities. These structural strategies are reinforcing their positions within the global technology ecosystem.
Tech Sector Performance and Institutional Activity
Institutional interest remains a significant factor influencing NASDAQ Technology Stocks. Major asset management firms, pension funds, and market entities have consistently reshaped their portfolios to reflect shifts in innovation-led enterprises. Data points suggest a concentration in high-liquidity stocks within the technology space, including those linked to machine learning, quantum computing, and enterprise software solutions.
Several companies among NASDAQ Technology Stocks have seen changes in institutional ownership percentages over the past few quarters. This trend highlights ongoing rebalancing decisions amid broader market uncertainty. Additionally, fund flows into technology exchange-traded products have supported demand for securities associated with digital services, high-speed computing, and next-generation connectivity solutions.
Subsector Strength: Semiconductor Firms and AI Integration
Semiconductor firms remain a central pillar within NASDAQ Technology Stocks. The supply chain normalization process, paired with increasing demand from sectors such as electric vehicles and mobile computing, has contributed to steady operational metrics across several chipmakers. The push for customized semiconductors for AI workloads has further reinforced demand visibility in the silicon segment.
Meanwhile, artificial intelligence stocks—particularly those integrating AI into cloud, SaaS, and cybersecurity frameworks—continue to feature prominently across discussions in the broader tech sector. From generative AI engines to infrastructure enablers, these companies are reconfiguring enterprise productivity models, cybersecurity architectures, and content creation workflows.
Valuation Metrics and Financial Positioning
Across the landscape of NASDAQ Technology Stocks, key valuation metrics such as price-to-sales ratios and debt-to-equity levels have experienced fluctuations in line with monetary policy shifts. Many technology companies maintain cash-rich balance sheets, allowing for continued capital allocation into innovation, without excessive reliance on external financing.
In addition to liquidity management, operational margins and recurring revenue models remain central themes among software and platform-based businesses. Market analysts frequently assess cost structures, subscription growth, and platform adoption rates to evaluate financial health. While high-growth companies typically carry elevated valuation multiples, financial resilience is often linked to revenue diversification and scalable delivery systems—key traits observed in many NASDAQ Technology Stocks.
Regulatory Scrutiny and Compliance Trends
Tech sector oversight has expanded in scope as companies navigate evolving data privacy standards, antitrust evaluations, and ethical frameworks for AI deployment. Firms within NASDAQ Technology Stocks operating in high-visibility domains such as social media, digital advertising, and biometric security continue to update compliance strategies in response to national and international regulatory standards.
These policy shifts introduce operational challenges but also present opportunities for companies positioned to develop compliant, secure, and interoperable technologies. Consequently, regulatory awareness is playing a more pronounced role in shaping market narratives around NASDAQ Technology Stocks.
Global Expansion and Market Penetration
Many of the firms under NASDAQ Technology Stocks have pursued international expansion through infrastructure development, regional partnerships, and localized product strategies. These global movements contribute to revenue diversification and increased exposure to emerging digital economies. From cloud service providers launching data centers abroad to software platforms tailoring offerings for specific languages and geographies, globalization remains a recurring theme.
Additionally, strategic emphasis on cross-border collaboration, particularly in AI research and clean-tech computing, continues to drive cross-sector partnerships. These efforts are not only enhancing technological capacity but also enabling companies in the technology sector to secure long-term enterprise contracts across continents.
NASDAQ Technology Stocks represent a complex and evolving segment of the financial market, shaped by digital innovation, institutional shifts, and sub-sector-specific developments. As the broader tech sector continues to respond to macroeconomic adjustments and regulatory frameworks, publicly traded companies in this space are redefining operational models and expanding into new verticals. From semiconductor firms to AI-enabled platforms, the sector’s performance reflects ongoing transformation across digital infrastructure and advanced computing landscapes.
0 notes
Text
Why Investing in ATE Technology is Crucial for Future Electronics

The global Automated Test Equipment (ATE) market is poised for substantial growth, driven by rising demand across various industries such as consumer electronics, automotive, aerospace, and defense. With a valuation of US$ 5.91 billion in 2020, the market is expected to expand at a CAGR of 3.9% from 2021 to 2031, reaching an estimated value of US$ 9.02 billion by 2031.
Key Drivers of the ATE Market
1. Increasing Adoption in Consumer Electronics and Semiconductors
The surge in demand for advanced semiconductor devices and electronic components is fueling the adoption of ATE. As manufacturers strive for higher efficiency and accuracy, ATE is playing a critical role in streamlining testing processes, reducing errors, and ensuring quality control. The rapid transition to a 28 nm node application processor manufacturing process has further driven the need for robust IC testing equipment.
2. Expansion in Automotive and Aerospace Sectors
Automated Test Equipment is widely used in the automotive and aerospace industries to test critical electronic systems, including ADAS (Advanced Driver Assistance Systems), infotainment systems, braking units, batteries, and navigation control systems. As electric and autonomous vehicles gain traction, the demand for ATE to ensure safety, reliability, and performance is escalating.
3. Advancements in Next-Generation Technologies
The proliferation of 5G networks, IoT ecosystems, and AI-driven applications has heightened the complexity of electronic devices, requiring more sophisticated testing solutions. ATE is instrumental in ensuring optimal performance for next-gen devices, making it indispensable for manufacturers.
Regional Insights: Asia Pacific Leads the Market
Asia Pacific is projected to dominate the ATE market, owing to the presence of key manufacturers and rapid technological advancements in China, Japan, South Korea, and Taiwan. The region’s booming consumer electronics industry, increasing industrial automation, and government initiatives for digital transformation are key growth factors. The expansion of the semiconductor industry in China further reinforces Asia Pacific’s leadership in the global ATE market.
Challenges and Opportunities
Challenges:
High Initial Investment Costs: ATE systems are expensive, posing a challenge for small and medium-sized enterprises (SMEs) looking to implement automated testing.
Complexity in High-Speed Testing: As electronic devices become more intricate, ensuring high-speed and high-precision testing remains a challenge for manufacturers.
Opportunities:
Miniaturization and Cost Reduction: Technological advancements are leading to the development of compact and cost-effective ATE solutions, making them more accessible.
Growth in Electric Vehicles (EVs): The ongoing penetration of EVs and autonomous vehicles is creating new opportunities for ATE manufacturers to develop specialized testing solutions.
Industry 4.0 and Smart Manufacturing: The integration of ATE with AI, machine learning, and data analytics is set to enhance automation and efficiency in manufacturing environments.
Strategic Initiatives by Key Players
Leading companies in the ATE market are focusing on mergers, acquisitions, and product innovations to strengthen their market presence. Key players include:
National Instruments Corporation
Advantest Corporation
Teradyne, Inc.
Chroma ATE Inc.
Roos Instruments
Xcerra Corporation
SPEA S.p.A.
These companies are investing heavily in R&D activities to develop cost-effective, high-performance ATE solutions, ensuring quality and efficiency in electronic manufacturing processes.
Conclusion
The global Automated Test Equipment (ATE) market is on a steady growth trajectory, driven by increasing demand from consumer electronics, automotive, and aerospace industries. With advancements in semiconductor technology, the expansion of 5G networks, and the rise of electric vehicles, ATE will remain a crucial element in ensuring quality and efficiency in manufacturing. As companies continue to innovate and expand their product portfolios, the ATE market is expected to witness sustained growth, making it a key area for investment and development in the coming years.Contact Us: Transparency Market Research Inc. CORPORATE HEADQUARTER DOWNTOWN, 1000 N. West Street, Suite 1200, Wilmington, Delaware 19801 USA Tel: +1-518-618-1030 USA - Canada Toll Free: 866-552-3453 Website: https://www.transparencymarketresearch.com Email: [email protected]
0 notes
Text
IEEE Transactions on Artificial Intelligence, Volume 5, Issue 12, December 2024
1) Editorial: Future Directions in Artificial Intelligence Research
Author(s): Hussein Abbass
Pages: 5858 - 5862
2) Ensuring Ethical Standards in the Development of Autonomous and Intelligent Systems
Author(s): Anetta Jedlickova
Pages: 5863 - 5872
3) Recent Advances in Generative AI and Large Language Models: Current Status, Challenges, and Perspectives
Author(s): Desta Haileselassie Hagos, Rick Battle, Danda B. Rawat
Pages: 5873 - 5893
4) A Review on Transferability Estimation in Deep Transfer Learning
Author(s): Yihao Xue, Rui Yang, Xiaohan Chen, Weibo Liu, Zidong Wang, Xiaohui Liu
Pages: 5894 - 5914
5) A Comprehensive Exploration of Real-Time 3-D View Reconstruction Methods
Author(s): Arya Agrawal, Teena Sharma, Nishchal K. Verma
Pages: 5915 - 5927
6) A Survey on Symbolic Knowledge Distillation of Large Language Models
Author(s): Kamal Acharya, Alvaro Velasquez, Houbing Herbert Song
Pages: 5928 - 5948
7) Games for Artificial Intelligence Research: A Review and Perspectives
Author(s): Chengpeng Hu, Yunlong Zhao, Ziqi Wang, Haocheng Du, Jialin Liu
Pages: 5949 - 5968
8) Exploring Machine Learning for Semiconductor Process Optimization: A Systematic Review
Author(s): Ying-Lin Chen, Sara Sacchi, Bappaditya Dey, Victor Blanco, Sandip Halder, Philippe Leray, Stefan De Gendt
Pages: 5969 - 5989
9) Efficient Evaluation Methods for Neural Architecture Search: A Survey
Author(s): Xiaotian Song, Xiangning Xie, Zeqiong Lv, Gary G. Yen, Weiping Ding, Jiancheng Lv, Yanan Sun
Pages: 5990 - 6011
10) Neuro-Symbolic AI for Military Applications
Author(s): Desta Haileselassie Hagos, Danda B. Rawat
Pages: 6012 - 6026
11) Label-Efficient Time Series Representation Learning: A Review
Author(s): Emadeldeen Eldele, Mohamed Ragab, Zhenghua Chen, Min Wu, Chee-Keong Kwoh, Xiaoli Li
Pages: 6027 - 6042
12) Direct Adversarial Latent Estimation to Evaluate Decision Boundary Complexity in Black Box Models
Author(s): Ashley S. Dale, Lauren Christopher
Pages: 6043 - 6053
13) Steganography in Style Transfer
Author(s): Ruolan Shi, Zichi Wang, Yunlong Hao, Xinpeng Zhang
Pages: 6054 - 6065
14) Model-Based Offline Reinforcement Learning With Uncertainty Estimation and Policy Constraint
Author(s): Jin Zhu, Chunhui Du, Geir E. Dullerud
Pages: 6066 - 6079
15) Game Theory Meets Data Augmentation
Author(s): Yuhan Kang, Samira Zare, Alex Tong Lin, Zhu Han, Stanley Osher, Hien Van Nguyen
Pages: 6080 - 6094
16) Epi-Curriculum: Episodic Curriculum Learning for Low-Resource Domain Adaptation in Neural Machine Translation
Author(s): Keyu Chen, Di Zhuang, Mingchen Li, J. Morris Chang
Pages: 6095 - 6108
17) Enhancing Aerial Object Detection With Selective Frequency Interaction Network
Author(s): Weijie Weng, Mengwan Wei, Junchi Ren, Fei Shen
Pages: 6109 - 6120
18) Unsupervised Domain Adaptation on Point Clouds via High-Order Geometric Structure Modeling
Author(s): Jiang-Xing Cheng, Huibin Lin, Chun-Yang Zhang, C. L. Philip Chen
Pages: 6121 - 6133
19) Deep Learning-Based Dual Watermarking for Image Copyright Protection and Authentication
Author(s): Sudev Kumar Padhi, Archana Tiwari, Sk. Subidh Ali
Pages: 6134 - 6145
20) Curious Feature Selection-Based Clustering
Author(s): Michal Moran, Goren Gordon
Pages: 6146 - 6158
21) Spatiotemporal Object Detection for Improved Aerial Vehicle Detection in Traffic Monitoring
Author(s): Kristina Telegraph, Christos Kyrkou
Pages: 6159 - 6171
22) DeepHGCN: Toward Deeper Hyperbolic Graph Convolutional Networks
Author(s): Jiaxu Liu, Xinping Yi, Xiaowei Huang
Pages: 6172 - 6185
23) Q-Cogni: An Integrated Causal Reinforcement Learning Framework
Author(s): Cristiano da Costa Cunha, Wei Liu, Tim French, Ajmal Mian
Pages: 6186 - 6195
24) From Behavior to Natural Language: Generative Approach for Unmanned Aerial Vehicle Intent Recognition
Author(s): Leyan Li, Rennong Yang, Maolong Lv, Ao Wu, Zilong Zhao
Pages: 6196 - 6209
25) Learning Multipursuit Evasion for Safe Targeted Navigation of Drones
Author(s): Jiaping Xiao, Mir Feroskhan
Pages: 6210 - 6224
26) OAFuser: Toward Omni-Aperture Fusion for Light Field Semantic Segmentation
Author(s): Fei Teng, Jiaming Zhang, Kunyu Peng, Yaonan Wang, Rainer Stiefelhagen, Kailun Yang
Pages: 6225 - 6239
27) An Approach for Privacy-Aware Mobile App Package Recommendation
Author(s): Shanpeng Liu, Buqing Cao, Jianxun Liu, Guosheng Kang, Min Shi, Xiong Li, Kenneth K. Fletcher
Pages: 6240 - 6252
28) Multiscale and Multilayer Contrastive Learning for Domain Generalization
Author(s): Aristotelis Ballas, Christos Diou
Pages: 6253 - 6266
29) Knowledge-Guided Evolutionary Optimization for Large-Scale Air Defense Resource Allocation
Author(s): Wenhua Li, Rui Wang, Yong Heng, Tao Zhang, Ling Wang
Pages: 6267 - 6279
30) A Brain-Inspired Theory of Collective Mind Model for Efficient Social Cooperation
Author(s): Zhuoya Zhao, Feifei Zhao, Shiwen Wang, Yinqian Sun, Yi Zeng
Pages: 6280 - 6289
31) A Game-Theoretic Approach to Containing Artificial General Intelligence: Insights From Highly Autonomous Aggressive Malware
Author(s): Timothy R. McIntosh, Teo Susnjak, Tong Liu, Paul Watters, Alex Ng, Malka N. Halgamuge
Pages: 6290 - 6303
32) A Composite Decomposition Method for Large-Scale Global Optimization
Author(s): Maojiang Tian, Minyang Chen, Wei Du, Yang Tang, Yaochu Jin, Gary G. Yen
Pages: 6304 - 6319
33) Optimal Output Feedback Tracking Control for Takagi–Sugeno Fuzzy Systems
Author(s): Wenting Song, Shaocheng Tong
Pages: 6320 - 6329
34) Quantum Face Recognition With Multigate Quantum Convolutional Neural Network
Author(s): Yijie Zhu, Ahmed Bouridane, M Emre Celebi, Debanjan Konar, Plamen Angelov, Qiang Ni, Richard Jiang
Pages: 6330 - 6341
35) Learning Empirical Inherited Intelligent MPC for Switched Systems With Network Security Communication
Author(s): Yiwen Qi, Yiwen Tang, Wenke Yu
Pages: 6342 - 6355
36) MSCS: Multiscale Consistency Supervision With CNN-Transformer Collaboration for Semisupervised Histopathology Image Semantic Segmentation
Author(s): Min-En Hsieh, Chien-Yu Chiou, Hung-Wen Tsai, Yu-Cheng Chang, Pau-Choo Chung
Pages: 6356 - 6368
37) Differentially Private and Heterogeneity-Robust Federated Learning With Theoretical Guarantee
Author(s): Xiuhua Wang, Shuai Wang, Yiwei Li, Fengrui Fan, Shikang Li, Xiaodong Lin
Pages: 6369 - 6384
38) Multimodal Fusion Induced Attention Network for Industrial VOCs Detection
Author(s): Yu Kang, Kehao Shi, Jifang Tan, Yang Cao, Lijun Zhao, Zhenyi Xu
Pages: 6385 - 6398
39) Simultaneous Learning and Planning Within Sensing Range: An Approach for Local Path Planning
Author(s): Lokesh Kumar, Arup Kumar Sadhu, Ranjan Dasgupta
Pages: 6399 - 6411
40) Generative Representation Learning in Recurrent Neural Networks for Causal Timeseries Forecasting
Author(s): Georgios Chatziparaskevas, Ioannis Mademlis, Ioannis Pitas
Pages: 6412 - 6425
41) Federated c-Means and Fuzzy c-Means Clustering Algorithms for Horizontally and Vertically Partitioned Data
Author(s): José Luis Corcuera Bárcena, Francesco Marcelloni, Alessandro Renda, Alessio Bechini, Pietro Ducange
Pages: 6426 - 6441
42) A Comprehensive Radiogenomic Feature Characterization of 19/20 Co-gain in Glioblastoma
Author(s): Padmaja Jonnalagedda, Brent Weinberg, Taejin L. Min, Shiv Bhanu, Bir Bhanu
Pages: 6442 - 6456
43) LSTM-Based Model Compression for CAN Security in Intelligent Vehicles
Author(s): Yuan Feng, Yingxu Lai, Ye Chen, Zhaoyi Zhang, Jingwen Wei
Pages: 6457 - 6471
44) Deep Imbalanced Learning for Multimodal Emotion Recognition in Conversations
Author(s): Tao Meng, Yuntao Shou, Wei Ai, Nan Yin, Keqin Li
Pages: 6472 - 6487
45) FIMKD: Feature-Implicit Mapping Knowledge Distillation for RGB-D Indoor Scene Semantic Segmentation
Author(s): Wujie Zhou, Yuxiang Xiao, Yuanyuan Liu, Qiuping Jiang
Pages: 6488 - 6499
46) Two-Stage Representation Refinement Based on Convex Combination for 3-D Human Poses Estimation
Author(s): Luefeng Chen, Wei Cao, Biao Zheng, Min Wu, Witold Pedrycz, Kaoru Hirota
Pages: 6500 - 6508
47) Cooperative Advantage Actor–Critic Reinforcement Learning for Multiagent Pursuit-Evasion Games on Communication Graphs
Author(s): Yizhen Meng, Chun Liu, Qiang Wang, Longyu Tan
Pages: 6509 - 6523
48) RADiff: Controllable Diffusion Models for Radio Astronomical Maps Generation
Author(s): Renato Sortino, Thomas Cecconello, Andrea De Marco, Giuseppe Fiameni, Andrea Pilzer, Daniel Magro, Andrew M. Hopkins, Simone Riggi, Eva Sciacca, Adriano Ingallinera, Cristobal Bordiu, Filomena Bufano, Concetto Spampinato
Pages: 6524 - 6535
49) Higher-Order Directed Community Detection by A Multiobjective Evolutionary Framework
Author(s): Jing Xiao, Jing Cao, Xiao-Ke Xu
Pages: 6536 - 6550
50) Cost-Efficient Feature Selection for Horizontal Federated Learning
Author(s): Sourasekhar Banerjee, Devvjiit Bhuyan, Erik Elmroth, Monowar Bhuyan
Pages: 6551 - 6565
51) Self-Model-Free Learning Versus Learning With External Rewards in Information Constrained Environments
Author(s): Prachi Pratyusha Sahoo, Kyriakos G. Vamvoudakis
Pages: 6566 - 6579
52) Event-Triggered Fuzzy Adaptive Stabilization of Parabolic PDE–ODE Systems
Author(s): Yuan-Xin Li, Bo Xu, Xing-Yu Zhang
Pages: 6580 - 6590
53) Optimal Control of Stochastic Markovian Jump Systems With Wiener and Poisson Noises: Two Reinforcement Learning Approaches
Author(s): Zhiguo Yan, Tingkun Sun, Guolin Hu
Pages: 6591 - 6600
54) Spatio-temporal Graph-Based Generation and Detection of Adversarial False Data Injection Evasion Attacks in Smart Grids
Author(s): Abdulrahman Takiddin, Muhammad Ismail, Rachad Atat, Erchin Serpedin
Pages: 6601 - 6616
55) AugDiff: Diffusion-Based Feature Augmentation for Multiple Instance Learning in Whole Slide Image
Author(s): Zhuchen Shao, Liuxi Dai, Yifeng Wang, Haoqian Wang, Yongbing Zhang
Pages: 6617 - 6628
56) Simplified Kernel-Based Cost-Sensitive Broad Learning System for Imbalanced Fault Diagnosis
Author(s): Kaixiang Yang, Wuxing Chen, Yifan Shi, Zhiwen Yu, C. L. Philip Chen
Pages: 6629 - 6644
57) Partial Domain Adaptation for Building Borehole Lithology Model Under Weaker Geological Prior
Author(s): Jing Li, Jichen Wang, Zerui Li, Yu Kang, Wenjun Lv
Pages: 6645 - 6658
58) Evolution of Web API Cooperation Network via Exploring Community Structure and Popularity
Author(s): Guosheng Kang, Yang Wang, Jianxun Liu, Buqing Cao, Yong Xiao, Yu Xu
Pages: 6659 - 6671
59) RATs-NAS: Redirection of Adjacent Trails on Graph Convolutional Networks for Predictor-Based Neural Architecture Search
Author(s): Yu-Ming Zhang, Jun-Wei Hsieh, Chun-Chieh Lee, Kuo-Chin Fan
Pages: 6672 - 6682
60) Get Rid of Your Trail: Remotely Erasing Backdoors in Federated Learning
Author(s): Manaar Alam, Hithem Lamri, Michail Maniatakos
Pages: 6683 - 6698
61) Reinforcement Learning for Solving Colored Traveling Salesman Problems: An Entropy-Insensitive Attention Approach
Author(s): Tianyu Zhu, Xinli Shi, Xiangping Xu, Jinde Cao
Pages: 6699 - 6708
62) Constrained Multiobjective Optimization via Relaxations on Both Constraints and Objectives
Author(s): Fei Ming, Bing Xue, Mengjie Zhang, Wenyin Gong, Huixiang Zhen
Pages: 6709 - 6722
63) Towards Better Accuracy-Efficiency Trade-Offs: Dynamic Activity Inference via Collaborative Learning From Various Width-Resolution Configurations
Author(s): Lutong Qin, Lei Zhang, Chengrun Li, Chaoda Song, Dongzhou Cheng, Shuoyuan Wang, Hao Wu, Aiguo Song
Pages: 6723 - 6738
64) MTECC: A Multitask Learning Framework for Esophageal Cancer Analysis
Author(s): Jianpeng An, Wenqi Li, Yunhao Bai, Huazhen Chen, Gang Zhao, Qing Cai, Zhongke Gao
Pages: 6739 - 6751
65) Compact Multitasking Multichromosome Genetic Algorithm for Heuristic Selection in Ontology Matching
Author(s): Xingsi Xue, Jerry Chun-Wei Lin, Tong Su
Pages: 6752 - 6766
0 notes
Text
Your Ideal Roadmap for CUET PG Physics Syllabus and Exam Preparation
The CUET PG (Common University Entrance Test for Postgraduate Programs) is a crucial gateway for students aspiring to pursue a Master's degree in Physics across various universities in India. Understanding the CUET PG Physics syllabus is essential for effective preparation and successful performance in the examination. This blog provides a detailed overview of the syllabus, helping candidates strategize their study plan.
Core Topics in the CUET PG Physics Syllabus
Classical Mechanics: This section covers Newtonian mechanics, kinematics, laws of motion, work-energy principle, and conservation laws. Students should focus on the motion of particles, rigid body dynamics, and systems of particles. Concepts like Lagrangian and Hamiltonian mechanics may also be included.
Electromagnetism: A significant portion of the syllabus revolves around electric fields, magnetic fields, electromagnetic induction, and Maxwell's equations. Candidates must grasp concepts such as Gauss's law, Ampere’s law, and the behavior of circuits and fields in various media.
Thermodynamics and Statistical Mechanics: This topic includes laws of thermodynamics, heat engines, entropy, and statistical distributions. Understanding thermodynamic processes, and the kinetic theory of gases, and applying statistical mechanics to derive thermodynamic properties is essential.
Quantum Mechanics: An integral part of the syllabus, quantum mechanics covers wave-particle duality, the Schrödinger equation, quantum states, and operators. Students should be comfortable with concepts like superposition, entanglement, and quantum statistics, which are vital for modern physics.
Optics: This section involves geometric and physical optics, interference, diffraction, and polarization. Mastery of lens and mirror equations, optical instruments, and wave optics principles is crucial for success.
Solid State Physics: Candidates must familiarize themselves with crystal structures, unit cells, Bravais lattices, and the electronic properties of materials. Understanding the basics of semiconductors and superconductors can provide a competitive edge.
Nuclear and Particle Physics: Topics such as nuclear reactions, decay processes, and particle interactions fall under this category. Students should also explore the fundamental forces and the Standard Model of particle physics.
Astrophysics and Cosmology: A relatively newer addition, this section includes topics like the structure of stars, cosmological principles, and the evolution of the universe. Candidates should study the laws governing celestial bodies and the fundamental concepts of cosmology.
Preparation Strategies
Understand the Weightage: Analyze the previous years’ papers to understand the weightage of various topics. This will help prioritize study areas and allocate time effectively.
Conceptual Clarity: Focus on understanding fundamental concepts rather than rote memorization. Utilize textbooks, online resources, and video lectures to reinforce your understanding.
Practice Regularly: Regular practice through problem-solving and mock tests is crucial. Utilize previous year question papers and sample papers to familiarize yourself with the exam format.
Join Online Coaching: Consider enrolling in online coaching programs specializing in CUET PG Physics. These programs can provide structured learning and expert guidance.
Stay Updated: Keep an eye on official notifications and updates regarding the CUET PG Physics syllabus and examination patterns. Changes can occur, and staying informed is key to effective preparation.
Conclusion
The CUET PG Physics course covers a wide range of topics that require extensive preparation. Candidates can increase their chances of passing the exam by learning the core subjects and using appropriate preparation tactics. As you prepare, keep in mind that constant effort, combined with a thorough knowledge of subjects, can help you achieve your academic goals. Good luck!
1 note
·
View note
Text
Ginger Market Developments, Trends & Opportunities till 2032
Ginger Market provides in-depth analysis of the market state of Ginger manufacturers, including best facts and figures, overview, definition, SWOT analysis, expert opinions, and the most current global developments. The research also calculates market size, price, revenue, cost structure, gross margin, sales, and market share, as well as forecasts and growth rates. The report assists in determining the revenue earned by the selling of this report and technology across different application areas.
Geographically, this report is segmented into several key regions, with sales, revenue, market share and growth Rate of Ginger in these regions till the forecast period
North America
Middle East and Africa
Asia-Pacific
South America
Europe
Key Attentions of Ginger Market Report:
The report offers a comprehensive and broad perspective on the global Ginger Market.
The market statistics represented in different Ginger segments offers complete industry picture.
Market growth drivers, challenges affecting the development of Ginger are analyzed in detail.
The report will help in the analysis of major competitive market scenario, market dynamics of Ginger.
Major stakeholders, key companies Ginger, investment feasibility and new market entrants study is offered.
Development scope of Ginger in each market segment is covered in this report. The macro and micro-economic factors affecting the Ginger Market
Advancement is elaborated in this report. The upstream and downstream components of Ginger and a comprehensive value chain are explained.
Browse More Details On This Report at @https://www.globalgrowthinsights.com/market-reports/ginger-market-100565
Global Growth Insights
Web: https://www.globalgrowthinsights.com
Our Other Reports:
Artificial Meat MarketMarket Growth
Dermo-Cosmetics MarketMarket Analysis
Hand Dryers MarketMarket Size
Global Glass Fiber Reinforced Gypsum (GFRG) Panels MarketMarket Share
Global Mountain Bicycles MarketMarket Growth
Aseptic Packaging For The Pharmaceutical MarketMarket
Automotive Simulation MarketMarket Share
Semiconductor Chip Handler MarketMarket Growth Rate
Transcranial Magnetic Stimulator MarketMarket Forecast
Global Cloud Natural Language Processing MarketMarket Size
Education Technology (EdTech) MarketMarket Growth
Digital English Language Learning MarketMarket Analysis
Macromolecule Hydrogel MarketMarket Size
Global Luxury Vinyl tile (LVT) MarketMarket Share
Global Virtual Power Plant MarketMarket Growth
Digital Diagnostics MarketMarket
LED Lens MarketMarket Share
UAV Propellers MarketMarket Growth Rate
Computer Keyboards MarketMarket Forecast
Global Peanut Allergy Therapeutics MarketMarket Size
Advanced Ceramics MarketMarket Growth
Drone Defense System MarketMarket Analysis
Urea Formaldehyde Concentrate (UFC) MarketMarket Size
Global Bike Tube & Tire MarketMarket Share
Global Rhodiola Rosea P.E. MarketMarket Growth
Aerospace Inconel Blisk MarketMarket
Commercial Airport Baggage Handling System MarketMarket Share
Mica Tape for Insulation MarketMarket Growth Rate
Radio Frequency (RF) Energy Harvesting MarketMarket Forecast
Global Architectural Acoustic Panels MarketMarket Size
Arsenic Removal MarketMarket Growth
Carbon Dioxide MarketMarket Analysis
Air Quality Apps MarketMarket Size
Global Positive Temperature Coefficient (PTC) Thermistors MarketMarket Share
Global X-ray Non-Destructive Testing Equipment MarketMarket Growth
Atorvastatin Calcium MarketMarket
Fermentation Airlock MarketMarket Share
Mica Paper MarketMarket Growth Rate
Baggage Towing Tractor MarketMarket Forecast
Global Foldable IOL Market MarketMarket Size
0 notes
Text
Best MCQ Books for Engineering Exam Preparation – EA Publications
Multiple-choice questions (MCQs) are a cornerstone of engineering exams, providing a structured way to assess knowledge, comprehension, and problem-solving prowess. This guide delves into the significance and advantages of MCQs across four key engineering disciplines: Electrical, Mechanical, Civil, and Electronics Engineering.
Why MCQ Questions Help in SSC JE Exam Preparations
MCQ questions are vital for SSC JE exam preparations, offering multiple benefits. They provide a comprehensive assessment of diverse topics, ensuring you cover the entire syllabus. Practicing MCQs improves time management skills, crucial for completing exams efficiently. They also enhance problem-solving abilities by simulating real exam conditions, helping you tackle questions with confidence. EA Publications offers specialized resources, including MCQ for Civil Engineering, MCQ for Electrical Engineering, MCQ for Mechanical Engineering, and MCQ for Electronics Engineering, ensuring you have the best tools for success. These practice questions refine your understanding, making you well-prepared for the SSC JE exam.
Importance of MCQs for Electrical Engineering

Comprehensive Testing: MCQ for Electrical Engineering spans a broad spectrum of topics, from power systems to circuit analysis, ensuring a robust evaluation of your expertise.
Time Management: Regular practice with MCQ for Electrical Engineering hones your time management skills, crucial for completing exams within the given timeframe.
Practical Application: Many electrical engineering MCQs replicate real-world problems, preparing you for practical fieldwork.
Skill Evaluation: These questions gauge various skills, including theoretical application, logical reasoning, and mathematical problem-solving.
Benefits of MCQs for Mechanical Engineering in Competitive Exams

Complete Course Coverage: MCQ for Mechanical Engineering encompasses a vast range of subjects, such as thermodynamics, fluid mechanics, and materials science, ensuring a comprehensive understanding of the field.
Fundamental Reinforcement: These questions reinforce core concepts and principles, deepening your subject knowledge.
Quick Revision: MCQs are excellent for rapid revision before exams or interviews.
Problem-Solving Practice: Tackling MCQs enhances your problem-solving abilities, essential for real-world engineering challenges.
Significance of MCQs for Civil Engineering

Mock tests and MCQ for Civil Engineering are crucial for both competitive exams and university assessments, offering numerous benefits:
Diverse Discipline Coverage: MCQs provide a thorough assessment across various civil engineering disciplines, including transportation, geotechnical, and structural engineering.
Scenario-Based Learning: Many MCQs present real-world scenarios, allowing students to apply their knowledge practically.
Critical Thinking: These questions encourage critical thinking and the examination of problems from multiple perspectives.
Competitive Edge: Practicing MCQs gives you an edge in exams and job interviews by honing your skills and knowledge.
Mastering MCQs for Electronics Engineering

MCQ for Electronics Engineering demands precision and a deep understanding of circuits, semiconductors, and signal processing. These questions are crucial for:
Technical Skill Development: Engaging with MCQs sharpens technical knowledge and problem-solving techniques, vital for both academic and competitive exam success.
Conceptual Understanding: MCQs test your grasp of electronic equipment and their applications, essential for engineering studies and professional practice.
Comprehensive Preparation: Whether preparing for competitive exams or academic assessments, MCQs are a critical part of your study regime.
Leveraging EA Publications' Coaching Resources
Preparing for competitive exams can be daunting, but the right resources can simplify the process. A well-crafted study guide rich in conceptual content is indispensable. Engineers Academy Publications (EA Publications) in India stands out as a premier provider of high-quality study materials, detailed notes, and comprehensive correspondence courses tailored to engineering students.
Their expertly curated study guides cover essential concepts across various engineering disciplines, reinforcing key topics with examples and practice questions. Detailed notes serve as excellent references for quick revision, ensuring no critical areas are overlooked. EA Publications' correspondence courses offer flexibility, allowing students to learn at their own pace while providing regular assessments and mock tests to track progress and improve time management skills.
With robust support and expert guidance, Engineers Academy equips students with the necessary tools to excel in all their engineering competitive exams and achieve their academic and professional goals in life.
Conclusion
MCQs are fundamental to the learning and assessment processes in engineering education. They provide an effective means to evaluate and enhance your understanding, problem-solving skills, and exam readiness in Electrical, Mechanical, Civil, and Electronics Engineering. Integrating MCQs into your study routine strengthens your knowledge base and boosts your confidence in tackling complex engineering challenges.
0 notes
Text
The strategic partnership enhances ACL Digital’s capabilities with PhoenixAI.tech, focusing on AI drone solutions for UAVs, IoT, and edge computing to revolutionize operations significantly Beyond Visual Line of Sight (BVLOS) missions.
San Jose, CA – 15 February 2024 – ACL Digital, an ALTEN group company, a design-led Digital Experience, Product Innovation, Solutions, Engineering Services, Digital Transformation, Telecommunications, Cloud, Networking, and AI/ML solutions leader, has forged an innovative partnership with PhoenixAI.tech, a leading advanced drone technology solutions provider to revolutionize AI-powered drone solutions and services. This collaboration marks a significant milestone in propelling the future of AI-powered drones and revolutionizing industries worldwide.
The partnership will leverage PhoenixAI.tech’s and ACL Digital’s pioneering work in developing advanced AI/ML algorithms for uncrewed vehicles, IoT, and C-V2X. Together, the companies will address critical challenges in the drone industry, including autonomous navigation, adaptability, task execution, and data processing.
The strategic alliance will empower drone operators and service providers to harness AI solutions and utilize machine-learning models to enhance drone capabilities. With a primary focus on advancing intelligent UAV technology solutions, combined with ACL Digital’s broad spectrum of offerings in Telecom, Semiconductor, Digital, and Device Engineering capabilities, this partnership will redefine the conduct of missions beyond the visual line of sight.
"This collaboration brings together ACL Digital’s proven expertise in product engineering and system integration capabilities with PhoenixAI.tech’s cutting-edge AI technology,” said Ramandeep Singh, CEO of ACL Digital. “This powerful combination will help us to provide innovative drone solutions that bolster safety measures, revolutionize operations, and create new opportunities across various industries. Moreover, AI/ML solutions will actively ensure safe drone operations, even during emergencies, by detecting obstacles like cranes, predicting geographical conditions, and assessing ground risks."
PhoenixAI.tech has achieved significant breakthroughs in drone applications through the following:
Autonomous Navigation: AI algorithms enable real-time analysis of surroundings, obstacle identification, and flight path adjustments for enhanced safety and efficiency.
Adaptability: AI-powered drones continuously learn and adapt, improving reliability and effectiveness.
Complex Task Execution: Drones execute precise maneuvers, multi-drone coordination, and complex data collection and analysis.
Real-time Data Processing and Edge Computing: Drones process and analyze data on the fly, which is critical for applications like surveillance, inspection, and search & rescue.
"The ability to fly drones Beyond the Visual Line of Sight (BVLOS) represents the next big opportunity for commercial drone operators,” said Rajesh Rasalkar, PhoenixAI.tech’s co-founder. “To transform the economics of Drone BVLOS deployment, PhoenixAI.tech is building the industry’s 1st AI-assisted Drone Autonomous solution with integrated intelligent routes and several other innovations that optimize the performance, security, and safety of Drones. We are excited to partner with ACL Digital to realize this vision."
About PhoenixAI.tech
PhoenixAI.tech, based in Sunnyvale, CA, is at the forefront of technological innovation in UAVs by building AI/ML applications in a wireless environment. By leveraging the power of reinforcement learning, we are revolutionizing how UAVs navigate and interact with their wireless environments, ensuring faster and more reliable connectivity for real-time control and response, higher throughput to transmit high-definition video and sensor data, improved safety by avoiding no-fly zones or crowded areas, and enhanced battery life.
PhoenixAI.tech’s AI/ML applications also support V2X and IoT initiatives, driving innovation for optimal connectivity and operational efficiency.
Visit us at https://phoenixai.tech/ to learn more about our cutting-edge solutions.
About ACL Digital
ACL Digital, an ALTEN Group Company, is a digital product innovation and engineering leader. We help our clients design and build innovative products (AI, Cloud, and Mobile ready), content and commerce-driven platforms, and connected, converged digital experiences for the modern world through a design-led Digital Transformation framework.
Headquartered in Silicon Valley, ACL Digital is a leader in design-led digital experience, innovation, enterprise modernization, and product engineering services converging to Technology, Media & Telecom. The company has a workforce of 57,100+ spread across more than 30+ countries.
Visit https://www.acldigital.com/ for more information.
0 notes
Text
Maximizing Efficiency with Nav Sooch: Harnessing AI in Semiconductor Design
In the dynamic landscape of technology, the semiconductor industry stands as a cornerstone, driving innovation across various sectors. With the ever-increasing demand for faster, smaller, and more power-efficient chips, the role of Artificial Intelligence (AI) in semiconductor design has become indispensable. Leveraging AI methodologies can significantly enhance the efficiency and effectiveness of the design process, leading to groundbreaking advancements in chip performance and functionality. This blog delves into the intricacies of how AI is revolutionizing semiconductor design with the help of professionals like Nav Sooch and explores the myriad ways it can be harnessed to propel the industry forward.
AI-Powered Design Optimization
AI-driven algorithms play a pivotal role in optimizing semiconductor designs for performance, power, and area (PPA) metrics. By utilizing machine learning techniques, designers can explore vast design spaces efficiently, identifying optimal configurations that traditional methods might overlook. Through iterative learning, AI models adapt and refine their strategies, continuously improving design outcomes. For instance, reinforcement learning algorithms can autonomously navigate the design space, making real-time adjustments to achieve desired performance targets while minimizing power consumption and chip area.
Moreover, AI enables the acceleration of the design exploration process by predicting the performance of potential design variations based on historical data and simulation results. This predictive capability empowers designers to make informed decisions early in the design cycle, reducing time-to-market and overall development costs. By harnessing AI-driven design optimization techniques with the help of professionals like Nav Sooch, semiconductor companies can stay ahead in a fiercely competitive market, delivering cutting-edge products that meet the ever-evolving demands of consumers.
Intelligent Layout Synthesis
Layout synthesis is a critical stage in semiconductor design, where the physical layout of the chip is generated from a high-level description. AI algorithms offer intelligent solutions to streamline this process, optimizing layout designs for factors such as routing congestion, signal integrity, and manufacturing constraints. Convolutional neural networks (CNNs) trained on vast datasets of layout examples can learn to generate efficient and manufacturable layouts autonomously.
Furthermore, AI-based layout synthesis tools can adapt to specific design objectives and constraints, providing designers with tailored solutions that meet their unique requirements. These tools also facilitate collaboration between designers and manufacturing teams by incorporating manufacturing feedback into the design process, ensuring that layouts are optimized for manufacturability and yield. By integrating AI into layout synthesis with the help of professionals like Nav Sooch, semiconductor companies can achieve higher design productivity, reduce time-to-market, and mitigate risks associated with manufacturing complexities.
Enhancing Yield Prediction and Optimization
Yield prediction is a crucial aspect of semiconductor manufacturing, as it directly impacts production costs and overall profitability. AI-powered techniques offer advanced capabilities for accurately predicting yield rates based on various process parameters, design factors, and historical manufacturing data. Machine learning models, trained on extensive datasets of fabrication outcomes, can identify patterns and correlations that influence yield, enabling proactive measures to improve manufacturing yields.
Moreover, AI-driven yield optimization strategies can optimize design layouts and process parameters to maximize yield rates while maintaining desired performance specifications. By leveraging predictive analytics and optimization algorithms with the help of professionals like Nav Sooch, semiconductor companies can minimize scrap rates, reduce production costs, and enhance overall manufacturing efficiency. The integration of AI into yield prediction and optimization processes empowers semiconductor manufacturers to achieve higher yields, ensuring the delivery of high-quality chips to meet market demands.
Autonomous Chip Floorplanning
Chip floorplanning, the process of arranging functional blocks within the chip's physical layout, is a critical step in semiconductor design. AI-based algorithms can autonomously generate floorplans that optimize performance, power, and area while adhering to design constraints and routing requirements. Reinforcement learning techniques can be employed to train agents that learn to navigate the design space efficiently, exploring different floorplan configurations and adapting based on performance feedback. By leveraging AI for autonomous chip floorplanning with the help of professionals like Nav Sooch, designers can expedite the layout process, reduce manual effort, and achieve better overall chip performance.
Predictive Maintenance in Manufacturing
In semiconductor manufacturing, equipment downtime due to unexpected failures can significantly impact production schedules and costs. AI-powered predictive maintenance systems analyze sensor data from manufacturing equipment to identify patterns indicative of potential failures. Machine learning algorithms trained on historical maintenance records can predict equipment failures before they occur, enabling proactive maintenance interventions to prevent costly downtimes. By implementing AI-driven predictive maintenance solutions, semiconductor manufacturers can optimize equipment uptime, reduce maintenance costs, and enhance overall manufacturing efficiency, ensuring smooth production operations and timely delivery of high-quality semiconductor products to the market.
The integration of AI technologies in semiconductor design holds immense potential to revolutionize the industry by driving innovation, improving efficiency, and accelerating time-to-market. From design optimization to layout synthesis and yield prediction, AI-powered solutions offer a multitude of benefits, enabling semiconductor companies to stay competitive in a rapidly evolving landscape. Embracing AI methodologies empowers designers to tackle complex design challenges effectively, paving the way for the development of next-generation semiconductor products that redefine the boundaries of technology. As AI continues to evolve, its impact on semiconductor design will undoubtedly shape the future of the industry, unlocking new possibilities and driving unprecedented levels of innovation.
0 notes
Text
UK Unveils Pivotal Critical Imports Strategy

The UK Government's announcement of its new Critical Imports and Supply Chain Strategy has been met with a wave of approval from industry leaders. This groundbreaking strategy aims to reinforce the UK's supply of crucial goods, including medicines, minerals, and semiconductors, thereby enhancing national economic security and resilience.
Industry Leaders Rally Behind Government's Vision
Ross Baker of Heathrow and Katrina Ross from the UK Chamber of Shipping have voiced their support for the strategy. Their focus is on facilitating smoother import processes and enhancing maritime supply chain resilience, crucial for the UK's vast import and export activities. Institute of Export & International Trade Weighs In Marco Forgione highlights the importance of this strategy in addressing supply chain issues faced by British businesses. He emphasizes the strategy's timeliness in mitigating the impacts of global trade challenges.
Impact on Key Sectors: Pharmaceuticals, Minerals, and Technology
Richard Torbett of ABPI lauds the strategy's role in ensuring a steady supply of medicines, vital for public health and the economy. This strategy comes as a response to the lessons learned during the pandemic, highlighting the importance of collaborative efforts for supply chain stability. Securing Critical Mineral Supplies Kirsty Benham of the Critical Minerals Association (UK) and Sean Sargent of Green Lithium express their approval, stressing the importance of securing mineral supplies for the UK's net-zero and energy security goals. The strategy's focus on critical minerals is essential for the UK's transition to green technology. Boosting Advanced Manufacturing and Technology Mike Hawes of SMMT and Katherine Bennett of the High-Value Manufacturing Catapult recognize the strategy's crucial role in supporting the manufacturing sector, especially in advancing green technologies and ensuring a smooth transition to net zero.
Looking Ahead: Implementation and Industry Cooperation
The strategy is not just a government initiative; it involves extensive industry participation and cooperation. From pharmaceuticals to high-tech manufacturing, leaders are eager for the implementation of this strategy, which promises to secure the UK's economic future against global uncertainties and supply chain disruptions. Sources: THX News & Department for Business and Trade. Read the full article
#CriticalmineralsUKstrategy#GlobalsupplychainUKimpact#Net-zerostrategyUK#PharmaceuticalsupplysecurityUK#SupplyChainStrategyUK#UKadvancedmanufacturingsupport#UKCriticalImportsStrategy#UKeconomicsecurity#UKimportresiliencemeasures#UKsemiconductorssupply
0 notes
Text
Top MCQ Books to Crack Engineering Exams
It is of the utmost importance for students studying for challenging engineering competitive exams such as the SSC JE and GATE papers to familiarize themselves with the multiple-choice question format in order to achieve higher scores. Practicing multiple choice problems is emphasized heavily in top coaching institutes. Engineers Academy Publications, one of the leading publishers of books and a leading provider of competitive exam preparation services, is a vital resource for engineering professionals and students looking for thorough preparation through multiple-choice questions covering a range of subjects. Engineers Publications, with an emphasis on Electronics, Electrical, Mechanical, and Civil Engineering, provides a varied collection of MCQ for electronics engineering, MCQ for electrical engineering, MCQ for mechanical engineering, and MCQ for civil engineering. These questions are intended to hone and solidify the comprehension of fundamental ideas in each field.
Importance of MCQ questions in Engineering Education
MCQ problems serve as an indispensable tool for assessing knowledge, understanding, and problem-solving abilities in the engineering disciplines. They aid in reinforcing all the fundamental concepts, enhancing retention power of students, and preparing all the individuals for tough competitive exams and real-world engineering challenges.
MCQ for Electronics Engineering

Reference book publications the Engineers Academy Publications provide aspirants with a wide range of MCQ for Electronics Engineering encompassing a wide spectrum of topics, ranging from semiconductor devices, digital electronics, analog circuits, to communication systems. These MCQ problems are curated to challenge and reinforce understanding across the breadth of electronics engineering, aiding in comprehensive preparation.
MCQ for Electrical Engineering
For all electrical engineering enthusiasts, Engineers Academy Publications offers MCQ for electrical engineering covering power systems, electrical machines, control systems, and more. These MCQ questions delve into the intricacies of electrical engineering concepts, providing a robust platform for assessment and learning.
MCQ for Mechanical Engineering

One of the best-selling reference books and postal courses of Engineers Academy Publication is for mechanical engineering students.MCQ for Mechanical Engineering preparing for different competitive examinations span over crucial engineering subjects such as thermodynamics, fluid mechanics, machine design, and manufacturing processes. These MCQ questions are tailored to enhance problem-solving skills and conceptual clarity in mechanical engineering.
Best questions to master MCQ for Civil Engineering
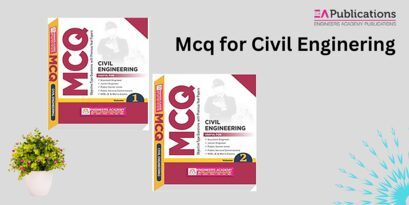
Covering structural engineering, geotechnical engineering, environmental engineering, and many more, Engineers Publications course book on MCQ for Civil Engineering provide a deep and comprehensive assessment of core civil engineering principles. They serve as a valuable and important resource for both students and professionals in the field.
Unique Features of top coaching book Publications' MCQ questions:
Topic-Wise Categorization: MCQ problems are organized by topic, enabling focused practice and targeted learning.
Comprehensive Coverage: Encompassing the breadth of each engineering discipline, ensuring a holistic understanding.
Difficulty Levels: Varied difficulty levels to challenge individuals at different stages of their learning journey.
Explanatory Answers: Detailed explanations accompanying MCQs, aiding in understanding concepts and rectifying mistakes.
Real Exam Simulation: Emulating exam-like scenarios to familiarize individuals with the examination pattern and time constraints.
Conclusion
Engineers Publications stands as an indispensable ally for engineering enthusiasts, offering a rich repository of MCQs that serve as a cornerstone for comprehensive understanding and preparation. By leveraging these meticulously crafted MCQs, aspirants can fortify their knowledge, hone problem-solving skills, and excel in their engineering endeavors. Embark on a journey of knowledge enhancement and mastery of engineering concepts with Engineers Publications' MCQs. Strengthen your foundation, assess your skills, and propel yourself towards success in the diverse realms of Electronics, Electrical, Mechanical, and Civil Engineering.
#MCQ for Mechanical Engineering#MCQ for Electrical Engineering#MCQ for Civil Engineering#MCQ for Electronics Engineering
0 notes
Text
STMicroelectronics ST-ONE series USD PD controller power increased to 140W
【Lansheng Technology Information】On April 12, 2023, STMicroelectronics launched ST-ONEHP, the first integrated digital controller chip in the industry to pass the USB-IF USB Power Delivery Extended Power Range specification certification.
As the third controller in the ST-ONE series, the ST-ONEHP has an output voltage of 28V, which simplifies the development and design of chargers and power adapters with a maximum rated power of 140W. The converter uses ST-ONE architecture, a non-complementary active clamp flyback zero-voltage switching (ZVS) topology, which ensures excellent energy efficiency for applications with high power output and high switching frequency. The chip integrates basic functions such as secondary rectifier and USB PD communication interface, which saves material cost and PCB size and layout complexity. Built-in synchronous rectifiers help maximize energy efficiency, and reinforced galvanic isolation circuitry allows designers to develop a solution that is both compact and safe.
Inside ST-ONEHP, an Arm® Cortex®-M0+ microcontroller is responsible for the overall control. The microcontroller comes preloaded with certified USB PD firmware, which simplifies the approval process for final product qualification for the USB Logo. The microcontroller has 64KByte flash memory that can store custom power conversion firmware.
The ST-ONEHP is ideally suited for use with STMicroelectronics' MasterGaN chips integrating the company's third-generation gallium nitride (GaN) power transistors and optimized gate drivers in a single package. Compared with traditional silicon transistors, this SiP solution reduces the difficulty of sampling GaN technology in energy-saving applications, with advantages such as superior thermal performance and switching energy efficiency.
Complementing the advantages of ST-ONE converters, MasterGaN helps to maximize performance and switching frequency, enabling the use of smaller passive components. By integrating the ST-ONEHP controller and a 9mm x 9mm MasterGaN1 half-bridge power stage, STMicroelectronics has produced an EVLONE140W charger reference design that is smaller than a standard 65W laptop charger.
The volume of the charger reference design is 90cm3, which is equivalent to the industry's advanced 25W/in3 power density, and the peak energy efficiency exceeds 94%. From an environmental point of view, this charger uses only a quarter of the plastic of ordinary chargers and is 2% more energy efficient than ordinary chargers. If these products were used in all chargers produced globally, CO2 emissions would be reduced by 3.5 million tonnes.
Lansheng Technology Limited, which is a spot stock distributor of many well-known brands, we have price advantage of the first-hand spot channel, and have technical supports.
Our main brands: STMicroelectronics, Toshiba, Microchip, Vishay, Marvell, ON Semiconductor, AOS, DIODES, Murata, Samsung, Hyundai/Hynix, Xilinx, Micron, Infinone, Texas Instruments, ADI, Maxim Integrated, NXP, etc
To learn more about our products, services, and capabilities, please visit our website at http://www.lanshengic.com
0 notes
Text
Mastering the ESE Electronics Syllabus: Your Path to Excellence in the Examination
Mastering the Electronics Syllabus for the Engineering Services Examination (ESE) requires a focused and systematic approach. Here's a comprehensive path to help you excel in the examination:
1. Understand the Syllabus: Begin by thoroughly understanding the ESE Electronics syllabus. Break it down into topics and subtopics to get a clear picture of what you need to cover.
2. Create a Study Plan: Devise a well-structured study plan that covers all the subjects and topics in the syllabus. Allocate sufficient time to each topic based on its complexity and your familiarity with it.
3. Study Material: Gather quality study materials such as textbooks, reference books, online resources, and lecture notes. Stick to reputable sources to ensure accuracy and clarity.
4. Basic Concepts: Start with building a strong foundation in fundamental concepts. Electronics is built on principles like Ohm's law, Kirchhoff's laws, and basic circuit analysis. Make sure you have a solid grasp of these.
5. Detailed Study: Proceed to more advanced topics, such as semiconductor devices, digital electronics, analog electronics, control systems, and communication systems. Understand the theories, working principles, and applications of various components and systems.
6. Practice Problems: Solve a wide range of practice problems to reinforce your understanding. Look for question banks, previous year's question papers, and sample papers specifically designed for ESE Electronics.
7. Numerical Skills: Develop your problem-solving skills by tackling numerical problems. Electronics often involves calculations, so practice is essential to improve your accuracy and speed.
8. Practical Application: Whenever possible, try to relate theoretical concepts to real-world applications. Understanding how concepts are applied in practical scenarios can deepen your understanding.
9. Circuit Design and Analysis: Learn how to design and analyze circuits, both analog and digital. Understand different types of amplifiers, oscillators, and logic gates. This knowledge is crucial for ESE.
10. Control Systems: Master the fundamentals of control systems, including block diagrams, signal flow graphs, and stability analysis. Know about PID controllers, state-space representation, and stability criteria.
11. Communication Systems: Study communication theory, modulation techniques, multiplexing, and basic signal processing. Understand concepts like amplitude modulation, frequency modulation, and digital modulation.
12. Study Groups and Discussions: Consider joining study groups or online forums where you can discuss complex topics, ask questions, and clarify doubts with fellow aspirants.
13. Mock Tests and Practice Papers: Regularly take mock tests and solve practice papers under exam conditions. This will help you familiarize yourself with the exam pattern, manage time effectively, and identify areas for improvement.
14. Time Management: Practice time management during your preparation and mock tests. Allocate time wisely to different sections of the paper based on your strengths and weaknesses.
15. Revision: Set aside dedicated time for revision. Consistently revisit concepts you've learned to reinforce your memory.
16. Stay Updated: Keep yourself updated with the latest developments in the field of electronics. Subscribe to relevant journals, magazines, and online resources.
17. Stay Healthy: Remember to take breaks, exercise, and maintain a healthy lifestyle. A balanced routine enhances your cognitive abilities and helps you stay focused.
18. Stay Positive: Maintain a positive attitude and believe in your abilities. Confidence plays a crucial role in exam performance.
19. Analyze Performance: After each mock test, analyze your performance. Identify strengths and weaknesses and adjust your study plan accordingly.
20. Last-Minute Preparation: In the final days before the exam, focus on quick revision, formulae, and important concepts. Avoid cramming new topics.
Remember, consistency, dedication, and a smart study approach are key to mastering the ESE Electronics syllabus. Stay focused, and your hard work will surely pay off on the examination day. Good luck!
0 notes
Text
Engineering vocab in Japanese (pt. 1)

Some common engineering related words in Japanese, for anyone interested in engineering or looking at studying it in Japan! This part covers electrical, electronic and information fields (including machine learning)
工学(こうがく)の分野(ぶんや)- fields of engineering
��械工学(きかいこうがく)- mechanical engineering
航空工学(こうくうこうがく)- aeronautical engineering
土木工学(どぼくこうがく)- civil engineering
材料工学(ざいりょうこうがく)- material engineering
電気工学(でんきこうがく)- electrical engineering
電子工学(でんしこうがく)- electronic engineering
情報工学(じょうほうこうがく)- information engineering (computer science)
発電(はつでん)- electricity generation
化石燃料(かせきねんりょう)- fossil fuels
再生可能(さいせいかのう)エネルギー - renewable energy
運動(うんどう)エネルギー - kinetic energy
タービン - turbine
火力発電(かりょくはつ)- thermal energy (from burning fuels)
原子発電(げんしはつでん)- nuclear power
水力発電(すいりょうはつでん)- hydroelectric power
地熱発電(じねんはつでん)- geothermal power
風力発電(ふうりょくはつでん)- wind power
風車(ふうしゃ)- wind turbine
太陽光発電(たいようこうはつでん)- solar power
電気・電子 - electrical and electronics
電子(でんし)- electron
回路(かいろ)- circuit
電圧(でんあつ)- voltage
電流(でんりゅう)- current
抵抗(ていこう)- resistance
増幅器(ぞうふくき)- amplifier
半導体(はんどうたい)- semiconductor
トランジスタ - transistor
コンデンサ - capacitor
電磁波(でんじは)- electromagnetic wave
電波(でんぱ)- radio wave (also phone signal)
電場(でんば)- electric field
磁場(じば)- magnetic field
粒子(りゅうし)- particle
信号処理(しんごうしょり)- signal processing
信号(しんごう)- signal
入力(にゅうりょく)- input
出力(しゅつりょく)- output
フィルタ - filter
ノイズ - noise
誤差(ごさ)- error
利得(りとく)- gain
周波数(しゅうはすう)- frequency
波長(はちょう)- wavelength
フーリエ変換(へんかん)- Fourier transform
制御(せいぎょ)システム - control system
伝達関数(でんたつかんすう)- transfer function
負(ふ)のフィードバック - negative feedback
最適制御(さいてきせいぎょ)- optimal control
機械学習(きかいがくしゅう)- machine learning
教師(きょうし)あり学習 - supervised learning
教師(きょうし)なし学習 - unsupervised learning
強化(きょうか)学習 - reinforcement learning
��練(くんれん)データ - training data
分類(ぶんるい)- classification
最適化(さいてきか)- optimisation
回帰(かいき)- regression
人工神経(じんこうしんけい)- artificial neuron
ニューラルネットワーク - neural network
神経網(しんけいもう)- neural network (less common)
ディープラーニング - deep learning
深層(しんそう)学習 - deep learning (less common)
126 notes
·
View notes