#Scrape Data from H&M
Explore tagged Tumblr posts
Text
How to Extract Products Data from H&M with Google Chrome

Data You Can Scrape From H&M
Product’s Name
Pricing
Total Reviews
Product’s Description
Product’s Details
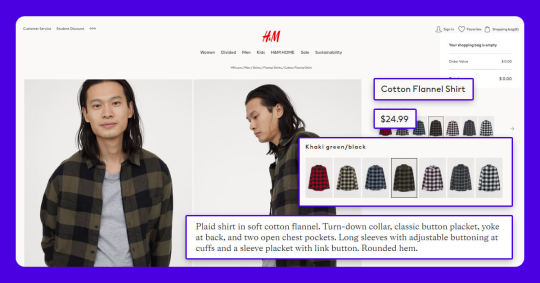
The screenshot provided below indicates various data fields, which we scrape at 3i Data Scraping:
Requests
Google’s Chrome Browser: You would require to download the Chrome browser and the extension requires the Chrome 49+ version.
Web Scraping for Chrome Extension: Web Scraper extension could be downloaded from Chrome’s Web Store. Once downloaded the extension, you would get a spider icon included in the browser’s toolbar.
Finding The URLs
H&M helps you to search products that you could screen depending on the parameters including product types, sizes, colors, etc. The web scraper assists you to scrape data from H&M as per the requirements. You could choose the filters for data you require and copy corresponding URLs. In Web Scraper toolbars, click on the Sitemap option, choose the option named “Edit metadata’ to paste the new URLs (as per the filter) as Start URL.
For comprehensive steps about how to extract H&M data, you may watch the video given here or continue to read:
Importing H&M Scraper
After you install the extension, you can right-click anyplace on the page and go to the ‘Inspect’ option as well as Developer Tool console would pop up. Just click on the ‘Web Scraper’ tab and go to the ‘Create new sitemap’ option as well as click on the ‘Import sitemap’ button. Now paste JSON underneath into Sitemap’s JSON box.
Running The Scraper
To start extracting, just go to the Sitemap option and click the ‘Scrape’ alternative from the drop-down menu. Another window of Chrome will come, permitting the extension for scrolling and collecting data. Whenever the extraction is completed, the browser will be closed automatically and sends the notice.
Downloading The Data
For downloading the extracted data in a CSV file format, which you could open with Google Sheets or MS Excel, go to the Sitemap’s drop-down menu > Export as CSV > Download Now.
Disclaimer: All the codes given in our tutorials are for illustration as well as learning objectives only. So, we are not liable for how they are used as well as assume no liabilities for any harmful usage of source codes. The presence of these codes on our website does not indicate that we inspire scraping or scraping the websites given in the codes as well as supplement the tutorial.
#data extraction#web scraping services#Extract data from H&M#H&M Data Scraping#Data Extractor#Web Scraping Services#Ecommerce data scraping services#Data Extractor from H&M
0 notes
Note
SCP-096
rating: +4203+–X
Item #: SCP-096
Object Class: Euclid
Special Containment Procedures: SCP-096 is to be contained in its cell, a 5 m x 5 m x 5 m airtight steel cube, at all times. Weekly checks for any cracks or holes are mandatory. There are to be absolutely no video surveillance or optical tools of any kind inside SCP-096's cell. Security personnel will use pre-installed pressure sensors and laser detectors to ensure SCP-096's presence inside the cell.
Any and all photos, video, or recordings of SCP-096's likeness are strictly forbidden without approval from Dr. ███ and O5-█.
Description: SCP-096 is a humanoid creature measuring approximately 2.38 meters in height. Subject shows very little muscle mass, with preliminary analysis of body mass suggesting mild malnutrition. Arms are grossly out of proportion with the rest of the subject's body, with an approximate length of 1.5 meters each. Skin is mostly devoid of pigmentation, with no sign of any body hair.
SCP-096's jaw can open to four (4) times the norm of an average human. Other facial features remain similar to an average human, with the exception of the eyes, which are also devoid of pigmentation. It is not yet known whether SCP-096 is blind or not. It shows no signs of any higher brain functions, and is not considered to be sapient.
SCP-096 is normally extremely docile, with pressure sensors inside its cell indicating it spends most of the day pacing by the eastern wall. However, when someone views SCP-096's face, whether it be directly, via video recording, or even a photograph, it will enter a stage of considerable emotional distress. SCP-096 will cover its face with its hands and begin screaming, crying, and babbling incoherently. Approximately one (1) to two (2) minutes after the first viewing, SCP-096 will begin running to the person who viewed its face (who will from this point on be referred to as SCP-096-1).
Documented speeds have varied from thirty-five (35) km/h to ███ km/h, and seems to depend on distance from SCP-096-1. At this point, no known material or method can impede SCP-096's progress. The actual position of SCP-096-1 does not seem to affect SCP-096's response; it seems to have an innate sense of SCP-096-1's location. Note: This reaction does not occur when viewing artistic depictions (see Document 096-1).
Upon arriving at SCP-096-1's location, SCP-096 will proceed to kill and [DATA EXPUNGED] SCP-096-1. 100% of cases have left no traces of SCP-096-1. SCP-096 will then sit down for several minutes before regaining its composure and becoming docile once again. It will then attempt to make its way back to its natural habitat, [DATA REDACTED]
Due to the possibility of a mass chain reaction, including breach of Foundation secrecy and large civilian loss of life, retrieval of subject should be considered Alpha priority.
Dr. ███ has also petitioned for immediate termination of SCP-096 (see Interview 096-1). Order is awaiting approval. Termination order has been approved, and is to be carried out by Dr. ███ on [DATA REDACTED]. See Incident-096-1-A.
Audio log from Interview 096-1:
Interviewer: Dr. ███
Interviewed: Captain (Ret.) █████████, former commander of retrieval team Zulu 9-A
Retrieval Incident #096-1-A
<Begin Log>
[████████ ████████ Time, Research Area ██]
Capt. █████████: It always sucks ass to get Initial Retrieval duty. You have no idea what the damn thing is capable of besides what jacked up information the field techies can scrape up, and you're lucky if they even tell you the whole story. They told us to "bag and tag." Didn't tell us jackshit about not looking at the damn thing.
Dr. ███: Could you describe the mission, please?
Capt. █████████: Yeah, sorry. We had two choppers, one with my team and one on backup with Zulu 9-B and Dr. ██████. We spotted the target about two clicks north of our patrol path. I'm guessing he wasn't facing our direction, else he would have taken us out then and there.
Dr. ███: Your report says SCP-096 didn't react to the cold? It was -██o C.
Capt. █████████: Actually, it was -██. And yes, it was butt naked and didn't so much as shiver. Anyway, we landed, approached the target, and Corporal ██ got ready to bag it. That's when Dr. ██████ called. I turned to answer it, and that's what saved me. The target must have turned and my whole squad saw it.
Dr. ███: That's when SCP-096 entered an agitated emotional state?
Capt. █████████: Yep. [Interviewed now pauses for a second before continuing] Sorry. Got the willies for a second.
Dr. ███: That's all right.
Capt. █████████: Yeah. Well, I never saw its face. My squad did, and they paid for it up the ass.
Dr. ███: Could you describe it a little more, please?
Capt. █████████: [Pauses] Yeah, yeah. It started screaming at us, and crying. Not animal roaring though, sounded exactly like a person. Really fucking creepy. [Pauses again] We started firing when it picked up Corporal ██ and ripped off his leg. God, he was screaming for our help… fuckin 'A… anyway, we were blowing chunks out of the target, round after round. Didn't do jackshit. I almost lost it when it started [DATA EXPUNGED] him.
Dr. ███: That's when you ordered the use of an [Papers are heard moving] AT-4 HEDT launcher?
Capt. █████████: An anti-tank gun. Started carrying it ever since SCP-███ got loose. I've seen those tear through tanks like tissue paper. Did the same thing to the target.
Dr. ███: There was significant damage to SCP-096?
Capt. █████████: It didn't even fucking flinch. It kept tearing apart my squad, but with half of its torso gone. [He draws a large half-circle across his torso]
Dr. ███: But it was taking damage?
Capt. █████████: If it was, it wasn't showing it. It must have lost all its organs, all its blood, but it didn't acknowledge any of it. Its bone structure wasn't hurt at all, though. It kept tearing my squad apart.
Dr. ███: So no actual structural damage. How many rounds would you say were fired at SCP-096?
Capt. █████████: At the least? A thousand. Our door gunner kept his GAU-19 on it for at least twenty seconds. Twenty fucking seconds. That's six hundred .50 caliber rounds pumped into the thing. Might as well been spitting at it.
Dr. ███: This is when Zulu 9-B arrived?
Capt. █████████: Yeah, and my squad was gone. Zulu 9-B managed to get the bag over its head, and it just sat down. We got it into the chopper and got it here. I don't know how I never saw its face. Maybe God or Buddha or whoever thought I should live. The jackass.
Dr. ███: We have obtained an artist's depiction of SCP-096's face. Would you like to view it?
Capt. █████████: [Pauses] You know, after hearing that thing's screams, and the screams of my men, I don't think I want to put a face to what I heard. No. Just… no.
Dr. ███: All right, I believe we are done here. Thank you, Captain.
[Chairs are heard moving, and footsteps leave the room. Captain (Ret.) █████████ is confirmed to have left Interview Room 22.]
Dr. ███: Let this be on record that I am formally requesting SCP-096 be terminated as soon as possible.
<End Log>
OHH GOTCHA
i looked it up. Hey same face/j!!
2 notes
·
View notes
Text
A Guide To Scraping H&M Products With Python And BeautifulSoup For Enhanced Business Insights
A Guide To Scraping H&M Products With Python And BeautifulSoup For Enhanced Business Insights
Retail industries are competing at a high pace. Hence, to stay competitive, retail data scraping has become imperative. This process involves extracting crucial information from competitors' websites, monitoring pricing strategies, and analyzing customer reviews. Retailers utilize H&M product data scraping service to gain insights into market trends, optimize pricing strategies, and enhance inventory management. By staying informed about competitors and market dynamics, businesses can make data-driven decisions, adapt swiftly to changes, and ultimately provide customers with a more competitive and responsive shopping experience. However, ethical practices and compliance with legal requirements are crucial to ensure the responsible use of retail data scraping.
About H&M
Hennes & Mauritz AB is abbreviated as H&M. Since 1947 it has grown into one of the world's prominent fashion retailers, offering a wide range of accessories, clothing, and footwear for men, women, and children. Known for its affordable and trendy fashion, H&M operates globally with a vast network of stores and an online presence, making fashion accessible to a broad consumer base. The company is also committed to sustainability and has initiatives to promote ethical and environmentally conscious practices in the fashion industry. Scrape H&M product data to gather information on product details, prices, and availability for analysis and business insights.
List of Data Fields
Product Name
Product Category
Description
SKU
Brand
Size
Availability
Price
Images
Reviews
Ratings
Specifications
Shipping Information
Significance of Scraping H&M Retail Data
Scraping H&M retail data holds significant strategic importance for businesses aiming to stay competitive and informed in the dynamic retail landscape. Here's a detailed exploration of its significance:
Competitor Intelligence: Web scraping H&M data provides retailers with a comprehensive understanding of their competitors' pricing, product offerings, and promotional strategies. This competitive intelligence is crucial for making informed decisions and staying relevant in the market.
Pricing Strategy Optimization: Retailers can use Ecommerce Data Scraping Services to optimize their pricing strategies by analyzing scraped pricing data from H&M. This includes adjusting prices to remain competitive, offering discounts strategically, and responding promptly to market changes.
Inventory Management Enhancement: Monitoring H&M's product availability and stock levels through web scraping allows retailers to fine-tune their inventory management. It helps minimize stockouts, prevent overstock situations, and ensure efficient supply chain operations.
Market Trend Identification: Scraping H&M data enables businesses to identify and capitalize on emerging market trends. Analyzing product preferences and trends on the H&M platform helps retailers align their offerings with evolving consumer demands.
Customer Preferences Analysis: Examining customer reviews, ratings, and feedback on H&M products using E-Commerce Product Data Scraper gives retailers insights into consumer preferences. This information is invaluable for tailoring product assortments and enhancing the customer experience.
Strategic Decision-Making: The scraped data from H&M serves as a foundation for strategic decision-making. Retailers can adapt their business strategies based on observed patterns, ensuring agility in response to changing market conditions.
Product Assortment Planning: Detailed information on H&M's product categories, styles, and assortments aids retailers in planning their product range. It helps in aligning offerings with current fashion trends and customer expectations.
User Experience Enhancement: Utilizing scraped data empowers retailers to enhance the overall user experience. By incorporating successful elements observed on the H&M platform, businesses can optimize their website design, marketing strategies, and customer engagement tactics.
Today, we'll explore scraping H&M products with Python and BeautifulSoup in an uncomplicated and elegant way. This article introduces you to real-world problem-solving, ensuring simplicity and practical results for a quick understanding.
To begin, ensure you have Python 3 installed. If not, install Python 3 before proceeding. Next, install Beautiful Soup with:pip3 install beautifulsoup4
Additionally, we require library requests, lxml, and soupsieve to fetch data, convert it to XML, and utilize CSS selectors. Install these libraries by using the following command:pip3 install requests soupsieve 1xml
Save this as scrapeHM.py.
If you execute it:python3 scrapeHM.py
You will observe the entire HTML page.
When deploying this in production and aiming to scale to numerous links, encountering IP blocks from H&M is likely. To address this, employing a rotating proxy service becomes essential. Utilizing a Proxies API enables routing calls through a vast pool of residential proxies, mitigating the risk of IP blocks.
Feel free to get in touch with iWeb Data Scraping for comprehensive information! Whether you seek web scraping service or mobile app data scraping, our team can assist you. Contact us today to explore your requirements and discover how our data scraping solutions can provide you with efficiency and reliability tailored to your unique needs.
Know More: https://www.iwebdatascraping.com/scraping-h-and-m-products-with-python-and-beautifulsoup.php
#ScrapingHandMProductsWithPythonAndBeautifulSoup#ScrapeHandMProductsUsingPythonandBeautifulSoup#HandMScraper#HandMproductdataCollectionservice#HandMproductdatascrapingservice#ExtractHandMProductsWithPythonAndBeautifulSoup#HandMProductsWithPythonAndBeautifulSoupdataextractor#HandMProductsWithPythonAndBeautifulSoupdataextraction
0 notes
Link
What Is H&M?
H&M is a multinational Clothing-Retail & Accessories providing company that provides clothes for men, women, teenagers, and Children. H&M operates in more than 74 countries with 5000+ stores in the world with various company brands and have over 1,26,000 full-time employees. H&M is the world’s 2nd largest clothing retail company. H&M provides online shopping in 33 countries. The estimated revenue of H&M is $25.191 Billion.
List Of Data Fields
At RetailGators, we extract the required data fields given below. We extract H&M using Web Scraper Chrome Extraction.
Product Name
Price
Number of Reviews
Product Description
Product Details
Category of Cloths
Product Color
Product Size
Product Type
How We Scrape Data From H&M?
H&M allows you to search for different products that can be filtered as per factors like product price, product type, size, color, and many others. The Scraper will allow you to scrape data from the H&M as per your requirements. You need to select filters for your data you need to copy the equivalent URL.
H&M Data Scraping Tool helps you to collect structured data from H&M from the traffic using H&M normally. H&M scraper helps you to extract product data from H&M fashion online store. It allows you to scrape the whole site, particular categories, and products.
H&M Scraper
After adding an extension, you need to right-click anywhere on a page, or you need to inspect and develop tools and a popup will come up. Click on the tab name Web Scraper and you can go on to ‘Create New Sitemaps’ after that you need to click on the button and can easily import the sitemap option. RetailGators helps you to download the data into many different formats like CSV, JSON, and Excel.
Why RetailGators?
We have a professional team that helps you immediately if you have any problems while using H&M Data Scraping Services. Our eCommerce Data Scraping Services is capable, reliable, and offer you quick results without any error.
Scraping H&M Scraping Services helps you to save your time, money, and efforts. We can scrape data in a couple of hours, which might take a week or days if you do it yourself.
We are working on H&M Data Scraping Service Using Google Chrome that extract the required data.
Our expert team of H&M Data Scraping Services API knows how to convert unstructured data into structured data.
So if you are looking for the best H&M Product Data Scraping Services, then contact RetailGators for all your queries.
source code: https://www.retailgators.com/how-to-scrape-product-data-from-h-and-m-using-google-chrome.php
#scrape h&m product data#extract h&m product data#Scrape Data from H&M#ecommerce web scraping tool#ecommerce data scraping service
0 notes
Text
top 10 free python programming books pdf online download
link :https://t.co/4a4yPuVZuI?amp=1
python download python dictionary python for loop python snake python tutorial python list python range python coding python programming python array python append python argparse python assert python absolute value python append to list python add to list python anaconda a python keyword a python snake a python keyword quizlet a python interpreter is a python code a python spirit a python eating a human a python ate the president's neighbor python break python basics python bytes to string python boolean python block comment python black python beautifulsoup python built in functions b python regex b python datetime b python to dictionary b python string prefix b' python remove b' python to json b python print b python time python class python certification python compiler python command line arguments python check if file exists python csv python comment c python interface c python extension c python api c python tutor c python.h c python ipc c python download c python difference python datetime python documentation python defaultdict python delete file python data types python decorator d python format d python regex d python meaning d python string formatting d python adalah d python float d python 2 d python date format python enumerate python else if python enum python exit python exception python editor python elif python environment variables e python numpy e python for everyone 3rd edition e python import e python int e python variable e python float python e constant python e-10 python format python function python flask python format string python filter python f string python for beginners f python print f python meaning f python string format f python float f python decimal f python datetime python global python global variables python gui python glob python generator python get current directory python getattr python get current time g python string format g python sleep g python regex g python print g python 3 g python dictionary g python set g python random python hello world python heapq python hash python histogram python http server python hashmap python heap python http request h python string python.h not found python.h' file not found python.h c++ python.h windows python.h download python.h ubuntu python.h not found mac python if python ide python install python input python interview questions python interpreter python isinstance python int to string in python in python 3 in python string in python meaning in python is the exponentiation operator in python list in python what is the result of 2 5 in python what does mean python json python join python join list python jobs python json parser python join list to string python json to dict python json pretty print python j complex python j is not defined python l after number python j imaginary jdoodle python python j-link python j+=1 python j_security_check python kwargs python keyerror python keywords python keyboard python keyword arguments python kafka python keyboard input python kwargs example k python regex python k means python k means clustering python k means example python k nearest neighbor python k fold cross validation python k medoids python k means clustering code python lambda python list comprehension python logging python language python list append python list methods python logo l python number l python array python l-bfgs-b python l.append python l system python l strip python l 1 python map python main python multiprocessing python modules python modulo python max python main function python multithreading m python datetime m python time python m flag python m option python m pip install python m pip python m venv python m http server python not equal python null python not python numpy python namedtuple python next python new line python nan n python 3 n python meaning n python print n python string n python example in python what is the input() feature best described as n python not working in python what is a database cursor most like python online python open python or python open file python online compiler python operator python os python ordereddict no python interpreter configured for the project no python interpreter configured for the module no python at no python 3.8 installation was detected no python frame no python documentation found for no python application found no python at '/usr/bin python.exe' python print python pandas python projects python print format python pickle python pass python print without newline p python re p python datetime p python string while loop in python python p value python p value from z score python p value calculation python p.map python queue python queue example python quit python qt python quiz python questions python quicksort python quantile qpython 3l q python download qpython apk qpython 3l download for pc q python 3 apk qpython ol q python 3 download for pc q python 3 download python random python regex python requests python read file python round python replace python re r python string r python sql r python package r python print r python reticulate r python format r python meaning r python integration python string python set python sort python split python sleep python substring python string replace s python 3 s python string s python regex s python meaning s python format s python sql s python string replacement s python case sensitive python try except python tuple python time python ternary python threading python tutor python throw exception t python 3 t python print .t python numpy t python regex python to_csv t python scipy t python path t python function python unittest python uuid python user input python uppercase python unzip python update python unique python urllib u python string u' python remove u' python json u python3 u python decode u' python unicode u python regex u' python 2 python version python virtualenv python venv python virtual environment python vs java python visualizer python version command python variables vpython download vpython tutorial vpython examples vpython documentation vpython colors vpython vector vpython arrow vpython glowscript python while loop python write to file python with python wait python with open python web scraping python write to text file python write to csv w+ python file w+ python open w+ python write w+ python open file w3 python w pythonie python w vs wb python w r a python xml python xor python xrange python xml parser python xlrd python xml to dict python xlsxwriter python xgboost x python string x-python 2 python.3 x python decode x python 3 x python byte x python remove python x range python yield python yaml python youtube python yaml parser python yield vs return python yfinance python yaml module python yaml load python y axis range python y/n prompt python y limit python y m d python y axis log python y axis label python y axis ticks python y label python zip python zipfile python zip function python zfill python zip two lists python zlib python zeros python zip lists z python regex z python datetime z python strftime python z score python z test python z transform python z score to p value python z table python 0x python 02d python 0 index python 0 is false python 0.2f python 02x python 0 pad number python 0b 0 python meaning 0 python array 0 python list 0 python string 0 python numpy 0 python matrix 0 python index 0 python float python 101 python 1 line if python 1d array python 1 line for loop python 101 pdf python 1.0 python 10 to the power python 101 youtube 1 python path osprey florida 1 python meaning 1 python regex 1 python not found 1 python slicing 1 python 1 cat 1 python list 1 python 3 python 2.7 python 2d array python 2 vs 3 python 2.7 download python 2d list python 2.7 end of life python 2to3 python 2 download 2 python meaning 2 pythons fighting 2 pythons collapse ceiling 2 python versions on windows 2 pythons fall through ceiling 2 python versions on mac 2 pythons australia 2 python list python 3.8 python 3.7 python 3.6 python 3 download python 3.9 python 3.7 download python 3 math module python 3 print 3 python libraries 3 python ide python3 online 3 python functions 3 python matrix 3 python tkinter 3 python dictionary 3 python time python 4.0 python 4 release date python 4k python 4 everyone python 44 mag python 4 loop python 474p remote start instructions python 460hp 4 python colt 4 python automl library python 4 missile python 4 download python 4 roadmap python 4 hours python 5706p python 5e python 50 ft water changer python 5105p python 5305p python 5000 python 5706p manual python 5760p 5 python data types 5 python projects for beginners 5 python libraries 5 python projects 5 python ide with icons 5 python program with output 5 python programs 5 python keywords python 64 bit python 64 bit windows python 64 bit download python 64 bit vs 32 bit python 64 bit integer python 64 bit float python 6 decimal places python 660xp 6 python projects for beginners 6 python holster 6 python modules 6 python 357 python 6 missile python 6 malware encryption python 6 hours python 7zip python 7145p python 7754p python 7756p python 7145p manual python 7145p remote start python 7756p manual python 7154p programming 7 python tricks python3 7 tensorflow python 7 days ago python 7 segment display python 7-zip python2 7 python3 7 ssl certificate_verify_failed python3 7 install pip ubuntu python 8 bit integer python 881xp python 8601 python 80 character limit python 8 ball python 871xp python 837 parser python 8.0.20 8 python iteration skills 8 python street dakabin python3 8 tensorflow python 8 puzzle python 8 download python 8 queens python 95 confidence interval python 95 percentile python 990 python 991 python 99 bottles of beer python 90th percentile python 98-381 python 9mm python 9//2 python 9 to 09 python 3 9 python 9 subplots pythonrdd 9 at rdd at pythonrdd.scala python 9 line neural network python 2.9 killed 9 python
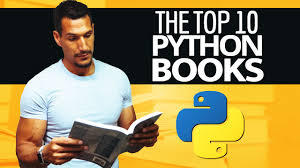
#pythonprogramming #pythoncode #pythonlearning #pythons #pythona #pythonadvanceprojects #pythonarms #pythonautomation #pythonanchietae #apython #apythonisforever #apythonpc #apythonskin #apythons #pythonbrasil #bpython #bpythons #bpython8 #bpythonshed #pythoncodesnippets #pythoncowboy #pythoncurtus #cpython #cpythonian #cpythons #cpython3 #pythondjango #pythondev #pythondevelopers #pythondatascience #pythone #pythonexhaust #pythoneğitimi #pythoneggs #pythonessgrp #epython #epythonguru #pythonflask #pythonfordatascience #pythonforbeginners #pythonforkids #pythonfloripa #fpython #fpythons #fpythondeveloper #pythongui #pythongreen #pythongame #pythongang #pythong #gpython #pythonhub #pythonhackers #pythonhacking #pythonhd #hpythonn #hpythonn✔️ #hpython #pythonista #pythoninterview #pythoninterviewquestion #pythoninternship #ipython #ipythonnotebook #ipython_notebook #ipythonblocks #ipythondeveloper #pythonjobs #pythonjokes #pythonjobsupport #pythonjackets #jpython #jpythonreptiles #pythonkivy #pythonkeeper #pythonkz #pythonkodlama #pythonkeywords #pythonlanguage #pythonlipkit #lpython #lpythonlaque #lpythonbags #lpythonbag #lpythonprint #pythonmemes #pythonmolurusbivittatus #pythonmorphs #mpython #mpythonprogramming #mpythonrefftw #mpythontotherescue #mpython09 #pythonnalchik #pythonnotlari #pythonnails #pythonnetworking #pythonnation #pythonopencv #pythonoop #pythononline #pythononlinecourse #pythonprogrammers #ppython #ppythonwallet #ppython😘😘 #ppython3 #pythonquiz #pythonquestions #pythonquizzes #pythonquestion #pythonquizapp #qpython3 #qpython #qpythonconsole #pythonregiusmorphs #rpython #rpythonstudio #rpythonsql #pythonshawl #spython #spythoniade #spythonred #spythonredbackpack #spythonblack #pythontutorial #pythontricks #pythontips #pythontraining #pythontattoo #tpythoncreationz #tpython #pythonukraine #pythonusa #pythonuser #pythonuz #pythonurbex #üpython #upython #upythontf #pythonvl #pythonvert #pythonvertarboricole #pythonvsjava #pythonvideo #vpython #vpythonart #vpythony #pythonworld #pythonwebdevelopment #pythonweb #pythonworkshop #pythonx #pythonxmen #pythonxlanayrct #pythonxmathindo #pythonxmath #xpython #xpython2 #xpythonx #xpythonwarriorx #xpythonshq #pythonyazılım #pythonyellow #pythonyacht #pythony #pythonyerevan #ypython #ypythonproject #pythonz #pythonzena #pythonzucht #pythonzen #pythonzbasketball #python0 #python001 #python079 #python0007 #python08 #python101 #python1 #python1k #python1krc #python129 #1python #python2 #python2020 #python2018 #python2019 #python27 #2python #2pythons #2pythonsescapedfromthezoo #2pythons1gardensnake #2pythons👀 #python357 #python357magnum #python38 #python36 #3pythons #3pythonsinatree #python4kdtiys #python4 #python4climate #python4you #python4life #4python #4pythons #python50 #python5 #python500 #python500contest #python5k #5pythons #5pythonsnow #5pythonprojects #python6 #python6s #python69 #python609 #python6ft #6python #6pythonmassage #python7 #python734 #python72 #python777 #python79 #python8 #python823 #python8s #python823it #python800cc #8python #python99 #python9 #python90 #python90s #python9798
1 note
·
View note
Text
Exploring D:BH fics (Part 5)
For this part, I’m going to discuss how I prepared the data and conducted the tests for differences in word use across fics from the 4 AO3 ratings (Gen/Teen/Mature/Explicit), as mentioned here.
Recap: Data was scraped from AO3 in mid-October. I removed any fics that were non-English, were crossovers and had less than 10 words. A small number of fics were missed out during the scrape - overall 13933 D:BH fics remain for analysis.
In this particular analysis, I dropped all non-rated fics, leaving 12647 D:BH fics for the statistical tests.
Part 1: Publishing frequency for D:BH with ratings breakdown Part 2: Building a network visualisation of D:BH ships Part 3: Topic modeling D:BH fics (retrieving common themes) Part 4: Average hits/kudos/comment counts/bookmarks received (split by publication month & rating) One-shots only. Part 5: Differences in word use between D:BH fics of different ratings Part 6: Word2Vec on D:BH fics (finding similar words based on word usage patterns) Part 7: Differences in topic usage between D:BH fics of different ratings Part 8: Understanding fanon representations of characters from story tags Part 9: D:BH character prominence in the actual game vs AO3 fics
What differentiates mature fics from explicit fics, gen from teen fics?
These are pretty open-ended questions, but perhaps the most rudimentary way (quantitatively) is to look at word use. It’s very crude and ignores word order and can’t capture semantics well - but it’s a start.
I’ve read some papers/writings where loglikelihood ratio tests and chi-squared tests have been used to test for these word use differences. But recently I came across this paper which suggests using other tests instead (e.g. trusty old t-tests, non-parametric Mann-Whitney U-tests, bootstrap tests). I went ahead with the non-parametric suggestion (specifically, the version for multiple independent groups, the Kruskal-Wallis test).
Now, on to pre-processing and other details.
1. Data cleaning. Very simple cleaning since I wanted to retain all words for potential analysis (yes, including the common stopwords like ‘the’, etc!). I just cleaned up the newlines, removed punctuation and numbers, and the ‘Work Text’ and ‘Chapter Text’ indicators from the HTML. At the end of cleaning, each story was basically just a list of words, e.g. [’connor’, ‘said’, ‘hello’, ‘i’, ‘m’, ‘not’, ‘a’, ‘deviant’].
2. Preparing a list of vocabulary for testing. With 12647 fics, you can imagine that there’s a huge amount of potential words to test. But a lot of them are probably rare words that aren’t used that often outside of a few fics. I’m trying to get an idea of general trends so those rare words aren’t helpful to this analysis.
I used Gensim’s dictionary function to help with this filtering. I kept only words that appeared in at least 250 fics. The number is pretty arbitrary - I selected because the smallest group (Mature fics) had 2463 fics; so 250 was about 10% of that figure. This left me with 9916 unique words for testing.
3. Counting word use for every fic. I counted the number of times each fic used each of the 9916 unique words.
Now obviously raw frequencies won’t do - a longer fic is probably going to have higher frequency counts for most words (versus a short fic) just by virtue of its length. To take care of this I normalised each frequency count for each fic by the fic’s length. E.g. ‘death’ appears 100 times in a 1000-word fic, the normalised count is 100/1000 = 0.1.
4. Performing the test (Kruskal-Wallis) and correcting for multiple comparisons. I used the Kruskal-Wallis test (from scipy) instead of the parametric ANOVA because it’s less sensitive to outliers; while the ANOVA looks at group means, the Kruskal-Wallis looks at group mean ranks (not group medians!). As an aside, you can assume the K-W test is comparing medians, if you are able to assume that the distributions of all the groups you’re comparing are identically shaped.
Because we’re doing so many comparisons (9916, one for each word), we’re bound to run into some false positives when testing for significance. To control for this, I used the Holm-Bonferroni correction (from multipy), correcting at α =.05. Even after correction, I had 9851 words with significant differences between the 4 groups.
[[For anyone unfamiliar with what group mean ranks, I’ll cover it here (hope I’ve got it down right more or less erp): - We have 12647 fics, and we have a normalised count for each of them on 9916 words. - For each word, e.g. ‘happy’, we rank the fics by their normalised count. So the fic with the lowest normalised count of ‘happy’ gets a rank of 1, the fic with the highest normalised count of ‘happy’ gets rank 12647. Every other fic gets a corresponding rank too. - We sum the ranks within each group (Gen/T/M/Explicit). Then we calculate the average of those group-wise ranks, getting the mean ranks. This information is used in calculating the test statistic for K-W.]]
5. Post-hoc tests. The results of the post-hoc tests aren’t depicted in the charts (didn’t have a good idea on how to go about it, might revisit this), but I’ll talk about them briefly here.
Following the K-W tests, I performed pairwise Dunn tests for each word (using scikit-posthocs). So basically the K-W test told me, “Hey, there’s some significant differences between the groups here” - but it didn’t tell me exactly where. The Dunn tests let me see, for e.g., if the differences lie between teen/mature, and/or mature/explicit, and so on.
Again, I applied a correction (Bonferroni) for each comparison at α =.05 - since for each word, we’re doing a total of 6 pairwise comparisons between the 4 ratings.
6. Visualisation. I really didn’t know what was the best way to show so many results, but decided for now to go with dot plots (from plotly).
There’s not much to say here since plotly works very well with the pandas dataframes I’ve been storing all my results in!
7. Final points to note
1) For the chart looking at words where mature fics ranked first in mean ranks and the chart looking at words where explicit fics ranked first in mean ranks, I kept only words that were significantly different between mature and explicit fics in the pairwise comparison. I was interested in how the content of these two ratings may diverge in D:BH.
2) For the mature/explicit-first charts, I showed only the top 200 words in terms of the K-W H-statistic (there were just too many words!). The H-statistic can be converted to effect sizes; larger H-statistics would correspond to larger effect sizes.
3) Most importantly, this method doesn’t seem to work very well for understanding teen and especially gen fics. Gen fics just appear as the absence of smut/violence/swearing, which isn’t very informative. This is an issue I’d like to continue working on - I’m sure more linguistic cues, especially contextual ones, will be helpful.
1 note
·
View note
Text
Papanicolaou smear (Pap smear, cervical smear) is a safe, noninvasive cytological examination for early detection of cervical cancer. During the 1900s, cervical cancer was one of the leading cause of death among women. It was until the year 1928, where a greek physician George Nicholas Papanicolaou was able to discover the difference between normal and malignant cervical cells by viewing the samples microscopically, hence Pap smear was invented.
For women ages 30 and above, this procedure can be done in conjunction with a test on Human papillomavirus (HPV), the most common sexually transmitted disease and primary causative agent for cervical cancer. The American Cancer Society recommends a Pap smear at least once every three years for women ages 21 to 29 who are not in a high-risk category and who have had negative results and who have had negative results from three previous Pap tests. While a Pap test and an HPV test is recommended every five years for women ages 30 to 65 years old. If a Pap smear is positive or suggests malignancy, a cervical biopsy can confirm the diagnosis.
Nurses play an important role in promoting public health awareness to inform, encourage and motivate the public in considering health screening such as pap smear. This pap smear study guide can help nurses understand their tasks and responsibilities during the procedure.
[toc]
Indications of Pap Smear
Pap smear is indicated for the following reasons:
Identify the presence of sexually transmitted disease such as human papillomavirus (HPV), herpes, chlamydia, cytomegalovirus, Actinomyces spp., Trichomonas vaginalis, and Candida spp.
Detect primary and metastatic neoplasms
Evaluate abnormal cervical changes (cervical dysplasia)
Detect condyloma, vaginal adenosis, and endometriosis
Assess hormonal function
Evaluate the patient’s response to chemotherapy and radiation therapy
Interfering Factors
These are factors or conditions that may alter the outcome of the study
Delay in fixing a specimen, allows the cells to dry therefore destroying the effectiveness of the stain and makes cytologic interpretation difficult
Improper collection site may cause rejection of the specimen. Samples for hormonal evaluation are taken from the vagina while samples for cancer screening are obtained from the vaginal fornix
Use of lubricating jelly on the speculum that may affect the viability of some organisms
Specimen collection during normal menstruation since blood can contaminate the sample
Douching, using tampons, or having sexual intercourse within 24 hours before the exam can wash away cellular deposits
Existing vaginal infections that may interfere with hormonal cytology
Pap Smear Procedure
Pap smear is performed by a practitioner and takes approximately about 5 to 10 minutes. The step-by-step procedure is as follows:
The patient is positioned. The client is assisted in a supine, dorsal lithotomy position with feet in stirrups.
A speculum is inserted. The practitioner puts on gloves and inserts an unlubricated plastic or metal speculum into the vagina and is opened gently to spread apart the vagina to access the cervix. The speculum may be moistened with saline solution or warm water to make insertion easier.
Cervical and vaginal specimens collection. After positioning the speculum, specimen from the vagina and cervix are taken. A cytobrush is inserted inside the cervix and rolls it firmly into the endocervical canal. The brush is then rotated one turn and removed. A plastic or wooden spatula is utilized to scrape the outer opening of the cervix and vaginal wall.
Collection technique (Using the conventional collection). The specimen from the brush and spatula is wiped on the slide and fixed immediately by immersing the slide in equal parts of 95% ethanol or by using a spray fixative.
Collection technique (Using the ThinPrep collection). The brush and spatula are immediately immersed in a ThinPrep solution with a swirling motion to release the material. The brush and spatula are then removed from the solution and the bottle lid is replaced and secured.
Label the specimen The slides are properly labeled with the patient’s name, age, initials of the health care provider collecting the specimen, date, and time of collection.
Specimens are sent to the laboratory The specimens are transported to the laboratory for cytologic analysis.
Bimanual examination may follow. After the removal of the speculum, a bimanual examination may be performed wherein the health care provider will insert two fingers of one hand inside the vaginal canal to feel the uterus and ovaries with the other hand on top of the abdomen.
Nursing Responsibility for Pap Smear
The following are the nursing interventions and nursing care considerations for a patient indicated for Pap smear.
Before the procedure
The following are the nursing interventions prior to pap smear:
Secure patient’s consent. The test must be adequately explained and understood by the patient before a written, and informed consent is obtained.
Obtain the patient’s health history. These include parity, date of last menstrual period, surgical status, contraceptive use, history of vaginal bleeding, history of previous Pap smears, and history of radiation or chemotherapy.
Ask lists of the patient’s current medications. If a patient is taking a vaginal antibiotic, the pap smear is delayed for one month after the treatment has been completed.
Explain that Pap smear is painless. The test requires that the cervix may be scraped and may experience minimal discomfort but no pain from the insertion of the speculum.
Avoid interfering factors. Having sexual intercourse within 24 hours, douching within 48 hours, using a tampon, or applying vaginal creams or lotions is avoided before the test since it can wash away cellular deposits and change the ph of the vagina.
Empty the bladder. Pap smear involves the insertion of the speculum into the vagina and could press down the lower abdomen.
After the procedure
The nurse should note the following nursing interventions after pap smear:
Cleanse the perineal area. Secretions or excess lubricant from the vagina are removed and cleansed.
Provide a sanitary pad. Slight spotting may occur after the pap smear.
Provide information about the recommended frequency of screening. The American Cancer Society recommends screening every three years for women aged 21 to 29 years old and co-testing for HPV and cytological screening every five years for women aged 30 to 65 years old.
Answer any questions or fears by the patient or family. Anxiety related with the pending test results may occur. Discussion of the implications of abnormal test results on the patient’s lifestyle may be provided to the patient.
Results
Normal findings in a Pap smear will indicate a negative result which means that no abnormal, malignant cells or atypical cells are found. While a positive result signifies that there are abnormal or unusual cells discovered, it is not synonymous to having cervical cancer.
The Bethesda System (TBS) is the current method for interpreting cervical cytology and it includes the following components.
1. Adequacy of specimen
Satisfactory for evaluation: Describe the presence or absence of endocervical transformation zone component and other quality indicators such as partially obscuring blood, inflammation.
Unsatisfactory for evaluation: Specimen is rejected (specify reason) or the specimen is processed and examined but unsatisfactory for evaluation of epithelial abnormalities (specify reason)
2. Interpretation/result
Negative for intraepithelial lesion or malignancy
Showing evidence of organism causing infection:
Trichomonas vaginalis; fungal organisms morphologically consistent with Candida spp.; a shift in flora indicative of bacterial vaginosis (coccobacillus); bacteria consistent with Actinomyces spp.; cellular changes consistent with herpes simplex virus.
Other non-neoplastic findings:
Reactive cellular changes related to inflammation (includes repair), radiation, intrauterine device use, atrophy, glandular cell status after hysterectomy.
Epithelial cell abnormalities
Squamous cell abnormalities
Atypical squamous cells of undetermined significance (ASC-US) cannot exclude HSIL (ASC-H):
Low-grade squamous intraepithelial lesion (LSIL) encompassing HPV, mild dysplasia, cervical intraepithelial neoplasm (CIN) grade 1
High-grade squamous intraepithelial lesion (HSIL) encompassing moderate and severe dysplasia, CIS/CIN grade 2 and CIN grade 3 with features suspicious for invasion (If invasion is suspected).
Squamous cell carcinoma: indicate the presence of cancerous cells.
Glandular cell
Atypical glandular cells (not otherwise specify)
Atypical glandular cells, favor neoplastic (not otherwise specify)
Endocervical adenocarcinoma in situ
Adenocarcinoma
Others
Endometrial cells (in woman >=40 years of age)
Gallery
Related images to help you understand pap smear better.
This slideshow requires JavaScript.
References and Sources
Additional resources and references for the Pap Smear study guide:
Adele Pillitteri. Maternal and Child Health Nursing:Care of the Childbearing and Childrearing Family. Lippincott Williams & Wilkins.
Anne M. Van Leeuwen, Mickey Lynn Bladh. Laboratory & Diagnostic Tests with Nursing Implications: Davis’s
Solomon, D., Davey, D., Kurman, R., Moriarty, A., O’connor, D., Prey, M., … & Young, N. (2002). The 2001 Bethesda System: terminology for reporting results of cervical cytology. Jama, 287(16), 2114-2119. [Link]
Suzanne C. Smeltzer. Brunner & Suddarth’s Handbook of Laboratory and Diagnostic Tests: Lippincott Williams & Wilkins
Pap Smear Nursing Care Planning and Responsibilities – Diagnostic and Procedure
Pap Smear (Papanicolaou Smear) Papanicolaou smear (Pap smear, cervical smear) is a safe, noninvasive cytological examination for early detection of cervical cancer.
#Actinomyces spp.#American Cancer Society#Atypical squamous cells of undetermined significance (ASCUS)#Candida spp#Carcinoma in situ (CIS)#cervical cancer#cervical dysplasia#cervical smear#chlamydia#cytomegalovirus#Diagnostic Procedure#George Papanicolaou#Herpes#High-grade squamous intraepithelial lesions (HSIL)#human papillomavirus#Low-grade squamous intraepithelial lesions (LSIL)#Pap smear#Papanicolaou smear#ThinPrep#Trichomonas vaginalis#vaginal speculum
1 note
·
View note
Text
Data Is Plural The Datasets We’re Looking At This Week By Jeremy Singer-Vine Jul. 6, 2022, at 12:00 PM .share .has-bugs .single-featured-image You’re reading Data Is Plural, a weekly newsletter of useful/curious datasets. Below you’ll find the July 6, 2022, edition, reprinted with permission at FiveThirtyEight. 2022.07.06 edition Banned and challenged books, mass expulsions, European air traffic, Shakespeare and Saturday Night Live. Banned and challenged books. A recent report from PEN America identified 1,500-plus decisions, made between July 2021 and March 2022, to ban books from classrooms and school libraries. A spreadsheet accompanying the report lists each decision’s date, type, state and school district, as well as each banned book’s title, authors, illustrators and translators. Related: Independent researcher Tasslyn Magnusson, in partnership with EveryLibrary, maintains a spreadsheet of both book bans and book challenges, with 3,000-plus entries since the 2021–22 school year. [h/t Gary Price] Mass expulsions. Political scientist Meghan M. Garrity’s Government-Sponsored Mass Expulsion dataset focuses on “policies in which governments systematically remove ethnic, racial, religious or national groups, en masse.” Using a combination of archival research and secondary sources, Garrity documents 139 such events, estimated to have expelled more than 30 million people between 1900 and 2020. For each expulsion, the dataset provides “information on the expelling country, onset, duration, region, scale, category of persons expelled and frequency.” To download it, visit the Journal of Peace Research’s replication data portal and search for “mass expulsion.” European air traffic. Eurocontrol, the main organization coordinating Europe’s air traffic management, publishes an “aviation intelligence portal” with a range of industry metrics, including traffic reports that count the daily number of flights by country, airport and operator. The portal also offers bulk datasets on topics such as airport traffic, flight efficiency, estimated CO2 emissions and more. [h/t Giuseppe Sollazzo] Shakespeare. The Folger Shakespeare “brings you the complete works of the world’s greatest playwright, edited for modern readers.” Its digital editions of the Bard’s plays and poems are available to read online and to download in various file formats. It also provides an API, with endpoints for synopses, roles, monologues, word frequencies and more. [h/t Cameron Armstrong] “Saturday Night Live.” Joel Navaroli’s snlarchives.net aims to catalog and cross-reference every episode, cast member, host, character, sketch, impression and other aspects of the show’s 47-and-counting seasons. An open-source project by Hendrik Hilleckes and Colin Morris scrapes much of that information into structured data files. As seen in: Morris’s 2017 analysis of gender representation in SNL sketches. Dataset suggestions? Criticism? Praise? Send feedback to [email protected]. Looking for past datasets? This spreadsheet contains them all. Visit data-is-plural.com to subscribe and to browse past editions. Source: The Datasets We’re Looking At This Week
0 notes
Text
How Web Scraping Is Used To Scrape Product Data From Target?
Search Target.com for the products you want to scrape and provide us with the URL for the results. In a scraper, enter a ZIP code for the store you want to acquire prices and stock availability for. You'll have a spreadsheet with product information such as Product Name, Store Availability Price, Online Availability, Rating, and Description, among other things.
Target Product’s Information and Pricing Scraper
Our Target Scraper will scrape information from any Target store using simply the search result URLs as well as the zip code.
Our Target.com data scraper will scrape the following information:
Name
Product availability
Pricing
Online Availability status
Store Address
Brand
Seller
Model
Product Images
Contact details
Product specification
Rating Histogram
Customer reviews
To demonstrate this, we took all of the products in the “Women " category from a nearby Target Store in Zip code 10001 and put them together in a table (in New York, CA). Check this link on how to do https://www.target.com/c/women/-/N-5xtd3.
Receive a product category link using Target.com through browsing several categories.
Set your Zip code 10001
The following would be the input for the Target.com data scraper:
Search listing or Keywords URLs at
https://www.target.com/c/sleepwear-pajamas-robes-women-s-clothing/-/N-5xtc3
The Zip code will be 10001
Then, using the parameters, search for a specific type of product, filter the results, and scrape various effects.
Let's look at a "Women's Short Sleeve V-Neck T-Shirt" created by Universal Thread from a Target Store near New York, NY as an example (with Zip Code 10001). Let's look at how to construct a scraper to accomplish this.
Search the keyword — Women's Short Sleeve V-Neck T-Shirt on Target.com.
Copy the search URL
https://www.target.com/c/sleepwear-pajamas-robes-women-s-clothing/-/N-5xtc3Z1vs54?Nao=0
Set your Zip code at 10001
The input to your Target.com data scraper will be:
Search listings or Keywords URL
https://www.target.com/c/sleepwear-pajamas-robes-women-s-clothing/-/N-5xtc3Z1vs54?Nao=0
Zip Code- 10001
JSON
#: "1", URL: "https://www.target.com/p/women-s-short-sleeve-v-neck-t-shirt-universal-thread/-/A-78673828?preselect=79646356#lnk=sametab", Image URL: "https://target.scene7.com/is/image/Target/GUEST_913b04a6-f0d4-4423-b266-9fa94cfbd46b?fmt=webp&wid=1400&qlt=80 | https://target.scene7.com/is/image/Target/GUEST_e1d4bcc4-4a82-4393-9003-4fc3dfb78f5f?fmt=webp&wid=1400&qlt=80 | https://edge.curalate.com/v1/img/7Q47X98QfRlIfAeCFpUclMkISbjtDBPtsw71O27c0ps=/h/1200?compression=0.75 | https://edge.curalate.com/v1/img/5WvyEL7PeAuDHLj_tQP1nKOZDo5TQ2Ote9kAfqj4_gA=/h/1200?compression=0.75 | https://edge.curalate.com/v1/img/z6D4nwiW3JNzVRhMf9-8c1WKZNvFwNbuSBINtXuiG2U=/h/1200?compression=0.75 | https://edge.curalate.com/v1/img/lx-ryGf4Ibq8zpf38NLSFYjDD2Nh4BYiilEicu241Jk=/h/1200?compression=0.75 | https://edge.curalate.com/v1/img/5ak-6Senb1FzcbxpokldcG1CItMVehMznoNAomKJQGU=/h/1200?compression=0.75 | https://edge.curalate.com/v1/img/6N8ivG74vAmUghu4MbHrTb7nTjikvRUKwy3NqV8aOe8=/h/1200?compression=0.75 | https://edge.curalate.com/v1/img/7Q47X98QfRlIfAeCFpUclMkISbjtDBPtsw71O27c0ps=/sc/80x80?compression=0.75 |", Name: "Women's Short Sleeve V-Neck T-Shirt - Universal Thread�", Brand: "Universal Thread", Store Address: "Manhattan Herald Square,112 W 34th St, New York, NY 10120-0101", Store Telephone: "(646) 968-4739", Store Availability Status: "YES", Online Availability Status: "YES", Seller: "Manhattan Herald Square", Product ID: "78265229", Category: "Women's Clothing", Sub Category: "Pajamas & Loungewear", Rating Histogram: "N/A", Price: "$8.00", Product Rating: "4", Review: "413", Quantity: "1", Product Size: "M", Size Chart: "XS | S | M | L | XL |XXL|1X | 2X | 3X | 4X", Color: "Green", Variation Color: "White | Gold | Light Brown | Cream | Black | Rose | Navy | Light Green | Pink | Charcoal Gray | Heather Gray | Yellow | Red | Blue | Pink/White | Mint/Red | Green/Gray | Yellow/white | Blue/white | Coral | Lavender | Dark Pink | Red/white", Description: "Crisp and clean with endless versatility, the Relaxed-Fit Short-Sleeve V-neck T-Shirt from Universal Thread� is a must-have in your collection of everyday basics. This short-sleeve T-shirt lets you easily wear it with everything from dark-wash jeans and plaid ponte pants to camo joggers and printed mini skirts. 100% cotton fabric provides you with breathable comfort from day to night and season to season, and the relaxed silhouette makes for ease of layering as well as a figure-flattering fit. Style yourself a casual-chic work-to-weekend look by half tucking it into high-rise skinny jeans paired with ankle boots, and add a faux-leather or sherpa jacket for a cozy finishing touch.", Highlights: "Model wears size S/4 and is 5'9.5" |Universal Thread short-sleeve V-neck tee adds to your everyday staples |Solid, stripes, and tie-dye designs vary by color |100% cotton fabric and relaxed fit provide breathable comfort for year-round wear |Pairs well with a variety of bottoms for versatile styling options", Sizing: "Women", Material: "100% Cotton", Length: "At Waist", Features: "Short Sleeve, Pullover", Neckline: "V Neck", Garment sleeve style: "Basic Sleeve", Color Name: "Moss", Care and Cleaning: "Machine Wash & Tumble Dry", TCIN: "79646356", UPC: "191905640696", Item Number (DPCI): "013-00-2097", Origin: "Imported" }
Why Choose Web Screen Scraping?
Affordable
You won't have to worry about proxy difficulties, working with numerous crawlers, or providing assistance.
Browser-Based
Web Screen Scraping provides a Target.com data extractor that is simple to sign up for and utilize in any browser.
Customized Crawler
For your scraping needs, Web Screen Scraping provides simple options. We also offer personalized crawlers that scrape all product data and prices from Target.com in order to meet your business needs.
Dropbox Delivery
Web Screen Scraping gives you the option of automating data uploads to Dropbox.
Easily Usable
You do not need to download any software or fill in any data forms. It's simple to schedule and run your crawler to scrape product prices from Target.com using a point-and-click interface.
Contact Web Screen Scraping for all your requirements or ask for a free quote!!
0 notes
Text
How to Extract Product Data from H&M with Google Chrome?
Data You Can Scrape from H&M
Product’s Name
Pricing
Total Reviews
Product’s Description
Product’s Details

Requests
Google’s Chrome Browser: You would require to download the Chrome browser and the extension requires the Chrome 49+ version.
Web Scraping for Chrome Extension: Web Scraper extension could be downloaded from Chrome’s Web Store. Once downloaded the extension, you would get a spider icon included in the browser’s toolbar.
Finding the URLs
H&M helps you to search products that you could screen depending on the parameters including product types, sizes, colors, etc. The web scraper assists you to scrape data from H&M as per the requirements. You could choose the filters for data you require and copy corresponding URLs. In Web Scraper toolbars, click on the Sitemap option, choose the option named “Edit metadata’ to paste the new URLs (as per the filter) as Start URL.
For comprehensive steps about how to extract H&M data, you may watch the video given here or continue to read:
Importing H&M Scraper
After you install the extension, you can right-click anyplace on the page and go to the ‘Inspect’ option as well as Developer Tool console would pop up. Just click on the ‘Web Scraper’ tab and go to the ‘Create new sitemap’ option as well as click on the ‘Import sitemap’ button. Now paste JSON underneath into Sitemap’s JSON box.
Running the Scraper
To start extracting, just go to the Sitemap option and click the 'Scrape' alternative from the drop-down menu. Another window of Chrome will come, permitting the extension for scrolling and collecting data. Whenever the extraction is completed, the browser will be closed automatically and sends the notice.
Downloading the Data
For downloading the extracted data in a CSV file format, which you could open with Google Sheets or MS Excel, go to the Sitemap’s drop-down menu > Export as CSV > Download Now.
Disclaimer: All the codes given in our tutorials are for illustration as well as learning objectives only. So, we are not liable for how they are used as well as assume no liabilities for any harmful usage of source codes. The presence of these codes on our website does not indicate that we inspire scraping or scraping the websites given in the codes as well as supplement the tutorial. These tutorials only assist in illustrating the method of programming data scrapers for well-known internet sites. We are not indebted to offer any help for the codes, in case, you add your different questions in a comments sector, and we might occasionally address them.
#scrap data from H&M#importing H&M scraper#product data scraping#Web scraper#Data scraping services#Extract product data
1 note
·
View note
Text
Scrape H&M Product Data Using Google Chrome And Reveal Fashion Trends
Scrape H&M Product Data Using Google Chrome And Reveal Fashion Trends

Retail data scraping is a transformative process that involves the automated extraction of valuable information from various online retail sources. This technique utilizes web scraping tools and algorithms to collect data from e-commerce websites, including product details, prices, customer reviews, and inventory levels. Retailers and businesses leverage data scraping to gain insights into market trends, monitor competitor activities, optimize pricing strategies, and enhance overall decision-making processes. By harnessing the power of retail data scraping , organizations can stay agile in the highly competitive retail landscape, adapt to consumer preferences, and make data-driven decisions to drive success in the dynamic world of online commerce. Scrape retail data to unlock a wealth of insights, including product trends, pricing strategies, competitor analysis, and consumer behavior. This invaluable information collected using Ecommerce Data Scraping Services empowers businesses to make informed decisions, optimize operations, and stay ahead in the ever-evolving retail landscape.
About H&M

H&M, the Swedish fashion trailblazer born in 1947, isn't just a retailer; it's a curator of style accessible to all. With a knack for blending affordability and trendsetting designs, H&M transforms fashion into a democratic art form. Beyond clothing, it's a cultural force, collaborating with icons and embracing sustainability. H&M isn't merely a store; it's a global fashion ecosystem that democratizes chic, turning every shopping experience into a rendezvous with affordable elegance and the pulse of contemporary style. Scrape H&M retail data to gain valuable insights into the latest fashion trends, pricing strategies, and inventory dynamics, empowering businesses to make informed decisions in the ever-evolving world of retail.
List of Data Fields

Product Names
Product Codes
IDs
Description
Regular Prices
Sales Price
Discounts
Product Categories
Images
Stock Availability
Ratings
Reviews
Product Specifications
Shipping Information
Why Scrape H&M Product Data?

Scraping H&M product data can be valuable for various reasons, providing insights and opportunities for businesses and consumers. Here are seven unique points explaining why scraping H&M product data could be beneficial:
Market Analysis and Trends:
Insight into Fashion Trends: H&M product data scraping services allow businesses to analyze current fashion trends. Companies can align their strategies with consumer preferences by understanding which products are popular and in demand.
Competitor Intelligence:
Assessing Competitor Offerings: By scraping H&M product data, businesses can gain insights into the competitors’ products. This information is crucial for benchmarking, identifying gaps in the market, and making informed decisions on product development and pricing.
Pricing Strategy Optimization:
Dynamic Pricing: Monitoring H&M product prices through scraping enables businesses to implement dynamic pricing strategies. It involves adjusting prices based on real-time market conditions, competitor pricing, and other factors to remain competitive and maximize revenue.
Inventory Management:
Stock Levels and Availability: Retail data scraper helps monitor specific items' stock levels and availability. This information is valuable for optimizing inventory management, preventing stockouts, and ensuring the stocking of popular items.
Customer Behavior Analysis:
Understanding Customer Preferences:Analyzing scraped data using H&M product data scraping service can provide insights into customer preferences and behavior. Businesses can use this information to tailor marketing campaigns, enhance user experiences, and offer personalized recommendations.
E-commerce Automation:
Automated Product Updates: For businesses operating in the e-commerce space, scraping H&M product data allows for automating and updating product catalogs. It ensures that product information, including prices and availability, remains accurate and up-to-date.
Research and Development:
Product Development Insights: Scraped data can be valuable for research and development teams. Understanding which features, styles or materials are trending in H&M's product offerings can guide the development of new products that align with current market demands.
Steps to Scrape H&M Data Using Google Chrome
Open Google Chrome and navigate to https://www2.hm.com/en_in/index.html in a new tab.
Please select the desired gender and product category; for this example, let's choose men's hoodies and sweatshirts.
Apply additional filters such as product type, size, and color to refine your search to scrape H&M product data using Google Chrome.
Right-click anywhere on the page and choose "Inspect" to open the developer tools console.
Within the developer tools, click on the "Web Scraper" tab, then hit "Create new sitemap" and choose "Import sitemap."
Paste the JSON code into the sitemap JSON box and click the "Import sitemap" button.
To initiate the scraping process, navigate to the 'Sitemap' and select "Scrape" from the drop-down menu.
A new Chrome window will open, enabling the extension to scroll through the page and collect the data.
Once the scraping is complete, the browser will automatically close and send a notification.
Click the "Refresh" button to view the scraped data.
Download and export the data in CSV format for further use.
Role of Google chrome in Scraping H&M Fashion Data

Web scraping involves extracting data from websites, and Google Chrome can play a significant role when scraping H&M fashion data. Here's how Google Chrome is helpful in web scraping:
Browser Interaction: Google Chrome is the interface through which users interact with the target website. Users can open the website, navigate through pages, and apply filters to choose the data they want to scrape.
Developer Tools: Chrome's Developer Tools provide features, including the Inspect Element tool, which allows users to examine a webpage's HTML and CSS structure. It is crucial to understand data organization and identify the scraped elements.
Network Monitoring: The Network tab in Chrome's Developer Tools helps monitor browser network requests. This information can be crucial for understanding how data is loaded dynamically through AJAX requests or other mechanisms.
Console: The Console tab in Developer Tools allows users to run JavaScript code directly. It can be handy for testing and experimenting with code snippets before incorporating them into a web scraping script.
Browser Extensions: Google Chrome supports various extensions, including web scraping tools. In the provided instructions, the "e-commerce product data Scraper" tab indicates using a specific Chrome extension designed for web scraping tasks.
Headless Browsing: Chrome can run in headless mode for more advanced scraping. It means the browser runs without a graphical user interface, making it more efficient for automated scraping tasks.
Data Export: Chrome allows users to export data in various formats, such as CSV. After scraping data using web scraping tools or custom scripts, users can often save or export the collected data for further analysis or storage.
Conclusion: In summary, scraping H&M product data goes beyond simple data collection; it provides a strategic advantage for businesses by offering insights into market trends, competitor activities, and customer behavior. This information can inform decision-making processes related to pricing, inventory management, and product development, ultimately contributing to the overall success of a business in the retail and fashion industry.
Feel free to get in touch with iWeb Data Scraping for comprehensive information! Whether you seek web scraping service or mobile app data scraping, our team can assist you. Contact us today to explore your requirements and discover how our data scraping solutions can provide you with efficiency and reliability tailored to your unique needs.
Know More: https://www.iwebdatascraping.com/scrape-h-and-m-product-data-using-google-chrome.php
#ScrapeHandMProductDataUsingGoogleChrome#ExtractProductsDatafromHandMwithGoogleChrome#HandMScraper#scrapeHandMproductdata#HandMPriceandDataScraper#HandMProductDatascrapingservice#ProductsDatafromHandMwithGoogleChromeExtractor
0 notes
Link
What Is H&M?
H&M is a multinational Clothing-Retail & Accessories providing company that provides clothes for men, women, teenagers, and Children. H&M operates in more than 74 countries with 5000+ stores in the world with various company brands and have over 1,26,000 full-time employees. H&M is the world’s 2nd largest clothing retail company. H&M provides online shopping in 33 countries. The estimated revenue of H&M is $25.191 Billion.
List Of Data Fields
At RetailGators, we extract the required data fields given below. We extract H&M using Web Scraper Chrome Extraction.
Product Name
Price
Number of Reviews
Product Description
Product Details
Category of Cloths
Product Color
Product Size
Product Type
How We Scrape Data From H&M?
H&M allows you to search for different products that can be filtered as per factors like product price, product type, size, color, and many others. The Scraper will allow you to scrape data from the H&M as per your requirements. You need to select filters for your data you need to copy the equivalent URL.
H&M Data Scraping Tool helps you to collect structured data from H&M from the traffic using H&M normally. H&M scraper helps you to extract product data from H&M fashion online store. It allows you to scrape the whole site, particular categories, and products.
H&M Scraper
After adding an extension, you need to right-click anywhere on a page, or you need to inspect and develop tools and a popup will come up. Click on the tab name Web Scraper and you can go on to ‘Create New Sitemaps’ after that you need to click on the button and can easily import the sitemap option. RetailGators helps you to download the data into many different formats like CSV, JSON, and Excel.
Why RetailGators?
We have a professional team that helps you immediately if you have any problems while using H&M Data Scraping Services. Our eCommerce Data Scraping Services is capable, reliable, and offer you quick results without any error.
Scraping H&M Scraping Services helps you to save your time, money, and efforts. We can scrape data in a couple of hours, which might take a week or days if you do it yourself.
We are working on H&M Data Scraping Service Using Google Chrome that extract the required data.
Our expert team of H&M Data Scraping Services API knows how to convert unstructured data into structured data.
So if you are looking for the best H&M Product Data Scraping Services, then contact RetailGators for all your queries.
source code: https://www.retailgators.com/how-to-scrape-product-data-from-h-and-m-using-google-chrome.php
#scrape h&m product data#scrape data from h&m#extract h&m product data#ecommerce web scraping tool#ecommerce data scraping service
0 notes
Text
Autumnal nights spent curled up in a sweater, candles lit on the nightstand with a book in hand, and early brisk mornings, walking through the blanket of rich-colored leaves under the cold sunlight peeking through the bare branches. Endless hours spent at the library, looking out the window at the red, orange, green-fading-yellow trees and the children running around, while having a hot mug of tea wrapped in my hands have been some of the many ways I have been spending my autumn.
Each season has a special part about it that I love, but Autumn is definitely the most visually pleasing. It’s been the most peaceful time so far this year despite how much busier I have been. I’ve been able to explore new things, new ideas, thoughts, and found gems to share:
Venetia Berry – I only found Venetia on Instagram a few days ago, and her art captured me. It’s simple, and complicated. Her website contains more of her art if you want to look at it more.
Dead Poets Society – The first time I watched this was last year. I didn’t appreciate it enough and knew I hadn’t, which is why when I saw it on Netflix on the night of my birthday, I sat up until 11 p.m. on a Thursday night with my thai food and cake, knowing that I had school the next day. Robin Williams was a master in his craft, and the entire story that is told in this film, is tragically beautiful.
You Are A Badass – This has become my bible. I have been obsessing over this book to several of my friends. There is so much that this book talks about that I hold to myself. It is empowering, guiding, nurturing, and eye opening to all the excuses we make and silly, dumb, little things that we do that hold us back from thriving. Highly highly highly recommend picking up this book.
The Mothers – A secret romance leading to an unexpected problem, with lingering conflicts after its cease. The first book I couldn’t put down in a long time. Bennet’s writing style is capturing, and simplistic, and her story is obsessive.
Lolita – Such a controversial book; beautifully written, a great masterpiece by Vladimir Nabokov, but the topic is so messed up. But so good, but so wrong. I felt very conflicted while reading this, but loved it at the same time.
The Princess Saves Herself In This One – I spent an hour sitting on the cold shiny floor of Target reading Amanda Lovelace’s collection of poetry, and though I haven’t read it all, I know I am going to really enjoy it from the few that I have already read. Her story is heart-wrenching and capturing, and her words are beautiful.
Mom jeans – You know the jeans: high waisted, loose, and the most comfortable, fashion sensible piece of clothing ever. Finally got myself four pairs at the thrift shop and they are now one of my favorite things I own.
Red – Lipstick, nails, sweaters, pens, the leaves contrasting against the crisp, blue sky— this color has been haunting me everywhere. It has become a staple to my wardrobe, and is now “my” color. With my blue eyes, it brings a lot of depth to my face that I love, and all I have to put on is a dark or bright red lipstick, and I am ready to go.
A bold lip – Just pop on a bold liquid lipstick, some mascara, put the lipstick in my bag, and I am out the door, and looking good.
Natasha, Pierre, and the Great Comet of 1812 – This musical album has consumed my autumn, and it feels so nice to fall back in love with a Broadway musical after so long. I cannot express how many times I have played “Dust and Ashes” over and over and over. It’s stunning, and Josh Groban’s voice is a masterpiece; the cast is complex, diverse, and unique; the entire musical is beautiful. Highly, highly recommend taking a listen to this. My favorite songs are ‘Dust and Ashes’, ‘Charming’, ‘Letters’, and ‘No One Else’.
Dear Hank and John – A funny, random podcast where they answer strange, obscure questions with the best advice they can scrape together. Walking down red and orange leaf-pasted sidewalks, earbuds in while cars pass by in a faint hum, it’s been my favorite thing to listen to for entertainment.
Iron and Wine – In preparation to seeing him in concert next weekend, I have been listening to his new album, ‘Beast Epic’, almost everyday. It’s great for background music, or to play at night, or to just sit with earbuds in, laying on your bed, and closing your eyes, and just listening to.
Slowing down – Despite all of the things I have done this season (which is a lot more than normal), I have finally slowed down and been able to be productive and take in life at the same time. Take a break to breathe, look at the world around you, put time into your work, and suddenly you begin to truly admire each day you live through and all that you do. Life gets too loud at times, too congested, and too fast. Take time to sit and breathe and think, truly listen to people, look at what is around you. Just for a moment.
Playing ukulele – Since school started, I’ve been learning how to play the ukulele as a class, and I have fallen in love with being able to create music. So much so that I have already written a song, and found a ukulele to call mine.
Thrifting – This is one of my favorite things to do now because 1. it’s cheap and affordable and 2. it is amazing for the environment and 3. the clothing there is unique. Rarely will you find someone else with the same piece of clothing or accessory that you find at the thrift. There is a lot of really cool stuff that can completely change your wardrobe.
Walking – I live in a small college town, so everything is in walking distance. Biking is usually my go to, but as the leaves have been changing and the air has altered itself to a comfortable temperature, I have really enjoyed walking. Go to the library, to the quaint little coffee shop, to the park, or just around town for a break from schoolwork. There’s so much more you can take in, more time to think, to experience, to be present, and overall, I have enjoyed it a lot.
Deleting my social media apps – I deleted the apps off of my phone for a week in the middle of October after finding that I was constantly on my phone and I was stressed out because there was so much I needed or wanted to do, yet I would reach for my phone several times, wasting away hours and hours of my. And it was the best week so far. I got so much done, and was happier, and found a love in reading again that I had been missing.
Learning – Analyzing literature, and working everyday to advance my French, I’ve been on a learning kick. I have always tried to broaden my knowledge, learn new skill, and overall better myself. But specifically recently, I’ve been reading a lot, and studying a lot. I’ve been using apps like Duolingo and Tiny Cards, while watching Crash Course on Youtube, and those help a lot.
Library – Probably my favorite place now. I have a little nook beside a large window sill, and a plant behind me, surrounded by the large print books that I have been going to almost everyday after school to get my work done.
John and Hank Green – These two brothers, quickly became a favorite when I came across their YouTube channel, Vlogbrothers, during a time when I was really down, and disconnected. Their personalities, the way they think, and just who they are as people in general.
People – My family has grown into being “people” as I have gotten older. There isn’t the facade that used to always be there when I was younger, but now I see them as the people, the humans they are, the ones that make mistakes, that have history to them, that they are, or were, just like me. And it’s begun to spread to everyone I see. Everyone has a story, a history, things they smile at when recalling the memories, or the regrets that weigh down their chest, and I want to learn more about these people that congest our planet.
Just being, and living – Sometimes, you have to let go of fear; let go of all that you are paranoid— just gone— and be.
What went up on the blog?
Who are you?// 5 tips to get to know yourself better
Awaiting a dream (Self #3)
Perception
and more! Every week there is a new blog post on Sunday. What were some of your favorite things this month? Let me know what you want to read for future blog posts, and I hope you have an amazing day!
As always,
Autumn Favorites Autumnal nights spent curled up in a sweater, candles lit on the nightstand with a book in hand, and early brisk mornings, walking through the blanket of rich-colored leaves under the cold sunlight peeking through the bare branches.
0 notes
Text
Golf ball size guide 2019
Golf balls are diversely manufactured, some are extremely straightforward others are progressively intricate. To get a simple picture about the structure of the present golf balls, we might want to clarify the rudiments as pursues:
Dimples
In early occasions golfers made out that anomalies in the outside of the ball would give the ball a chance to fly higher and farer. Before long, directly after the Gutta Percha balls the supposed dimples were deliberately printed into the outside of each ball. Still these days, a large portion of the ball makers are trying out various shapes and quantities of dimples. The standard goes: the more dimples a ball has, the higher it goes. At that point, balls having such a large number of dimples, do fly excessively high and are inadequate with regards to separate. Most organizations have discovered their best number of dimples, for the most part they are positioning between 300-500 for each ball.
Specialized foundation:
Very much hit golf balls go around 200 km/h (120 m/h). Once off the tee, the ball begins to back off on the grounds that since air is adhering to it while flying, much the same as water adheres to a ball on the off chance that it falls into water. Since air is adhering to the surface it streams over, it bodes well that the less zone on a ball, the less staying and the less drag there is to survived.
It looks evident that a smooth covering on a cleaned circle would go more distant than a ball with a roughed, lemon cleaned surface. In any case, at the speed that golf balls go, it doesn't exactly work that way.
Envision to be a minor element, maybe the measure of a miniaturized scale shellfish - little enough to fit between grains of sand. Presently envision to ride on the outside of a golf ball in flight, or in the breeze burrow at the lab. You understand that directly at the outside of the ball, the air is still and it adheres to the plastic as the air atoms are hauled along like syrup running from the edge on a little pitcher at a prominent flapjack breakfast place. Yet, making tracks in an opposite direction from the surface, say similar to the thickness of three sheets of paper, we see that the air is going full speed. Here we're in, what we in golf science call, the "free stream" which moves at 200 km/h.
Be that as it may, the air gushing over a golf ball shapes a "limit layer" of moderately moderate moving air. It's particular. Directly at the surface, the air is trapped. A millimeter far from the surface, the air is going maxing out. In the middle of - in the limit layer - it's simply slurping along.
That moderate moving air in the limit layer is a wellspring of drag. It gives the air a chance to adhere to the surface and tumble behind the ball in wild whipping whirlpools. The vitality in the limit layer is lost vitality. The tumbling air behind the ball permits a huge (moderately enormous, it's only a golf ball) district of low strain to frame, making a halfway vacuum that would suck the ball back toward the tee. In this way, the more slender the limit layer, the less slurpy haul there is, and the sooner the air behind the ball can get back up to the "free stream" speed. The less drag, the more distant the ball will be driven.
Here's the place the dimples carry out their responsibility. Dimples make the particles in the layer tumble. They begin bothering against each other. The limit layer moves toward becoming "tempestuous." The atoms in the layer are never again simply sliding over the surface delicately shaking. Presently, they're rolling and bobbing and knocking each other along. At the point when the atoms are in a fierce limit layer, they're drawing nearer to the free-stream speed. There is to a lesser extent a contrast between the speed of the tumbling atoms and the speed of the ball.
Things being what they are, the wind stream in a tempestuous limit layer on a dimpled golf ball is more slender than a smooth or "laminar" stream on, state, a ping-pong ball. Limit layers are laminar or tempestuous, or some place in the middle. We state they're "experiencing significant change." Dimples make the progress fast - not a smooth change, a tempestuous one, ha! (A little liquid elements choke there...) When the layer is fierce and dainty, the ball loses less vitality to the free stream air. Furthermore, drag is lower. Isn't that strange? The dimples make the ball grow less drag.
Pressure
A few balls are demonstrated with a pressure of 80, 90 or 100 and so on. Initially it was utilized as an estimation for nature of 3-piece balls, where a long elastic was streched arround the center. The elastic had a lenght of approx. 20 meters and was streched at a factor of approx. multiple times of its unique, contingent upon the pressure that is needed. Along these lines it is injured arround the center. It was said that the more tightly the windings, the better the ball performed. This made a long-standing observation that pressure influences golf ball separation and execution.
Since golf ball innovation utilizes more up to date heat-safe strings with more current and better twisting hardware for three piece balls, golf ball pressure has moved toward becoming just a state of feel. Presently with the accessibility of the predictable nature of a two piece ball, pressure as an estimation of value is fairly outdated.
Definition: Today "pressure" in the golf ball industry identifies with an esteem communicated by a number in the range from 0 to 200 that is given a golf ball. This number characterizes the diversion that a golf ball experiences when exposed to a compressive burden. Pressure essentially measures how much the shape a golf ball changes under a consistent weight.
Estimation: All three-piece balls and some two-piece balls are estimated for pressure. A ball that doesn't pack is evaluated 200; a ball that avoids 2/10ths of an inch or more is appraised zero. Between those two boundaries, for each 1/1000ths of an inch that the ball packs, it drops one point from 200 and the pressure rating is then settled.
Most balls have pressure appraisals of either 80, 90, or 100; the lower the pressure, the gentler the vibe. Only one out of every odd ball checked 80, 90, or 100 is actually that rating. The genuine rating can fall generally inside 3-5 on either side of the sign. Any ball that drops out of this range is generally sold as range ball, or as X-outs.
Demonstrate: There have been a few distributed writings to demonstrate that golf ball pressure relates more to feel and your own superstition than its exhibition. The ends were, in the event that you take diverse evaluated golf balls which have a similar development, streamlined features, and spread material, and utilize a programmed golf swing machine, for example, the Iron Man, the yardage contrast between the balls hit were immaterial, under two yards.
Spread
The fronts of the present golf balls are made of various materials, for example, Balata, Surlyn, Zylin, or Elastomer. The principle challenge is to discover a spread, that gives a touchy, delicate inclination for the ball while sufficiently hard not to be cut still after a huge number of shots. In this manner, ball producers have exceptional machines shooting the ball against a divider with a speed of more that 250 km/h.
Balata: as a sort of normal elastic and the gentlest of all other spread sorts, Balata is less cut safe. Be that as it may, with every single other part of development being equivalent, a balata-secured ball will turn simpler and is favored by players who request most extreme feel and control. This implies more command over shots where the activity of the ball is basic.
Surlyn: Surlyn was the first and most strong spread material that upset the development of the golf ball when it was presented in the mid 80's. It is an exchange name for a gathering of thermoplastic pitches created by the Dupont Corporation. Most producers of sturdy spreads use either Surlyn or a comparative material mix. The accentuation today is to give both solidness and feel.
This strong spread offers preferable cut and scraped area obstruction over the balata spread. A Surlyn secured ball by and large feels harder than balata secured balls. The hardness of this spread material records for a lower turn rate.
The main factor for most golfers is financial aspects: a considerable lot of the present customary golfers have swung to sturdy secured balls essentially in light of the fact that they know an incidental miss-hit won't cut the spread.
Pieces
Picking a golf ball we for the most part discover data on the crate referencing to be a 1-, 2-, or 3-piece ball. In basic words, the clarification is as per the following:
1-piece balls
This ball is only from time to time utilized as a making a move. It is a decent ball for fledglings, shabby in its creation, and for the most part utilized on driving reaches. It is normally produced using a strong bit of Surlyn with dimples formed in. It is modest and entirely sturdy. On contact with the club face, the one-piece ball has a gentler vibe.
2-piece balls
A Two-Piece golf ball is utilized by most conventional ordinary golfers since it consolidates solidness with greatest separation. These balls are made with a solitary strong center (more often than not a hard plastic) encased in the ball's spread. The strong center is ordinarily a high-vitality acrylate or gum and is secured by an intense, cut-confirmation mixed spread that gives the two-piece ball more separation than some other ball. These "hard" balls are canvassed in either Surlyn, a claim to fame plastic exclusive to the Du Pont Company, or a comparable sort of material. The two piece is basically indestructible and with its high move separate, it is by a wide margin the most well known golf ball among normal golfers.
3-piece balls
Three-Piece golf balls or wound balls have either a strong elastic metal fluid focus (center) which is secured by numerous yards of flexible windings, over which is formed a front of solidly Surlyn, Surlyn like, or balata. Wound balls are milder and take more turn, permitting an able golfer more command over the ball's flight when hit. It ordinarily has a higher turn rate than a two piece ball and is increasingly controllable by great players. A Surlyn spread is a thermoplastic tar that is more enthusiastically than a balata and is significantly increasingly tough. A balata-secured, fluid focused, three piece ball takes more time to make than a two-piece ball. The injury development over a fluid focus, joined with a delicate manufactured balata spread, creates an extremely high turn rate, p
0 notes
Text
AACE Armored Amphibious Combat Earthmover
AACE Armored Amphibious Combat Earthmover
AACE Armored Amphibious Combat Earthmover is an amphibious, armored, tracked, combat earthmover; designed for the preparation of river banks during river crossing missions. It is capable of performing bulldozing, rough grading, excavating, hauling, and scraping operations. As compared to standard heavy-duty vehicles; AACE has the capability to take in ballast from the soil ground to its ballast…
View On WordPress
0 notes
Text
How to Extract eBay Data for Original Comic Art Sales Information?

Data Fields to Be Scrapped
There is an example shown of the artwork drawn by hand in pencil by some artist and then another artist inks the drawing over them. Typically, 11 × 17-inch panels are used. The vitality of the drawing style, as well as the obvious skill, appeal to everyone.
Get two panels of original art for inside pages from Spiderman comics from the 1980s a few years ago, around 2010. You can pay perhaps $200 or $300 for them and made slightly more than twice that much when you sell them a year later.
Nonetheless, if you are interested in purchasing several pieces in the $200 level right now and wanted to get additional information before doing so.
Below written is the full code with the main output in two csv files.
The leading 800 listings of original comic art from Marvel comics in the form of internal pages, covers, or splash pages are ordered by price in the first csv file. The following fields are scraped from eBay in the csv:
the title (which usually includes a 20-word description of the item, character, type of page)
Price
Link to the item's full eBay sales page complete list of all figures in the artwork *just after first eBay search, the software cycles through the page numbers of new matches at the bottom. eBay flags the application as a bot and prevents it from scraping pages with numbers greater than four. This is fine because it only includes goods that are normally sold for less than $75, and nearly none of them are original comic art – they are largely copies or fan art.
The second file format is doing the same thing, but for things that have previously been sold, using the same search criteria. Because it requires Selenium.
If you execute Selenium more than two or three times in an hour, eBay will disable it and you will have to manually download the HTML of sold comic art.
Expected Result
You can check the result by executing the code once a day and looking through the csv file for mostly lesser-known characters of $100-$300 US dollar range currently for the sale.
Tools that are used: Python, requests, BeautifulSoup, pandas
Here are the below steps that we will follow:
We will scrape the following product
https://ebay.to/3qaWDIw
Using the “original comic art” as the search string
only cover, interior pages, or splash pages
only comic art from Marvel or DC
comics above the price of $50
sorted by price + shipping and highest to lowest
200 results per page
We'll find a comprehensive of available original comic art based on your search parameters. We'll retrieve the title / brief explanation of the listing (as a single string), the page URL of the real listing, and the price for each listing.
We'll get the main comic book character's name in one field and the identities of all the characters in the image in a second field for each listing.
We'll make a CSV file using an eBay product data scraper in the following format: a title, a price, a link, a character, and a character with several characters.
Installing all the Packages for the Project
!pip install requests --upgrade --quiet !pip install bs4 --upgrade --quiet !pip install pandas --upgrade --quiet !pip install datetime --upgrade --quiet !pip install selenium --upgrade --quiet !pip install selenium_stealth --upgrade --quiet
Initially use the time package so that you can keep the record of the program’s progress and slowly use the date and time in the csv file name
import time from datetime import date from datetime import datetime now = datetime.now() today = date.today() today = today.strftime("%b-%d-%Y") date_time = now.strftime("%H-%M-%S") today = today + "-" + date_time print("date and time:", today) date and time: Jul-17-2021-15-14-55
Create a Function to Print the Data and Time
def update_datetime(): global now global today global date_time now = datetime.now() today = date.today() today = today.strftime("%b-%d-%Y") date_time = now.strftime("%H-%M-%S") today = today + "-" + date_time print("date and time:", today)
Next Scrape the search URL
To download the page, use the requests package.
Employ Beautiful Soup (BS4) to look for appropriate HTML tags, parse them.
Transform the artwork information to a Pandas dataframe.
import requests from bs4 import BeautifulSoup # original comic art, marvel or dc only, buy it now, over 50, interior splash or cover, sorted by price high to low orig_comicart_marv_dc_50plus_200perpage = 'https://www.ebay.com/sch/i.html?_dcat=3984&_fsrp=1&Type=Cover%7CInterior%2520Page%7CSplash%2520Page&_from=R40&_nkw=original+comic+art&_sacat=0&Publisher=Marvel%2520Comics%7CDC%2520Comics%7CUltimate%2520Marvel%7CMarvel%2520Age%7CMarvel%2520Adventures%7CMarvel&LH_BIN=1&_udlo=50&_sop=16&_ipg=200' orig_comicart_marv_dc_50plus_200perpage_sold = 'https://www.ebay.com/sch/i.html?_fsrp=1&_from=R40&_sacat=0&LH_Sold=1&_mPrRngCbx=1&_udlo=50&_udhi&LH_BIN=1&_samilow&_samihi&_sadis=15&_stpos=10002&_sop=16&_dmd=1&_ipg=200&_fosrp=1&Type=Cover%7CInterior%2520Page%7CSplash%2520Page&LH_Complete=1&_nkw=original%20comic%20art&_dcat=3984&rt=nc&Publisher=DC%2520Comics%7CMarvel%7CMarvel%2520Comics' search_url = orig_comicart_marv_dc_50plus_200perpage # there is a way to use headers in this function call to change the # user agent so the site thinks the request is coming from # different computers with different broswers but I could not get this working # response = requests.get(url, headers=headers) if (response.status_code != 200): raise Exception('Failed to load page {}'.format(url)) page_contents = response.text doc = BeautifulSoup(page_contents, 'html.parser')
Unless there is an error, the response function will return 200. If this is the case, display the error code; otherwise, continue. doc is a BeautifulSoup (BS4) object that makes searching for HTML tags and navigating the Document Object Model a breeze (DOM)
Now Save the HTML Files
# first use the date and time in the file name filename = 'comic_art_marvel_dc-' + today + '.html' with open(filename, 'w') as f: f.write(page_contents)
We can use h3 tags with the class's-item title' to acquire the listing's title/description.
title_class = 's-item__title' title_tags = doc.find_all('h3', {'class': title_class})
This locates all of the h3 tags in the BS4 documentation.
# make a list for all the titles title_list = []
loop through the tags and obtain only the contents of each one
for i in range(len(title_tags)): # make sure there are contents first if (title_tags[i].contents): title_contents = title_tags[i].contents[0] title_list.append(title_contents) len(title_list) 202 print(title_list[:5]) ['WHAT IF ASTONISHING X-MEN #1 ORIGINAL J. SCOTT CAMPBELL COMIC COVER ART MARVEL', 'CHAMBER OF DARKNESS #7 COVER ART (VERY FIRST BERNIE WRIGHTSON MARVEL COVER) 1970', 'MANEELY, JOE - WILD WESTERN #46 GOLDEN AGE MARVEL COMICS COVER (LARGE ART) 1955', 'Superman vs Captain Marvel Double page splash art by Rich Buckler DC 1978 YOWZA!', 'SIMON BISLEY 1990 DOOM PATROL #39 ORIGINAL COMIC COVER ART PAINTING DC COMICS'] since the price is in the same area of the html page as the title, let's use the findNext function. this time we will search for a 'span' element with class = 's-item__price'. also, when I tried to run separate functions to find the title, and then the price, there were sometimes duplicate title tags -- to the length of the lists would not match. I would get a title list with 202 items and a price list 200 items -- so these could not be joined in a dataframe. Also, I imagine using findNext() and findPrevious() might make the whole search process a little faster.
We'll use the findNext function because the price is in the same section of the html page as the title. We'll look for a'span' element with the class's-item price' this time. Furthermore, whenever I tried to execute separate functions to get the title and then the price, there were occasionally duplicate page titles - the lengths of the lists didn't match. You would get a 202-item title list and a 200-item price list, which couldn't be combined in a data frame.
In addition, You can use findNext() and findPrevious()that will speed up the entire search process.
price_class = 's-item__price' price_list = [] for i in range(len(title_tags)): # make sure there are contents first if (title_tags[i].contents): title_contents = title_tags[i].contents[0] title_list.append(title_contents) price = title_tags[i].findNext('span', {'class': price_class}) if(i==1): print(price)
This displays the price information during the last item listed on the first search page, out of a total of 200.
print(price.contents) ['$60.00']
Now you need to check if you are getting a string and not a tag, and if so Strip the Dollar sign
from __future__ import division, unicode_literals import codecs from re import sub if (isinstance(price_string, str)): price_string = sub(r'[^\d.]', '', price_string) else: price_string = price.contents[0].contents[0] price_string = sub(r'[^\d.]', '', price_string) print(price_string) 60.00
Converting the Price into a Floating-Point Decimal
price_num = float(price_string) print(price_num) 60.0
Place it All together in a Loop and Add all the Prices to a List
for i in range(len(title_tags)): if (title_tags[i].contents): title_contents = title_tags[i].contents[0] title_list.append(title_contents) price = title_tags[i].findNext('span', {'class': price_class}) if price.contents: price_string = price.contents[0] if (isinstance(price_string, str)): price_string = sub(r'[^\d.]', '', price_string) else: price_string = price.contents[0].contents[0] price_string = sub(r'[^\d.]', '', price_string) price_num = float(price_string) price_list.append(price_num) print(len(price_list)) 202 print(price_list[:5]) [50000.0, 45000.0, 18000.0, 16000.0, 14999.99]
now find an anchor tag with a reference and add the links to each distinct art listing
item_page_link = title_tags[i].findPrevious('a', href=True) link_list = []
Clearing the Other Lists
title_list.clear() price_list.clear() for i in range(len(title_tags)): if (title_tags[i].contents): title_contents = title_tags[i].contents[0] title_list.append(title_contents) price = title_tags[i].findNext('span', {'class': price_class}) if price.contents: price_string = price.contents[0] if (isinstance(price_string, str)): price_string = sub(r'[^\d.]', '', price_string) else: price_string = price.contents[0].contents[0] price_string = sub(r'[^\d.]', '', price_string) price_num = float(price_string) price_list.append(price_num) item_page_link = title_tags[i].findPrevious('a', href=True) # {'class': 's-item__link'}) if item_page_link.text: href_text = item_page_link['href'] link_list.append(item_page_link['href']) len(link_list) 202 print(link_list[:5])
Creating a DataFrame using the Dictionary
import pandas as pd title_price_link_df = pd.DataFrame(title_and_price_dict) len(title_price_link_df) 202 print(title_price_link_df[:5]) title ... link 0 WHAT IF ASTONISHING X-MEN #1 ORIGINAL J. SCOTT... ... https://www.ebay.com/itm/123753951902?hash=ite... 1 CHAMBER OF DARKNESS #7 COVER ART (VERY FIRST B... ... https://www.ebay.com/itm/312520261257?hash=ite... 2 MANEELY, JOE - WILD WESTERN #46 GOLDEN AGE MAR... ... https://www.ebay.com/itm/312525381131?hash=ite... 3 Superman vs Captain Marvel Double page splash ... ... https://www.ebay.com/itm/233849382971?hash=ite... 4 SIMON BISLEY 1990 DOOM PATROL #39 ORIGINAL COM... ... https://www.ebay.com/itm/153609370179?hash=ite... [5 rows x 3 columns]
We're simply interested in the top six pages of results produced by our search address for now. We would potentially obtain 1200 listings ordered by price if the URL returned 200 listings per page. Unfortunately, eBay stops processing requests after the fourth page, resulting in 800 listings. Given the current traffic on eBay, this should be enough to get all products over $75. The listings below this amount are almost entirely made up of fan art rather than actual comic art.
So, the quick and simple method is to check for the pages in the lower-left corner and click on each one to receive the connections to that page.
links_with_pgn_text = [] for a in doc.find_all('a', href=True): if a.text: href_text = a['href'] if (href_text.find('pgn=') != -1): links_with_pgn_text.append(a['href']) len(links_with_pgn_text) 7 print(links_with_pgn_text[:3])
Converting this into Function
def build_pagelink_list(url): response = requests.get(url) if (response.status_code != 200): raise Exception('Failed to load page {}'.format(url)) page_contents = response.text doc = BeautifulSoup(page_contents, 'html.parser') for a in doc.find_all('a', href=True): if a.text: href_text = a['href'] if (href_text.find('pgn=') != -1): links_with_pgn_text.append(a['href']) #below gets run if there is only 1 page of listings if (len(links_with_pgn_text) < 1): links_with_pgn_text.append(url) links_with_pgn_text.clear() build_pagelink_list(orig_comicart_marv_dc_50plus_200perpage) len(links_with_pgn_text) 7 print(links_with_pgn_text)
Extracting the Old Items
Now we'll scrape the internet for auctioned listings and prices. The long-term aim is to be able to detect products listed for sale and compare their pricing to those of recently sold items to determine whether current listings are reasonably priced or underpriced and worth considering purchasing.
This second link only returns results for things that have already been sold, according to eBay. However, because this search yields fewer than 200 results, we'll have to manually download the file for this notebook. This procedure, however, is automated using Selenium, and the code for it can be found below.
orig_comicart_marv_dc_50plus_200perpage_sold = 'https://www.ebay.com/sch/i.html?_fsrp=1&_from=R40&_sacat=0&LH_Sold=1&_mPrRngCbx=1&_udlo=50&_udhi&LH_BIN=1&_samilow&_samihi&_sadis=15&_stpos=10002&_sop=16&_dmd=1&_ipg=200&_fosrp=1&Type=Cover%7CInterior%2520Page%7CSplash%2520Page&LH_Complete=1&_nkw=original%20
Select File->Save Page as webpage HTML only from Chrome if you need to save the page manually.
"sold listings.html" is the name of the file.
!apt update !apt install chromium-chromedriver --quiet from selenium import webdriver from selenium_stealth import stealth Hit:1 https://cloud.r-project.org/bin/linux/ubuntu bionic-cran40/ InRelease Ign:2 https://developer.download.nvidia.com/compute/cuda/repos/ubuntu1804/x86_64 InRelease Hit:3 http://security.ubuntu.com/ubuntu bionic-security InRelease Ign:4 https://developer.download.nvidia.com/compute/machine-learning/repos/ubuntu1804/x86_64 InRelease Hit:5 https://developer.download.nvidia.com/compute/cuda/repos/ubuntu1804/x86_64 Release Hit:6 http://ppa.launchpad.net/c2d4u.team/c2d4u4.0+/ubuntu bionic InRelease Hit:7 https://developer.download.nvidia.com/compute/machine-learning/repos/ubuntu1804/x86_64 Release Hit:8 http://archive.ubuntu.com/ubuntu bionic InRelease Hit:9 http://archive.ubuntu.com/ubuntu bionic-updates InRelease Hit:10 http://ppa.launchpad.net/cran/libgit2/ubuntu bionic InRelease Hit:11 http://archive.ubuntu.com/ubuntu bionic-backports InRelease Hit:12 http://ppa.launchpad.net/deadsnakes/ppa/ubuntu bionic InRelease Hit:14 http://ppa.launchpad.net/graphics-drivers/ppa/ubuntu bionic InRelease Reading package lists... Done Building dependency tree Reading state information... Done 41 packages can be upgraded. Run 'apt list --upgradable' to see them. Reading package lists... Building dependency tree... Reading state information... chromium-chromedriver is already the newest version (91.0.4472.101-0ubuntu0.18.04.1). 0 upgraded, 0 newly installed, 0 to remove and 41 not upgraded. def selenium_run(url): options = webdriver.ChromeOptions() options.add_argument('--headless') options.add_argument('--no-sandbox') options.add_argument('--disable-dev-shm-usage') options.add_argument("start-maximized") options.add_experimental_option("excludeSwitches", ["enable-automation"]) options.add_experimental_option('useAutomationExtension', False) # open it, go to a website, and get results driver = webdriver.Chrome('chromedriver',options=options) # uncomment below and change paths if running locally (and comment the line above) #PATH = '/Users/jmartin/Downloads/chromedriver' #driver = webdriver.Chrome(options=options, executable_path=r"/Users/jmartin/Downloads/chromedriver") stealth( driver, user_agent = 'Mozilla/5.0 (Windows NT 10.0; Win64; x64) AppleWebKit/537.36 (KHTML, like Gecko) Chrome/83.0.4103.53 Safari/537.36', languages = "en", vendor = "Google Inc.", platform = "Win32", webgl_vendor = "Intel Inc.", renderer = "Intel Iris OpenGL Engine", fix_hairline = False, run_on_insecure_origins = False ) driver.delete_all_cookies() driver.get(url) update_datetime() #html_file_name = "sold_page_source-" + today + ".html" html_file_name = "sold_listings.html" with open(html_file_name, "w") as f: f.write(driver.page_source) return html_file_name fname = selenium_run(orig_comicart_marv_dc_50plus_200perpage_sold) date and time: Jul-17-2021-15-17-25 print(fname) sold_listings.html with open(fname) as fp: doc = BeautifulSoup(fp, 'html.parser') def selenium_run(url): options = webdriver.ChromeOptions() options.add_argument('--headless') options.add_argument('--no-sandbox') options.add_argument('--disable-dev-shm-usage') options.add_argument("start-maximized") options.add_experimental_option("excludeSwitches", ["enable-automation"]) options.add_experimental_option('useAutomationExtension', False) # open it, go to a website, and get results driver = webdriver.Chrome('chromedriver',options=options) # uncomment below and change paths if running locally (and comment the line above) #PATH = '/Users/jmartin/Downloads/chromedriver' #driver = webdriver.Chrome(options=options, executable_path=r"/Users/jmartin/Downloads/chromedriver") stealth( driver, user_agent = 'Mozilla/5.0 (Windows NT 10.0; Win64; x64) AppleWebKit/537.36 (KHTML, like Gecko) Chrome/83.0.4103.53 Safari/537.36', languages = "en", vendor = "Google Inc.", platform = "Win32", webgl_vendor = "Intel Inc.", renderer = "Intel Iris OpenGL Engine", fix_hairline = False, run_on_insecure_origins = False ) driver.delete_all_cookies() driver.get(url) update_datetime() #html_file_name = "sold_page_source-" + today + ".html" html_file_name = "sold_listings.html" with open(html_file_name, "w") as f: f.write(driver.page_source) return html_file_name fname = selenium_run(orig_comicart_marv_dc_50plus_200perpage_sold) date and time: Sep-27-2021-13-19-13 print(fname) sold_listings.html with open(fname) as fp: doc = BeautifulSoup(fp, 'html.parser')
For the sold products page, the classes for the title, link, and price tags are a little different.
title_class = 'lvtitle' price_class = 'bold bidsold' link_class = 'vip'
obtain a session URL, and then remove cookies from the session to avoid website blocking
s = requests.session()
Place it all into one function which will scrape for current or sold listings based on the function arguments.
def scrape_titles_and_prices(url, document): s.cookies.clear() update_datetime() if document: using_local_doc=True doc = document title_class = 'lvtitle' price_class = 'bold bidsold' link_class = 'vip' else: print('processing a link: ', url) using_local_doc = False response = requests.get(url) if (response.status_code != 200): raise Exception('Failed to load page {}'.format(url)) page_contents = response.text doc = BeautifulSoup(page_contents, 'html.parser') filename = 'comic_art_marvel_dc' + today + '.html' if searching_sold: sold_html_file = filename with open(filename, 'w') as f: f.write(page_contents) title_class = 's-item__title' price_class = 's-item__price' link_class = 's-item__link' title_tags = doc.find_all('h3', {'class': title_class}) title_list = [] price_list = [] link_list = [] for i in range(len(title_tags)): if (title_tags[i].contents): if using_local_doc: title_contents = title_tags[i].contents[0].contents[0] else: title_contents = title_tags[i].contents[0] title_list.append(title_contents) price = title_tags[i].findNext('span', {'class': price_class}) if price.contents: if len(price.contents)>1 and using_local_doc: price_string = price.contents[1].contents[0] else: price_string = price.contents[0] if (isinstance(price_string, str)): price_string = sub(r'[^\d.]', '', price_string) else: price_string = price.contents[0].contents[0] price_string = sub(r'[^\d.]', '', price_string) price_num = float(price_string) price_list.append(price_num) item_page_link = title_tags[i].findPrevious('a', href=True) # {'class': 's-item__link'}) if item_page_link.text: href_text = item_page_link['href'] link_list.append(item_page_link['href']) title_and_price_dict = { 'title': title_list, 'price': price_list, 'link': link_list } title_price_link_df = pd.DataFrame(title_and_price_dict) # returns a data frame return title_price_link_df result = scrape_titles_and_prices("", doc) date and time: Jul-17-2021-15-18-43 print(result[:10]) Empty DataFrame Columns: [title, price, link] Index: []
Exporting the Result to a .csv File
You might get an issue using.to csv in future tests after starting this project, therefore You will have to reduce the version of pandas to get this to work.
!pip uninstall pandas !pip install pandas==1.1.5 Found existing installation: pandas 1.3.3 Uninstalling pandas-1.3.3: Would remove: /usr/local/lib/python3.7/dist-packages/pandas-1.3.3.dist-info/* /usr/local/lib/python3.7/dist-packages/pandas/* Proceed (y/n)? y Successfully uninstalled pandas-1.3.3 Collecting pandas==1.1.5 Downloading pandas-1.1.5-cp37-cp37m-manylinux1_x86_64.whl (9.5 MB) |████████████████████████████████| 9.5 MB 7.3 MB/s Requirement already satisfied: numpy>=1.15.4 in /usr/local/lib/python3.7/dist-packages (from pandas==1.1.5) (1.19.5) Requirement already satisfied: python-dateutil>=2.7.3 in /usr/local/lib/python3.7/dist-packages (from pandas==1.1.5) (2.8.2) Requirement already satisfied: pytz>=2017.2 in /usr/local/lib/python3.7/dist-packages (from pandas==1.1.5) (2018.9) Requirement already satisfied: six>=1.5 in /usr/local/lib/python3.7/dist-packages (from python-dateutil>=2.7.3->pandas==1.1.5) (1.15.0) Installing collected packages: pandas ERROR: pip's dependency resolver does not currently take into account all the packages that are installed. This behaviour is the source of the following dependency conflicts. google-colab 1.0.0 requires requests~=2.23.0, but you have requests 2.26.0 which is incompatible. Successfully installed pandas-1.1.5 update_datetime() fname = "origcomicart" + "-sold-" + today + ".csv" result.to_csv(fname, index=None) print(fname) date and time: Sep-27-2021-13-25-01 origcomicart-sold-Sep-27-2021-13-25-01.csv
Cycle Through all the Links in the CSV File
Go into each link and visit the individual listing page to collect the identity of the character, as well as all characters on the art, now that we have a.csv file with all the sold listings (the same goes for a csv file with all the current listings).
import csv def indiv_page_link_cycler(csv_name): with open(csv_name, newline='') as f: reader = csv.reader(f) data = list(reader) # go through each link and add character to each list # skip header row for i in range(1, len(data)): if(i%50==0): update_datetime() print(i,' :links processed') link = data[i][2] response = requests.get(link) if (response.status_code != 200): raise Exception('Failed to load page {}'.format(url)) page_contents = response.text doc = BeautifulSoup(page_contents, 'html.parser') searched_word = 'Character' selection_class = 'attrLabels' character_tags = doc.find_all('td', {'class': selection_class}) for j in range(len(character_tags)): if (character_tags[j].contents): fullstring = character_tags[j].contents[0] if ("Character" or "character") in fullstring: character = character_tags[j].findNext('span') data[i].append(character.text) data[0].append('characters') data[0].append('multi-characters') fname = csv_name[:-4] fname = fname + "_chars.csv" with open(fname, 'w') as file: writer = csv.writer(file) writer.writerows(data)
Copy and paste the csv format files name from the previous output
indiv_page_link_cycler(fname) date and time: Sep-27-2021-13-26-48 50 :links processed date and time: Sep-27-2021-13-27-27 100 :links processed date and time: Sep-27-2021-13-28-08 150 :links processed date and time: Sep-27-2021-13-28-47 200 :links processed
Each entry is added with the identities of the characters in a new csv file. The file is identical to the one above, with the addition of "_chars" at the end.
!pip install requests --upgrade --quiet !pip install bs4 --upgrade --quiet !pip install pandas --upgrade --quiet !pip install datetime --upgrade --quiet !pip install selenium --upgrade --quiet !pip install selenium_stealth --upgrade --quiet !apt update !apt install chromium-chromedriver
Get:1 http://security.ubuntu.com/ubuntu bionic-security InRelease [88.7 kB] Get:2 https://cloud.r-project.org/bin/linux/ubuntu bionic-cran40/ InRelease [3,626 B] Ign:3 https://developer.download.nvidia.com/compute/cuda/repos/ubuntu1804/x86_64 InRelease Get:4 http://ppa.launchpad.net/c2d4u.team/c2d4u4.0+/ubuntu bionic InRelease [15.9 kB] Ign:5 https://developer.download.nvidia.com/compute/machine-learning/repos/ubuntu1804/x86_64 InRelease Hit:6 https://developer.download.nvidia.com/compute/cuda/repos/ubuntu1804/x86_64 Release Hit:7 https://developer.download.nvidia.com/compute/machine-learning/repos/ubuntu1804/x86_64 Release Hit:8 http://archive.ubuntu.com/ubuntu bionic InRelease Get:9 http://archive.ubuntu.com/ubuntu bionic-updates InRelease [88.7 kB] Hit:10 http://ppa.launchpad.net/cran/libgit2/ubuntu bionic InRelease Get:11 http://security.ubuntu.com/ubuntu bionic-security/main amd64 Packages [2,221 kB] Hit:12 http://ppa.launchpad.net/deadsnakes/ppa/ubuntu bionic InRelease Get:13 http://archive.ubuntu.com/ubuntu bionic-backports InRelease [74.6 kB] Hit:14 http://ppa.launchpad.net/graphics-drivers/ppa/ubuntu bionic InRelease Get:15 http://security.ubuntu.com/ubuntu bionic-security/universe amd64 Packages [1,418 kB] Get:18 http://ppa.launchpad.net/c2d4u.team/c2d4u4.0+/ubuntu bionic/main Sources [1,780 kB] Get:19 http://archive.ubuntu.com/ubuntu bionic-updates/main amd64 Packages [2,658 kB] Get:20 http://ppa.launchpad.net/c2d4u.team/c2d4u4.0+/ubuntu bionic/main amd64 Packages [911 kB] Get:21 http://archive.ubuntu.com/ubuntu bionic-updates/universe amd64 Packages [2,188 kB] Fetched 11.4 MB in 3s (3,327 kB/s) Reading package lists... Done Building dependency tree Reading state information... Done 41 packages can be upgraded. Run 'apt list --upgradable' to see them. Reading package lists... Done Building dependency tree Reading state information... Done The following additional packages will be installed: chromium-browser chromium-browser-l10n chromium-codecs-ffmpeg-extra Suggested packages: webaccounts-chromium-extension unity-chromium-extension The following NEW packages will be installed: chromium-browser chromium-browser-l10n chromium-chromedriver chromium-codecs-ffmpeg-extra 0 upgraded, 4 newly installed, 0 to remove and 41 not upgraded. Need to get 86.0 MB of archives. After this operation, 298 MB of additional disk space will be used. Get:1 http://archive.ubuntu.com/ubuntu bionic-updates/universe amd64 chromium-codecs-ffmpeg-extra amd64 91.0.4472.101-0ubuntu0.18.04.1 [1,124 kB] Get:2 http://archive.ubuntu.com/ubuntu bionic-updates/universe amd64 chromium-browser amd64 91.0.4472.101-0ubuntu0.18.04.1 [76.1 MB] Get:3 http://archive.ubuntu.com/ubuntu bionic-updates/universe amd64 chromium-browser-l10n all 91.0.4472.101-0ubuntu0.18.04.1 [3,937 kB] Get:4 http://archive.ubuntu.com/ubuntu bionic-updates/universe amd64 chromium-chromedriver amd64 91.0.4472.101-0ubuntu0.18.04.1 [4,837 kB] Fetched 86.0 MB in 4s (19.2 MB/s) Selecting previously unselected package chromium-codecs-ffmpeg-extra. (Reading database ... 160837 files and directories currently installed.) Preparing to unpack .../chromium-codecs-ffmpeg-extra_91.0.4472.101-0ubuntu0.18.04.1_amd64.deb ... Unpacking chromium-codecs-ffmpeg-extra (91.0.4472.101-0ubuntu0.18.04.1) ... Selecting previously unselected package chromium-browser. Preparing to unpack .../chromium-browser_91.0.4472.101-0ubuntu0.18.04.1_amd64.deb ... Unpacking chromium-browser (91.0.4472.101-0ubuntu0.18.04.1) ... Selecting previously unselected package chromium-browser-l10n. Preparing to unpack .../chromium-browser-l10n_91.0.4472.101-0ubuntu0.18.04.1_all.deb ... Unpacking chromium-browser-l10n (91.0.4472.101-0ubuntu0.18.04.1) ... Selecting previously unselected package chromium-chromedriver. Preparing to unpack .../chromium-chromedriver_91.0.4472.101-0ubuntu0.18.04.1_amd64.deb ... Unpacking chromium-chromedriver (91.0.4472.101-0ubuntu0.18.04.1) ... Setting up chromium-codecs-ffmpeg-extra (91.0.4472.101-0ubuntu0.18.04.1) ... Setting up chromium-browser (91.0.4472.101-0ubuntu0.18.04.1) ... update-alternatives: using /usr/bin/chromium-browser to provide /usr/bin/x-www-browser (x-www-browser) in auto mode update-alternatives: using /usr/bin/chromium-browser to provide /usr/bin/gnome-www-browser (gnome-www-browser) in auto mode Setting up chromium-chromedriver (91.0.4472.101-0ubuntu0.18.04.1) ... Setting up chromium-browser-l10n (91.0.4472.101-0ubuntu0.18.04.1) ... Processing triggers for man-db (2.8.3-2ubuntu0.1) ... Processing triggers for hicolor-icon-theme (0.17-2) ... Processing triggers for mime-support (3.60ubuntu1) ... Processing triggers for libc-bin (2.27-3ubuntu1.2) ... /sbin/ldconfig.real: /usr/local/lib/python3.7/dist-packages/ideep4py/lib/libmkldnn.so.0 is not a symbolic link from __future__ import division, unicode_literals import requests from bs4 import BeautifulSoup from re import sub from decimal import Decimal import pandas as pd import requests import random import time import os import csv from datetime import date from datetime import datetime import codecs from selenium import webdriver from selenium_stealth import stealth import time import random s = requests.session() s.cookies.clear() now = datetime.now() today = date.today() today = today.strftime("%b-%d-%Y") date_time = now.strftime("%H-%M-%S") today = today + "-" + date_time def update_datetime(): global now global today global date_time now = datetime.now() today = date.today() today = today.strftime("%b-%d-%Y") date_time = now.strftime("%H-%M-%S") today = today + "-" + date_time print("date and time:", today) html_doc = """ <html><head><title>place holder</title></head> """ s.cookies.clear() # this just initializes the beautiful soup doc as a global variable doc = BeautifulSoup(html_doc, 'html.parser') def scrape_titles_and_prices(url, document): s.cookies.clear() update_datetime() if document: using_local_doc=True doc = document title_class = 'lvtitle' price_class = 'bold bidsold' link_class = 'vip' else: print('processing a link: ', url) using_local_doc = False response = requests.get(url) if (response.status_code != 200): raise Exception('Failed to load page {}'.format(url)) page_contents = response.text doc = BeautifulSoup(page_contents, 'html.parser') filename = 'comic_art_marvel_dc' + today + '.html' if searching_sold: sold_html_file = filename with open(filename, 'w') as f: f.write(page_contents) title_class = 's-item__title' price_class = 's-item__price' link_class = 's-item__link' title_tags = doc.find_all('h3', {'class': title_class}) title_list = [] price_list = [] link_list = [] for i in range(len(title_tags)): if (title_tags[i].contents): if using_local_doc: title_contents = title_tags[i].contents[0].contents[0] else: title_contents = title_tags[i].contents[0] title_list.append(title_contents) price = title_tags[i].findNext('span', {'class': price_class}) if price.contents: if len(price.contents)>1 and using_local_doc: price_string = price.contents[1].contents[0] else: price_string = price.contents[0] if (isinstance(price_string, str)): price_string = sub(r'[^\d.]', '', price_string) else: price_string = price.contents[0].contents[0] price_string = sub(r'[^\d.]', '', price_string) price_num = float(price_string) price_list.append(price_num) item_page_link = title_tags[i].findPrevious('a', href=True) # {'class': 's-item__link'}) if item_page_link.text: href_text = item_page_link['href'] link_list.append(item_page_link['href']) title_and_price_dict = { 'title': title_list, 'price': price_list, 'link': link_list } title_price_link_df = pd.DataFrame(title_and_price_dict) return title_price_link_df def build_pagelink_list(url): response = requests.get(url) if (response.status_code != 200): raise Exception('Failed to load page {}'.format(url)) page_contents = response.text doc = BeautifulSoup(page_contents, 'html.parser') for a in doc.find_all('a', href=True): if a.text: href_text = a['href'] if (href_text.find('pgn=') != -1): links_with_pgn_text.append(a['href']) if (len(links_with_pgn_text) < 1): links_with_pgn_text.append(url) def scrape_all_pages(): time.sleep(random.randint(1, 4)) for i in range(0, len(links_with_pgn_text)): next_page_url = links_with_pgn_text[i] frames.append(scrape_titles_and_prices(next_page_url,"")) time.sleep(random.randint(1, 2)) # main program def main_scraping(url): build_pagelink_list(url) scrape_all_pages() if (len(frames) > 1): result = pd.concat(frames, ignore_index=True) else: result = frames result.sort_values(by=['price']) update_datetime() fname = "origcomicart" + "_" + today + ".csv" result.to_csv(fname, index=None) return fname def parse_local_file(fname): with open(fname) as fp: document = BeautifulSoup(fp, 'html.parser') frames.append(scrape_titles_and_prices("", document)) if (len(frames) > 1): result = pd.concat(frames, ignore_index=True) else: result = frames[0] result.sort_values(by=['price']) update_datetime() fname = "origcomicart" + "_" + today + ".csv" result.to_csv(fname, index=None) return fname def indiv_page_link_cycler(csv_name): with open(csv_name, newline='') as f: reader = csv.reader(f) data = list(reader) for i in range(1, len(data)): if(i%50==0): update_datetime() print(i,' :links processed') link = data[i][2] response = requests.get(link) if (response.status_code != 200): raise Exception('Failed to load page {}'.format(url)) page_contents = response.text doc = BeautifulSoup(page_contents, 'html.parser') searched_word = 'Character' selection_class = 'attrLabels' character_tags = doc.find_all('td', {'class': selection_class}) for j in range(len(character_tags)): if (character_tags[j].contents): fullstring = character_tags[j].contents[0] if ("Character" or "character") in fullstring: character = character_tags[j].findNext('span') data[i].append(character.text) data[0].append('characters') data[0].append('multi-characters') fname = csv_name[:-4] fname = fname + "_chars.csv" with open(fname, 'w') as file: writer = csv.writer(file) writer.writerows(data) def add_headers(csv_file): with open(csv_file, newline='') as f: reader = csv.reader(f) data = list(reader) data[0].append('characters') data[0].append('multi-characters') fname = csv_file[:-4] fname = fname + "_append.csv" with open(fname, 'w') as file: writer = csv.writer(file) writer.writerows(data) def selenium_run(url): # open it, go to a website, and get results driver = webdriver.Chrome('chromedriver',options=options) # set selenium options to be headless, .. options = webdriver.ChromeOptions() options.add_argument('--headless') options.add_argument('--no-sandbox') options.add_argument('--disable-dev-shm-usage') #wd.get("https://www.ebay.com/sch/i.html?_fsrp=1&_from=R40&_sacat=0&LH_Sold=1&_mPrRngCbx=1&_udlo=50&_udhi&LH_BIN=1&_samilow&_samihi&_sadis=15&_stpos=10002&_sop=16&_dmd=1&_ipg=200&_fosrp=1&Type=Cover%7CInterior%2520Page%7CSplash%2520Page&LH_Complete=1&_nkw=original%20comic%20art&_dcat=3984&rt=nc&Publisher=DC%2520Comics%7CMarvel%7CMarvel%2520Comics") #print(wd.page_source) # results #PATH = '/Users/jmartin/Downloads/chromedriver' #options = webdriver.ChromeOptions() #options.add_argument("start-maximized") #options.add_argument("--headless") #options.add_experimental_option("excludeSwitches", ["enable-automation"]) #options.add_experimental_option('useAutomationExtension', False) #driver = webdriver.Chrome(options=options, executable_path=r"/Users/jmartin/Downloads/chromedriver") stealth( driver, user_agent = 'Mozilla/5.0 (Windows NT 10.0; Win64; x64) AppleWebKit/537.36 (KHTML, like Gecko) Chrome/83.0.4103.53 Safari/537.36', languages = "en", vendor = "Google Inc.", platform = "Win32", webgl_vendor = "Intel Inc.", renderer = "Intel Iris OpenGL Engine", fix_hairline = False, run_on_insecure_origins = False ) driver.delete_all_cookies() driver.get(url) update_datetime() #html_file_name = "sold_page_source-" + today + ".html" html_file_name = "sold_listings.html" with open(html_file_name, "w") as f: f.write(driver.page_source) return html_file_name orig_comicart_marv_dc_50plus_200perpage = 'https://www.ebay.com/sch/i.html?_dcat=3984&_fsrp=1&Type=Cover%7CInterior%2520Page%7CSplash%2520Page&_from=R40&_nkw=original+comic+art&_sacat=0&Publisher=Marvel%2520Comics%7CDC%2520Comics%7CUltimate%2520Marvel%7CMarvel%2520Age%7CMarvel%2520Adventures%7CMarvel&LH_BIN=1&_udlo=50&_sop=16&_ipg=200' orig_comicart_marv_dc_50plus_200perpage_sold = 'https://www.ebay.com/sch/i.html?_fsrp=1&_from=R40&_sacat=0&LH_Sold=1&_mPrRngCbx=1&_udlo=50&_udhi&LH_BIN=1&_samilow&_samihi&_sadis=15&_stpos=10002&_sop=16&_dmd=1&_ipg=200&_fosrp=1&Type=Cover%7CInterior%2520Page%7CSplash%2520Page&LH_Complete=1&_nkw=original%20comic%20art&_dcat=3984&rt=nc&Publisher=DC%2520Comics%7CMarvel%7CMarvel%2520Comics' # run the main scraping function searching_sold = False links_with_pgn_text = [] data = [] frames = [] #search for current orig comic listings search_url = orig_comicart_marv_dc_50plus_200perpage current_sales_csv = main_scraping(search_url) indiv_page_link_cycler(current_sales_csv) # now try to save the html for the sold data # usually it blocks the sales data links_with_pgn_text.clear() data.clear() frames.clear() sold_html_file = "" searching_sold = True search_url = orig_comicart_marv_dc_50plus_200perpage_sold #seach listing for sold items #below can also be run locally with selenium after you install the webdriver #and change the PATH variable so it points to your local installation directory sold_html_file = selenium_run(orig_comicart_marv_dc_50plus_200perpage_sold) #sold_html_file = "sold_listings.html" update_datetime() print("now parsing sold items") past_sales_csv = parse_local_file(sold_html_file) indiv_page_link_cycler(past_sales_csv) date and time: Jul-08-2021-13-50-40 processing a link: https://www.ebay.com/sch/i.html?_dcat=3984&_fsrp=1&Type=Cover%7CInterior%2520Page%7CSplash%2520Page&_from=R40&_nkw=original+comic+art&_sacat=0&Publisher=Marvel%2520Comics%7CDC%2520Comics%7CUltimate%2520Marvel%7CMarvel%2520Age%7CMarvel%2520Adventures%7CMarvel&LH_BIN=1&_udlo=50&_sop=16&_ipg=200&_pgn=1 date and time: Jul-08-2021-13-50-42 processing a link: https://www.ebay.com/sch/i.html?_dcat=3984&_fsrp=1&Type=Cover%7CInterior%2520Page%7CSplash%2520Page&_from=R40&_nkw=original+comic+art&_sacat=0&Publisher=Marvel%2520Comics%7CDC%2520Comics%7CUltimate%2520Marvel%7CMarvel%2520Age%7CMarvel%2520Adventures%7CMarvel&LH_BIN=1&_udlo=50&_sop=16&_ipg=200&_pgn=2 date and time: Jul-08-2021-13-50-45 processing a link: https://www.ebay.com/sch/i.html?_dcat=3984&_fsrp=1&Type=Cover%7CInterior%2520Page%7CSplash%2520Page&_from=R40&_nkw=original+comic+art&_sacat=0&Publisher=Marvel%2520Comics%7CDC%2520Comics%7CUltimate%2520Marvel%7CMarvel%2520Age%7CMarvel%2520Adventures%7CMarvel&LH_BIN=1&_udlo=50&_sop=16&_ipg=200&_pgn=3 date and time: Jul-08-2021-13-50-47 processing a link: https://www.ebay.com/sch/i.html?_dcat=3984&_fsrp=1&Type=Cover%7CInterior%2520Page%7CSplash%2520Page&_from=R40&_nkw=original+comic+art&_sacat=0&Publisher=Marvel%2520Comics%7CDC%2520Comics%7CUltimate%2520Marvel%7CMarvel%2520Age%7CMarvel%2520Adventures%7CMarvel&LH_BIN=1&_udlo=50&_sop=16&_ipg=200&_pgn=4 date and time: Jul-08-2021-13-50-51 processing a link: https://www.ebay.com/sch/i.html?_dcat=3984&_fsrp=1&Type=Cover%7CInterior%2520Page%7CSplash%2520Page&_from=R40&_nkw=original+comic+art&_sacat=0&Publisher=Marvel%2520Comics%7CDC%2520Comics%7CUltimate%2520Marvel%7CMarvel%2520Age%7CMarvel%2520Adventures%7CMarvel&LH_BIN=1&_udlo=50&_sop=16&_ipg=200&_pgn=5&rt=nc date and time: Jul-08-2021-13-50-57 processing a link: https://www.ebay.com/sch/i.html?_dcat=3984&_fsrp=1&Type=Cover%7CInterior%2520Page%7CSplash%2520Page&_from=R40&_nkw=original+comic+art&_sacat=0&Publisher=Marvel%2520Comics%7CDC%2520Comics%7CUltimate%2520Marvel%7CMarvel%2520Age%7CMarvel%2520Adventures%7CMarvel&LH_BIN=1&_udlo=50&_sop=16&_ipg=200&_pgn=6&rt=nc date and time: Jul-08-2021-13-51-01 date and time: Jul-08-2021-13-51-33 50 :links processed date and time: Jul-08-2021-13-52-06 100 :links processed date and time: Jul-08-2021-13-52-39 150 :links processed date and time: Jul-08-2021-13-53-12 200 :links processed date and time: Jul-08-2021-13-53-44 250 :links processed date and time: Jul-08-2021-13-54-17 300 :links processed date and time: Jul-08-2021-13-54-51 350 :links processed date and time: Jul-08-2021-13-55-23 400 :links processed date and time: Jul-08-2021-13-55-56 450 :links processed date and time: Jul-08-2021-13-56-26 500 :links processed date and time: Jul-08-2021-13-56-58 550 :links processed date and time: Jul-08-2021-13-57-29 600 :links processed date and time: Jul-08-2021-13-58-02 650 :links processed date and time: Jul-08-2021-13-58-34 700 :links processed date and time: Jul-08-2021-13-59-06 750 :links processed date and time: Jul-08-2021-13-59-36 800 :links processed date and time: Jul-08-2021-13-59-42 now parsing sold items date and time: Jul-08-2021-13-59-42 date and time: Jul-08-2021-13-59-42 date and time: Jul-08-2021-14-00-19 50 :links processed date and time: Jul-08-2021-14-00-57 100 :links processed
Final Code
from __future__ import division, unicode_literals import requests from bs4 import BeautifulSoup from re import sub from decimal import Decimal import pandas as pd import requests import random import time import os import csv from datetime import date from datetime import datetime import codecs from selenium import webdriver from selenium_stealth import stealth import time import random s = requests.session() s.cookies.clear() now = datetime.now() today = date.today() today = today.strftime("%b-%d-%Y") date_time = now.strftime("%H-%M-%S") today = today + "-" + date_time def update_datetime(): global now global today global date_time now = datetime.now() today = date.today() today = today.strftime("%b-%d-%Y") date_time = now.strftime("%H-%M-%S") today = today + "-" + date_time print("date and time:", today) html_doc = """ <html><head><title>place holder</title></head> """ s.cookies.clear() # this just initializes the beautiful soup doc as a global variable doc = BeautifulSoup(html_doc, 'html.parser') def scrape_titles_and_prices(url, document): s.cookies.clear() update_datetime() if document: using_local_doc=True doc = document title_class = 'lvtitle' price_class = 'bold bidsold' link_class = 'vip' else: print('processing a link: ', url) using_local_doc = False response = requests.get(url) if (response.status_code != 200): raise Exception('Failed to load page {}'.format(url)) page_contents = response.text doc = BeautifulSoup(page_contents, 'html.parser') filename = 'comic_art_marvel_dc' + today + '.html' if searching_sold: sold_html_file = filename with open(filename, 'w') as f: f.write(page_contents) title_class = 's-item__title' price_class = 's-item__price' link_class = 's-item__link' title_tags = doc.find_all('h3', {'class': title_class}) title_list = [] price_list = [] link_list = [] for i in range(len(title_tags)): if (title_tags[i].contents): if using_local_doc: title_contents = title_tags[i].contents[0].contents[0] else: title_contents = title_tags[i].contents[0] title_list.append(title_contents) price = title_tags[i].findNext('span', {'class': price_class}) if price.contents: if len(price.contents)>1 and using_local_doc: price_string = price.contents[1].contents[0] else: price_string = price.contents[0] if (isinstance(price_string, str)): price_string = sub(r'[^\d.]', '', price_string) else: price_string = price.contents[0].contents[0] price_string = sub(r'[^\d.]', '', price_string) price_num = float(price_string) price_list.append(price_num) item_page_link = title_tags[i].findPrevious('a', href=True) # {'class': 's-item__link'}) if item_page_link.text: href_text = item_page_link['href'] link_list.append(item_page_link['href']) title_and_price_dict = { 'title': title_list, 'price': price_list, 'link': link_list } title_price_link_df = pd.DataFrame(title_and_price_dict) return title_price_link_df def build_pagelink_list(url): response = requests.get(url) if (response.status_code != 200): raise Exception('Failed to load page {}'.format(url)) page_contents = response.text doc = BeautifulSoup(page_contents, 'html.parser') for a in doc.find_all('a', href=True): if a.text: href_text = a['href'] if (href_text.find('pgn=') != -1): links_with_pgn_text.append(a['href']) if (len(links_with_pgn_text) < 1): links_with_pgn_text.append(url) def scrape_all_pages(): time.sleep(random.randint(1, 4)) for i in range(0, len(links_with_pgn_text)): next_page_url = links_with_pgn_text[i] frames.append(scrape_titles_and_prices(next_page_url,"")) time.sleep(random.randint(1, 2)) # main program def main_scraping(url): build_pagelink_list(url) scrape_all_pages() if (len(frames) > 1): result = pd.concat(frames, ignore_index=True) else: result = frames result.sort_values(by=['price']) update_datetime() fname = "origcomicart" + "_" + today + ".csv" result.to_csv(fname, index=None) return fname def parse_local_file(fname): with open(fname) as fp: document = BeautifulSoup(fp, 'html.parser') frames.append(scrape_titles_and_prices("", document)) if (len(frames) > 1): result = pd.concat(frames, ignore_index=True) else: result = frames[0] result.sort_values(by=['price']) update_datetime() fname = "origcomicart" + "_" + today + ".csv" result.to_csv(fname, index=None) return fname def indiv_page_link_cycler(csv_name): with open(csv_name, newline='') as f: reader = csv.reader(f) data = list(reader) for i in range(1, len(data)): if(i%50==0): update_datetime() print(i,' :links processed') link = data[i][2] response = requests.get(link) if (response.status_code != 200): raise Exception('Failed to load page {}'.format(url)) page_contents = response.text doc = BeautifulSoup(page_contents, 'html.parser') searched_word = 'Character' selection_class = 'attrLabels' character_tags = doc.find_all('td', {'class': selection_class}) for j in range(len(character_tags)): if (character_tags[j].contents): fullstring = character_tags[j].contents[0] if ("Character" or "character") in fullstring: character = character_tags[j].findNext('span') data[i].append(character.text) data[0].append('characters') data[0].append('multi-characters') fname = csv_name[:-4] fname = fname + "_chars.csv" with open(fname, 'w') as file: writer = csv.writer(file) writer.writerows(data) def add_headers(csv_file): with open(csv_file, newline='') as f: reader = csv.reader(f) data = list(reader) data[0].append('characters') data[0].append('multi-characters') fname = csv_file[:-4] fname = fname + "_append.csv" with open(fname, 'w') as file: writer = csv.writer(file) writer.writerows(data) def selenium_run(url): options = webdriver.ChromeOptions() options.add_argument('--headless') options.add_argument('--no-sandbox') options.add_argument('--disable-dev-shm-usage') options.add_argument("start-maximized") options.add_experimental_option("excludeSwitches", ["enable-automation"]) options.add_experimental_option('useAutomationExtension', False) # open it, go to a website, and get results driver = webdriver.Chrome('chromedriver',options=options) # uncomment below and change paths if running locally (and comment the line above) #PATH = '/Users/jmartin/Downloads/chromedriver' #driver = webdriver.Chrome(options=options, executable_path=r"/Users/jmartin/Downloads/chromedriver") stealth( driver, user_agent = 'Mozilla/5.0 (Windows NT 10.0; Win64; x64) AppleWebKit/537.36 (KHTML, like Gecko) Chrome/83.0.4103.53 Safari/537.36', languages = "en", vendor = "Google Inc.", platform = "Win32", webgl_vendor = "Intel Inc.", renderer = "Intel Iris OpenGL Engine", fix_hairline = False, run_on_insecure_origins = False ) driver.delete_all_cookies() driver.get(url) update_datetime() #html_file_name = "sold_page_source-" + today + ".html" html_file_name = "sold_listings.html" with open(html_file_name, "w") as f: f.write(driver.page_source) return html_file_name orig_comicart_marv_dc_50plus_200perpage = 'https://www.ebay.com/sch/i.html?_dcat=3984&_fsrp=1&Type=Cover%7CInterior%2520Page%7CSplash%2520Page&_from=R40&_nkw=original+comic+art&_sacat=0&Publisher=Marvel%2520Comics%7CDC%2520Comics%7CUltimate%2520Marvel%7CMarvel%2520Age%7CMarvel%2520Adventures%7CMarvel&LH_BIN=1&_udlo=50&_sop=16&_ipg=200' orig_comicart_marv_dc_50plus_200perpage_sold = 'https://www.ebay.com/sch/i.html?_fsrp=1&_from=R40&_sacat=0&LH_Sold=1&_mPrRngCbx=1&_udlo=50&_udhi&LH_BIN=1&_samilow&_samihi&_sadis=15&_stpos=10002&_sop=16&_dmd=1&_ipg=200&_fosrp=1&Type=Cover%7CInterior%2520Page%7CSplash%2520Page&LH_Complete=1&_nkw=original%20comic%20art&_dcat=3984&rt=nc&Publisher=DC%2520Comics%7CMarvel%7CMarvel%2520Comics' # run the main scraping function searching_sold = False links_with_pgn_text = [] data = [] frames = [] #search for current orig comic listings search_url = orig_comicart_marv_dc_50plus_200perpage current_sales_csv = main_scraping(search_url) indiv_page_link_cycler(current_sales_csv) # now try to save the html for the sold data # usually it blocks the sales data links_with_pgn_text.clear() data.clear() frames.clear() sold_html_file = "" searching_sold = True search_url = orig_comicart_marv_dc_50plus_200perpage_sold #seach listing for sold items #below can also be run locally with selenium after you install the webdriver #and change the PATH variable so it points to your local installation directory sold_html_file = selenium_run(orig_comicart_marv_dc_50plus_200perpage_sold) #sold_html_file = "sold_listings.html" update_datetime() print("now parsing sold items") past_sales_csv = parse_local_file(sold_html_file) indiv_page_link_cycler(past_sales_csv) date and time: Sep-27-2021-14-02-53 processing a link: https://www.ebay.com/sch/i.html?_dcat=3984&_fsrp=1&Type=Cover%7CInterior%2520Page%7CSplash%2520Page&_from=R40&_nkw=original+comic+art&_sacat=0&Publisher=Marvel%2520Comics%7CDC%2520Comics%7CUltimate%2520Marvel%7CMarvel%2520Age%7CMarvel%2520Adventures%7CMarvel&LH_BIN=1&_udlo=50&_sop=16&_ipg=200&_pgn=1 date and time: Sep-27-2021-14-02-55 processing a link: https://www.ebay.com/sch/i.html?_dcat=3984&_fsrp=1&Type=Cover%7CInterior%2520Page%7CSplash%2520Page&_from=R40&_nkw=original+comic+art&_sacat=0&Publisher=Marvel%2520Comics%7CDC%2520Comics%7CUltimate%2520Marvel%7CMarvel%2520Age%7CMarvel%2520Adventures%7CMarvel&LH_BIN=1&_udlo=50&_sop=16&_ipg=200&_pgn=2 date and time: Sep-27-2021-14-02-59 processing a link: https://www.ebay.com/sch/i.html?_dcat=3984&_fsrp=1&Type=Cover%7CInterior%2520Page%7CSplash%2520Page&_from=R40&_nkw=original+comic+art&_sacat=0&Publisher=Marvel%2520Comics%7CDC%2520Comics%7CUltimate%2520Marvel%7CMarvel%2520Age%7CMarvel%2520Adventures%7CMarvel&LH_BIN=1&_udlo=50&_sop=16&_ipg=200&_pgn=3 date and time: Sep-27-2021-14-03-02 processing a link: https://www.ebay.com/sch/i.html?_dcat=3984&_fsrp=1&Type=Cover%7CInterior%2520Page%7CSplash%2520Page&_from=R40&_nkw=original+comic+art&_sacat=0&Publisher=Marvel%2520Comics%7CDC%2520Comics%7CUltimate%2520Marvel%7CMarvel%2520Age%7CMarvel%2520Adventures%7CMarvel&LH_BIN=1&_udlo=50&_sop=16&_ipg=200&_pgn=4 date and time: Sep-27-2021-14-03-05 processing a link: https://www.ebay.com/sch/i.html?_dcat=3984&_fsrp=1&Type=Cover%7CInterior%2520Page%7CSplash%2520Page&_from=R40&_nkw=original+comic+art&_sacat=0&Publisher=Marvel%2520Comics%7CDC%2520Comics%7CUltimate%2520Marvel%7CMarvel%2520Age%7CMarvel%2520Adventures%7CMarvel&LH_BIN=1&_udlo=50&_sop=16&_ipg=200&_pgn=5&rt=nc date and time: Sep-27-2021-14-03-10 processing a link: https://www.ebay.com/sch/i.html?_dcat=3984&_fsrp=1&Type=Cover%7CInterior%2520Page%7CSplash%2520Page&_from=R40&_nkw=original+comic+art&_sacat=0&Publisher=Marvel%2520Comics%7CDC%2520Comics%7CUltimate%2520Marvel%7CMarvel%2520Age%7CMarvel%2520Adventures%7CMarvel&LH_BIN=1&_udlo=50&_sop=16&_ipg=200&_pgn=6&rt=nc date and time: Sep-27-2021-14-03-15 processing a link: https://www.ebay.com/sch/i.html?_dcat=3984&_fsrp=1&Type=Cover%7CInterior%2520Page%7CSplash%2520Page&_from=R40&_nkw=original+comic+art&_sacat=0&Publisher=Marvel%2520Comics%7CDC%2520Comics%7CUltimate%2520Marvel%7CMarvel%2520Age%7CMarvel%2520Adventures%7CMarvel&LH_BIN=1&_udlo=50&_sop=16&_ipg=200&_pgn=7&rt=nc date and time: Sep-27-2021-14-03-19 date and time: Sep-27-2021-14-03-51 50 :links processed date and time: Sep-27-2021-14-04-23 100 :links processed date and time: Sep-27-2021-14-04-55 150 :links processed date and time: Sep-27-2021-14-05-28 200 :links processed date and time: Sep-27-2021-14-06-02 250 :links processed date and time: Sep-27-2021-14-06-35 300 :links processed date and time: Sep-27-2021-14-07-07 350 :links processed date and time: Sep-27-2021-14-07-40 400 :links processed date and time: Sep-27-2021-14-08-14 450 :links processed date and time: Sep-27-2021-14-08-46 500 :links processed date and time: Sep-27-2021-14-09-20 550 :links processed date and time: Sep-27-2021-14-09-52 600 :links processed date and time: Sep-27-2021-14-10-25 650 :links processed date and time: Sep-27-2021-14-10-56 700 :links processed date and time: Sep-27-2021-14-11-30 750 :links processed date and time: Sep-27-2021-14-12-03 800 :links processed date and time: Sep-27-2021-14-12-11 date and time: Sep-27-2021-14-12-11 now parsing sold items date and time: Sep-27-2021-14-12-11 date and time: Sep-27-2021-14-12-11
Summary
Our purpose for this research was to obtain two csv files relating to original comic art on eBay.
The first csv file featured all of Marvel and DC's current lists of inside pages, covers, and splash pages.
The second csv file had similar data about things that had been sold.
This allowed us to swiftly (far faster than eBay's UI) search the current offerings for paintings in a specific price range and characters that looked interesting. We may then seek comparable art in the csv of sold things to evaluate if this was a decent buy.
The Steps that We Used
To access the HTML content from a URL generated by eBay's filtering system, use the requests library.
Use BeautifulSoup to look for tags (p, div, a, etc.) in the HTML text of the original search page results for the data we needed, such as listing title and description, link to the full listing, and price
Include this information in your listings.
Make a pandas data frame out of the lists and save it as a csv file.
Then browse through the links and open each full artwork listing with requests, then utilize BeautifulSoup to get the character details.
Create a csv file with the data frame.
Because eBay prohibited the use of requests to obtain this html content for sold items, we had to store the html content as an html file using Selenium. We then opened this file in BeautifulSoup and parsed it using the same methods.
For more details, contact 3i Data Scraping today
Request for a quote!!
0 notes