#Streaming with AWS Lambda
Explore tagged Tumblr posts
Text
#Custom Python Runtime#Streaming with AWS Lambda#Amazon Streaming Services#Serverless Streaming Solutions#Streaming Architecture AWS#Spotify
0 notes
Text
Barely right - Dottore x reader
Series masterlist Notes: It's been a little more than one month in Snezhnaya. A little more than one month as The Doctor's assistant. Reupload from my old blog. Steal this to get Enterobius vermicularis put in your dinner. Tags: Dottore x reader, start of a slow slow burn, reader is not a good person. Minors DNI
Not even the damnable flickering of the harsh lights could dampen the giddy feeling in your gut. It was just a matter of time before the courier was set to arrive and you could finally progress your personal project.
Not that one and a half week was a lot of time for a shipment like this, not by any means, but striking while the iron is hot is imperative. For well over a month, you'd sworn to never let the flames consuming your soul burn as brightly as the night you left. Not even if it would turn out to be what kept you warm in this frozen wasteland.
As if on cue, and you supposed it was, a shiver ran through your body. Even with the added labcoat that you insisted on wearing despite no one else bothering to, the frigid air still managed to settle as pins in your bones.
Snow had been pelting outside for what felt like days on end, the grey skies showing no hint of mercy. For a nation of supposed love, there was little compassion to be found in the land or people. Not that you'd met an awful lot of folks to begin with; there were the two who stopped you at the border, an officer - Denis maybe? - who'd aided with the recruitment process and… Save for the guards you silently greeted every morning that was about it.
How harrowingly lonely now that it was laid out before you. Still, cold neutrality was better than being surrounded by false kindness as you'd been in Fontaine. But that score would soon be settled, as soon as you had the water sample you could-
A sharp bark of laughter had the bottle you'd been cleaning slip momentarily, heart rate spiking as you managed to catch it. Right, the clones of your superior should probably also count for something.
Or 'segments' as he'd called them. How he'd managed to create something that absurd, something so far from everything you knew of this world, held your mind by the throat, especially paired with the wave of a hand he'd given upon your inquiry, brushing it off as 'a temporary measure'.
For now, you'd simply count yourself lucky that they were there, it counted for social interaction even if they were technically not separate people. A sigh escaped your lips, seeing two of them off to the right end, clearly about to start yelling with a broken piece of machinery at the feet of one. Separate enough to cause problems.
Setting the remaining dirty glassware aside, you cleared your throat, politely at first and then with a little more vigor, "Lambda?" No reaction, how annoying, "Lambda! Could you and," shit who was that? "Your brother take that outside?"
Several pairs of hauntingly bright 'eyes' fixed upon you. Though you'd heard plenty of the Harbingers and their unsavory traits, you'd never expected something this odd, having been caught completely by surprise upon first discovering one of the segments. So far, they'd been annoying at best and unnerving at worst.
A few snickers whisked through the room with the cold stream of air from the ever running ventilation. "Lambda isn't here, try again Mademoiselle."
Fantastic. You rubbed at your cheeks, trying to recall the rest of those blasted nonsensical names and already feeling a headache coming on, and not even one you could remove by putting distance between the heavy scent of bleach and yourself. This was a waste of time, you had tissue biopsies to prepare, toxins to purify, fungal colonies to transfer onto fresh plates before the slime condensate lost all moisture to the unforgiving dry air, and probably more you were forgetting.
"Quiet. She's right, you two are utterly insufferable. Either get back to work or get out, and I'm certain you're both aware what the wisest choice would be."
Silence ensued, only broken by sharp clicks of metal against the harsh floors. The voice was too languid to be the original, a look in the direction of the approaching figure confirming your suspicion. This one you knew; Omega. With his neatly styled hair and clattering ornaments, it was impossible to mistake him for any of the others.
A rough hand pushed the segment aside, effectively crushing any nonchalance as Omega stumbled, The Doctor himself easily recognizable with the stubbled chin and rowdy teal hair. "Don't get too full of yourself, go prepare."
Grace reclaimed, Omega turned on his heel, utterly unperturbed by how the rest seemed to collectively sigh at his fluttering tailcoat. Even in a sea of misfits, he managed to stand out, a single magpie strutting around a murder of crows.
"And you," his voice might as well have cut through your flesh with how it stung, leaving you utterly confused by the calm continuation. "What have you been doing?"
Before you could answer, The Doctor had clicked his tongue and clearly decided you weren't doing well, encroaching on your space and reaching for various of your sealed tubes that lined the racks, most of them failures to be discarded. You could only fear a mind that could keep up with the speed of his hands, plucking each tube and turning to read the label before placing it back and repeating with the next. Methodical and precise for all of the minute it took him to have inspected it all to his apparent dissatisfaction.
The Doctor waved a gloved hand at the full sink, lips turned down in what you assumed to be disgust. "Have you been cleaning after yourself?"
After yourself? Was that now wrong, or had you missed some crucial detail about procedures during the first hectical day? Another mistake would be beyond frustrating to add to the tally. And why was his tone so painfully accusatory?
"I've been-"
Your brows furrowed when he waved dismissively, not even deigning to look in your direction. If knocking his mask off wouldn't be tantamount to a death wish, and you didn't have unfinished business, perhaps you'd have done it.
"Xi, if I find out you've been neglecting your assignments one more time…" Something clearly passed between The Doctor and the approaching segment, no bigger than an adolescent boy, stomped towards your station, begrudgingly dragging a stepping stool and steel basket with him. "Are we clear?"
The round cheeks and pout would have been endearing if not for the glaring discrepancy between those soft organic features and the upper half of his face being purely mechanical. A glowing red 'eye' sat at the middle, the steady buzz from it seemingly intensifying as the child-thing positioned itself. You were certain that if it could, it would have gladly rolled its eyes at its creator a thousand times over.
Muffled chuckles from around the room were drowned out by clattering glass as Xi began clearing your station with sweeping motions that seemed like reckless abandon. Swiftly, you leaned over and pushed everything important to the back of the table, hoping he would either take the hint or be unable to reach.
"As for you," less frigid but just as firm, Dottore's voice commanded your attention back to him, "a shipment arrived from Fontaine."
Swift as a viper from the dunes he allegedly grew up in, you weren't exactly sure, having only heard rumors, a hand curled around your bicep before you'd finished bowing your head in adieu.
"I wasn't finished."
His eyes weren't needed to envision the pointed look in them, you at least supposed the 'real' Doctor would have eyes, the flex of his sculpted jaw was enough to carry his irritation across. Your eyes briefly searched the room for any sign of the crate, uncertainty for whether you would even be able to pick anything out in the buzzing mess that made up the main laboratory settling in the back of your mind.
Steeling yourself, you tugged at your arm, "Give me a moment to unpack the contents and I'll be all yours, Doctor."
The slight twitch of his lips didn't go unnoticed, yet his grip remained unrelenting.
"Remind me, when did I sign off on this?"
"What?" Your brows furrowed at the question as you tugged absently on your arm, still torn between concern for the reagents and how far you were willing to push your luck.
"She had Omega do it ten days ago," a bright voice from one of the younger segments filled the silence, confirming the suspicion that although they'd all returned to work, the interaction was still being carefully monitored.
Dottore himself repeated the statement, free hand swiping a gloved finger along the table and inspecting it for any trace of grime. It had the hairs on the back of your neck standing.
"You weren't around, and Omega said that if I wanted it swiftly it'd be easier to have him sign rather than wait for you," hopefully the implied concern for his schedule wasn't lost, "and since I don't know how they've been packaged, I'd appreciate tending to them sooner rather than later."
A frown settled on both your lips, with Dottore's grip tightening further, "Let me make it perfectly clear, unless I am - against all odds - not present in Snezhnaya at the given time, you will wait for my approval."
As if reading your mind, he swiftly continued, "They are additions, parts, not replacements."
Finally released, you sidestepped his looming presence, keenly aware of the pointed beak following your movements towards the door.
#yeah yeah whatever man#*yeets this*#yeeting this out there because I can't write anything new anyways#dottore#dottore x reader#dottore x you#il dottore x you#il dottore x reader#zandik x you#zandik x reader#il dottore#genshin impact x reader#fatui harbingers x reader#fatui x reader#fatui harbingers#crow with a pen
40 notes
·
View notes
Text
Just completed the forth week of MLOps Zoomcamp.
The lessons covered include:
Three ways of model deployment: Online (web and streaming) and offline (batch)
Web service: model deployment with Flask
Streaming: consuming events with AWS Kinesis and Lambda
Batch: scoring data offline
The link to the course is below: https://github.com/DataTalksClub/mlops-zoomcamp
3 notes
·
View notes
Text
java full stack
A Java Full Stack Developer is proficient in both front-end and back-end development, using Java for server-side (backend) programming. Here's a comprehensive guide to becoming a Java Full Stack Developer:
1. Core Java
Fundamentals: Object-Oriented Programming, Data Types, Variables, Arrays, Operators, Control Statements.
Advanced Topics: Exception Handling, Collections Framework, Streams, Lambda Expressions, Multithreading.
2. Front-End Development
HTML: Structure of web pages, Semantic HTML.
CSS: Styling, Flexbox, Grid, Responsive Design.
JavaScript: ES6+, DOM Manipulation, Fetch API, Event Handling.
Frameworks/Libraries:
React: Components, State, Props, Hooks, Context API, Router.
Angular: Modules, Components, Services, Directives, Dependency Injection.
Vue.js: Directives, Components, Vue Router, Vuex for state management.
3. Back-End Development
Java Frameworks:
Spring: Core, Boot, MVC, Data JPA, Security, Rest.
Hibernate: ORM (Object-Relational Mapping) framework.
Building REST APIs: Using Spring Boot to build scalable and maintainable REST APIs.
4. Database Management
SQL Databases: MySQL, PostgreSQL (CRUD operations, Joins, Indexing).
NoSQL Databases: MongoDB (CRUD operations, Aggregation).
5. Version Control/Git
Basic Git commands: clone, pull, push, commit, branch, merge.
Platforms: GitHub, GitLab, Bitbucket.
6. Build Tools
Maven: Dependency management, Project building.
Gradle: Advanced build tool with Groovy-based DSL.
7. Testing
Unit Testing: JUnit, Mockito.
Integration Testing: Using Spring Test.
8. DevOps (Optional but beneficial)
Containerization: Docker (Creating, managing containers).
CI/CD: Jenkins, GitHub Actions.
Cloud Services: AWS, Azure (Basics of deployment).
9. Soft Skills
Problem-Solving: Algorithms and Data Structures.
Communication: Working in teams, Agile/Scrum methodologies.
Project Management: Basic understanding of managing projects and tasks.
Learning Path
Start with Core Java: Master the basics before moving to advanced concepts.
Learn Front-End Basics: HTML, CSS, JavaScript.
Move to Frameworks: Choose one front-end framework (React/Angular/Vue.js).
Back-End Development: Dive into Spring and Hibernate.
Database Knowledge: Learn both SQL and NoSQL databases.
Version Control: Get comfortable with Git.
Testing and DevOps: Understand the basics of testing and deployment.
Resources
Books:
Effective Java by Joshua Bloch.
Java: The Complete Reference by Herbert Schildt.
Head First Java by Kathy Sierra & Bert Bates.
Online Courses:
Coursera, Udemy, Pluralsight (Java, Spring, React/Angular/Vue.js).
FreeCodeCamp, Codecademy (HTML, CSS, JavaScript).
Documentation:
Official documentation for Java, Spring, React, Angular, and Vue.js.
Community and Practice
GitHub: Explore open-source projects.
Stack Overflow: Participate in discussions and problem-solving.
Coding Challenges: LeetCode, HackerRank, CodeWars for practice.
By mastering these areas, you'll be well-equipped to handle the diverse responsibilities of a Java Full Stack Developer.
visit https://www.izeoninnovative.com/izeon/
2 notes
·
View notes
Text
Advanced Techniques in Full-Stack Development
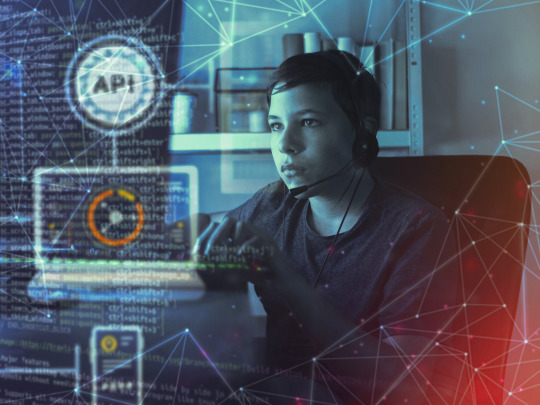
Certainly, let's delve deeper into more advanced techniques and concepts in full-stack development:
1. Server-Side Rendering (SSR) and Static Site Generation (SSG):
SSR: Rendering web pages on the server side to improve performance and SEO by delivering fully rendered pages to the client.
SSG: Generating static HTML files at build time, enhancing speed, and reducing the server load.
2. WebAssembly:
WebAssembly (Wasm): A binary instruction format for a stack-based virtual machine. It allows high-performance execution of code on web browsers, enabling languages like C, C++, and Rust to run in web applications.
3. Progressive Web Apps (PWAs) Enhancements:
Background Sync: Allowing PWAs to sync data in the background even when the app is closed.
Web Push Notifications: Implementing push notifications to engage users even when they are not actively using the application.
4. State Management:
Redux and MobX: Advanced state management libraries in React applications for managing complex application states efficiently.
Reactive Programming: Utilizing RxJS or other reactive programming libraries to handle asynchronous data streams and events in real-time applications.
5. WebSockets and WebRTC:
WebSockets: Enabling real-time, bidirectional communication between clients and servers for applications requiring constant data updates.
WebRTC: Facilitating real-time communication, such as video chat, directly between web browsers without the need for plugins or additional software.
6. Caching Strategies:
Content Delivery Networks (CDN): Leveraging CDNs to cache and distribute content globally, improving website loading speeds for users worldwide.
Service Workers: Using service workers to cache assets and data, providing offline access and improving performance for returning visitors.
7. GraphQL Subscriptions:
GraphQL Subscriptions: Enabling real-time updates in GraphQL APIs by allowing clients to subscribe to specific events and receive push notifications when data changes.
8. Authentication and Authorization:
OAuth 2.0 and OpenID Connect: Implementing secure authentication and authorization protocols for user login and access control.
JSON Web Tokens (JWT): Utilizing JWTs to securely transmit information between parties, ensuring data integrity and authenticity.
9. Content Management Systems (CMS) Integration:
Headless CMS: Integrating headless CMS like Contentful or Strapi, allowing content creators to manage content independently from the application's front end.
10. Automated Performance Optimization:
Lighthouse and Web Vitals: Utilizing tools like Lighthouse and Google's Web Vitals to measure and optimize web performance, focusing on key user-centric metrics like loading speed and interactivity.
11. Machine Learning and AI Integration:
TensorFlow.js and ONNX.js: Integrating machine learning models directly into web applications for tasks like image recognition, language processing, and recommendation systems.
12. Cross-Platform Development with Electron:
Electron: Building cross-platform desktop applications using web technologies (HTML, CSS, JavaScript), allowing developers to create desktop apps for Windows, macOS, and Linux.
13. Advanced Database Techniques:
Database Sharding: Implementing database sharding techniques to distribute large databases across multiple servers, improving scalability and performance.
Full-Text Search and Indexing: Implementing full-text search capabilities and optimized indexing for efficient searching and data retrieval.
14. Chaos Engineering:
Chaos Engineering: Introducing controlled experiments to identify weaknesses and potential failures in the system, ensuring the application's resilience and reliability.
15. Serverless Architectures with AWS Lambda or Azure Functions:
Serverless Architectures: Building applications as a collection of small, single-purpose functions that run in a serverless environment, providing automatic scaling and cost efficiency.
16. Data Pipelines and ETL (Extract, Transform, Load) Processes:
Data Pipelines: Creating automated data pipelines for processing and transforming large volumes of data, integrating various data sources and ensuring data consistency.
17. Responsive Design and Accessibility:
Responsive Design: Implementing advanced responsive design techniques for seamless user experiences across a variety of devices and screen sizes.
Accessibility: Ensuring web applications are accessible to all users, including those with disabilities, by following WCAG guidelines and ARIA practices.
full stack development training in Pune
2 notes
·
View notes
Text
Top 11 Elixir Services companies in the World in 2025

Introduction:
Elixir has become a highly scalable programming language in the ever-changing world of software development. Its concurrent and fault-tolerant architectural design gives Elixir an edge that is being recognized by many of today's tech giants. This article will delve into what constitutes the top Elixir services companies in the world in 2025 — companies with a spirit of innovation that have delivered reliable, high-performance solutions. This list tells you the trusted names in the industry, from hiring the best Elixir developers in the world to getting a trustworthy Elixir development company.
1. Icreativez Technologies
iCreativez is among the elite Elixir Services companies around the globe. It caters to startups, enterprises, and tech-driven firms with an impeccable custom software solution. Their team of well-seasoned Elixir engineers builds scalable backends, real-time apps, and distributed systems.
Key Services:
Elixir development tailored to client specifications.
Real-time application architecture.
Phoenix framework solutions.
DevOps integration with Elixir.
API and microservices development.
iCreativez is certainly not a run-of-the-mill Elixir development house; it is an agency for Elixir development that many businesses rely on for high performance, scalability, and innovation. Agile methodologies and great client communication have made them the preferred partner across the globe.
Contact Information:
website: www.icreativez.com
Founder: Mehboob Shar
Location: Pakistan
Service: 24/7 Availaible
2. Amazon Web Services
AWS uses Elixir in several backend processes and serverless applications, but mainly, they are known for cloud infrastructure. In fact, as the coolest among the Elixir fans, the company makes a dimension with the language's efficiency concerning tasks of real-time communication and data.
Specific Application of Elixir at AWS Includes:
Real-time serverless applications.
Lambda function enhancements.
Scalable messaging systems.
3. BigCommerce
Being one of the best e-commerce platforms, BigCommerce flaunts itself as one of the hot Elixir companies for 2025 due to the seamless use of Elixir in powering fast and reliable backend systems. The application has been made to operate with Elixir to improve their work from order processing and inventory management process to customer interaction.
Core Contributions:
APIs powered by Elixir
Inventory real-time updates
Order routing and automation
4. Arcadia
A data science-driven energy platform, Arcadia projects itself best as the Elixir development programming company, processing enormous, enormous data through complete and swift Elixir processing. They visualize the concurrency properties of the language to stream energy data over distributed pipelines.
Services Include:
Energy data analytics
Real-time performance dashboards
Integration of Elixir and machine learning
5. Altium
Altium has the latest PCB design software. Their engineering team has gone to Elixir for their backend tooling and simulation environments. The incorporation of the Elixir technology into high-computation design environments marks them out as a top Elixir development company.
Key Solutions:
Real-time circuit simulations
API integration
Collaborative hardware design tools
6. SmartBear
SmartBear is a known brand in the tool industry for Swagger and TestComplete. The company has used Elixir in the development of test automation platforms and scalable analytics. This focus on developer experience places them among the best Elixir Services companies worldwide.
Specializations:
Automated testing platforms
Elixir backend for QA dashboards
Developer support tools
7. migSO
MigSO is a project management and engineering company using Elixir to manage complex industrial and IT projects. Custom Elixir solutions allow organizations to keep real-time tab on large-scale project metrics.
Notable Features:
Real-time monitoring systems
Industrial process integration
Elixir dashboards for reporting
8. Digis
Digis is a global software development company that offers dedicated Elixir teams. They are well known to be a very efficient Elixir development agency that provides customized staffing for businesses that need to rapidly scale in a cost-effective manner.
Core Services:
Dedicated Elixir teams
Agile product development
Custom application architecture
9. Mobitek Marketing Group
Mobitek integrates Elixir with their MarTech to real-time data processing, campaigns tracking, and audience segmentation. As a contemporary digital company, they proceed with Elixir in the development of intelligent and adaptive marketing platforms.
Expertise includes:
Real-time data tracking
Campaign performance backend
CRM development platforms powered by Elixir
10. Green Apex
Green Apex must have been in Elixir Development Services across eCommerce, fintech, and healthcare for full-stack development since always. They write applications that can accommodate thousands of concurrent users with apparent ease.
Notable Strengths:
Realtime chat and notification
Microservices building
Scalable cloud apps
11. iDigitalise - Digital Marketing Agency
iDigitalise is known first as a digital marketing agency; however, they have also built some strong analytical and automated platforms using Elixir. The use of Elixir puts them among a few very hidden gems when it comes to considering top Elixir development companies and developers.
What They Have:
Elixir-supported analytic dashboards
Backend for marketing automation
SEO and campaign data processing in real-time
What Is So Special About Elixir in 2025?
It is often said that with an increasing number of companies worldwide turning towards Elixir, such popularity is hardly incidental. With the low-latency e-commerce handling of huge concurrency, Elixir finds its niche in industry verticals, including fintech, healthcare, eCommerce, and IoT.
For the best Elixir services in the world, differentiate between the ones with Elixir developers and, in addition, have proven records of practical application with worked-out projects using Elixir in complex real-world applications.
FAQs:
Q1: What are the best Elixir development companies in 2025?
Companies specializing in scalable, real-time Elixir applications, such as iCreativez, Digis, and Green Apex, are all mentioned among the very best.
Q2: Why does Elixir appeal to top companies?
Elixir is propitious for contemporary purposes whereby the require of applications extends beyond the handling and manipulating of real-time data to the aspect of engaging users.
Q3: Which industries benefit the most from implementing Elixir?
Fintech, e-commerce, healthcare, and data analytics would maximize the use of Elixir due to its concurrency benefits.
Q4: How do I select a competent Elixir development agency?
Choose an agency that has solid portfolios, some expertise in real-time systems, and some experience using Elixir with frameworks like Phoenix.
Conclusion
Elixir indeed urges organizations to rethink their strategies regarding backend development and real-time systems. The world's elite Elixir services superstars not only bring great software but also continue to set the standards for performance, scalability, and innovation in the year 2025.
Whether you're a startup looking for the best Elixir development company the above-mentioned companies are indeed the role models. Among them, iCreativez remains a clear leader, setting trends and delivering world-class Elixir solutions across the globe.
0 notes
Text
Building a Real-Time Aircraft Tracking System with AWS Lambda, Kinesis, and DynamoDB
Aviation data has always been fascinating. Planes crisscross the globe. Each one sends out tiny bursts of information as it soars through the sky. Thanks to platforms like the OpenSky Network, we can now tap into that data in real time. In this post, I’ll walk you through how I built a fully serverless aircraft tracking system using AWS. The idea is simple. Fetch live aircraft positions. Stream…
0 notes
Text
Serverless Computing Market Growth Analysis and Forecast Report 2032
The Serverless Computing Market was valued at USD 19.30 billion in 2023 and is expected to reach USD 70.52 billion by 2032, growing at a CAGR of 15.54% from 2024-2032.
The serverless computing market has gained significant traction over the last decade as organizations increasingly seek to build scalable, agile, and cost-effective applications. By allowing developers to focus on writing code without managing server infrastructure, serverless architecture is reshaping how software and cloud applications are developed and deployed. Cloud service providers such as Amazon Web Services (AWS), Microsoft Azure, and Google Cloud Platform (GCP) are at the forefront of this transformation, offering serverless solutions that automatically allocate computing resources on demand. The flexibility, scalability, and pay-as-you-go pricing models of serverless platforms are particularly appealing to startups and enterprises aiming for digital transformation and faster time-to-market.
Serverless Computing Market adoption is expected to continue rising, driven by the surge in microservices architecture, containerization, and event-driven application development. The market is being shaped by the growing demand for real-time data processing, simplified DevOps processes, and enhanced productivity. As cloud-native development becomes more prevalent across industries such as finance, healthcare, e-commerce, and media, serverless computing is evolving from a developer convenience into a strategic advantage. By 2032, the market is forecast to reach unprecedented levels of growth, with organizations shifting toward Function-as-a-Service (FaaS) and Backend-as-a-Service (BaaS) to streamline development and reduce operational overhead.
Get Sample Copy of This Report: https://www.snsinsider.com/sample-request/5510
Market Keyplayers:
AWS (AWS Lambda, Amazon S3)
Microsoft (Azure Functions, Azure Logic Apps)
Google Cloud (Google Cloud Functions, Firebase)
IBM (IBM Cloud Functions, IBM Watson AI)
Oracle (Oracle Functions, Oracle Cloud Infrastructure)
Alibaba Cloud (Function Compute, API Gateway)
Tencent Cloud (Cloud Functions, Serverless MySQL)
Twilio (Twilio Functions, Twilio Studio)
Cloudflare (Cloudflare Workers, Durable Objects)
MongoDB (MongoDB Realm, MongoDB Atlas)
Netlify (Netlify Functions, Netlify Edge Functions)
Fastly (Compute@Edge, Signal Sciences)
Akamai (Akamai EdgeWorkers, Akamai Edge Functions)
DigitalOcean (App Platform, Functions)
Datadog (Serverless Monitoring, Real User Monitoring)
Vercel (Serverless Functions, Edge Middleware)
Spot by NetApp (Ocean for Serverless, Elastigroup)
Elastic (Elastic Cloud, Elastic Observability)
Backendless (Backendless Cloud, Cloud Code)
Faundb (Serverless Database, Faundb Functions)
Scaleway (Serverless Functions, Object Storage)
8Base (GraphQL API, Serverless Back-End)
Supabase (Edge Functions, Supabase Realtime)
Appwrite (Cloud Functions, Appwrite Database)
Canonical (Juju, MicroK8s)
Market Trends
Several emerging trends are driving the momentum in the serverless computing space, reflecting the industry's pivot toward agility and innovation:
Increased Adoption of Multi-Cloud and Hybrid Architectures: Organizations are moving beyond single-vendor lock-in, leveraging serverless computing across multiple cloud environments to increase redundancy, flexibility, and performance.
Edge Computing Integration: The fusion of serverless and edge computing is enabling faster, localized data processing—particularly beneficial for IoT, AI/ML, and latency-sensitive applications.
Advancements in Developer Tooling: The rise of open-source frameworks, CI/CD integration, and observability tools is enhancing the developer experience and reducing the complexity of managing serverless applications.
Serverless Databases and Storage: Innovations in serverless data storage and processing, including event-driven data lakes and streaming databases, are expanding use cases for serverless platforms.
Security and Compliance Enhancements: With growing concerns over data privacy, serverless providers are focusing on end-to-end encryption, policy enforcement, and secure API gateways.
Enquiry of This Report: https://www.snsinsider.com/enquiry/5510
Market Segmentation:
By Enterprise Size
Large Enterprise
SME
By Service Model
Function-as-a-Service (FaaS)
Backend-as-a-Service (BaaS)
By Deployment
Private Cloud
Public Cloud
Hybrid Cloud
By End-user Industry
IT & Telecommunication
BFSI
Retail
Government
Industrial
Market Analysis
The primary growth drivers include the widespread shift to cloud-native technologies, the need for operational efficiency, and the rising number of digital-native enterprises. Small and medium-sized businesses, in particular, benefit from the low infrastructure management costs and scalability of serverless platforms.
North America remains the largest regional market, driven by early adoption of cloud services and strong presence of major tech giants. However, Asia-Pacific is emerging as a high-growth region, fueled by growing IT investments, increasing cloud literacy, and the rapid expansion of e-commerce and mobile applications. Key industry verticals adopting serverless computing include banking and finance, healthcare, telecommunications, and media.
Despite its advantages, serverless architecture comes with challenges such as cold start latency, vendor lock-in, and monitoring complexities. However, advancements in runtime management, container orchestration, and vendor-agnostic frameworks are gradually addressing these limitations.
Future Prospects
The future of the serverless computing market looks exceptionally promising, with innovation at the core of its trajectory. By 2032, the market is expected to be deeply integrated with AI-driven automation, allowing systems to dynamically optimize workloads, security, and performance in real time. Enterprises will increasingly adopt serverless as the default architecture for cloud application development, leveraging it not just for backend APIs but for data science workflows, video processing, and AI/ML pipelines.
As open standards mature and cross-platform compatibility improves, developers will enjoy greater freedom to move workloads across different environments with minimal friction. Tools for observability, governance, and cost optimization will become more sophisticated, making serverless computing viable even for mission-critical workloads in regulated industries.
Moreover, the convergence of serverless computing with emerging technologies—such as 5G, blockchain, and augmented reality—will open new frontiers for real-time, decentralized, and interactive applications. As businesses continue to modernize their IT infrastructure and seek leaner, more responsive architectures, serverless computing will play a foundational role in shaping the digital ecosystem of the next decade.
Access Complete Report: https://www.snsinsider.com/reports/serverless-computing-market-5510
Conclusion
Serverless computing is no longer just a developer-centric innovation—it's a transformative force reshaping the global cloud computing landscape. Its promise of simplified operations, cost efficiency, and scalability is encouraging enterprises of all sizes to rethink their application development strategies. As demand for real-time, responsive, and scalable solutions grows across industries, serverless computing is poised to become a cornerstone of enterprise digital transformation. With continued innovation and ecosystem support, the market is set to achieve remarkable growth and redefine how applications are built and delivered in the cloud-first era.
About Us:
SNS Insider is one of the leading market research and consulting agencies that dominates the market research industry globally. Our company's aim is to give clients the knowledge they require in order to function in changing circumstances. In order to give you current, accurate market data, consumer insights, and opinions so that you can make decisions with confidence, we employ a variety of techniques, including surveys, video talks, and focus groups around the world.
Contact Us:
Jagney Dave - Vice President of Client Engagement
Phone: +1-315 636 4242 (US) | +44- 20 3290 5010 (UK)
0 notes
Text
h
Technical Skills (Java, Spring, Python)
Q1: Can you walk us through a recent project where you built a scalable application using Java and Spring Boot? A: Absolutely. In my previous role, I led the development of a microservices-based system using Java with Spring Boot and Spring Cloud. The app handled real-time financial transactions and was deployed on AWS ECS. I focused on building stateless services, applied best practices like API versioning, and used Eureka for service discovery. The result was a 40% improvement in performance and easier scalability under load.
Q2: What has been your experience with Python in data processing? A: I’ve used Python for ETL pipelines, specifically for ingesting large volumes of compliance data into cloud storage. I utilized Pandas and NumPy for processing, and scheduled tasks with Apache Airflow. The flexibility of Python was key in automating data validation and transformation before feeding it into analytics dashboards.
Cloud & DevOps
Q3: Describe your experience deploying applications on AWS or Azure. A: Most of my cloud experience has been with AWS. I’ve deployed containerized Java applications to AWS ECS and used RDS for relational storage. I also integrated S3 for static content and Lambda for lightweight compute tasks. In one project, I implemented CI/CD pipelines with Jenkins and CodePipeline to automate deployments and rollbacks.
Q4: How have you used Docker or Kubernetes in past projects? A: I've containerized all backend services using Docker and deployed them on Kubernetes clusters (EKS). I wrote Helm charts for managing deployments and set up autoscaling rules. This improved uptime and made releases smoother, especially during traffic spikes.
Collaboration & Agile Practices
Q5: How do you typically work with product owners and cross-functional teams? A: I follow Agile practices, attending sprint planning and daily stand-ups. I work closely with product owners to break down features into stories, clarify acceptance criteria, and provide early feedback. My goal is to ensure technical feasibility while keeping business impact in focus.
Q6: Have you had to define technical design or architecture? A: Yes, I’ve been responsible for defining the technical design for multiple features. For instance, I designed an event-driven architecture for a compliance alerting system using Kafka, Java, and Spring Cloud Streams. I created UML diagrams and API contracts to guide other developers.
Testing & Quality
Q7: What’s your approach to testing (unit, integration, automation)? A: I use JUnit and Mockito for unit testing, and Spring’s Test framework for integration tests. For end-to-end automation, I’ve worked with Selenium and REST Assured. I integrate these tests into Jenkins pipelines to ensure code quality with every push.
Behavioral / Cultural Fit
Q8: How do you stay updated with emerging technologies? A: I subscribe to newsletters like InfoQ and follow GitHub trending repositories. I also take part in hackathons and complete Udemy/Coursera courses. Recently, I explored Quarkus and Micronaut to compare their performance with Spring Boot in cloud-native environments.
Q9: Tell us about a time you challenged the status quo or proposed a modern tech solution. A: At my last job, I noticed performance issues due to a legacy monolith. I advocated for a microservices transition. I led a proof-of-concept using Spring Boot and Docker, which gained leadership buy-in. We eventually reduced deployment time by 70% and improved maintainability.
Bonus: Domain Experience
Q10: Do you have experience supporting back-office teams like Compliance or Finance? A: Yes, I’ve built reporting tools for Compliance and data reconciliation systems for Finance. I understand the importance of data accuracy and audit trails, and have used role-based access and logging mechanisms to meet regulatory requirements.
0 notes
Text
Introduction to AWS Data Engineering: Key Services and Use Cases
Introduction
Business operations today generate huge datasets which need significant amounts of processing during each operation. Data handling efficiency is essential for organization decision making and expansion initiatives. Through its cloud solutions known as Amazon Web Services (AWS) organizations gain multiple data-handling platforms which construct protected and scalable data pipelines at affordable rates. AWS data engineering solutions enable organizations to both acquire and store data and perform analytical tasks and machine learning operations. A suite of services allows business implementation of operational workflows while organizations reduce costs and boost operational efficiency and maintain both security measures and regulatory compliance. The article presents basic details about AWS data engineering solutions through their practical applications and actual business scenarios.
What is AWS Data Engineering?
AWS data engineering involves designing, building, and maintaining data pipelines using AWS services. It includes:
Data Ingestion: Collecting data from sources such as IoT devices, databases, and logs.
Data Storage: Storing structured and unstructured data in a scalable, cost-effective manner.
Data Processing: Transforming and preparing data for analysis.
Data Analytics: Gaining insights from processed data through reporting and visualization tools.
Machine Learning: Using AI-driven models to generate predictions and automate decision-making.
With AWS, organizations can streamline these processes, ensuring high availability, scalability, and flexibility in managing large datasets.
Key AWS Data Engineering Services
AWS provides a comprehensive range of services tailored to different aspects of data engineering.
Amazon S3 (Simple Storage Service) – Data Storage
Amazon S3 is a scalable object storage service that allows organizations to store structured and unstructured data. It is highly durable, offers lifecycle management features, and integrates seamlessly with AWS analytics and machine learning services.
Supports unlimited storage capacity for structured and unstructured data.
Allows lifecycle policies for cost optimization through tiered storage.
Provides strong integration with analytics and big data processing tools.
Use Case: Companies use Amazon S3 to store raw log files, multimedia content, and IoT data before processing.
AWS Glue – Data ETL (Extract, Transform, Load)
AWS Glue is a fully managed ETL service that simplifies data preparation and movement across different storage solutions. It enables users to clean, catalog, and transform data automatically.
Supports automatic schema discovery and metadata management.
Offers a serverless environment for running ETL jobs.
Uses Python and Spark-based transformations for scalable data processing.
Use Case: AWS Glue is widely used to transform raw data before loading it into data warehouses like Amazon Redshift.
Amazon Redshift – Data Warehousing and Analytics
Amazon Redshift is a cloud data warehouse optimized for large-scale data analysis. It enables organizations to perform complex queries on structured datasets quickly.
Uses columnar storage for high-performance querying.
Supports Massively Parallel Processing (MPP) for handling big data workloads.
It integrates with business intelligence tools like Amazon QuickSight.
Use Case: E-commerce companies use Amazon Redshift for customer behavior analysis and sales trend forecasting.
Amazon Kinesis – Real-Time Data Streaming
Amazon Kinesis allows organizations to ingest, process, and analyze streaming data in real-time. It is useful for applications that require continuous monitoring and real-time decision-making.
Supports high-throughput data ingestion from logs, clickstreams, and IoT devices.
Works with AWS Lambda, Amazon Redshift, and Amazon Elasticsearch for analytics.
Enables real-time anomaly detection and monitoring.
Use Case: Financial institutions use Kinesis to detect fraudulent transactions in real-time.
AWS Lambda – Serverless Data Processing
AWS Lambda enables event-driven serverless computing. It allows users to execute code in response to triggers without provisioning or managing servers.
Executes code automatically in response to AWS events.
Supports seamless integration with S3, DynamoDB, and Kinesis.
Charges only for the compute time used.
Use Case: Lambda is commonly used for processing image uploads and extracting metadata automatically.
Amazon DynamoDB – NoSQL Database for Fast Applications
Amazon DynamoDB is a managed NoSQL database that delivers high performance for applications that require real-time data access.
Provides single-digit millisecond latency for high-speed transactions.
Offers built-in security, backup, and multi-region replication.
Scales automatically to handle millions of requests per second.
Use Case: Gaming companies use DynamoDB to store real-time player progress and game states.
Amazon Athena – Serverless SQL Analytics
Amazon Athena is a serverless query service that allows users to analyze data stored in Amazon S3 using SQL.
Eliminates the need for infrastructure setup and maintenance.
Uses Presto and Hive for high-performance querying.
Charges only for the amount of data scanned.
Use Case: Organizations use Athena to analyze and generate reports from large log files stored in S3.
AWS Data Engineering Use Cases
AWS data engineering services cater to a variety of industries and applications.
Healthcare: Storing and processing patient data for predictive analytics.
Finance: Real-time fraud detection and compliance reporting.
Retail: Personalizing product recommendations using machine learning models.
IoT and Smart Cities: Managing and analyzing data from connected devices.
Media and Entertainment: Streaming analytics for audience engagement insights.
These services empower businesses to build efficient, scalable, and secure data pipelines while reducing operational costs.
Conclusion
AWS provides a comprehensive ecosystem of data engineering tools that streamline data ingestion, storage, transformation, analytics, and machine learning. Services like Amazon S3, AWS Glue, Redshift, Kinesis, and Lambda allow businesses to build scalable, cost-effective, and high-performance data pipelines.
Selecting the right AWS services depends on the specific needs of an organization. For those looking to store vast amounts of unstructured data, Amazon S3 is an ideal choice. Companies needing high-speed data processing can benefit from AWS Glue and Redshift. Real-time data streaming can be efficiently managed with Kinesis. Meanwhile, AWS Lambda simplifies event-driven processing without requiring infrastructure management.
Understanding these AWS data engineering services allows businesses to build modern, cloud-based data architectures that enhance efficiency, security, and performance.
References
For further reading, refer to these sources:
AWS Prescriptive Guidance on Data Engineering
AWS Big Data Use Cases
Key AWS Services for Data Engineering Projects
Top 10 AWS Services for Data Engineering
AWS Data Engineering Essentials Guidebook
AWS Data Engineering Guide: Everything You Need to Know
Exploring Data Engineering Services in AWS
By leveraging AWS data engineering services, organizations can transform raw data into valuable insights, enabling better decision-making and competitive advantage.
youtube
#aws cloud data engineer course#aws cloud data engineer training#aws data engineer course#aws data engineer course online#Youtube
0 notes
Text
AWS Training in Bangalore: Architecting Fundamentals Explained
Mastering AWS Infrastructure for Scalable Cloud Solutions
Learn AWS Cloud Architecting from the best AWS training institute in Bangalore with Eduleem.
In today’s cloud-driven world, AWS (Amazon Web Services) dominates the industry with its scalability, security, and flexibility. Whether you're a developer, system administrator, or IT professional, mastering AWS infrastructure is key to advancing your career.
By enrolling in an AWS training course in Bangalore, professionals gain expertise in cloud computing, networking, and security. Learning from an industry-recognized AWS institute in Bangalore prepares individuals to architect and deploy fault-tolerant, scalable AWS solutions.
Understanding AWS Infrastructure: Core Components
1️⃣ Compute Services: Powering Cloud Applications
Amazon EC2 (Elastic Compute Cloud): Virtual servers for running applications with flexible scalability.
AWS Lambda: Serverless compute service for automatic scaling without infrastructure management.
2️⃣ Storage Solutions: Secure & Scalable Data Management
Amazon S3 (Simple Storage Service): Object storage for secure and cost-effective data storage.
Amazon EBS (Elastic Block Store): Persistent storage volumes for EC2 instances.
3️⃣ Networking & Security: Ensuring Seamless Connectivity
Amazon VPC (Virtual Private Cloud): Isolated cloud resources for enhanced security.
AWS IAM (Identity & Access Management): Granular access control to AWS resources.
4️⃣ Load Balancing & Auto Scaling: Maximizing Performance
Elastic Load Balancer (ELB): Distributes traffic across multiple EC2 instances for reliability.
Auto Scaling Groups: Automatically adjusts capacity based on demand.
5️⃣ Database Services: Optimizing Data Handling
Amazon RDS (Relational Database Service): Managed database solutions for high availability.
DynamoDB: A NoSQL database for scalable, low-latency applications.
Prepare for the AWS Certified Solutions Architect Exam Want to master AWS Cloud Architecting? Check out our AWS Certified Solutions Architect—Associate Exam: Preparation Guide and get ready for certification!
How AWS Infrastructure Benefits Businesses
📌 Netflix: Uses AWS Auto Scaling and EC2 instances to manage millions of daily video streams. 📌 Airbnb: Leverages Amazon S3, RDS, and CloudFront for seamless global operations. 📌 Spotify: Implements AWS Lambda and DynamoDB for highly efficient music streaming services.
With AWS being the backbone of global enterprises, professionals skilled in AWS cloud computing are in high demand. Learning from the best AWS training institute in Bangalore can open doors to high-paying roles.
Why Choose Eduleem for AWS Training in Bangalore?
Eduleem offers the best AWS training in Bangalore, providing:
🔥 Practical AWS Labs: Work on real-world cloud projects with expert guidance. 📜 Industry-Certified Training: Get trained by AWS-certified professionals. 🔧 Hands-on Cloud Experience: Master AWS EC2, S3, VPC, IAM, and Auto Scaling. 🚀 Career Support & Placement Assistance: Secure top cloud computing jobs.
Whether you're aiming to become a cloud architect, AWS engineer, or solutions architect, learning from the best AWS training institute in Bangalore will help you excel in AWS training and certification.
Conclusion: Build a Future-Proof Career in AWS with Eduleem
AWS expertise is crucial for IT professionals and businesses alike. If you're looking to master AWS architecture, enrolling in the best AWS course in Bangalore is your next step.
🎯 Join Eduleem’s AWS Training Today!
#aws#azure#cloudsecurity#cloudsolutions#devops#eduleem#AWSTraining#CloudComputing#AWS#DevOps#AWSCourse#CloudArchitect#BestAWSTraining#Eduleem#AWSInfrastructure#AmazonWebServices
0 notes
Text

Serverless Computing: The Future of Scalable Cloud Applications
In today’s digital landscape, businesses are shifting towards serverless computing to enhance efficiency and scalability. This revolutionary cloud architecture eliminates the need for managing servers, allowing developers to focus solely on code while cloud providers handle infrastructure provisioning and scaling.
Why Serverless Computing? Serverless computing offers automatic scaling, cost efficiency, and faster time to market. Unlike traditional cloud infrastructure, where businesses pay for pre-allocated resources, serverless follows a pay-as-you-go model, billing only for actual execution time.
How It Enhances Cloud Infrastructure Serverless computing optimizes cloud infrastructure by dynamically allocating resources. Platforms like AWS Lambda, Azure Functions, and Google Cloud Functions enable real-time scaling, making it ideal for unpredictable workloads. This architecture also enhances security, as cloud providers continuously manage updates and patches.
Use Cases and Future Outlook From microservices to event-driven applications, serverless is transforming how businesses operate in the cloud. As AI and IoT adoption rise, serverless architectures will play a crucial role in handling vast data streams efficiently. With cloud infrastructure evolving rapidly, serverless computing is set to become the backbone of next-generation applications.
Conclusion Serverless computing is revolutionizing cloud applications by providing seamless scalability, reducing costs, and enhancing flexibility. As businesses strive for agility, embracing serverless will be key to leveraging the full potential of modern cloud infrastructure.
0 notes
Text
How to Ace a Data Engineering Interview: Tips & Common Questions
The demand for data engineers is growing rapidly, and landing a job in this field requires thorough preparation. If you're aspiring to become a data engineer, knowing what to expect in an interview can help you stand out. Whether you're preparing for your first data engineering role or aiming for a more advanced position, this guide will provide essential tips and common interview questions to help you succeed. If you're in Bangalore, enrolling in a Data Engineering Course in Hebbal, Data Engineering Course in Indira Nagar, or Data Engineering Course in Jayanagar can significantly boost your chances of success by providing structured learning and hands-on experience.
Understanding the Data Engineering Interview Process
Data engineering interviews typically consist of multiple rounds, including:
Screening Round – A recruiter assesses your background and experience.
Technical Round – Tests your knowledge of SQL, databases, data pipelines, and cloud computing.
Coding Challenge – A take-home or live coding test to evaluate your problem-solving abilities.
System Design Interview – Focuses on designing scalable data architectures.
Behavioral Round – Assesses your teamwork, problem-solving approach, and communication skills.
Essential Tips to Ace Your Data Engineering Interview
1. Master SQL and Database Concepts
SQL is the backbone of data engineering. Be prepared to write complex queries and optimize database performance. Some important topics include:
Joins, CTEs, and Window Functions
Indexing and Query Optimization
Data Partitioning and Sharding
Normalization and Denormalization
Practice using platforms like LeetCode, HackerRank, and Mode Analytics to refine your SQL skills. If you need structured training, consider a Data Engineering Course in Indira Nagar for in-depth SQL and database learning.
2. Strengthen Your Python and Coding Skills
Most data engineering roles require Python expertise. Be comfortable with:
Pandas and NumPy for data manipulation
Writing efficient ETL scripts
Automating workflows with Python
Additionally, learning Scala and Java can be beneficial, especially for working with Apache Spark.
3. Gain Proficiency in Big Data Technologies
Many companies deal with large-scale data processing. Be prepared to discuss and work with:
Hadoop and Spark for distributed computing
Apache Airflow for workflow orchestration
Kafka for real-time data streaming
Enrolling in a Data Engineering Course in Jayanagar can provide hands-on experience with these technologies.
4. Understand Data Pipeline Architecture and ETL Processes
Expect questions on designing scalable and efficient ETL pipelines. Key topics include:
Extracting data from multiple sources
Transforming and cleaning data efficiently
Loading data into warehouses like Redshift, Snowflake, or BigQuery
5. Familiarize Yourself with Cloud Platforms
Most data engineering roles require cloud computing expertise. Gain hands-on experience with:
AWS (S3, Glue, Redshift, Lambda)
Google Cloud Platform (BigQuery, Dataflow)
Azure (Data Factory, Synapse Analytics)
A Data Engineering Course in Hebbal can help you get hands-on experience with cloud-based tools.
6. Practice System Design and Scalability
Data engineering interviews often include system design questions. Be prepared to:
Design a scalable data warehouse architecture
Optimize data processing pipelines
Choose between batch and real-time data processing
7. Prepare for Behavioral Questions
Companies assess your ability to work in a team, handle challenges, and solve problems. Practice answering:
Describe a challenging data engineering project you worked on.
How do you handle conflicts in a team?
How do you ensure data quality in a large dataset?
Common Data Engineering Interview Questions
Here are some frequently asked questions:
SQL Questions:
Write a SQL query to find duplicate records in a table.
How would you optimize a slow-running query?
Explain the difference between partitioning and indexing.
Coding Questions: 4. Write a Python script to process a large CSV file efficiently. 5. How would you implement a data deduplication algorithm? 6. Explain how you would design an ETL pipeline for a streaming dataset.
Big Data & Cloud Questions: 7. How does Apache Kafka handle message durability? 8. Compare Hadoop and Spark for large-scale data processing. 9. How would you choose between AWS Redshift and Google BigQuery?
System Design Questions: 10. Design a data pipeline for an e-commerce company that processes user activity logs. 11. How would you architect a real-time recommendation system? 12. What are the best practices for data governance in a data lake?
Final Thoughts
Acing a data engineering interview requires a mix of technical expertise, problem-solving skills, and practical experience. By focusing on SQL, coding, big data tools, and cloud computing, you can confidently approach your interview. If you’re looking for structured learning and practical exposure, enrolling in a Data Engineering Course in Hebbal, Data Engineering Course in Indira Nagar, or Data Engineering Course in Jayanagar can provide the necessary training to excel in your interviews and secure a high-paying data engineering job.
0 notes
Text
Best Java Full Stack Learning Path | Complete Roadmap
The Ultimate Guide to Becoming a Java Full Stack Developer
Java Full Stack Development is a highly sought-after skill, requiring expertise in both front-end and back-end technologies. This guide provides a structured roadmap to help you become a proficient Java Full Stack Developer.
Master Core Java
Before diving into full-stack development, you must have a solid foundation in Core Java. Key topics include syntax, data types, object-oriented programming (OOP), exception handling, collections framework, multithreading, and Java 8+ features such as lambda expressions and streams API. Understanding these concepts will help you write efficient and scalable Java applications.
Learn Front-End Development
A Full Stack Developer must be proficient in front-end technologies to build interactive and user-friendly applications. Start by learning HTML, CSS, and JavaScript, which form the foundation of web development. Then, move on to modern front-end frameworks like React.js and Angular to build dynamic and responsive web applications. Learning TypeScript is also beneficial, especially for Angular development. Platforms like FreeCodeCamp, MDN Web Docs, and YouTube tutorials provide excellent resources to master front-end technologies.
Backend Development with Java & Spring Boot
Java is widely used for back-end development, and Spring Boot is the go-to framework for building enterprise-level applications. Key concepts include JDBC (Java Database Connectivity), Spring MVC (Model-View-Controller), RESTful API development, and Spring Data JPA for database operations. Additionally, learning Spring Security for authentication and authorization, and understanding microservices architecture for scalable applications is essential.
Database Management (SQL & NoSQL)
Databases are a crucial part of any full-stack application. A Full Stack Developer should be proficient in SQL databases (MySQL, PostgreSQL, Oracle) and NoSQL databases (MongoDB, Firebase, Cassandra). Important topics include database normalization, writing SQL queries (JOIN, GROUP BY, INDEXING), and using ORM tools like Hibernate for efficient data handling.
Version Control & Deployment
Version control and deployment skills are essential for collaborative development and efficient software deployment. Learn Git and GitHub to manage your code, collaborate with teams, and track changes. Additionally, understanding containerization with Docker and Kubernetes, CI/CD tools like Jenkins and GitHub Actions, and cloud platforms like AWS, Azure, and Google Cloud will help you deploy applications effectively. Resources like GitHub Docs, DevOps courses, and online tutorials will help you master these tools.
Build Real-World Projects
The best way to reinforce your skills is by building real-world projects. Some project ideas include: ✔ E-commerce Website (Spring Boot + React/Angular) ✔ Blog Application (User Authentication, CRUD Operations) ✔ Library Management System (Spring Boot + MySQL) ✔ Online Book Store (Hibernate ORM + REST API)
We provide industry-driven master’s programs designed to help you excel in high-demand fields.
Start learning today, take one step at a time, and stay updated with the latest trends in Java Full Stack Development.
0 notes
Text
Azure vs. AWS: A Detailed Comparison
Cloud computing has become the backbone of modern IT infrastructure, offering businesses scalability, security, and flexibility. Among the top cloud service providers, Microsoft Azure and Amazon Web Services (AWS) dominate the market, each bringing unique strengths. While AWS has held the position as a cloud pioneer, Azure has been gaining traction, especially among enterprises with existing Microsoft ecosystems. This article provides an in-depth comparison of Azure vs. AWS, covering aspects like database services, architecture, and data engineering capabilities to help businesses make an informed decision.
1. Market Presence and Adoption
AWS, launched in 2006, was the first major cloud provider and remains the market leader. It boasts a massive customer base, including startups, enterprises, and government organizations. Azure, introduced by Microsoft in 2010, has seen rapid growth, especially among enterprises leveraging Microsoft's ecosystem. Many companies using Microsoft products like Windows Server, SQL Server, and Office 365 find Azure a natural choice.
2. Cloud Architecture: Comparing Azure and AWS
Cloud architecture defines how cloud services integrate and support workloads. Both AWS and Azure provide robust cloud architectures but with different approaches.
AWS Cloud Architecture
AWS follows a modular approach, allowing users to pick and choose services based on their needs. It offers:
Amazon EC2 for scalable compute resources
Amazon VPC for network security and isolation
Amazon S3 for highly scalable object storage
AWS Lambda for serverless computing
Azure Cloud Architecture
Azure's architecture is designed to integrate seamlessly with Microsoft tools and services. It includes:
Azure Virtual Machines (VMs) for compute workloads
Azure Virtual Network (VNet) for networking and security
Azure Blob Storage for scalable object storage
Azure Functions for serverless computing
In terms of architecture, AWS provides more flexibility, while Azure ensures deep integration with enterprise IT environments.
3. Database Services: Azure SQL vs. AWS RDS
Database management is crucial for any cloud strategy. Both AWS and Azure offer extensive database solutions, but they cater to different needs.
AWS Database Services
AWS provides a wide range of managed database services, including:
Amazon RDS (Relational Database Service) – Supports MySQL, PostgreSQL, SQL Server, MariaDB, and Oracle.
Amazon Aurora – High-performance relational database compatible with MySQL and PostgreSQL.
Amazon DynamoDB – NoSQL database for low-latency applications.
Amazon Redshift – Data warehousing for big data analytics.
Azure Database Services
Azure offers strong database services, especially for Microsoft-centric workloads:
Azure SQL Database – Fully managed SQL database optimized for Microsoft applications.
Cosmos DB – Globally distributed, multi-model NoSQL database.
Azure Synapse Analytics – Enterprise-scale data warehousing.
Azure Database for PostgreSQL/MySQL/MariaDB – Open-source relational databases with managed services.
AWS provides a more mature and diverse database portfolio, while Azure stands out in SQL-based workloads and seamless Microsoft integration.
4. Data Engineering and Analytics: Which Cloud is Better?
Data engineering is a critical function that ensures efficient data processing, transformation, and storage. Both AWS and Azure offer data engineering tools, but their capabilities differ.
AWS Data Engineering Tools
AWS Glue – Serverless data integration service for ETL workloads.
Amazon Kinesis – Real-time data streaming.
AWS Data Pipeline – Orchestration of data workflows.
Amazon EMR (Elastic MapReduce) – Managed Hadoop, Spark, and Presto.
Azure Data Engineering Tools
Azure Data Factory – Cloud-based ETL and data integration.
Azure Stream Analytics – Real-time event processing.
Azure Databricks – Managed Apache Spark for big data processing.
Azure HDInsight – Fully managed Hadoop and Spark services.
Azure has an edge in data engineering for enterprises leveraging AI and machine learning via Azure Machine Learning and Databricks. AWS, however, excels in scalable and mature big data tools.
5. Pricing Models and Cost Efficiency
Cloud pricing is a major factor when selecting a provider. Both AWS and Azure offer pay-as-you-go pricing, reserved instances, and cost optimization tools.
AWS Pricing: Charges are based on compute, storage, data transfer, and additional services. AWS also offers AWS Savings Plans for cost reductions.
Azure Pricing: Azure provides cost-effective solutions for Microsoft-centric businesses. Azure Hybrid Benefit allows companies to use existing Windows Server and SQL Server licenses to save costs.
AWS generally provides more pricing transparency, while Azure offers better pricing for Microsoft users.
6. Security and Compliance
Security is a top priority in cloud computing, and both AWS and Azure provide strong security measures.
AWS Security: Uses AWS IAM (Identity and Access Management), AWS Shield (DDoS protection), and AWS Key Management Service.
Azure Security: Provides Azure Active Directory (AAD), Azure Security Center, and built-in compliance features for enterprises.
Both platforms meet industry standards like GDPR, HIPAA, and ISO 27001, making them secure choices for businesses.
7. Hybrid Cloud Capabilities
Enterprises increasingly prefer hybrid cloud strategies. Here, Azure has a significant advantage due to its Azure Arc and Azure Stack technologies that extend cloud services to on-premises environments.
AWS offers AWS Outposts, but it is not as deeply integrated as Azure’s hybrid solutions.
8. Which Cloud Should You Choose?
Choose AWS if:
You need a diverse range of cloud services.
You require highly scalable and mature cloud solutions.
Your business prioritizes flexibility and a global cloud footprint.
Choose Azure if:
Your business relies heavily on Microsoft products.
You need strong hybrid cloud capabilities.
Your focus is on SQL-based workloads and enterprise data engineering.
Conclusion
Both AWS and Azure are powerful cloud providers with unique strengths. AWS remains the leader in cloud services, flexibility, and scalability, while Azure is the go-to choice for enterprises using Microsoft’s ecosystem.
Ultimately, the right choice depends on your organization’s needs in terms of database management, cloud architecture, data engineering, and overall IT strategy. Companies looking for a seamless Microsoft integration should opt for Azure, while businesses seeking a highly scalable and service-rich cloud should consider AWS.
Regardless of your choice, both platforms provide the foundation for a strong, scalable, and secure cloud infrastructure in today’s data-driven world.
0 notes
Text
Data Preparation for Machine Learning in the Cloud: Insights from Anton R Gordon
In the world of machine learning (ML), high-quality data is the foundation of accurate and reliable models. Without proper data preparation, even the most sophisticated ML algorithms fail to deliver meaningful insights. Anton R Gordon, a seasoned AI Architect and Cloud Specialist, emphasizes the importance of structured, well-engineered data pipelines to power enterprise-grade ML solutions.
With extensive experience deploying cloud-based AI applications, Anton R Gordon shares key strategies and best practices for data preparation in the cloud, focusing on efficiency, scalability, and automation.
Why Data Preparation Matters in Machine Learning
Data preparation involves multiple steps, including data ingestion, cleaning, transformation, feature engineering, and validation. According to Anton R Gordon, poorly prepared data leads to:
Inaccurate models due to missing or inconsistent data.
Longer training times because of redundant or noisy information.
Security risks if sensitive data is not properly handled.
By leveraging cloud-based tools like AWS, GCP, and Azure, organizations can streamline data preparation, making ML workflows more scalable, cost-effective, and automated.
Anton R Gordon’s Cloud-Based Data Preparation Workflow
Anton R Gordon outlines an optimized approach to data preparation in the cloud, ensuring a seamless transition from raw data to model-ready datasets.
1. Data Ingestion & Storage
The first step in ML data preparation is to collect and store data efficiently. Anton recommends:
AWS Glue & AWS Lambda: For automating the extraction of structured and unstructured data from multiple sources.
Amazon S3 & Snowflake: To store raw and transformed data securely at scale.
Google BigQuery & Azure Data Lake: As powerful alternatives for real-time data querying.
2. Data Cleaning & Preprocessing
Cleaning raw data eliminates errors and inconsistencies, improving model accuracy. Anton suggests:
AWS Data Wrangler: To handle missing values, remove duplicates, and normalize datasets before ML training.
Pandas & Apache Spark on AWS EMR: To process large datasets efficiently.
Google Dataflow: For real-time preprocessing of streaming data.
3. Feature Engineering & Transformation
Feature engineering is a critical step in improving model performance. Anton R Gordon utilizes:
SageMaker Feature Store: To centralize and reuse engineered features across ML pipelines.
Amazon Redshift ML: To run SQL-based feature transformation at scale.
PySpark & TensorFlow Transform: To generate domain-specific features for deep learning models.
4. Data Validation & Quality Monitoring
Ensuring data integrity before model training is crucial. Anton recommends:
AWS Deequ: To apply statistical checks and monitor data quality.
SageMaker Model Monitor: To detect data drift and maintain model accuracy.
Great Expectations: For validating schemas and detecting anomalies in cloud data lakes.
Best Practices for Cloud-Based Data Preparation
Anton R Gordon highlights key best practices for optimizing ML data preparation in the cloud:
Automate Data Pipelines – Use AWS Glue, Apache Airflow, or Azure Data Factory for seamless ETL workflows.
Implement Role-Based Access Controls (RBAC) – Secure data using IAM roles, encryption, and VPC configurations.
Optimize for Cost & Performance – Choose the right storage options (S3 Intelligent-Tiering, Redshift Spectrum) to balance cost and speed.
Enable Real-Time Data Processing – Use AWS Kinesis or Google Pub/Sub for streaming ML applications.
Leverage Serverless Processing – Reduce infrastructure overhead with AWS Lambda and Google Cloud Functions.
Conclusion
Data preparation is the backbone of successful machine learning projects. By implementing scalable, cloud-based data pipelines, businesses can reduce errors, improve model accuracy, and accelerate AI adoption. Anton R Gordon’s approach to cloud-based data preparation enables enterprises to build robust, efficient, and secure ML workflows that drive real business value.
As cloud AI evolves, automated and scalable data preparation will remain a key differentiator in the success of ML applications. By following Gordon’s best practices, organizations can enhance their AI strategies and optimize data-driven decision-making.
0 notes