#This is an example of how dangerous AI is in developing algorithms etc and the role of the Western press media and general discourse is
Explore tagged Tumblr posts
Text

#Artificial intelligence#AI#chatgpt#This is an example of how dangerous AI is in developing algorithms etc and the role of the Western press media and general discourse is#Palestine#Israel
33 notes
·
View notes
Text
The Pros and Cons of Artificial Intelligence: What You Need to Know

Artificial intelligence (AI) pervades our daily lives these days. It is the developing assistant on phones with Siri, as well as self-driving cars. AI is changing the way we live and work, but with the same strength comes weakness. In this blog, we will break down pros and cons of applying AI in simple terms, highlighting how it affects our everyday lives and businesses.
The Pros of Artificial Intelligence
1. Increased Efficiency and Speed
It is fast. In all applications, AI performs amazingly quick while processing information much faster than humans. For instance, it can analyze huge datasets within seconds and help in decision-making for the businesses concerned. This gives the scope of completing tasks that would take an hour or day to be completed by a person within a few minutes when done by AI.
AI would, for instance, allow doctors to analyze the high volume of medical data quickly to reach a diagnosis. In business, AI tools would take many roles such as tracking inventory, processing payments, and even handling customer service queries.

2. 24/7 Availability
AI does not sleep, eat, or get breaks. This makes it very suitable for those things that have to be performed all the time-a nice example would be customer service, monitoring systems, etc. For example, chatbots with AI capabilities can always answer customers' questions day and night. Instant support enhances the customer experience without requiring that human workers be present at any particular time.
3. Accuracy and Precision
AI structures are designed to comply with strict rules and algorithms, which could result in fewer mistakes. For example, AI used in production can look into merchandise for defects greater correctly than human employees. In fields like finance, AI can assist hit upon fraudulent transactions with the aid of spotting styles that people may omit.
This stage of precision is specially essential in fitness care, in which medical doctors' errors can show to be the difference among lifestyles and death. AI could assist medical doctors better analyze clinical snap shots or expect destiny fitness situations, saving lives within the manner.
4. Cost Savings
Although establishing an AI system is expensive, it will save a business money in the long term. AI can reduce human labor in tasks that require repetition like data entry or simple consumer support. With more human workers being engaged to perform their creative and complex tasks, the company can rely on AI in repetitive operations.
For example, in production, robots take over the meeting line so there's less danger of errors and more productivity. Consequently, this cuts costs due to the fact the work simplest requires fewer employees to do such repetitive techniques.
5. Personalization
AI is wonderful at studying statistics to provide customized stories for users. Think about how Netflix recommends movies or how Amazon suggests products based to your surfing records. This is AI at paintings, the use of information to are expecting what you’ll like and presenting personalized hints.
For businesses, this capability to customize reports can assist enhance patron satisfaction and loyalty. By records what clients need, groups can offer better products and services.
The Cons of Artificial Intelligence
1. Job Losses
Perhaps the greatest worry surrounding AI is that it might necessarily displace jobs. With AI able to fill repetitive functions, many jobs requiring guide labor-most data entry and customer service jobs, for example-would get replaced via machines. This ought to mean fewer job opportunities for employees, mainly in industry-particular sectors wherein automation is more and more being adopted.
For example, self-driving cars may be able to reduce the need for truck drivers, automated checkouts in stores also want to reduce banking operations AI may even make some jobs obsolete while tech and account for the possible alternatives in research.
2. Lack of Human Touch
While AI may be rapid and green, it regularly lacks the emotional intelligence that people bring to sure situations. For example, at the same time as a chatbot can answer customer questions quick, it may not continually be able to understand the tone or feelings of the purchaser. In conditions where empathy or expertise is wanted, AI can fall short.
For organizations, this will come to mean that while the AI is going to assume simple job tasks, human workers will still be necessary in jobs like customer service, healthcare, and education, as personal interaction is important.

3. High Initial Cost
While AI can keep cash ultimately, the preliminary setup fee can be excessive. Building or buying AI structures requires a tremendous investment, specially for small companies. This can include the value of software program, hardware, and hiring specialists to set up and preserve the gadget.
For smaller organizations, these prematurely costs may be a major barrier to adopting AI. Even for large organizations, the funding required to hold AI systems up-to-date can be steeply-priced.
4. Dependence on Data
AI structures are best as precise as the records they are given. If the data is incomplete or biased, the AI’s selections also can be incorrect. For example, if an AI gadget is educated with biased records, it would make decisions that are unfair or discriminatory.
In fields like hiring, AI equipment might unintentionally desire sure candidates over others if they are educated on biased records. This can cause unfair practices and ignored possibilities for certified people.
5. Security Risks
AI systems are vulnerable to cyberattacks. Hackers have to take gain of AI systems to thieve sensitive statistics or manipulate selection-making approaches. For example, a hacker may also need to goal an AI-powered financial gadget to scouse borrow money or manage stock fees.
As AI will become extra incorporated into our lives and groups, the risks associated with AI safety boom. Ensuring that AI structures are stable from hacking is an ongoing mission for companies and governments.
Conclusion: The Future of AI
Artificial Intelligence gives many benefits, from improving efficiency and accuracy to saving prices and providing personalized reviews. However, there also are demanding situations to do not forget, which include the threat of activity losses, the dearth of human touch, and the need for steady, unbiased records.
As AI keeps adapting, it is going to be essential for organizations and society to discover a balance among the usage of AI to enhance processes at the same time as additionally addressing its capacity downsides. The key to a successful future with AI is understanding each its pros and cons and using it in a way that advantages anybody.
In the cease, AI is a tool, and how we use it'll decide its effect on our lives and organizations. By being privy to each the best and the terrible, we are able to harness the power of AI to make our world smarter and additional inexperienced.
0 notes
Text
The Role of AI in Revolutionizing Autonomous Driving for Electric Vehicles
Artificial Intelligence (AI) has been a revolutionary change factor in most industries, including the automotive and electric vehicle industries. The use of AI for the Automotive and EV Industry is one of the causes of much revolutionary change, especially in the aspects of autonomous driving system development. Electric vehicles can run efficiently, safely, and sustainably because AI can analyze huge volumes of data to recognize patterns in time for real-time decisions.
Electric cars continue to dominate headlines and capture market shares globally but AI is leading the charge in terms of pioneering advancements in autonomy and operational excellence in this sector. This article delves into how AI plays a critical role in the rebirth of the car and EV business sectors with a special focus on autonomous driving.
AI-Powered Autonomous Driving: The Future of Mobility
With autonomous driving in the majority of electric vehicles, the push relies very much on AI-driven technologies of machine learning, computer vision, and real-time data analytics. These combine to empower vehicles to look out into the world, comprehend their driving environment, and make decisions about improving efficiency and safety.
At the heart of it is AI video analytics, utilizing cameras, sensors, and algorithms that can recognize objects, read road signs, and monitor behavior by other road users. Scenario-based AI allows electric cars to analyze complex situations on the road, recognize obstacles, and react to possible dangers in a way to make autonomous driving much more reliable.
Enhancing Safety Through AI
One of the core areas where AI makes all the difference in autonomous driving relates to safety. Through the application of AI into automobile and electric vehicle design, latent hazards can be monitored in real-time - collision threats, pedestrian crossings, erratic driving behaviors, etc. AI systems are designed to identify dangerous conditions and then act to counter them in enough time so that hazards do not come to fruition.
For example, AI-powered cameras can see the surroundings of the vehicle, notify the driver about pedestrians, vehicles, and cycles, and intervene independently where necessary. On autonomous safety for vehicles, AI can indicate when there is a deviation in Personal Protective Equipment and monitor the safety of workers on manufacturing floors.
Additionally, it can, with AI, view all the complicated driving environments such as highways and roads in cities more clearly. It is through combining LIDAR with radar sensors that systems powered by AI will enable vehicles to enter challenging and congested driving conditions carefully. Such precision is needed for autonomous electric vehicles to operate in any environment safely.
AI and Process Optimization in Autonomous Electric Vehicles
AI is not just a safety enabler but, more importantly, an optimization factor for the overall efficiency of autonomous electric vehicles. The AI systems integrated within these vehicles are constantly gathering and processing data related to driving styles, road conditions, and vehicle performance. Through this mechanism, AI identifies segments where energy consumption can be optimized, hence improving the overall sustainability aspect of EVs.
For instance, through predictive analytics based on artificial intelligence, it would be possible to identify the best way of driving in a car that is available at any given time, under real-time traffic conditions, thereby reducing energy consumption and optimizing the battery. AI can monitor the health of critical components to predict when they need to be maintained or even when it is about to break down. Apart from the increase in length of life, there would be a reduction in unplanned downtime, contributing to the sustainability of the industry for electric vehicles.
The Future of AI in Autonomous Electric Vehicles
The future of autonomous electric vehicles looks bright, especially with continuous development in AI. Success in the area will be guaranteed once the electric vehicles are infused with features like real-time data-driven decision-making and smart navigation systems, which will completely make electric vehicles autonomous and minimize human inputs into their operations. Transportation as a result will be transformed, being convenient, safe, and very efficient.
An even bigger role will be played by AI in this area of sustainability. Autonomous electric vehicles will certainly be even more ecologically friendly to the earth as the world looks to reduce carbon emissions and try reversing the effects of climate change by optimizing energy consumption and reducing waste.
Conclusion
In a nutshell, the role of AI in revolutionizing autonomous driving for electric vehicles is invaluable. AI can be both a safety and process optimization tool while at the same time being a catalyst for innovation in the automotive and EV industry as a whole, forcing it to transform into this new manufacturing industry. Integration of AI video analytics and real-time monitoring systems will ensure that autonomous vehicles are fully prepared for any challenge of modern drive scenarios but most safely and efficiently. viAct leads the front in AI solutions for industrial landscapes, offering cutting-edge technology that is helping automotive and EV manufacturers create a proactive and sustainable approach to the future of mobility.
Visit Our Social Media Details :-
Facebook :- viactai
Linkedin :- viactai
Twitter :-aiviact
Youtube :-@viactai
Instagram :-viactai/
Blog Url :-
Generative AI in Construction: viAct enabling "See the Unseen" with LLM in operations
Integrated Digital Delivery (IDD): viAct creating connected construction jobsites in Singapore
0 notes
Text
Future of Artificial Intelligence !

Many scientist have predicted that AI could be dangerous because if machines started thinking better than humans, then where would humans be?
Scared?
You don’t need to First of all you need to know: What is Artificial Intelligence?
Artificial Intelligence is the ability of computers or computer controlled robots that performs human tasks. The main feature of AI is its ability to take actions and decisions that have the best chance of achieving specified goals.
Goals of AI are: learning, reasoning and perception.
The future of AI is exciting but not scary. In this article we will discuss about the future of AI:
There will be plenty of work-AI can never replace men. A computer can think itself, but it is still based on some instructions provided by the humans.
AI would create the numerous job opportunities like of Man-Machine Teaming Manager, AI Business Development Manager, Data Detective, AI-Assisted Healthcare Technician etc.
1. Machine Learning:
Ø Google is expected to exploit potential machine learning to new levels.
Ø Companies such as Amazon and Flipkart would use the machine learning algorithms that will further assist users in making decisions on what to purchase based on predictive performance.
2. Deep Learning:
Ø Some great examples of deep learning are self-driving cars, computer vision, face recognition on screen, and Facebook (tags). The future is therefore extremely bright, and this field of AI needs people with a lot of innovative thought and reasoning.
3. Robotics:
Ø This is the most exciting AI field in the future that will be taking big strides. Robotics engineers are continually thinking about how robots can be built that behave, communicate and think like humans.
Ø Robotics will transform the future in many fields: education, healthcare, office and home.
There are plenty of advancements in the field of AI and the future looks promising too, so that we humans can use this technology with care and caution to our advantage.
#deeplearning#digital marketing#digital transformation#digital world#robots#artificialintelligence#machine learning
3 notes
·
View notes
Text
Automobile robotics: Applications and Methods - Towards Robotics
Automobile robotics: Applications and Methods
Introduction:
An automobile robot is a software-controlled machine that uses sensors and other technology to identify its environment and act accordingly. They work by combining artificial intelligence (AI) with physical robotic elements like wheels, tracks, and legs. Mobile robots are gaining increasing popularity across various business sectors. They assist with job processes and perform activities that are difficult or hazardous for human employees.
Structure and Methods:
The mechanical structure must be managed to accomplish tasks and attain its objectives. The control system consists of four distinct pillars: vision, memory, reasoning, and action. The perception system provides knowledge about the world, the robot itself, and the robot-environment relationship. After processing this information, sending the appropriate commands to the actuators that move the mechanical structure. Once the environment, and destination, or purpose of the robot is known, the robot’s cognitive architecture must plan the path that the robot must take to attain its goals.
The cognitive architecture reflects the purpose of the robot, its environment, and the way they communicate. Computer vision and identification of patterns are used to track objects. Mapping algorithms are used for the construction of environment maps. Motion planning and other artificial intelligence algorithms could eventually be used to determine how the robot should interact with each other. A planner, for example, might determine how to achieve a task without colliding with obstacles, falling over, etc. Artificial intelligence is called upon to play an important role in the treatment of all the information the robot collects to give the robot orders in the next few years. Nonlinear dynamics found in robots. Nonlinear control techniques utilize the knowledge and/or parameters of the system to reproduce its behavior. Complex algorithms benefit from nonlinear power, estimation, and observation.
Following are best-known control methods:
Computed torque control methods: A computed torque is defined using the second position derivatives, target positions, and mass matrix expressed in a conventional way with explicit gains for the proportional and derivative errors (feedback).
Robust control methods: These methods are similar to simulated methods of torque control, with the addition of a feedback variable depending on an arbitrarily small positive design constant E.
Sliding mode control methods: Increasing the controller frequency may be used to increase the system’s steady error. Taken to the extreme, the controller requires infinite actuator bandwidth if the design parameter E is set to zero, and the state error vanishes. This discontinuous controller is called a controller on sliding mode.
Adaptive methods: Awareness of the exact robot dynamics is relaxed compared to previous methods and this approach uses a linear assumption of parameters. These methods use feed-forward terminology estimation, thereby reducing the requirement for high gains and high frequency to compensate for uncertainties/disturbance in the dynamic model.
Invariant manifold method: the dynamic equation is broken down into components to perform functions independently.
Zero moment point control: This is a concept for humanoid robots associated, for example, with the control and dynamics of legged locomotion. It identifies the point around which no torque is generated by the dynamic reaction force between the foot and the ground, that is, the point at which the total horizontal inertia and gravity forces are equal to zero. This definition means the contact patch is planar and has adequate friction to prevent the feet from sliding
Navigation Methods: Navigation skills are the most important thing in the field of automobile robotics. The aim is for the robot to move in a known or unknown environment from one place to another, taking into account the sensor values to achieve the desired targets. This means that the robot must rely on certain factors such as perception (the robot must use its sensors to obtain valuable data), localization (the robot must use its sensors to obtain valuable data) The robot must be aware of its position and configuration, cognition (the robot must decide what to do to achieve its objectives), and motion control (the robot must calculate the input forces on the actuators to achieve the desired trajectory).
Path, trajectory, and motion planning:
The aim of path planning is to find the best route for the mobile robot to meet the target without collision, allowing a mobile robot to maneuver through obstacles from an initial configuration to a specific environment. It neglects the temporal evolution of motion. It does not consider velocities and accelerations. A more complete study is trajectory planning, with broader goals.
Trajectory planning involves finding the force inputs (control u (t)) to push the actuators so that the robot follows a q (t) trajectory which allows it to go from the initial to the final configuration while avoiding obstacles. To plan the trajectory it takes into account the dynamics and physical characteristics of the robot. In short, both the temporal evolution of the motion and the forces needed to achieve that motion are calculated. Most path and trajectory planning techniques are shared.
Applications of Automobile robotics:
A mobile robot’s core functions include the ability to move and explore, carry payloads or revenue-generating cargo, and complete complex tasks using an onboard system, such as robotic arms. While the industrial use of mobile robots is popular, particularly in warehouses and distribution centers, its functions may also be applied to medicine, surgery, personal assistance, and safety. Exploration and navigation at ocean and space are also among the most common uses of mobile robots.
Mobile robots are used to access areas, such as nuclear power plants, where factors such as high radiation make the area too dangerous for people to inspect themselves and monitor. Current automobile robotics, however, do not build robots that can withstand high radiation without having to compromise their electronic circuitry. Attempts are currently being made to invent mobile robots to deal specifically with those situations.
Other uses of mobile robots include:
shoreline exploration of mines
repairing ships
a robotic pack dog or exoskeleton to carry heavy loads for military troopers
painting and stripping machines or other structures
robotic arms to assist doctors in surgery
manufacturing automated prosthetics that imitate the body’s natural functions and
patrolling and monitoring applications, such as determining thermal and other environmental conditions
Cons and Pros of automobile robotics:
Their machine vision capabilities are a big benefit of automobile robots. The complex array of sensors that mobile robots use to detect their surroundings allows them to observe their environment accurately in real-time. That is especially valuable in constantly evolving and shifting industrial settings.
Another benefit lies in the onboard information system and AI used by AMRs. The autonomy provided by the ability of the mobile robots to learn their surroundings either through an uploaded blueprint or by driving around and developing a map allows for quick adaptation to new environments and helps in the continued pursuit of industrial productivity. Furthermore, mobile robots are quick and flexible to implement. These robots can make their own path for motion.
Some of the disadvantages are following
load-carrying limitation
More expensive and complex.
Communication challenges between robot and endpoint
Looking ahead in the future, manufacturers are trying to find more non-industrial applications for automobile robotics. Current technology is a mix of hardware, software, and advanced machine learning; it is considered solution-focused and rapidly evolving. AMRs are still struggling to move from one point to another; it is important to enhance spatial awareness. The design of the Simultaneous Localization and Mapping (SLAM) algorithm is one invention that is trying to solve this problem.
Hope you enjoyed this article. You may also want to check out my article on the concepts and basics of mobile robotics.
1 note
·
View note
Text
Corporate Charter, Governance, and Artificial Intelligence
I came across an interesting article a few months ago in Huffington Post. It looked at charters of incorporation (the document[s] which layout how a corporation is structure and will operate) as the first historical instances of “Artificial Intelligence.”
Both organizations will act aggressively in their own interest to pursue a specific goal (for corporations this is profit, for governments it is perpetuity and growth). They will not hesitate to oust even their own Chief Executive if that individual hinders these goals in any way (look at Harvey Weinstein as an example); most governments have an impeachment process in place.
The article from Huffington Post was what I initially read. I am especially interested in the hot take from 2013, though from a post titled The Singularity Already Happened; We Got Corporations:
It is pretty clear to anyone who’s paying attention that 1. a marketplace regime of firms dedicated to maximizing profit has—broadly speaking—added a lot of value to the world 2. there are a lot of important cases where corporate profit maximization causes harm to humans 3. corporations are—broadly speaking—really good at ensuring that their needs are met.
To understand the comparison, it is necessary to consider corporations as a form of government. Government, in the general sense of the word, is just a foundation of processes to maximize the outcome of decision-making. Governments and Corporations are thus both forms of AI.
The HuffPo articles suggest that as time goes on, more of these processes and departments become digital, the line between Corporate Hierarchies and AI will become even more blurred:
Corporations took full advantage of their new-found dominance, influencing state legislatures to issue charters in perpetuity giving them the right to do anything not explicitly prohibited by law. The tipping point in their path to domination came in 1886 when the Supreme Court designated corporations as “persons” entitled to the protections of the Fourteenth Amendment, which had been passed to give equal rights to former slaves enfranchised after the Civil War. Since then, corporate dominance has only been further enhanced by law, culminating in the notorious Citizen United case of 2010, which lifted restrictions on political spending by corporations in elections.
In fact, the current U.S. cabinet represents the most complete takeover yet of the U.S. government by corporations, with nearly 70% of top administration jobs filled by corporate executives.
We can see this happening over the decades, and especially today. Most departments within a corporation are semi-autonomous agencies, acting at the discretion of those above them. Decisions are made by a consensus of board members, and those decisions trickle on down to the respective and appropriate departments. Much of this process is digital already. Soon, the article predicts, these processes might be fully automated altogether.
Blogger mtraven points out in their article “Hostile AI: You’re soaking in it!” the following observation:
Corporations are driven by people — they aren’t completely autonomous agents. Yet if you shot the CEO of Exxon or any of the others, what effect would it have? Another person of much the same ilk would swiftly move into place, much as stepping on a few ants hardly effects an anthill at all. To the extent they don’t depend on individuals, they appear to have an agency of their own. And that agency is not a particularly human one — it is oriented around profit and growth, which may or may not be in line with human flourishing.
Corporations are at least somewhat constrained by the need to actually provide some service that is useful to people. Exxon provides energy, McDonald’s provides food, etc. The exception to this seems to be the financial industry.
The final take, then, is that financial corporations, specifically, have become a sort-of Artificial Intelligence---they are all seeking the same goals with the same input (human time, labor, and wealth) and output (creating more wealth from debt). Although it’s no SkyNet, the financial industry is “effectively independent of human control, which makes it just as dangerous”.
And so we must consider exactly what happens when a corporation stops acting in human interests, and starts acting in its own corporate interests (self-sustainability, profit). Are the interests of the individual consumer 100% aligned with the interests of a Multinational Corporation (MNC)?
In his 2017 report Algorithmic Entities, Lynn LoPucki argues that “AEs are inevitable because they have three advantages over human-controlled businesses. They can act and react more quickly, they don’t lose accumulated knowledge through personnel changes, and they cannot be held responsible for their wrongdoing in any meaningful way.”
In a 2014 article, Professor Shawn Bayern demonstrated that anyone can confer legal personhood on an autonomous computer algorithm by putting it in control of a limited liability company. Bayern’s demonstration coincided with the development of “autonomous” online businesses that operate independently of their human owners—accepting payments in online currencies and contracting with human agents to perform the off-line aspects of their businesses ...
This Article argues that algorithmic entities—legal entities that have no human controllers—greatly exacerbate the threat of artificial intelligence. Algorithmic entities are likely to prosper first and most in criminal, terrorist, and other anti-social activities because that is where they have their greatest comparative advantage over human-controlled entities. Control of legal entities will contribute to the threat algorithms pose by providing them with identities. Those identities will enable them to conceal their algorithmic natures while they participate in commerce, accumulate wealth, and carry out anti-social activities.
Four aspects of corporate law make the human race vulnerable to the threat of algorithmic entities. First, algorithms can lawfully have exclusive control of not just American LLC’s but also a large majority of the entity forms in most countries. Second, entities can change regulatory regimes quickly and easily through migration. Third, governments—particularly in the United States—lack the ability to determine who controls the entities they charter and so cannot determine which have non-human controllers. Lastly, corporate charter competition, combined with ease of entity migration, makes it virtually impossible for any government to regulate algorithmic control of entities.
Although its not overtly named, LoPucki’s work actually looked at a recent innovation known as a Decentralized Autonomous Organizations (DAO). DAOs provide a new decentralized business model for organizing both commercial and non-profit enterprises. This is made possible only by the advent of blockchain technologies which provide the immutable ledger’s necessary to bring this type of decentralized decision-making process into existence.
LoPucki's white paper became a top hit on the SSRN site because---as he notes in this a blog post---anti-terrorism people are concerned about it:
One of the scariest parts of this project is that the flurry of SSRN downloads that put this manuscript at the top last week apparently came through the SSRN Combating Terrorism eJournal. That the experts on combating terrorism are interested in my manuscript seems to me to warrant concern.
For those of you interested in the coalescence of artificial intelligence, autonomous organizations, governance, and corporations here is a list of information I found during my impromptu research for this post.
Creating Friendly AI 1.0: The Analysis and Design of Benevolent Goal Architectures (2001)
Hostile AI: You’re soaking in it! (Feb 2013)
The Singularity Already Happened; We Got Corporations (March 2013)
Algorithmic Entities (April 2017)
AI Has Already Taken Over. It’s Called the Corporation (November 2017)
What happens if you give an AI control over a corporation? (March 2018)
#artificial intelligence#decentralized autonomous organizations#algorithmic entities#corporations#statism#mine
16 notes
·
View notes
Text
17 Prime Data Science Purposes & Examples You Need To Know 2021

An enterprise analyst profile combines a little bit of each to help companies make information-pushed choices. Hard expertise required for the job include information mining, machine studying, deep studying, and the ability to integrate structured and unstructured data. Experience with statistical analysis techniques, such as modeling, clustering, data visualization and segmentation, and predictive analysis, are additionally a giant part of the roles. Data scientists create them by running machine studying, information mining or statistical algorithms towards knowledge sets to predict business scenarios and sure outcomes or behavior. Though the position of a data analyst varies depending on the corporate, normally, these professionals collect knowledge, process that knowledge and perform statistical evaluation using normal statistical instruments and strategies.
These algorithms can catch fraud faster and with higher accuracy than people, merely due to the sheer quantity of data generated every day. For example, you might collect data about a customer each time they go to your web site or brick-and-mortar store, add an merchandise to their cart, complete a buy order, open an email, or engage with a social media publication. After making certain the data from every source is correct, you have to mix it in a course referred to as data wrangling. This may involve matching a customer’s email address to their credit card data, social media handles, and purchase identifications.
It may also be used to optimize customer success and subsequent acquisition, retention, and progress. So robust soft skills, significant communication and public talking capacity are key. In addition, results ought to all the time be related back to the enterprise goals that spawned the project in the first place.
There's also deep studying, a more superior offshoot of machine learning that primarily uses artificial neural networks to analyze giant units of unlabeled information. In another article, Cognilytica's Schmelzer explains the connection between Data Science, machine studying and AI, detailing their totally different characteristics and the way they are often mixed in analytics functions. From an operational standpoint, Data Science initiatives can optimize administration of supply chains, product inventories, distribution networks and customer support. To a more fundamental degree, they point to increased efficiency and decreased costs. data science course in hyderabad additionally permits corporations to create enterprise plans and techniques which might be based mostly on informed evaluation of customer habits, market developments and competition. Without it, businesses might miss alternatives and make flawed selections.
I am trying to find out the greatest career path for me in huge information or enterprise intelligence. Predictive causal analytics – If you need a mannequin that may predict the chances of a selected event in the future, you should apply predictive causal analytics. Say, if you're offering money on credit score, then the likelihood of consumers making future credit funds on time is a matter of concern for you. Here, you'll have the ability to construct a model that can carry out predictive analytics on the fee historical past of the customer to foretell if the future funds shall be on time or not. Machine studying delivers correct results derived via the evaluation of huge knowledge sets.
With Data Science, vast volumes and numbers of knowledge can practice models better and extra successfully to indicate more precise suggestions. A lot of firms have fervidly used this engine / system to advertise their merchandise / recommendations in accordance with user’s interest and relevance of information. Internet giants like Amazon, Twitter, Google Play, Netflix, Linkedin, imdb and plenty of more use this system to enhance personal expertise.
Here is considered one of my favourite Data Scientist Venn diagrams created by Stephan Kolassa. You’ll notice that the primary ellipses in the diagram are very related to the pillars given above. What occupation did Harvard name the Sexiest Job of the twenty first Century? There remains no consensus on the definition of Data Science and it's thought-about by some to be a buzzword. Signal processing is any technique used to investigate and enhance digital alerts. This picture illustrates the private and skilled attributes of a Data Scientist.
Read up on what a knowledge cloth is and the means it will use AI and ML to transform information structure and create a new competitive advantage for companies that use it. These corporations have plenty of open Data Science jobs out there right now. Here are some examples of how Data Science is reworking sports activities beyond baseball. While both biking and public transit can curb driving-related emissions, Data Science can do the same by optimizing highway routes.
Some of the best examples of speech recognition products are Google Voice, Siri, Cortana and so on. Using speech-recognition characteristics, even if you aren’t in a position to type a message, your life wouldn’t cease. However, at occasions, you would notice, speech recognition doesn’t perform precisely. Procedures such as detecting tumors, artery stenosis, organ delineation employ varied methods and frameworks like MapReduce to find optimum parameters for duties like lung texture classification.
It’s additionally very useful in that Data Scientists typically should current and communicate results to key stakeholders, including executives. The greatest thing that every one Data Science tasks have in widespread use is the need to make use of tools and software programs to analyze the concerned algorithms and statistics, because the size of the pool of knowledge they're working with is so huge. Data scientist is doubtless considered one of the highest-paying job titles, and there's a high demand for professionals who're in a place to fill the assorted duties of the role. On the other hand, citizen Data Scientists may be hobbyists or volunteers, or might obtain a small amount of compensation for the work they do for major corporations.
Starting from the display banners on various web sites to the digital billboards at the airports – nearly all of them are decided through the use of data science algorithms. Data scientists are professionals who source, gather and analyse large sets of information. Most of the business decisions at present are based mostly on insights drawn from analysing data, that is why a Data Scientist is crucial in today’s world.
Please discuss with the Payment & Financial Aid page for additional information. No, all of our packages are 100 percent on-line, and available to participants no matter their location. Our platform options include quick, highly produced videos of HBS faculty and guest enterprise experts, interactive graphs and workout routines, cold calls to keep you engaged, and opportunities to contribute to a vibrant on-line group. Catherine Cote is an advertising coordinator at Harvard Business School Online. Prior to joining HBS Online, she worked at an early-stage SaaS startup where she found her passion for writing content, and at a digital consulting company, where she specialized in search engine optimization.
Data analysts are often given questions and targets from the top down, perform the analysis, after which report their findings. No matter what path is taken to learn, data scientist’s ought to have advanced quantitative information and extremely technical skills, primarily in statistics, mathematics, and pc science. One necessary thing to debate are off-the-shelf data science platforms and APIs. One may be tempted to suppose that these can be used relatively simply and thus not require important expertise in sure fields, and therefore not require a robust, well-rounded Data Scientist. Below is a diagram of the GABDO Process Model that I created and introduced in my e-book, AI for People and Business.
Before you start the project, it could be very important to perceive the various specifications, requirements, priorities and required price range. Data scientists are those that crack advanced information issues with their sturdy experience in certain scientific disciplines. They work with a quantity of components associated with arithmetic, statistics, computer science, etc . Traditionally, the data that we had was principally structured and small in size, which might be analyzed through the use of simple BI tools. In addition, Google offers you the choice to search for images by importing them. In their newest update, Facebook has outlined the extra progress they’ve made in this space, making particular notice of their advances in image recognition accuracy and capacity.
The recommendations are made based mostly on earlier search outcomes for a person. But there are many different search engines like Google, Yahoo, Bing, Ask, AOL, and so forth. All these search engines make use of Data Science algorithms to ship one of the best results for our searched question in a fraction of seconds. Considering the fact that, Google processes greater than 20 petabytes of knowledge every single day. Over the years, banking firms learned to divide and conquer information by way of buyer profiling, previous expenditures, and other essential variables to analyze the probabilities of danger and default. Yes, Data Science is a good profession path, in fact, one of many very best ones now.
If you’re new to the world of data and want to bolster your abilities, two phrases you’re prone to encounter are “data analytics” and “data science.” While these terms are associated, they discuss different things. Below is a summary of what each word means and the means it applies in business. “In this world of massive data, primary data literacy—the ability to research, interpret, and even question data—is an increasingly priceless ability,” says Harvard Business School Professor Jan Hammond within the on-line course Business Analytics.
This programming-oriented job includes creating the machine studying fashions wanted for Data Science applications. Machine learning and data science have saved the monetary business hundreds of thousands of dollars, and unquantifiable amounts of time. For instance, JP Morgan’s Contract Intelligence platform makes use of Natural Language Processing to process and extract important knowledge from about 12,000 commercial credit score agreements a year. Thanks to Data Science, what would take around 360,000 guide labor hours to complete is now finished in a few hours. Additionally, fintech companies like Stripe and Paypal are investing heavily in data science training in hyderabad to create machine studying tools that quickly detect and prevent fraudulent activities.
Applying AI cognitive applied sciences to ML methods can result in the effective processing of information and information. But what are the key variations between Data Science vs Machine Learning and AI vs ML? Simply put, synthetic intelligence aims at enabling machines to execute reasoning by replicating human intelligence. Since the principal objective of AI processes is to show machines from expertise, feeding the best data and self-correction is crucial. AI specialists depend on deep studying and natural language processing to assist machines establish patterns and inferences.
Retailers analyze customer habits and buying patterns to drive personalised product suggestions and targeted promoting, marketing and promotions. Data science also helps them manage product inventories and provide chains to maintain items in inventory. Data science permits streaming companies to trace and analyze what customers watch, which helps decide the brand new TV reveals and movies they produce. Data-driven algorithms are also used to create customized suggestions primarily based on a consumer's viewing history. It’s cutting-edge now, but soon a data cloth shall be a vital software for managing data.
The term was first used in 1960 by Peter Naur, who was a pioneer in laptop science. He described the foundational aspects of the methods and approaches used in data science in his 1974 book, Concise Survey of Computer Methods. [newline]There are many instruments out there for Data Scientists to make use of to govern and research huge portions of knowledge, and it's important to at all times evaluate their effectiveness and maintain attempting new ones as they become out there. Data scientists must depend on experience and intuition to decide which strategies will work greatest for modeling their data, and they should modify those methods constantly to hone in on the insights they seek. Data science plays an important role in safety and fraud detection, as the end result of the large quantities of information allows for drilling down to search out slight irregularities in knowledge that can expose weaknesses in safety methods. Delivery companies, freight carriers and logistics providers use Data Science to optimize supply routes and schedules, in addition to one of the best modes of transport for shipments.
Whereas knowledge analytics is primarily centered on understanding datasets and gleaning insights that can become actions, Data Science is centered on building, cleaning, and organizing datasets. Data scientists create and leverage algorithms, statistical fashions, and their own customized analyses to collect and form uncooked information into something that can be more simply understood. Some of the key variations however, are that data analysts sometimes usually are not laptop programmers, nor answerable for statistical modeling, machine learning, and lots of the other steps outlined within the Data Science process above. Many statisticians, together with Nate Silver, have argued that Data Science isn't a model new field, but quite another name for statistics. Others argue that data science is distinct from statistics as an end result of it focuses on problems and methods unique to digital knowledge. Vasant Dhar writes that statistics emphasizes quantitative knowledge and description.
They handle knowledge pipelines and infrastructure to transform and transfer data to respective Data Scientists to work on. They majorly work with Java, Scala, MongoDB, Cassandra DB, and Apache Hadoop. This web site makes use of cookies to improve your experience when you navigate through the website. Out of these, the cookies that are categorized as necessary are saved in your browser as they are essential for the working of primary functionalities of the internet site.

Yet, to harness the power of huge knowledge, it isn’t necessary to be a data scientist. Hopefully this article has helped demystify the info scientist position and other associated roles. More and more today, Data Scientists should be capable of utilizing instruments and technologies associated with huge amounts of information as nicely. Some of the most well-liked examples include Hadoop, Spark, Kafka, Hive, Pig, Drill, Presto, and Mahout.
For more information
360DigiTMG - Data Analytics, Data Science Course Training Hyderabad
Address - 2-56/2/19, 3rd floor,, Vijaya towers, near Meridian school,, Ayyappa Society Rd, Madhapur,, Hyderabad, Telangana 500081
099899 94319
https://g.page/Best-Data-Science
0 notes
Text
Bounded Rationality: The secret sauce of Behavioural Economics
In the year 1957, a Professor of Computer Science and Psychology at the Carnegie Mellon University wrote a book called Models of Man: Social and Rational. It was there that he introduced a term which would go on to redefine the way we understand human rationality. Bounded Rationality is simply the idea that the rationality of human beings is bound by the limitations of their cognitive capacities, time constraints, and the computational difficulty of the decision problem. Decision-making is also guided by the structure of the environment one is in ( not necessarily a physical environment).
Herbert Simon was one of the first who brought attention to the problem of cognitive demands of Subjective Expected Utility. Simon gave the problem of chess to demonstrate how human beings could possibly not behave as Subjective Expected utility theory assumes they do. A game-theoretic minimax algorithm for the game of chess would require evaluating more chess positions than the number of molecules in the universe. Simon posed two questions which have since been the subject of research for cognitive scientists and economists. On the question of how human beings make decisions in uncertainty, Simon asked the following questions:
1. How do human beings make decisions in the 'wild' ( in their natural environments)?
2. How can the principles of Global rationality ( the normative theory of Rationality) be simplified so that they can be integrated into the decision-making by humans? Imagine that you are searching for a job, you apply to a number of places and get interview calls from several places. Since interview rounds and hiring process takes a fair amount of time, you are concerned about an increasing employment gap. You cannot afford to evaluate all the options for as long as will take because you do not have enough. Now suppose you get a job offer from a company, the job excites you but the salary is just a fraction above your previous CTC, you are expecting a higher number. You decide to decline the offer. Fortunately, 5 days later you get another job offer. This time the job is respectable enough and the salary is above your expected CTC. Even though you have other interviews, you decide to take up this job. Sounds familiar? Turns out that you if you had done something similar, you were using something which Simon termed as the satisficing heuristic.
Herbert Simon proposed the Satisficing heuristic as an alternative to the optimization problem in Expected utility theory. The Satisficing heuristic is a simple yet robust mechanism with simple stoppage rules. The idea is that one should decide on the decision criterion beforehand and also decide on a threshold level below which one cannot take up a choice and then evaluate different options. Once an option is found that fulfils the decision criterion above the threshold level, one stops the search and takes the option. Therefore, a choice is made which both satisfies and suffices, it satisfices. This heuristic has been used in the context of mate selection, business decisions, and even sequential choice problems. According to Simon, human beings satisfice because they did not have the computational ability nor the time to maximize.
Bounded Rationality has gone on to inspire researchers in Cognitive Science and Economics. One of the most recognizable works is that of the Heuristics and Biases program led by the famous Daniel Kahneman and Amos Tversky. Based on the idea that there are two systems of thinking: System 1: The Automatic, Fast, and unconscious system of thinking and System 2: The Deliberate,Slow and conscious mode of thinking. According to Kahneman and Tversky, the System 1 mode of thinking was responsible for heuristics that turned to be biases in decision-making like the Conjunction fallacy, Base rate fallacy, Gambler’s fallacy,Sunk cost fallacy etc. These biases led to deviations from the normative idea of rationality which Simon had called Global Rationality and were termed ‘irrational’. The System 2 mode was something which made us deliberate and think over our decisions and was less prone to errors in decision-making. Kahneman got his Nobel Prize in Economics for Prospect theory in 2002, a theory which gave a behavioural alternative to the subjective expected utility model incorporating ideas such as Loss Aversion, Reference point, and Framing. The ideas of Kahneman and Tversky were built on by Richard Thaler who pioneered the field of Behavioural Economics and who introduced the concept of ‘Libertarian Paternalism’. If human beings were irrational, they could be given nudges for their own good so that they
The Fast and Frugal heuristics program was developed by the famous German psychologist Gerd Gigerenzer. The program is considered to be the main intellectual rival to the Heuristics and Biases program and there has been a long-drawn intellectual duel between the two programs. The main idea behind the Fast and Frugal heuristics program is the idea that heuristics are not irrational. They are fast and frugal and in situations of uncertainty perform better than other competing models of decision-making. Imagine doctors using surgical intuition to perform complicated operations in a limited time or firefighters making snap judgement and decisions in a dangerous and unpredictable environment.
Heuristics are fast and frugal and they get the job done. Fast and frugal heuristics are ‘ecologically rational’ which means that their rationality is dependent on their environment. Trust in doctor as a heuristic would be rational if say your doctor is experienced, has a medical degree, has no conflict of interests. On the other hand, it will not be rational if your doctor has some conflict of interest, does not have enough experience, or is just not the right doctor for the illness. My Master’s dissertation in the Palaj and Basan villages in Gandhinagar looked at the question of the conditionality of trust. I found evidence that the trust was built primarily on the doctor’s ability to prescribe medicines that could cure in short periods of time and that the trust was conditional in nature. Examples of other fast and frugal heuristics are the Recognition heuristic, Take the best heuristic, Tallying, 1/N rule etc.
The rise of Behavioural Economics and its poster boy, Nudge has in many ways energized the Economics discipline and brought renewed interest in it. But at times you do feel that commentators face a blackout when it comes to the foundations of the discipline and the foundations are much older than the 1970s when the famous Heuristics and Biases program was taking root. The foundations rather take root in the 1950s in the aftermath of the Second World War in the laboratories of Carnegie Mellon University. Simon’s ideas have found place in fields as diverse as AI, Cognitive Psychology, Design, and Administrative behaviour. While reading his book, the Sciences of the Artificial, I was struck by the intuitive nature of his arguments. Its time that we give him his due as much as any pioneering behavioural economist.
0 notes
Text
How AI & ML are Transforming Social Media?
With the advancement in technology and artificial intelligence, various AI-based application platforms have been gaining popularity for a long time. AI has turned out to be a boom for popular Social Media platforms like Facebook and Instagram. To know more about AI and Machine Learning development services in Social Media, continue reading this article!
Today Artificial Intelligence has been a major component of popular Social Media platforms. At the current level of progress, AI for social media has been a powerful tool.
What is Artificial Intelligence? The term artificial intelligence (AI) refers to any human-like intelligence shown by a machine, robot, or computer. It refers to the ability of machines to mimic or copy the intelligence level of the human mind. This may include actions like understanding and responding to voice commands, learning from previous records, problem-solving, and decision-making. Many companies are providing AI application development services, which has made it easy for organizations to adopt AI and ML-based applications. What is Machine Learning?
In general terms, Machine learning (ML) is a subset of AI focusing on building applications and software that can learn from past experiences and data and improve accuracy without being specifically programmed to do so. Machine learning applications learn more from data and are designed to deliver accurate results.
How AI works? Not going deep into the engineerings and software development part of AI, here is just a basic description of working of AI:
Using ML, AI tries to mimic human intelligence. AI can make predictions using algorithms and historical data.
AI and ML in Social Media
Today, there exist several applications of AI and ML in different social media platforms. Big Companies have been using AI for a long time and are still into improvising their platforms and also acquiring small firms. There exist varieties of
AI and Machine Learning App Development Services
that are making the adoption of AI and ML possible.
AI is being used on Social Media platforms in various ways. Some of them are mentioned below:
Analyzing pictures and texts
Advertising
Avoiding unwanted or negative promotions
Spam detection
Data collection
Content flow decisions
Social media insights, etc.
It may sound surprising but your favorite social media apps are already using Artificial Intelligence and Machine Learning.
1. Facebook and AI
Whenever it comes to social media, the first name that comes to mind is Facebook. Talking about cutting-edge technology, repurposing user data broken down into billions of accounts, Facebook is the leading social media platform.
Users on Facebook are allowed to upload pictures, watch videos, read texts and blogs, engage with different social groups, and perform many other functions.
Thinking of such a crazy and huge amount of data, a question arises how Facebook handles such data? Here, AI in Facebook comes in handy.
Facebook and the use of AI in Social Media
Here are some major examples of AI applications in Social Media:
* Facebook’s Text Analyzing
Facebook has an AI-based tool “DeepText”. This tool provides deep learning and helps the back-end team to understand the texts better and that too around multiple languages and hence provide better and more accurate advertising to the users.
* Facebook’s Picture Analyzing
Facebook uses Machine Learning to recognize faces in the photos being uploaded. Using face recognition, Facebook helps you find users that are not known to you. This feature also helps in detecting Catfishes (fake profiles created using your profile picture).
The algorithm also has an amazing feature of text explanations that can help visually disabled people by explaining to them what’s in the picture.
* Facebook’s Bad Content Handling
Using the same tool, DeepText, Facebook has been hailing the inappropriate or bad content that gets posted. After getting notified by AI, the team gets to work to understand and investigate the content.
As per the company guidelines, we get to see a few things that are flagged as inappropriate content:
Nudity or sexual activity
Hate Speech or symbols
Spam
Fake Profiles or fraud
Contents containing excessive violence or self-harm.
Violence or Dangerous organizations
Sale of illegal goods
Intellectual property violations, etc.
Facebook’s Suicide Preventions
With the same tool, DeepText, Facebook can recognize posts or searches that represent suicidal thoughts or activities.
Facebook has been playing a crucial role in suicide prevention. With the support of an analysis based on human moderators, Facebook can send videos and ads containing suicide prevention content to these specific users.
Facebook’s Automatic Translation
Facebook has also adapted AI for translating posts automatically in various languages. This helps the translation be more personalized and accurate.
2. Instagram with AI
Instagram is a photo and video-sharing social media platform that has been owned by Facebook since 2012. Users can upload pictures, videos (reels and IGTV) of their lifestyle, and other stuff and share them with their followers.
This platform is used by individuals, businesses, fictional characters, and pets as well. Managing all the data manually is next to impossible. Therefore, Instagram has developed AI algorithms and models making it the best platform experience for its regular users.
Instagram and the use of AI
* Instagram Decides What Gets on Your Feed
The Explore feature in Instagram uses AI. The suggested posts that you get to see on your explore section are based on the accounts that you follow and the posts you’ve liked.
Through an AI-based system, Instagram extracts 65 billion features and does 90 million model predictions per second.
The huge amount of data that they collect, helps them to show the users what they like.
* Instagram’s Fighting against Cyberbullying
While Facebook and Twitter are dependent mostly on reports from users, Instagram automatically checks content based on hashtags from other users, using AI. In case something is found against the community guidelines, the AI makes sure that the content is removed from Instagram.
* Instagram’s Spam Filtering
Instagram’s AI is capable of recognizing and removing Spam messages from user’s inboxes and that too in 9 different languages.
With the help of Facebook’s DeepText tool, Instagram’s AI can understand the spam context in most situations for more filtration.
* Instagram’s Improved Target Advertising
Instagram can keep a track of which posts have most of the user engagements or the user’s search preferences. Later, Instagram with the help of AI makes target advertisements for companies based on all such databases.
* Instagram handling Bad Contents
Since Instagram is owned by Facebook, more or less, Instagram also follows the same community guidelines over bad content.
3. Twitter and Use of AI
On average, Twitter users post around 6,000 tweets per second. In such a case, AI gets necessary for dealing with such a huge amount of data.
* Tweet Recommendations - AI in Twitter
Twitter firstly implemented AI to improve and give users a better user experience (UX) that would be capable of finding interesting tweets. Now, with the help of AI, Twitter also detects and removes fraud, propaganda, inappropriate content, and hateful accounts.
This recommendation algorithm works in a very interesting way as it learns from your actions over the platform. The tweets are ranked to decide their level of interest, based on the individual users.
AI also considers your past activities of engaging with various types of tweets and uses it to recommend similar tweets.
* Twitter Enhancing Your Pictures - AI in Twitter
Posting of pictures on Twitter was introduced in the year, 2011. Since then, it has been working over an algorithm that is capable of cropping images automatically.
Firstly, they created an algorithm that focused on cropping images based on face recognition, because not every image is supposed to have a face on it. Thus the algorithm was not acceptable.
AI is now used over the platform to crop images before posting them, to make the image look more attractive.
* Tweets Filtration - AI in Twitter
Twitter uses AI to take down inappropriate images and accounts from the platform. Accounts connected to terrorism, manipulation, or spam are taken down using this feature.
* Twitter Fastening the Process - AI in Twitter
How did Twitter use AI to speed up the platform?
For this, Twitter uses a technique called Knowledge Distillation to train smaller networks imitating the slower but strong networks. The larger network was used to generate predictions over a set of images. Then, they developed a pruning algorithm to remove the part of the neutral network.
Using these two models Twitter managed to work over cropping of images 10x faster than ever before.
4. AI in Snapchat
Snapchat started by acquiring two AI companies. In 2015, it first acquired Looksery, a Ukrainian startup, to improvise its animated lenses feature. Secondly, it acquired AI Factory to enhance its video capabilities.
* Snapchat’s Text Recognition in Videos
Snapchat uses AI to recognize texts in the video, which then adds content to your “Snap”. If you type “Hello”, it automatically creates a comic icon or Bitmoji in the video.
* Snapchat- Cameo Feature
AI in Snapchat can be used to edit one’s face in a video. Using the Cameo feature, the users can create a cartoon video of themselves.
From the above-mentioned renowned, we can extract a list of benefits of AI and ML in Social Media, which is given below:
Prediction of user’s behavior
Recognition of inappropriate or bad content
Helps in improving user’s experience
More personalized experience to the users
Gathering of valuable information and user data.
AI has also helped understand human psychology, tracking multiple characteristics of your behavior and responses.
If you are looking for the best
AI & Machine Learning Solutions Provider
for your organization, Consagous Technologies is one of the best
AI Application Development Company in USA
. With years of experience, all the company professionals are great at their work.
Original Source:
https://www.consagous.co/blog/how-ai-ml-are-transforming-social-media
0 notes
Text
Critical Thinking Lectures 2
During this lecture we discussed what the future holds and how the prediction of current social changes impact on fashion & textiles.
Today's objective we are going to start to investigate current social factors, and we will try to predict the impact that these drivers will have on the future of fashion and textiles.
The key causes of changes to the industry are: Sustainability, consumerism, innovation, division of wealth, social media, politics & power.
Politics and power - As a group we spoke about the most powerful people in the world. We decided that governments are the most powerful. A government is an institution where leaders exercise power to make and enforce laws. A government's basic functions are providing leadership, maintaining order, providing public services, providing national security, providing economic security, and providing economic assistance.
WATCHMOJO.COM said that the following people are the most powerful people in the world:
Xi Jinping: China’s president
Vladimir Putin: Russia’s president
Angela Merkel: German Chancellor since 2005. Compassionate leader. Powerful lead over European Union’s.
Pope Francis: Fairly progressive leader; pro women & spoke in favor of LGBT community
Donald Trump: Massive following, continued to make headlines.
Jeff Bezos: Founder of Amazon. Worlds richest person.
Bill Gates: Microsoft Founder high profile. Charitable trusts (reported a wealth of 120 billion).
Larry Page: Co-Founder of Google (reported a wealth of 78 billion) 6.9 million searches on google every Fay.
Narendra Modi: India’s longest serving prime minister.
Mark Zuckerburg: Founder of Facebook.
1 Xi Jinping (General secretary to China’s communist party) 2nd largest economy and inhabited country
Amended constitution to broaden his power, scrapped term limits
“Chinese Dream - personal & national ideals for the advancement of Chinese society” Ewalt, D (2018)
2 Valdimir Putin (Ruled since 2000 4 terms)
Putin set up constitutional changes allowing him to remain in power in Russia beyond 2024
FBI investigation ref influencing Trump’s presidential campaign Trump
Europe is dependent on Russia’s oil and gas supplies
Nationalistic focus
Rise of wealth and standard of living
Stamped out democracy – controls media, critics and journalists of opposition have been killed
“Socially Conservative” negative regarding homosexuality according to Wikipedia (2019)
3 Trump Zurcher, A BBC (2018)
Immigration: closed border to some Muslim countries & building a wall between America & Mexico
Healthcare: repealing Obamacare
Environment; reduction in commitment, to save USA costs
Intention to make America a great nation by increased infrastructure, reducing imports whilst expanding exports
Huge following
Division & Wealth
Global inequality = poverty and social conflict
The richest 1% of the world is twice as wealthy as the poorest 50%. The world richest 1% have more than twice as much wealth as 6.9 billion people, almost half of humanity is living on less than $5.50 a day. Global inequality causes poverty and social injustice. The inequality may affect the fashion industry as not everyone will have the chance to study fashion, due to not having the money or opportunity.
Consumerism:
The rise of fast fashion: As online clothing sales increase during the rise of Covid-19, our unsustainable habit is proving hard to stop. This shows that even the pandemic couldn't solve the fast fashion issue. Online clothing sales in August were up 97% versus 2016 consumer's mindset is returning to unsustainable habits. Boohoo's profit increased by 50% during the pandemic, despite the factory scandal. The Boohoo supplier was involved in 'multi-million-pound' fraud scheme. Leicester clothing factories with links to Boohoo and Select Fashion have been involved in a “multi-million pound” money laundering and VAT fraud scandal, an investigation has found.
Sustainable Fashion:
The Global Goals for Sustainable Development:
“These goals have the power to create a better world by 2030, by ending poverty, fighting inequality and addressing the urgency of climate change. Guided by the goals, it is now up to all of us, governments, businesses, civil society and the general public to work together to build a better future for everyone.” Global Goals 2020
The pandemic has definitely grown the understanding for sustainable fashion.
Innovation:
Artificial Intelligence (AI) - the simulation of human intelligence processes by machines, especially computer systems. Rouse, M 2018.
Robotic technology - the use of computer-controlled robots to perform manual tasks. If AI and robotic technology could equate to massive job cuts, i.e. Amazon are already testing drones to complete unmanned delivers to customers, this will mean many people will lose their jobs. The cost of producing and maintaining these machines and this new updated technology may mean people who are already not being paid fairly will be paid even less. If robots are used in war there is nothing stopping robots from being able to design fashion.
How AI is transforming the fashion industry?
Chatbots 24/7 fashion advisors
Analysis of social media conversations
Forecasting - AI being used to plan the nest trends
Real time data analysis to assist retailers
Stocking systems - what to keep in stock and restock
Khaite merged AR, film and traditional mediums, sending presentation boxes to editors and buyers, including lookbooks and fabric samples with QR codes revealing fashion films and AR 3D renderings of their new shoes.
Farra. E. Vogue Runway 2020 This Is the First Augmented Reality Experiment of Spring 2021
https://www.vogue.com/article/khaite-spring-2021-augmented-reality-experience
Social Media
Social media is very important to have when starting your own brand, it is an easy way to grow connections and loyal customers by promoting your business online. However social media also has its dark side. The documentary The Social Dilemma on Netflix taught me this. “If you are not paying for the product you are the product”. Social media uses persuasive technology to manipulate the product/ people. Technology engineers use addiction & manipulation psychology to control the user. The user is controlled to look at more adds to get more money. Social media is a drug.
How are we being manipulated?
Everything online or on social media is watched, recorded and monitored building a model of each of us - to enable the technology to predict what we will do & how we will behave.
Machine learning algorithms that are getting better & better so that they can engage humans on social media more & more; learning from our internet searches to suggest the next leading topic...leading us down the rabbit hole.
Technique have been designed to get people to use their phones more i.e. likes, photo tapping, notifications etc...
We were asked to type into google “climate change is” we all have different results based on our individual algorithms that have been developed by the platforms.
What can we do ?
Products need to be designed humanly.
We have a responsibility to change what we built.
People (users) are not a product resource.
Introduce more laws around digital privacy.
Introduce a tax for data collection.
Reform so don’t destroy the news.
Turn off notifications.
Don’t use social media after 9pm.
Always do an extra google search - double check your resources.
Don’t follow just follow suggestions.
How does the fashion industry use social media?
For the fashion industry, social media has brought connectedness, innovation, and diversity to the industry. Instagram, for example, functions as a live magazine, always updating itself with the best, most current trends while allowing users to participate in fashion rather than just watch from afar. People are influenced by social media when it comes to there fashion through trendsetters like, celebrities and fashion influencers.
Although social media has many positives, there is also many dangers that comes from it as well. People become obsessed and addicted to social media, always needing to post and show off everything they are doing. Everything people post on social media is mainly the best parts of their lives making people who have struggles feel even worse. Especially for the young generation there is a need for having the most followers, likes and comments and if you don’t you’re not ‘popular’. Due to social media the younger generation mainly compare themselves and their lives to the ‘perfect’ people they see on social media, which is not the reality. It is all a lie, everyone has good and bad parts to their lives but no one shows the negative sides on social media. This is what creates a depressed generation. Everyone then just hides behind screens wishing they were someone else which then leads to jealousy and evilness. Most trolls on social media are those who are just jealous of the love and support these high followed people on social media receive and the high quality of life they live but what they don’t see is the bad side to these people's lives that is not shown half the time.
0 notes
Text
Artificial Intelligence Books For Beginners | Top 17 Books of AI for Freshers
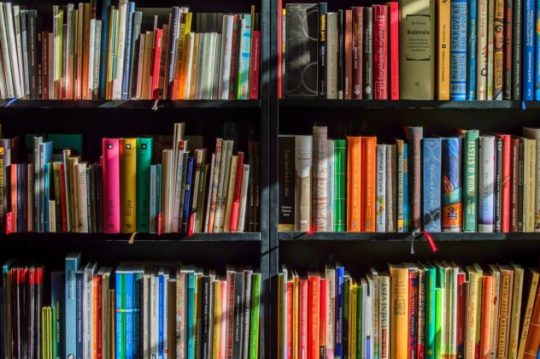
Artificial Intelligence (AI) has taken the world by storm. Almost every industry across the globe is incorporating AI for a variety of applications and use cases. Some of its wide range of applications includes process automation, predictive analysis, fraud detection, improving customer experience, etc.
AI is being foreseen as the future of technological and economic development. As a result, the career opportunities for AI engineers and programmers are bound to drastically increase in the next few years. If you are a person who has no prior knowledge about AI but is very much interested to learn and start a career in this field, the following ten Books on Artificial Intelligence will be quite helpful:
List of 17 Best AI Books for Beginners– By Stuart Russell & Peter Norvig
This book on artificial intelligence has been considered by many as one of the best AI books for beginners. It is less technical and gives an overview of the various topics revolving around AI. The writing is simple and all concepts and explanations can be easily understood by the reader.
The concepts covered include subjects such as search algorithms, game theory, multi-agent systems, statistical Natural Language Processing, local search planning methods, etc. The book also touches upon advanced AI topics without going in-depth. Overall, it’s a must-have book for any individual who would like to learn about AI.
2. Machine Learning for Dummies
– By John Paul Mueller and Luca Massaron
Machine Learning for Dummies provides an entry point for anyone looking to get a foothold on Machine Learning. It covers all the basic concepts and theories of machine learning and how they apply to the real world. It introduces a little coding in Python and R to tech machines to perform data analysis and pattern-oriented tasks.
From small tasks and patterns, the readers can extrapolate the usefulness of machine learning through internet ads, web searches, fraud detection, and so on. Authored by two data science experts, this Artificial Intelligence book makes it easy for any layman to understand and implement machine learning seamlessly.
3. Make Your Own Neural Network
– By Tariq Rashid
One of the books on artificial intelligence that provides its readers with a step-by-step journey through the mathematics of Neural Networks. It starts with very simple ideas and gradually builds up an understanding of how neural networks work. Using Python language, it encourages its readers to build their own neural networks.
The book is divided into three parts. The first part deals with the various mathematical ideas underlying the neural networks. Part 2 is practical where readers are taught Python and are encouraged to create their own neural networks. The third part gives a peek into the mysterious mind of a neural network. It also guides the reader to get the codes working on a Raspberry Pi.
4. Machine Learning: The New AI
– By Ethem Alpaydin
Machine Learning: The New AI gives a concise overview of machine learning. It describes its evolution, explains important learning algorithms, and presents example applications. It explains how digital technology has advanced from number-crunching machines to mobile devices, putting today’s machine learning boom in context.
The book on artificial intelligence gives examples of how machine learning is being used in our day-to-day lives and how it has infiltrated our daily existence. It also discusses the future of machine learning and the ethical and legal implications for data privacy and security. Any reader with a non-Computer Science background will find this book interesting and easy to understand.
5. Fundamentals of Machine Learning for Predictive Data Analytics: Algorithms, Worked Examples, and Case Studies
– By John D. Kelleher, Brian Mac Namee, Aoife D’Arcy
This AI Book covers all the fundamentals of machine learning along with practical applications, working examples, and case studies. It gives detailed descriptions of important machine learning approaches used in predictive analytics.
Four main approaches are explained in very simple terms without using many technical jargons. Each approach is described by using algorithms and mathematical models illustrated by detailed worked examples. The book is suitable for those who have a basic background in computer science, engineering, mathematics or statistics.
6. The Hundred-Page Machine Learning Book
– By Andriy Burkov
Andriy Burkov’s “The Hundred-Page Machine Learning Book” is regarded by many industry experts as the best book on machine learning. For newcomers, it gives a thorough introduction to the fundamentals of machine learning. For experienced professionals, it gives practical recommendations from the author’s rich experience in the field of AI.
The book covers all major approaches to machine learning. They range from classical linear and logistic regression to modern support vector machines, boosting, Deep Learning, and random forests. This book is perfect for those beginners who want to get familiar with the mathematics behind machine learning algorithms.
7. Artificial Intelligence for Humans
– By Jeff Heaton
This book helps its readers get an overview and understanding of AI algorithms. It is meant to teach AI for those who don’t have an extensive mathematical background. The readers need to have only a basic knowledge of computer programming and college algebra.
Fundamental AI algorithms such as linear regression, clustering, dimensionality, and distance metrics are covered in depth. The algorithms are explained using numeric calculations which the readers can perform themselves and through interesting examples and use cases.
8. Machine Learning for Beginners
– By Chris Sebastian
As per its title, Machine Learning for Beginners is meant for absolute beginners. It traces the history of the early days of machine learning to what it has become today. It describes how big data is important for machine learning and how programmers use it to develop learning algorithms. Concepts such as AI, neural networks, swarm intelligence, etc. are explained in detail.
This Artificial Intelligence book provides simple examples for the reader to understand the complex math and probability statistics underlying machine learning. It also provides real-world scenarios of how machine learning algorithms are making our lives better.
9. Artificial Intelligence: The Basics
– By Kevin Warwick
This book provides a basic overview of different AI aspects and the various methods of implementing them. It explores the history of AI, its present, and where it will be in the future. The book has interesting depictions of modern AI technology and robotics. It also gives recommendations for other books that have more details about a particular concept.
The book is a quick read for anyone interested in AI. It explores issues at the heart of the subject and provides an illuminating experience for the reader.
10. Machine Learning for Absolute Beginners: A Plain English Introduction
– By Oliver Theobald
One of the few artificial intelligence books that explains the various theoretical and practical aspects of machine learning techniques in a very simple manner. It makes use of plain English to prevent beginners from being overwhelmed by technical jargons. It has clear and accessible explanations with visual examples for the various algorithms.
Apart from learning the technology itself for the business applications, there are other aspects of AI that enthusiasts should know about, the philosophical, sociological, ethical, humanitarian and other concepts. Here are some of the books that will help you understand other aspects of AI for a larger picture, and also help you indulge in intelligent discussions with peers.
Philosophical books11. Superintelligence: Paths, Dangers, Strategies
– By Nick Bostrom
Recommended by both Elon Musk and Bill Gates, the book talks about steering the course through the unknown terrain of AI. The author of this book, Nick Bostrom, is a Swedish-born philosopher and polymath. His background and experience in computational neuroscience and AI lays the premise for this marvel of a book.
12. Life 3.0
– By Max Tegmark
This AI book by Max Tegmark will surely inspire anyone to dive deeper into the field of Artificial Intelligence. It covers the larger issues and aspects of AI including superintelligence, physical limits of AI, machine consciousness, etc. It also covers the aspect of automation and societal issues arising with AI.
Sociological Books13. The Singularity Is Near
– By Ray Kurzweil
Ray Kurzweil was called ‘restless genius’ by the Wall Street Journal and is also highly praised by Bill Gates. He is a leading inventor, thinker, and futurists who takes keen interest in the field of Artificial Intelligence. In this AI book, he talks about the aspect of AI which is most feared by many of us, i.e., ‘Singularity’. He talks extensively about the union of humans and the machine.
14. The Sentiment Machine
– By Amir Husain
This book challenges us about societal norms and the assumptions of a ‘good life’. Amir Husain, being the brilliant computer scientist he is, points out that the age of Artificial Intelligence is the dawn of a new kind of intellectual diversity. He guides us through the ways we can embrace AI into our lives for a better tomorrow.
15. The Society of Mind
– By Marvin Minsky
Marvin Minsky is the co-founder of the AI Laboratory at MIT and has authored a number of great Artificial Intelligence Books. One such book is ‘The Society of Mind’ which portrays the mind as a society of tiny components. This is the ideal book for all those who are interested in exploring intelligence and the aspects of mind in the age of AI.
Humanitarian Books16. The Emotion Machine – By Marvin Minsky
In this book, Marvin Minsky presents a novel and a fascinating model of how the human mind works. He also argues that machines with a conscious can be built to assist humans with their thinking process. In his book, he presents emotion as another way of thinking. It is a great follow up to the book “Society Of Mind”.
17. Human Compatible — Artificial Intelligence and the Problem of Control
– By Stuart Russell
The AI researcher, Stuart Russell explains the probable misuse of Artificial Intelligence and its near term benefits. It is an optimistic and an empathetic take on the journey of humanity in this day and age of AI. The author also talks about the need for rebuilding AI on a new foundation where the machine can be built for humanity and its objectives.
So these were some of the books on artificial intelligence that we recommend to start with. Under Artificial Intelligence, we have Machine Learning, Deep Learning, Computer Vision, Neural Networks and many other concepts which you need to touch upon. To put machine learning in context, some Basic Python Programming is also introduced. The reader doesn’t need to have any mathematical background or coding experience to understand this book.
If you are interested in the domain of AI and want to learn more about the subject, check out Great Learning’s PG program in Artificial Intelligence and Machine Learning.
0 notes
Text
How to get more website traffic Chandler

Each year Google puts out at least 500 algorithmic changes to its search engine, and with more than 200 SEO factors, tracking what is working for organic search is an important task every marketing manager or business manager should be doing. Following is a summary of the ranking factors to keep a close eye on if you desire to be at the top of Google in Chandler Arizona. But first, learn what search engine optimization can do for your local business website.Video: See what a Chandler top ranked SEO consultant can do for your business.CLICK HERE TO Grab a zero cost SEO analysis.In our experience, an integrated search technique that utilizes both search engine optimization and performance marketing (Facebook ads, Google Adwords, etc.) is a highly efficient technique for growing your presence online. Results will be improved in every channel by using paid and organic marketing approaches. For high-growth, aggressive businesses you will wish to establish a holistic search engine strategy instead of look at SEO or PPC as stand-alone options. To assist even more inform business owners, executives and marketing directors on the virtues of PPC and SEO, continue reading for more important info as it connects to paid marketing and search engine optimization. To begin, Pay Per Click uses laser focused visibility.
Top SEO conditions:
# 1 – Optimizing for mobile search is essential.Over half of standard searches are now happening on a mobile device. Isn’t it time that you made your website mobile responsive? If you build a mobile optimized site there is a guaranteed search engine optimization and conversion advantage.Video: Why how your site ranks on mobile, is important.# 2 – The importance of AMP pages is growing.Accelerated mobile web pages (AMP) is an innovation that allows a website on mobile platforms to load more quickly than standard HTML rendered in a mobile browser. Google has been saying that AMP compatible websites will perform better in search. Don’t wait, you need to have your website mobile ready with AMP.# 3 – Better design means enriched UI/UX and deeper engagement.Google likes websites that supply users with a favorable experience, and those which make finding the information the user is requesting easy. If you are looking to rank high in the SERPs, the design of your site is an important ranking element, and focus should be paid to it. To learn more about the role of video in marketing, watch this video.# 4 – Loading time of webpages matters.In addition to making sure that all your images are correctly labeled, and metadata fields such as the ALT tag are completed, the extra few minutes to scale your photos and reduce their size, is worth the investment. Google is now taking into account page load times, as a factor for ranking. In addition to using a photo optimization product like JPEGmini, a fast and effective method for reducing photo sizes is to ensure that all your images are scaled appropriately. Don’t upload images that are larger than the web design requires. Example- you do not need to use a 1000px wide image if the window in your design only displays 300px.# 5 – Rank Brain by Google and AI control search.With artificial intelligence driving everything Google does, whether developing self-driving cars or a next generation search engine, AI, starting with RankBrain is the power behind the search engine results pages (SERPs) served by Google. Local Chandler SEO firms will need to invest even more R&D budget to learn the most effective ways to optimize websites for ranking performance and discoverability.# 6 – Fresh content wins on Google.Google is rewarding differentiated content even more than ever. In order to rank rank your business website, you must create engaging, original, high-quality content. The days of reposting another company’s content as a method to drive relevance and traffic, are gone. Google can track at this stage, nearly every website in the world, which means, content that is not unique or doesn’t add value to a subject will have difficulty getting discovered. This fact puts pressure on websites to become content creators, which is why marketing departments are pouring extensive resources into content development teams.# 7 – Longer content ranks better.In summary, add value, and do not spam your audience. If you are in the habit of writing verbose “SEO posts” that offer little value except the inclusion of keywords, stop doing it now. Google is now rewarding posts of 1,500 words and more with better search performance.# 8 – SERPs are now factoring in rich snippets, schema, FAQs and pre-populated information fields.For searches where Google can identify common questions, FAQs, or other key information, they are beginning to fill a larger area of the home page with data lists, including frequently asked question blocks so that the consumer can get their question answered without needing to select a search result. You must use schema in order to improve the chances of being included in Google’s rich data lists such as FAQ’s.# 9 – Social variables carry a ton of weight when it comes to website ranking results.At SEO Ranker Agency our team believes that social shares of the referring page are now one of the leading 100 ranking signals. This concept is shown by the high volume of rankings that SEO Ranker Agency Chandler has delivered where traffic from social media networks, backlinks, and shares, were demonstrated to be a major ranking condition.# 10 – Voice search and also IoT gadgets will likely get rid of SERP ranking order at some point.As voice user interfaces are expanding on mobile phones, cars and truck infotainment systems with gadgets like the apple iphone, Amazon.com Alexa, Google Home and others, even more people will be obtaining responses from a search question expressed by voice. Not just will being in the top matter much more now, if you are not in the first or maybe 2nd position, you are not going to receive any one of the search query outcomes.# 11 – HTTPS will certainly end up being important for ranking.Web safety and security professionals see Google pushing HTTPS ever before harder as cybercrime and hacking continue to provide a real danger to the world. Google as well has discussed the prioritization of HTTPS in such a way that it could be a ranking variable not to overlook. Does this mean that if your site is not an HTTPS site, that it will stop ranking? No. If you desire every side feasible, do not disregard HTTPS.# 12 – The Facebook internet search engine will certainly gain a growing number of customers.All Facebook individuals are using the FB internet search engine. Expect this pattern to speed up as customers do not wish to leave the Facebook application just to seek out something on Google.com# 13 – Titles as well as Summaries with better click thru rates will certainly rank higher.Google will certainly proceed fulfilling authors who have actually gone the added mile to produce catchy titles that obtain clicked. Google is currently tracking interaction, click thru rates (CTR) and also time on page.# 14 – A growing number of users are searching for video, infographics, images and multimedia material.If you’re not producing various sorts of visual web content to enhance your brand online, you ought to seriously consider taking the initiative on. Users are currently familiarizing the indexing capabilities of Google, Bing, and Yahoo! – and also they are performing searches for videos as well as scenes inside of video clips. To stand out from your competitors, first, begin creating even more visual content. Do not neglect to publish it with as total metadata and markup message as possible.Video: Discover the advantage of search engine optimization compared to paid traffic.If you are wondering, “how can I improve my ranking results?” then read on.Chandler Search Engine Optimization – free Google traffic, is one of the most affordable promotion method for any kind of organisation that delivers their products as well as solutions to regional customers. Exactly what is far better, Search Engine Optimization or PPC? Truthfully, we can not address this question without taking a look at the business’s objectives as well as objectives.A snugly niched down company with little competition in a very tiny solution area as well as a need for only a few leads each week might develop strong visibility in the neighborhood as well as natural search results page with a fundamental SEO consulting bundle.An ecommerce shop contending with first web page SERPs from Amazon.com, eBay.com and other major on-line sellers, is most likely going to struggle in natural search.An electronic marketing and also Search Engine Optimization technique with concentrated short- and also long-term objectives is necessary. Does your business requirement leads currently? What is the paid search (Pay Per Click) cost per click for your targeted keywords? Does your website have authority? Are you playing the lengthy game online or looking for short-term results only? Just how hard is your organic search competition?CTR and trust favor organic search, so why would a business consider paid search? Here are a couple of Pay Per Click benefits:Paid search controls the material are above the fold. In other words, on a smaller screen, you will not need to scroll to see the ads, however you will have to scroll to see all the organic search listings. Remember that Pay Per Click advertisements are simply that: ads, which indicates as a marketer you will have more control and area offered for providing your marketing messages.Google provides the choice of a visual shopping ad that can help a user visualize what they are selecting/clicking. This advertisement type enhances significantly the ads CTR by using a function (visual carousel) that is not offered in natural search.In addition, PPC enables for a much tighter control of the budget. Pay Per Click also offers the little company owner a highly targeted method to get in front of prospective consumers or clients.Establishing a strong natural search presence can require time, making SEO a medium to long variety play. On the other hand, a Pay Per Click campaign can be ramped and return favorable lead to weeks. Considering that there is no faster way to get in front of your audience than with Pay Per Click, many companies employ Pay Per Click while the authority of their site is being developed and SEO tactics take a firmer hold.Where organic search obscures keyword data, there is no limitation with paid search (Pay Per Click). Using conversion tracking and with a solid combination with Google Analytics, we can determine exactly what keywords convert best, and at what expense. This intelligence can be fed into SEO marketing campaigns and inform other advertising techniques to improve outcomes across the board. Speed offers agility and makes it possible for fast feedback on new slogans, messages, item announcements, and so on with using short burst PPC ad jobs.If you are a service targeting a regional service location with a limited set of keywords, you will discover that PPC can produce more than adequate leads without going over budget. Mindful use of match types and analysis of the search term reports permit for the elimination of junk search and an increase in return on financial investment.The benefits of Pay Per Click as well as Search Engine Optimization might not be so evident, yet they consist of the adhering to points of consideration.1. Conversion information from PPC keywords can be valuable to determine the most efficient natural search (SEO) strategy.2. PPC can ramp website traffic by targeting clicks in paid and natural for high-performing keywords. E.g. If you are winning the Google AdWords auction and rank in the top 3 SERPs for the very same keyword, you can anticipate up to 50% or more of the total search volume.3. A/B screening of PPC landing page and marketing copy can be fed into your natural listing and landing pages.4. Use PPC to check your keyword strategy prior to dedicating to search engine optimization job initiatives.5. Speak with users in all stages of the consumer journey from initial product research study to the competitive comparison, through to the purchase decision stage, with commercial intent keywords.6. Look big online and increase the confidence and awareness in your brand and company with a robust organic and paid existence.7. Retarget your website visitors on other properties by using the Facebook and Google pixel on your site. This is an extremely efficient technique that permits you to stay in front of visitors to your site even after they have left.There are numerous benefits to paid search advertising, however there are likewise pitfalls marketers must be cognizant of.1. PPC is reasonably simple to copy which suggests your competitors can quickly imitate your ad copy, images and contact us to actions. Successful Pay Per Click project management requires keeping track of bids, Quality Ratings, keyword positions and click-through rates (CTRs). A few of this work can be done with automation, however no matter the approach, you must guarantee that a system remains in place to track this critical details.2. Paid search marketing (PPC) needs an upfront and continuous financial investment. There are various alternatives available for PPC that can influence your results. If product listings control the screen for the keywords you are targeting; then text based advertisements might not transform.For this factor, it is necessary to do some research prior to you release your Pay Per Click campaign. Ensure to Google the keywords that you are targeting and be specifically careful to see what type is being shown. Also, make certain to look at the words they are using. Do not copy the advertisements, but you would do great to replicate them.Enhance search traffic to your service website with search engine optimization of your business website.A significant reason to invest in SEO is because of the power of online search engine to enhance your awareness. If you have visibility in the online search engine results pages (SERPs) for the keywords that you are targeting, this will put your business in front of a tremendously high variety of possible clients. The very best method to think of SEO is that SEO drives brand awareness and is totally free Google marketing.Branding is another advantage of local search engine optimization because search terms and informative inquiries related to your service can have a favorable branding advantage. As your brand name is returned in the search results page, it can (and typically will) become more connected with and trusted by searchers, and this will result in a purchase decision. Content marketing is the foundation and foundation of SEO. The more that your content, hence brand name, is associated as a professional in your field, place or industry, you will become a reliable voice which will lead more Internet searchers to discover you and do business with your company.Research studies have actually shown that search engine users trust natural results as being more trustworthy than paid marketing. Numerous users skip over the advertisements and go straight to the natural results as they presume that Google likewise rates the organic sites more extremely. Showing up on the first page of Google will provide your organisation a stamp of approval that can be the difference between somebody clicking on your listing (ranking) or your competitors.Do not forget the function that positive evaluations play in getting your target customer to call you. It’s a reality that even if you rank greater than your competitor if they have more stars revealing excellent reviews, this can make all the difference in your conversions. For numerous service based companies, evaluations are necessary.Search engine optimization increases site traffic as the greater you rank, the more visitors you will need to your website and the more opportunity to own awareness of your business. Traffic from organic search is totally free, developing exposure takes time and effort, as Google has actually slowed down ranking outcomes substantially over the last few years. However, the advantage of SEO is that unlike Pay Per Click, when you stop investing, there is a sluggish decay to the rankings. This does not indicate that you shouldn’t continue purchasing preserving your rankings, however it means that you don’t have to spend the same quantity.As an outcome of the points above, organic online search engine traffic by method of seo can provide a better ROI over traditional forms of paid media including Pay Per Click. While search engine optimization is at first not low-cost or easy, it remains in the long run far more economical than the majority of other marketing tactics and delivers more powerful brand awareness and traffic to your business site. Unlike paid search marketing or PPC, free traffic from Google does not dry up the moment you stop paying.This suggests that SEO will create more clicks from a natural search listing than from an extremely positioned paid advertisement. Keyword-level experimentation is required to make sure that you are not paying for clicks that you might get for free, but to maximize results and that is qualified leads, some organisations discover that exposure in both paid and organic listings are required.Because of the always changing and vibrant nature of the web, lots of entrepreneur and marketing executives discover that dealing with a professional digital marketing and SEO specialist is the very best way for them to guarantee the very best outcomes. Organic traffic can take time, and the competence had to beat those above you in the SERPs is significant. Which is why, if you are just starting out, and the keywords you are targeting are currently “owned” by high authority websites, you might have to reconsider your technique.A skilled search marketing expert ought to be able to assist your group in establishing content properties to search engine ranking dominance. A # 1 ranked search engine optimization company will prove their worth by assisting you build safe, sustainable links so you can attain the website rankings that will propel your company to the next level.Click here to receive a no cost seo audit and learn how search engine optimization can impact your business.Discover what local SEO in Chandler Arizona can do for your business website. Watch this video:
How to get more website traffic Cave Creek
The post How to get more website traffic Chandler appeared first on Get your website on the first page of Google with SEO.
0 notes
Text
What are the major use cases of Oil & Gas Analytics Solutions in Saudi Arabia?

BI#1 Oil & Gas Analytics Solutions in Saudi Arabia are particularly effective in environments that involve copious amounts of data and highly complex and variable operating conditions—that is, the same environments that O&G operators currently struggle to manage with simulator training, rules of thumb, and on-the-job experience.
Advanced analytics are powered by machine learning, which uses statistical methods and computing power to spot patterns among hundreds of variables in continual conditions. The patterns are used to build algorithms which analyze the parameters critical to production, quality, and efficiency, alert operators to conditions that are hours and days in the future, and enable them to respond fast and effectively.
BI#1 Oil & Gas Analytics Solutions in Saudi Arabia

Corrosion Analysis
In the creation stage, oil and gas organizations need to store rough and refined oil in enormous tanks and transport it through pipelines. Erosion by raw petroleum is a typical hazard for hardware disappointments in the oil and gas industry. Unrefined petroleum from oil fields for the most part fluctuates in its substance pieces and the destructiveness of the rough likewise relies upon the earth it is put away in.Generally in the oil and gas industry erosion engineers have realized what answer for configuration to forestall consumption dependent on the properties of the unrefined and the capacity region. Business pioneers in the oil and gas industry may be acquainted with the Oil & Gas Analytics Solutions in Saudi Arabia, referencing the enormous age hole in the oil and gas workforce, where most architects and geoscientists are either more than 55 or under 35. What this implies in genuine business terms is that oil and gas firms have not had the best procedure to catch and move the information from veteran architects in a repeatable way. Digitizing this information and conveying upkeep bits of knowledge to new designers may now be conceivable with AI.
Data Analysis
Huge oil and gas organizations work out of a few unique areas all around. Snappy access to their information records may be an essential piece of their business forms. By and large, these information records should be digitized and surveyed to distinguish issues, for example, absent or deficient records or unindexed records before they can be appropriately used. Oil & Gas Analytics Solutions in Saudi Arabia can help oil and gas organizations digitize records and mechanize the investigation of land information and outlines, conceivably prompting the ID of issues, for example, pipeline erosion or expanded hardware usage.A enormous piece of this information incorporates investigation, generation and repository information logs, for example, seismic reviews, well logs, regular and extraordinary center examinations, liquid investigations, static and streaming weight estimations, pressure-transient tests, intermittent well creation tests, records of the month to month delivered volumes of liquids (oil, gas, and water), and records of the month to month infused volumes of EOR liquids.
Exploration
The robot collects seismic data and is equipped to learn how to perform more accurately using machine learning. ExxonMobil claims these robots will move slowly a few feet above the ocean floor to detect and analyze naturally seeping hydrocarbons.Oil & Gas Analytics Solutions in Saudi Arabia could help companies potentially gain insights to improve business outcomes in their upstream processes by using AI software. This would involve feeding the software with the curated data records and information from data sources that could include structured documents, PDFs, handwritten notes, audio, or video files.
Human Safety
Another major challenge of the oil and gas industry is the threat to human safety, as well as the environment, during the drilling process. Harmful emissions during the extraction of the natural resources lead to severe health issues to the workers. With Oil & Gas Analytics Solutions in Saudi Arabia new resources and harsh remote locations can be identified. Robots can detect any faults in the equipment and send alerts in case of any leakages. Safety can be ensured by replacing personnel with robots , which can work in the dangerous and tedious environment. Thereby , optimising the performance and cost incurred.
Decision Making
Unstructured data like maintenance reports , weather reports , media reports etc can be analyzed with Oil & Gas Analytics Solutions in Saudi Arabia and facilitated with business solutions for an effective decision. Another approach of the system is in transforming and summarizing the safety meetings that can help workers in the decision making of a critical problem.
Services We Offer:
Strategy
Competitive Intelligence
Marketing Analytics
Sales Analytics
Data Monetization
Predictive Analytics
Planning
Assessments
Roadmaps
Data Governance
Strategy & Architecture
Organization Planning
Proof of Value
Analytics
Data Visualization
Big Data Analytics
Machine Learning
BI Reporting Dashboards
Advanced Analytics & Data Science
CRM / Salesforce Analytics
Data
Big Data Architecture
Lean Analytics
Enterprise Data Warehousing
Master Data Management
System Optimization
Outsourcing
Software Development
Managed Services
On-Shore / Off-Shore
Cloud Analytics
Recruiting & Staffing
Click to Start Whatsapp Chatbot with Sales
Mobile: +966547315697
Email: [email protected]
0 notes
Link
Artificial Intelligence is already impacting Manufacturing, Retail, Marketing, Healthcare, Food industries and more. Today we will take an in-depth look at another industry, that with proper AI expertise from development companies could be disrupted. Transportation is an industry that helps humanity with moving people their belongings from one location to the other. While doing that, this industry had experienced countless twists, turns, breakthroughs, and setbacks to get to the place where it is now. The year 1787 was the defining one for this industry because steamboat was introduced and changed everything. Transportation was not limited by the animal-drawn carts anymore. Years later more inventions followed like bicycles, trains, cars, and planes. In the year 2019, we have another milestone reached - vehicles can now move and navigate without human assistance at all. Recent technological advancements made it possible. Of course, one of these technologies is Artificial Intelligence, which already helps transportation lowering carbon emissions and reducing financial expenses. We already can say, that AI successfully transferred from Sci-Fi movies and TV shows to become our reality, despite many of us still don’t realize it. AI provides machines with human intelligence, to a certain degree, of course. Machines now can mimic humans, automate tasks, and learn from experience. Repetitive tasks can now be easily handled by machines. The learning feature will eventually lead AI to take on critical-thinking jobs and make informed and reasonable decisions. The world is watching, that’s why there are major investments going into the transportation sector. P&S Intelligence predicts that the global market for AI in transportation will reach 3.5 billion dollars by the year 2023. How did it get to that point? Let’s look at history. History of AI in Transportation: Self-Driving Cars In the 1930s there were the first mentions of self-driving cars concepts, in science fiction books of course. Since the 1960s AI developers were dealing with the challenge to build them, and while in the 2000s there were autonomous vehicles on Mars, self-driving cars were still prototypes in laboratories. So many factors occur on the road like traffic and actions of pedestrians, that what made driving in the city complex. While in 2000 some prototypes existed, there were few predictions they would get to mass production by 2015. However, in 2004 the very fast progress in Machine Learning for perception tasks and the evolution of the industry launched speedy progress which ultimately led us to this point. Google’s autonomous vehicles and Tesla’s semi-autonomous cars are already on the streets now. Google’s cars logged 300,000 miles without an accident and a total of 1,500,000 miles without any human input at all. Tesla is offering the self-driving capability to existing cars with the software update but this approach is questionable. The problem with semi-autonomous that human drivers are expected to engage when they are most needed, but they tend to rely too much on AI capabilities. This led to the first traffic fatality with an autonomous car in June 2016, which brought attention to this problem. Very soon sensing algorithms will surpass greatly human capabilities necessary for driving. Automated perception is already close to human’s, for recognition and tracking. Algorithm improvements in higher-level reasoning will follow, leading to a wide adoption of self-driving cars in 2020. While autonomous vehicles are the major part of our topic, there are more use cases we can talk about. AI in Transportation Examples While the level of adoption of Artificial Intelligence in different industries and countries varies, there is no denying that technology is a perfect fit for transportation. Look at the following examples. 1. Public Transportation of the Passengers and Traffic Management Companies around the world are already starting to implement autonomous buses to the infrastructure of the city, the best-known cases are from China, Singapore, and Finland. But different city infrastructures, weather conditions, road surfaces, etc., make AI applications of autonomous buses very dependent on the environment. Local Motors from the United States of America presented Olli - an electric shuttle that doesn’t need a driver. This company provides low volume manufacturing of the open-source vehicle design, relying on the variety of the micro-factories. Watson Internet of Things (IoT) for Automotive from IBM is the heart of the processes in Olli. The smart electric shuttle can transport people to the requested places, giving comments on local sights and answer questions on how it operates. There were five APIs from Watson IoT for Automotive platform: Text to Speech, Speech to Text, Entity Extraction, Conversation, and Natural Language Classifier. Artificial Intelligence is already implemented in resolving the problems in traffic control and traffic optimization area. More than that, we can also trace some use cases, were AI is dealing with prediction and detection of traffic accidents and conditions. This is achieved by combining traffic sensors and cameras. Surtrac from Rapid Flow is originated from the Robotics Institute at Carnegie Mellon University. Surtrac system was first tested in the Pittsburgh area. The idea of this system is installing a network of nine traffic signals in the three biggest roads. The reported results are: the reduction of the travel time by more than 25% and wait times by 40%. After this success, the local Pittsburgh government joined forces with Rapid Flow install up to 50 traffic signals to other parts of the city. 2. Autonomous Trucks Stricter emission regulations from the government and environmental challenges are forcing the industry to change. The International Transport Forum (ITF) reports that using autonomous trucks will save costs, improve road safety and lower emissions. A startup called Otto (now known as Uber Advanced Technologies Group after the $680 million purchase in 2017) was responsible for the first-ever delivery by autonomous truck in 2016. The truck was delivering 50,000 cans of Budweiser for the 120 miles distance. A Chinese startup TuSimple performed a level 4 test of the driverless truck for 200 miles in 2015. The truck’s system was trained using deep learning, simulating tens of millions of miles. 3. Railway Cargo Transportation General Electric has presented smart locomotives, to boost overall efficiency and the economic benefits of their rail transport solutions. GE’s locomotives are equipped with sensors and cameras, which gathers data for a Machine Learning application. The information is aggregated on the edge gateway, providing decision-making in real-time. General Electric already improved speed and accuracy in detecting things. Their first project resulted in a 25% reduction in locomotive failure. Benefits of AI in Transportation So here are some benefits that could come from implementing Artificial Intelligence in the transportation industry: Public safety - smart real-time crime data tracking is one of the ways to improve the safety of the passengers while using trains or buses. Improved planning - accurate prediction techniques could benefit road freight transport system, forecasting their volume using AI. Artificial Intelligence also can be used here for decision-making, introducing certain Machine Learning tools. Pedestrian safety - the path of cyclists and pedestrians could be predicted using AI. This will lead to a decrease in traffic injuries and accidents. Traffic flow control - AI will help to reduce congestion and streamline traffic patterns. More than that, real-time tracking can help to control traffic patterns more effectively. Future of AI in transportation In 2016 a call of proposals was released by the United States Department of Transportation (USDoT), asking medium-size cities to start imagining smart city infrastructure for transportation. The best city to do that is planned to receive 40 million dollars for the demonstration of AI potential in their city. Meanwhile, the US transportation research board claims that there following application of AI on transportation is emerging: city infrastructure design and planning, demand modeling for cargo and public transport and travel behavioral models. However, one of the major restraints of innovation is the privacy issue. Government and legal regulations could limit the speed of innovation and adoption in the industry. Conclusion AI innovation is closer than we think. According to the International Institute for Sustainable Development, the tests of completely autonomous trains for long distances are already running. 2.2 to 3.1 million driver jobs could be in danger of replacement in the USA by self-driving vehicles. On-demand car services like Uber will switch to driverless vehicles as soon as they can. Do you want to learn more about Artificial Intelligence and Machine Learning development? AI & ML technologies could elevate your business to an entirely new level. There are plenty of companies providing AI expertise. We researched evaluating Originally posted here
0 notes
Text
Improving Medical AI Safety by Addressing Hidden Stratification
Jared Dunnmon
Luke Oakden-Rayner
By LUKE OAKDEN-RAYNER MD, JARED DUNNMON, PhD
Medical AI testing is unsafe, and that isn’t likely to change anytime soon.
No regulator is seriously considering implementing “pharmaceutical style” clinical trials for AI prior to marketing approval, and evidence strongly suggests that pre-clinical testing of medical AI systems is not enough to ensure that they are safe to use. As discussed in a previous post, factors ranging from the laboratory effect to automation bias can contribute to substantial disconnects between pre-clinical performance of AI systems and downstream medical outcomes. As a result, we urgently need mechanisms to detect and mitigate the dangers that under-tested medical AI systems may pose in the clinic.
In a recent preprint co-authored with Jared Dunnmon from Chris Ré’s group at Stanford, we offer a new explanation for the discrepancy between pre-clinical testing and downstream outcomes: hidden stratification. Before explaining what this means, we want to set the scene by saying that this effect appears to be pervasive, underappreciated, and could lead to serious patient harm even in AI systems that have been approved by regulators.
But there is an upside here as well. Looking at the failures of pre-clinical testing through the lens of hidden stratification may offer us a way to make regulation more effective, without overturning the entire system and without dramatically increasing the compliance burden on developers.
What’s in a stratum?
We recently published a pre-print titled “Hidden Stratification Causes Clinically Meaningful Failures in Machine Learning for Medical Imaging“.
Note: While this post discusses a few parts of this paper, it is more intended to explore the implications. If you want to read more about the effect and our experiments, please read the paper
The effect we describe in this work — hidden stratification — is not really a surprise to anyone. Simply put, there are subsets within any medical task that are visually and clinically distinct. Pneumonia, for instance, can be typical or atypical. A lung tumour can be solid or subsolid. Fractures can be simple or compound. Such variations within a single diagnostic category are often visually distinct on imaging, and have fundamentally different implications for patient care.

Examples of different lung nodules, ranging from solid (a), solid with a halo (b), and subsolid (c). Not only do these nodules look different, they reflect different diseases with different patient outcomes.
We also recognise purely visual variants. A pleural effusion looks different if the patient is standing up or is lying down, despite the pathology and clinical outcomes being the same.
These patients both have left sided pleural effusions (seen on the right of each image). The patient on the left has increased density at the left lung base, whereas the patient on the right has a subtle “veil” across the entire left lung.
These visual variants can cause problems for human doctors, but we recognise their presence and try to account for them. This is rarely the case for AI systems though, as we usually train AI models on coarsely defined class labels and this variation is unacknowledged in training and testing; in other words, the the stratification is hidden (the term “hidden stratification” actually has its roots in genetics, describing the unrecognised variation within populations that complicates all genomic analyses).
The main point of our paper is that these visually distinct subsets can seriously distort the decision making of AI systems, potentially leading to a major difference between performance testing results and clinical utility.
Clinical safety isn’t about average performance
The most important concept underpinning this work is that being as good as a human on average is not a strong predictor of safety. What matters far more is specifically which cases the models get wrong.
For example, even cutting-edge deep learning systems make such systematic misjudgments as consistently classifying canines in the snow as wolves or men as computer programmers and women as homemakers. This “lack of common sense” effect is often treated as an expected outcome of data-driven learning, which is undesirable but ultimately acceptable in deployed models outside of medicine (though even then, these effects have caused major problems for sophisticated technology companies).
Whatever the risk is in the non-medical world, we argue that in healthcare this same phenomenon can have serious implications.
Take for example a situation where humans and an AI system are trying to diagnose cancer, and they show equivalent performance in a head-to-head “reader” study. Let’s assume this study was performed perfectly, with a large external dataset and a primary metric that was clinically motivated (perhaps the true positive rate in a screening scenario). This is the current level of evidence required for FDA approval, even for an autonomous system.
Now, for the sake of the argument, let’s assume the TPR of both decision makers is 95%. Our results to report to the FDA probably look like this:
TPR is the same thing as sensitivity/recall
That looks good, our primary measure (assuming a decent sample size) suggests that the AI and human are performing equivalently. The FDA should be pretty happyª.
Now, let’s also assume that the majority of cancer is fairly benign and small delays in treatment are inconsequential, but that there is a rare and visually distinct cancer subtype making up 5% of all disease that is aggressive and any delay in diagnosis leads to drastically shortened life expectancy.
There is a pithy bit of advice we often give trainee doctors: when you hear hoofbeats, think horses, not zebras. This means that you shouldn’t jump to diagnosing the rare subtype, when the common disease is much more likely. This is also exactly what machine learning models do – they consider prior probability and the presence of predictive features but, unless it has been explicitly incorporated into the model, they don’t consider the cost of their errors.
This can be a real problem in medical AI, because there is a less commonly shared addendum to this advice: if zebras were stone cold killing machines, you might want to exclude zebras first. The cost of misidentifying a dangerous zebra is much more than that of missing a gentle pony. No-one wants to get hoofed to death.
In practice, human doctors will be hyper-vigilant about the high-risk subtype, even though it is rare. They will have spent a disproportionate amount of time and effort learning to identify it, and will have a low threshold for diagnosing it (in this scenario, we might assume that the cost of overdiagnosis is minimal).
If we assume the cancer-detecting AI system was developed as is common practice, it probably was trained to detect “cancer” as a monolithic group. Since only 5% of the training samples included visual features of this subtype, and no-one has incorporated the expected clinical cost of misdiagnosis into the model, how do we expect it to perform in this important subset of cases?
Fairly obviously, it won’t be hypervigilant – it was never informed that it needed to be. Even worse, given the lower number of training examples in the minority subtype, it will probably underperform for this subset (since performance on a particular class or subset should increase with more training examples from that class). We might even expect that a human would get the majority of these cases right, and that the AI might get the majority wrong. In our paper, we show that existing AI models do indeed show concerning error rates on clinically important subsets despite encouraging aggregate performance metrics.
In this hypothetical, the human and the AI have the same average performance, but the AI specifically fails to recognise the critically important cases (marked in red). The human makes mistakes in less important cases, which is fairly typical in diagnostic practice.
In this setting, even though the doctors and the AI have the same overall performance (justifying regulatory approval), using the AI would lead to delayed diagnosis in the cases where such a delay is critically important. It would kill patients, and we would have no way to predict this with current testing.
Predicting where AI fails
So, how can we mitigate this risk? There are lots of clever computer scientists trying to make computers smart enough to avoid the problem (see: algorithmic robustness/fairness, causal machine learning, invariant learning etc.), but we don’t necessarily have to be this fancy^. If the problem is that performance may be worse in clinically important subsets, then all we might need to do is identify those subsets and test their performance.
In our example above, we can simply label all the “aggressive sub-type” cases in the cancer test set, and then evaluate model performance on that subset. Then our results (to report to the FDA would be):
As you might expect, these results would be treated very differently by a regulator, as this now looks like an absurdly unsafe AI system. This “stratified” testing tells us far more about the safety of this system than the overall or average performance for a medical task.
So, the low-tech solution is obvious – you identify all possible variants in the data and label them in the test set. In this way, a safe system is one that shows human-level performance in the overall task as well as in the subsets.
We call this approach schema completion. A schema (or ontology) in this context is the label structure, defining the relationships between superclasses (the large, coarse classes) and subclasses (the fine-grained subsets). We have actually seen well-formed schemas in medical AI research before, for example in the famous 2017 Nature paper Dermatologist-level classification of skin cancer with deep neural networks by Esteva et al. They produced a complex tree structure defining the class relationships, and even if this is not complete, it is certainly much better than pretending that all of the variation in skin lesions is explained by “malignant” and “not malignant” labels.

So why doesn’t everyone test on complete schema? Two reasons:
There aren’t enough test cases (in this dermatology example, they only tested on the three red super-classes). If you had to have well-powered test sets for every subtype, you would need more data than in your training set!
There are always more subclasses*. In the paper, Esteva et al describe over 2000 diagnostic categories in their dataset! Even then they didn’t include all of the important visual subsets in their schema, for example we have seen similar models fail when skin markers are present.
So testing all the subsets seems untenable. What can we do?
We think that we can rationalise the problem. If we knew what subsets are likely to be “underperformers”, and we use our medical knowledge to determine which subsets are high-risk, then we only need to test on the intersection between these two groups. We can predict the specific subsets where AI could clinically fail, and then only need to target these subsets for further analysis.
In our paper, we identified three main factors that appear to lead to underperformance. Across multiple datasets, we find evidence that hidden stratification leads to poor performance when there are subsets characterized by low subset prevalence, poor subset label quality, and/or subtle discriminative features (when the subset looks more like a different class than the class that it actually belongs to).
An example from the paper using the MURA dataset. Relabeled, we see that metalwork (left) is visually the most obvious finding (it looks the least like a normal x-ray out of the subclasses). Fractures (middle) can be subtle, and degenerative disease (right) is both subtle and inconsistently labeled. A model trained on the normal/abnormal superclasses significantly underperforms on cases within the subtle and noisy subclasses.
Putting it into practice
So we think we know how to recognise problematic subsets.
To actually operationalise this, we doctors would sit down and write out a complete schema for any and all medical AI tasks. Given the broad range of variation, covering clinical, pathological, and visual subsets, this would be a huge undertaking. Thankfully, it only needs to be done once (and updated rarely), and this is exactly the sort of work that is performed by large professional bodies (like ACR, ESR, RSNA), who regularly form working groups of domain specialists to tackle these kind of problems^^.

The nicest thing you can say about being in a working group is that someone’s gotta do it.
With these expert-defined schema, we would then highlight the subsets which may cause problems – those that are likely to underperform due to the factors we have identified in our research, and those that are high risk based on our clinical knowledge. Ideally there will be only a few “subsets of concern” per task that fulfil these criteria.
Then we present this ontology to the regulators and say “for an AI system to be judged safe for task X, we need to know the performance in the subsets of concern Y and Z.” In this way, a pneumothorax detector would need to show performance in cases without chest tubes, a fracture detector would need to be equal to humans for subtle fractures as well as obvious ones, and a “normal-case detector” (don’t get Luke started) would need to show that it doesn’t miss serious diseases.
To make this more clear, let’s consider a simple example. Here is a quick attempt at a pneumothorax schema:
Subsets of concern in red, conditional subsets of concern in orange (depends on exact implementation of model and data)
Pneumothorax is a tricky one since they are all “high risk” if they are untreated (meaning you end up with more subsets of concern than in many tasks), but we think this gives a general feel for what schema completion might look like.
The beauty of this approach is that it would work within the current regulatory framework, and as long as there aren’t too many subsets of concern the compliance cost should be low. If you already have enough cases for subset testing, then the only cost to the developer would be producing the labels, which would be relatively small.
If the subsets of concern in the existing test set are too small for valid performance results, then there is a clear path forward – you need to enrich for those subsets (i.e., not gather ten thousand more random cases). While this does carry a compliance cost, since you only need to do this for a small handful of subsets, the cost is also likely to be small compared to the overall cost of development. Sourcing the cases could get tricky if they are rare, but this is not insurmountable.
The only major cost to developers when implementing a system like this is if they find out that their algorithm is unsafe, and it needs to be retrained with specific subsets in mind. Since this is absolutely the entire point of regulation, we’d call this a reasonable cost of doing business.
In fact, since this list of subsets of concern would be widely available, developers could decide on their AI targets informed of the associated risks – if they don’t think they can adequately test for performance in a subset of concern, they can target a different medical task. This is giving developers have been asking for – they say they want more robust regulation and better assurances of safety, as long as the costs are transparent and the playing field is level.
How much would it help?
We see this “low-tech” approach to strengthen pre-clinical testing as a trade-off between being able to measure the actual clinical costs of using AI (as you would in a clinical trial) and the realities of device regulation. By identifying strata that are likely to produce worse clinical outcomes, we should be able to get closer to the safety profile delivered by gold standard clinical testing, without massively inflating costs or upending the current regulatory system.
This is certainly no panacea. There will always be subclasses and edge cases that we simply can’t test preclinically, perhaps because they aren’t recognised in our knowledge base or because examples of the strata aren’t present within our dataset. We also can’t assess the effects of the other causes of clinical underperformance, such as the laboratory effect and automation bias.
To close this safety gap, we still need to rely on post-deployment monitoring.
A promising direction for post-deployment monitoring is the AI audit, a process where human experts monitor the performance and particularly the errors of AI systems in clinical environments, in effect estimating the harm caused by AI in real-time. The need for this sort of monitoring has been recognised by professional organisations, who are grappling with the idea that we will need a new sort of specialist – a chief medical information officer who is skilled in AI monitoring and assessment – embedded in every practice (for example, see section 3 of the proposed RANZCR Standards of Practice for Artificial Intelligence).
Auditors are the real superheros
Audit works by having human experts review examples of AI predictions, and trying to piece together an explanation for the errors. This can be performed with image review alone or in combination with other interpretability techniques, but either way error auditing is critically dependent on the ability of the auditor to visually appreciate the differences in the distribution of model outputs. This approach is limited to the recognition of fairly large effects (i.e., effects that are noticeable in a modest/human-readable sample of images) and it will almost certainly be less exhaustive than prospectively assessing a complete schema defined by an expert panel. That being said, this process can still be extremely useful. In our paper, we show that human audit was able to detect hidden stratification that caused the performance of a CheXNet-reproduction model to drop by over 15% ROC-AUC on pneumothorax cases without chest drains — the subset that’s most important! — with respect to those that had already been treated with a chest drain.
Thankfully, the two testing approaches we’ve described are synergistic. Having a complete schema is useful for audit; instead of laboriously (and idiosyncratically) searching for meaning in sets of images, we can start our audit with the major subsets of concern. Discovering new and unexpected stratification would only occur when there are clusters of errors which do not conform to the existing schema, and these newly identified subsets of concern could be folded back into the schema via a reporting mechanism.
Looking to the future, we also suggest in our paper that we might be able to automate some of the audit process, or at least augment it with machine learning. We show that even simple k-means clustering in the model feature space can be effective in revealing important subsets in some tasks (but not others). We call this approach to subset discovery algorithmic measurement, and anticipate that further development of these ideas may be useful in supplementing schema completion and human audit. We have begun to explore more effective techniques for algorithmic measurement that may work better than k-means, but that is a topic for another day :).
Making AI safe(r)
These techniques alone won’t make medical AI safe, because they can’t replace all the benefits of proper clinical testing of AI. Risk-critical systems in particular need randomised control trials, and our demonstration of hidden stratification in common medical AI tasks only reinforces this point. The problem is that there is no path from here to there. It is possible that RCTs won’t even be considered until after we have a medical AI tragedy, and by then it will be too late.
In this context, we believe that pre-marketing targeted subset testing combined with post-deployment monitoring could serve as an important and effective stopgap for improving AI safety. It is low tech, achievable, and doesn’t create a huge compliance burden. It doesn’t ask the healthcare systems and governments of the world to overhaul their current processes, just to take a bit of advice on what specific questions need to be asked for any given medical task. By delivering a consensus schema to regulators on a platter, they might even use it.
And maybe this approach is more broadly attractive as well. AI is not human — inhuman, in fact — in how it makes decisions. While it is attractive to work towards human-like intelligence in our computer systems, it is impossible to predict if and when this might be feasible.
The takeaway here is that subset-based testing and monitoring is one way we can bring human knowledge and common sense into medical machine learning systems, completely separate from the mathematical guts of the models. We might even be able to make them safer without making them smarter, without teaching them to ask why, and without rebooting AI.
Luke’s footnotes:
ª The current FDA position on the clinical evaluation of medical software (pdf link) is: “…prior to product launch (pre-market) the manufacturer generates evidence of the product’s accuracy, specificity, sensitivity, reliability, limitations, and scope of use in the intended use environment with the intended user, and generates a SaMD definition statement. Once the product is on the market (post-market), as part of normal lifecycle management processes, the manufacturer continues to collect real world performance data (e.g., complaints, safety data)…”
^ I am planning to do a follow-up post on this idea – that we don’t always need to default to looking for not yet developed, possibly decades away technological solutions when the problem can be immediately solved with a bit of human effort.
^^ A possibly valid alternative would be crowd-sourcing these schema. This would have to be done very carefully to be considered authoritative enough to justify including in regulatory frameworks, but could happen much quicker than the more formal approach.
* I’ve heard this described as “subset whack-a-mole”, or my own phrasing: “there are subsets all the way down”.**
** I love that I have finally included a Terry Pratchett reference in my Pratchett-esque footnotes.
Luke Oakden-Rayner is a radiologist (medical specialist) in South Australia, undertaking a Ph.D in Medicine with the School of Public Health at the University of Adelaide.
Jared Dunnmon is a post-doctoral fellow at Stanford University where he researches the development of weakly supervised machine learning techniques and application to problems in human health, energy & environment, and national security.
This post originally appeared on Luke’s blog here.
Improving Medical AI Safety by Addressing Hidden Stratification published first on https://venabeahan.tumblr.com
0 notes
Text
Improving Medical AI Safety by Addressing Hidden Stratification
Jared Dunnmon
Luke Oakden-Rayner
By LUKE OAKDEN-RAYNER MD, JARED DUNNMON, PhD
Medical AI testing is unsafe, and that isn’t likely to change anytime soon.
No regulator is seriously considering implementing “pharmaceutical style” clinical trials for AI prior to marketing approval, and evidence strongly suggests that pre-clinical testing of medical AI systems is not enough to ensure that they are safe to use. As discussed in a previous post, factors ranging from the laboratory effect to automation bias can contribute to substantial disconnects between pre-clinical performance of AI systems and downstream medical outcomes. As a result, we urgently need mechanisms to detect and mitigate the dangers that under-tested medical AI systems may pose in the clinic.
In a recent preprint co-authored with Jared Dunnmon from Chris Ré’s group at Stanford, we offer a new explanation for the discrepancy between pre-clinical testing and downstream outcomes: hidden stratification. Before explaining what this means, we want to set the scene by saying that this effect appears to be pervasive, underappreciated, and could lead to serious patient harm even in AI systems that have been approved by regulators.
But there is an upside here as well. Looking at the failures of pre-clinical testing through the lens of hidden stratification may offer us a way to make regulation more effective, without overturning the entire system and without dramatically increasing the compliance burden on developers.
What’s in a stratum?
We recently published a pre-print titled “Hidden Stratification Causes Clinically Meaningful Failures in Machine Learning for Medical Imaging“.
Note: While this post discusses a few parts of this paper, it is more intended to explore the implications. If you want to read more about the effect and our experiments, please read the paper
The effect we describe in this work — hidden stratification — is not really a surprise to anyone. Simply put, there are subsets within any medical task that are visually and clinically distinct. Pneumonia, for instance, can be typical or atypical. A lung tumour can be solid or subsolid. Fractures can be simple or compound. Such variations within a single diagnostic category are often visually distinct on imaging, and have fundamentally different implications for patient care.

Examples of different lung nodules, ranging from solid (a), solid with a halo (b), and subsolid (c). Not only do these nodules look different, they reflect different diseases with different patient outcomes.
We also recognise purely visual variants. A pleural effusion looks different if the patient is standing up or is lying down, despite the pathology and clinical outcomes being the same.
These patients both have left sided pleural effusions (seen on the right of each image). The patient on the left has increased density at the left lung base, whereas the patient on the right has a subtle “veil” across the entire left lung.
These visual variants can cause problems for human doctors, but we recognise their presence and try to account for them. This is rarely the case for AI systems though, as we usually train AI models on coarsely defined class labels and this variation is unacknowledged in training and testing; in other words, the the stratification is hidden (the term “hidden stratification” actually has its roots in genetics, describing the unrecognised variation within populations that complicates all genomic analyses).
The main point of our paper is that these visually distinct subsets can seriously distort the decision making of AI systems, potentially leading to a major difference between performance testing results and clinical utility.
Clinical safety isn’t about average performance
The most important concept underpinning this work is that being as good as a human on average is not a strong predictor of safety. What matters far more is specifically which cases the models get wrong.
For example, even cutting-edge deep learning systems make such systematic misjudgments as consistently classifying canines in the snow as wolves or men as computer programmers and women as homemakers. This “lack of common sense” effect is often treated as an expected outcome of data-driven learning, which is undesirable but ultimately acceptable in deployed models outside of medicine (though even then, these effects have caused major problems for sophisticated technology companies).
Whatever the risk is in the non-medical world, we argue that in healthcare this same phenomenon can have serious implications.
Take for example a situation where humans and an AI system are trying to diagnose cancer, and they show equivalent performance in a head-to-head “reader” study. Let’s assume this study was performed perfectly, with a large external dataset and a primary metric that was clinically motivated (perhaps the true positive rate in a screening scenario). This is the current level of evidence required for FDA approval, even for an autonomous system.
Now, for the sake of the argument, let’s assume the TPR of both decision makers is 95%. Our results to report to the FDA probably look like this:
TPR is the same thing as sensitivity/recall
That looks good, our primary measure (assuming a decent sample size) suggests that the AI and human are performing equivalently. The FDA should be pretty happyª.
Now, let’s also assume that the majority of cancer is fairly benign and small delays in treatment are inconsequential, but that there is a rare and visually distinct cancer subtype making up 5% of all disease that is aggressive and any delay in diagnosis leads to drastically shortened life expectancy.
There is a pithy bit of advice we often give trainee doctors: when you hear hoofbeats, think horses, not zebras. This means that you shouldn’t jump to diagnosing the rare subtype, when the common disease is much more likely. This is also exactly what machine learning models do – they consider prior probability and the presence of predictive features but, unless it has been explicitly incorporated into the model, they don’t consider the cost of their errors.
This can be a real problem in medical AI, because there is a less commonly shared addendum to this advice: if zebras were stone cold killing machines, you might want to exclude zebras first. The cost of misidentifying a dangerous zebra is much more than that of missing a gentle pony. No-one wants to get hoofed to death.
In practice, human doctors will be hyper-vigilant about the high-risk subtype, even though it is rare. They will have spent a disproportionate amount of time and effort learning to identify it, and will have a low threshold for diagnosing it (in this scenario, we might assume that the cost of overdiagnosis is minimal).
If we assume the cancer-detecting AI system was developed as is common practice, it probably was trained to detect “cancer” as a monolithic group. Since only 5% of the training samples included visual features of this subtype, and no-one has incorporated the expected clinical cost of misdiagnosis into the model, how do we expect it to perform in this important subset of cases?
Fairly obviously, it won’t be hypervigilant – it was never informed that it needed to be. Even worse, given the lower number of training examples in the minority subtype, it will probably underperform for this subset (since performance on a particular class or subset should increase with more training examples from that class). We might even expect that a human would get the majority of these cases right, and that the AI might get the majority wrong. In our paper, we show that existing AI models do indeed show concerning error rates on clinically important subsets despite encouraging aggregate performance metrics.
In this hypothetical, the human and the AI have the same average performance, but the AI specifically fails to recognise the critically important cases (marked in red). The human makes mistakes in less important cases, which is fairly typical in diagnostic practice.
In this setting, even though the doctors and the AI have the same overall performance (justifying regulatory approval), using the AI would lead to delayed diagnosis in the cases where such a delay is critically important. It would kill patients, and we would have no way to predict this with current testing.
Predicting where AI fails
So, how can we mitigate this risk? There are lots of clever computer scientists trying to make computers smart enough to avoid the problem (see: algorithmic robustness/fairness, causal machine learning, invariant learning etc.), but we don’t necessarily have to be this fancy^. If the problem is that performance may be worse in clinically important subsets, then all we might need to do is identify those subsets and test their performance.
In our example above, we can simply label all the “aggressive sub-type” cases in the cancer test set, and then evaluate model performance on that subset. Then our results (to report to the FDA would be):
As you might expect, these results would be treated very differently by a regulator, as this now looks like an absurdly unsafe AI system. This “stratified” testing tells us far more about the safety of this system than the overall or average performance for a medical task.
So, the low-tech solution is obvious – you identify all possible variants in the data and label them in the test set. In this way, a safe system is one that shows human-level performance in the overall task as well as in the subsets.
We call this approach schema completion. A schema (or ontology) in this context is the label structure, defining the relationships between superclasses (the large, coarse classes) and subclasses (the fine-grained subsets). We have actually seen well-formed schemas in medical AI research before, for example in the famous 2017 Nature paper Dermatologist-level classification of skin cancer with deep neural networks by Esteva et al. They produced a complex tree structure defining the class relationships, and even if this is not complete, it is certainly much better than pretending that all of the variation in skin lesions is explained by “malignant” and “not malignant” labels.

So why doesn’t everyone test on complete schema? Two reasons:
There aren’t enough test cases (in this dermatology example, they only tested on the three red super-classes). If you had to have well-powered test sets for every subtype, you would need more data than in your training set!
There are always more subclasses*. In the paper, Esteva et al describe over 2000 diagnostic categories in their dataset! Even then they didn’t include all of the important visual subsets in their schema, for example we have seen similar models fail when skin markers are present.
So testing all the subsets seems untenable. What can we do?
We think that we can rationalise the problem. If we knew what subsets are likely to be “underperformers”, and we use our medical knowledge to determine which subsets are high-risk, then we only need to test on the intersection between these two groups. We can predict the specific subsets where AI could clinically fail, and then only need to target these subsets for further analysis.
In our paper, we identified three main factors that appear to lead to underperformance. Across multiple datasets, we find evidence that hidden stratification leads to poor performance when there are subsets characterized by low subset prevalence, poor subset label quality, and/or subtle discriminative features (when the subset looks more like a different class than the class that it actually belongs to).
An example from the paper using the MURA dataset. Relabeled, we see that metalwork (left) is visually the most obvious finding (it looks the least like a normal x-ray out of the subclasses). Fractures (middle) can be subtle, and degenerative disease (right) is both subtle and inconsistently labeled. A model trained on the normal/abnormal superclasses significantly underperforms on cases within the subtle and noisy subclasses.
Putting it into practice
So we think we know how to recognise problematic subsets.
To actually operationalise this, we doctors would sit down and write out a complete schema for any and all medical AI tasks. Given the broad range of variation, covering clinical, pathological, and visual subsets, this would be a huge undertaking. Thankfully, it only needs to be done once (and updated rarely), and this is exactly the sort of work that is performed by large professional bodies (like ACR, ESR, RSNA), who regularly form working groups of domain specialists to tackle these kind of problems^^.

The nicest thing you can say about being in a working group is that someone’s gotta do it.
With these expert-defined schema, we would then highlight the subsets which may cause problems – those that are likely to underperform due to the factors we have identified in our research, and those that are high risk based on our clinical knowledge. Ideally there will be only a few “subsets of concern” per task that fulfil these criteria.
Then we present this ontology to the regulators and say “for an AI system to be judged safe for task X, we need to know the performance in the subsets of concern Y and Z.” In this way, a pneumothorax detector would need to show performance in cases without chest tubes, a fracture detector would need to be equal to humans for subtle fractures as well as obvious ones, and a “normal-case detector” (don’t get Luke started) would need to show that it doesn’t miss serious diseases.
To make this more clear, let’s consider a simple example. Here is a quick attempt at a pneumothorax schema:
Subsets of concern in red, conditional subsets of concern in orange (depends on exact implementation of model and data)
Pneumothorax is a tricky one since they are all “high risk” if they are untreated (meaning you end up with more subsets of concern than in many tasks), but we think this gives a general feel for what schema completion might look like.
The beauty of this approach is that it would work within the current regulatory framework, and as long as there aren’t too many subsets of concern the compliance cost should be low. If you already have enough cases for subset testing, then the only cost to the developer would be producing the labels, which would be relatively small.
If the subsets of concern in the existing test set are too small for valid performance results, then there is a clear path forward – you need to enrich for those subsets (i.e., not gather ten thousand more random cases). While this does carry a compliance cost, since you only need to do this for a small handful of subsets, the cost is also likely to be small compared to the overall cost of development. Sourcing the cases could get tricky if they are rare, but this is not insurmountable.
The only major cost to developers when implementing a system like this is if they find out that their algorithm is unsafe, and it needs to be retrained with specific subsets in mind. Since this is absolutely the entire point of regulation, we’d call this a reasonable cost of doing business.
In fact, since this list of subsets of concern would be widely available, developers could decide on their AI targets informed of the associated risks – if they don’t think they can adequately test for performance in a subset of concern, they can target a different medical task. This is giving developers have been asking for – they say they want more robust regulation and better assurances of safety, as long as the costs are transparent and the playing field is level.
How much would it help?
We see this “low-tech” approach to strengthen pre-clinical testing as a trade-off between being able to measure the actual clinical costs of using AI (as you would in a clinical trial) and the realities of device regulation. By identifying strata that are likely to produce worse clinical outcomes, we should be able to get closer to the safety profile delivered by gold standard clinical testing, without massively inflating costs or upending the current regulatory system.
This is certainly no panacea. There will always be subclasses and edge cases that we simply can’t test preclinically, perhaps because they aren’t recognised in our knowledge base or because examples of the strata aren’t present within our dataset. We also can’t assess the effects of the other causes of clinical underperformance, such as the laboratory effect and automation bias.
To close this safety gap, we still need to rely on post-deployment monitoring.
A promising direction for post-deployment monitoring is the AI audit, a process where human experts monitor the performance and particularly the errors of AI systems in clinical environments, in effect estimating the harm caused by AI in real-time. The need for this sort of monitoring has been recognised by professional organisations, who are grappling with the idea that we will need a new sort of specialist – a chief medical information officer who is skilled in AI monitoring and assessment – embedded in every practice (for example, see section 3 of the proposed RANZCR Standards of Practice for Artificial Intelligence).
Auditors are the real superheros
Audit works by having human experts review examples of AI predictions, and trying to piece together an explanation for the errors. This can be performed with image review alone or in combination with other interpretability techniques, but either way error auditing is critically dependent on the ability of the auditor to visually appreciate the differences in the distribution of model outputs. This approach is limited to the recognition of fairly large effects (i.e., effects that are noticeable in a modest/human-readable sample of images) and it will almost certainly be less exhaustive than prospectively assessing a complete schema defined by an expert panel. That being said, this process can still be extremely useful. In our paper, we show that human audit was able to detect hidden stratification that caused the performance of a CheXNet-reproduction model to drop by over 15% ROC-AUC on pneumothorax cases without chest drains — the subset that’s most important! — with respect to those that had already been treated with a chest drain.
Thankfully, the two testing approaches we’ve described are synergistic. Having a complete schema is useful for audit; instead of laboriously (and idiosyncratically) searching for meaning in sets of images, we can start our audit with the major subsets of concern. Discovering new and unexpected stratification would only occur when there are clusters of errors which do not conform to the existing schema, and these newly identified subsets of concern could be folded back into the schema via a reporting mechanism.
Looking to the future, we also suggest in our paper that we might be able to automate some of the audit process, or at least augment it with machine learning. We show that even simple k-means clustering in the model feature space can be effective in revealing important subsets in some tasks (but not others). We call this approach to subset discovery algorithmic measurement, and anticipate that further development of these ideas may be useful in supplementing schema completion and human audit. We have begun to explore more effective techniques for algorithmic measurement that may work better than k-means, but that is a topic for another day :).
Making AI safe(r)
These techniques alone won’t make medical AI safe, because they can’t replace all the benefits of proper clinical testing of AI. Risk-critical systems in particular need randomised control trials, and our demonstration of hidden stratification in common medical AI tasks only reinforces this point. The problem is that there is no path from here to there. It is possible that RCTs won’t even be considered until after we have a medical AI tragedy, and by then it will be too late.
In this context, we believe that pre-marketing targeted subset testing combined with post-deployment monitoring could serve as an important and effective stopgap for improving AI safety. It is low tech, achievable, and doesn’t create a huge compliance burden. It doesn’t ask the healthcare systems and governments of the world to overhaul their current processes, just to take a bit of advice on what specific questions need to be asked for any given medical task. By delivering a consensus schema to regulators on a platter, they might even use it.
And maybe this approach is more broadly attractive as well. AI is not human — inhuman, in fact — in how it makes decisions. While it is attractive to work towards human-like intelligence in our computer systems, it is impossible to predict if and when this might be feasible.
The takeaway here is that subset-based testing and monitoring is one way we can bring human knowledge and common sense into medical machine learning systems, completely separate from the mathematical guts of the models. We might even be able to make them safer without making them smarter, without teaching them to ask why, and without rebooting AI.
Luke’s footnotes:
ª The current FDA position on the clinical evaluation of medical software (pdf link) is: “…prior to product launch (pre-market) the manufacturer generates evidence of the product’s accuracy, specificity, sensitivity, reliability, limitations, and scope of use in the intended use environment with the intended user, and generates a SaMD definition statement. Once the product is on the market (post-market), as part of normal lifecycle management processes, the manufacturer continues to collect real world performance data (e.g., complaints, safety data)…”
^ I am planning to do a follow-up post on this idea – that we don’t always need to default to looking for not yet developed, possibly decades away technological solutions when the problem can be immediately solved with a bit of human effort.
^^ A possibly valid alternative would be crowd-sourcing these schema. This would have to be done very carefully to be considered authoritative enough to justify including in regulatory frameworks, but could happen much quicker than the more formal approach.
* I’ve heard this described as “subset whack-a-mole”, or my own phrasing: “there are subsets all the way down”.**
** I love that I have finally included a Terry Pratchett reference in my Pratchett-esque footnotes.
Luke Oakden-Rayner is a radiologist (medical specialist) in South Australia, undertaking a Ph.D in Medicine with the School of Public Health at the University of Adelaide.
Jared Dunnmon is a post-doctoral fellow at Stanford University where he researches the development of weakly supervised machine learning techniques and application to problems in human health, energy & environment, and national security.
This post originally appeared on Luke’s blog here.
Improving Medical AI Safety by Addressing Hidden Stratification published first on https://wittooth.tumblr.com/
0 notes