#data science using tableau
Explore tagged Tumblr posts
Text
What EDAV does:
Connects people with data faster. It does this in a few ways. EDAV:
Hosts tools that support the analytics work of over 3,500 people.
Stores data on a common platform that is accessible to CDC's data scientists and partners.
Simplifies complex data analysis steps.
Automates repeatable tasks, such as dashboard updates, freeing up staff time and resources.
Keeps data secure. Data represent people, and the privacy of people's information is critically important to CDC. EDAV is hosted on CDC's Cloud to ensure data are shared securely and that privacy is protected.
Saves time and money. EDAV services can quickly and easily scale up to meet surges in demand for data science and engineering tools, such as during a disease outbreak. The services can also scale down quickly, saving funds when demand decreases or an outbreak ends.
Trains CDC's staff on new tools. EDAV hosts a Data Academy that offers training designed to help our workforce build their data science skills, including self-paced courses in Power BI, R, Socrata, Tableau, Databricks, Azure Data Factory, and more.
Changes how CDC works. For the first time, EDAV offers CDC's experts a common set of tools that can be used for any disease or condition. It's ready to handle "big data," can bring in entirely new sources of data like social media feeds, and enables CDC's scientists to create interactive dashboards and apply technologies like artificial intelligence for deeper analysis.
4 notes
·
View notes
Text
Data Science Courses | Manual and Automation Testing Course
Cinute Digital Pvt. Ltd. is the leading premium software testing and IT training institute located in Mira Road, Mumbai. We offer in-demand online and on-premise courses including Manual and Automation Software Testing, API Testing with Postman, Data Science and Machine Learning using Python and R, SQL, Big Data and Hadoop, Business Intelligence and data visualization with Tableau, PowerBI and Excel, Prompt Engineering, Python and Java Programming, Database Management Systems, and ISTQB Certification. Our State-Of-The-Art training center provides students with hands-on, practical skills combined with theory, concepts and live projects. Taught by industry experts, our courses prepare students for successful careers in IT, software testing, data science, and beyond. Join Cinute Digital for the most most up-to date and cutting-edge training!
2 notes
·
View notes
Text
Business Analytics vs. Data Science: Understanding the Key Differences
In today's data-driven world, terms like "business analytics" and "data science" are often used interchangeably. However, while they share a common goal of extracting insights from data, they are distinct fields with different focuses and methodologies. Let's break down the key differences to help you understand which path might be right for you.
Business Analytics: Focusing on the Present and Past
Business analytics primarily focuses on analyzing historical data to understand past performance and inform current business decisions. It aims to answer questions like:
What happened?
Why did it happen?
What is happening now?
Key characteristics of business analytics:
Descriptive and Diagnostic: It uses techniques like reporting, dashboards, and data visualization to summarize and explain past trends.
Structured Data: It often works with structured data from databases and spreadsheets.
Business Domain Expertise: A strong understanding of the specific business domain is crucial.
Tools: Business analysts typically use tools like Excel, SQL, Tableau, and Power BI.
Focus: Optimizing current business operations and improving efficiency.
Data Science: Predicting the Future and Building Models
Data science, on the other hand, focuses on building predictive models and developing algorithms to forecast future outcomes. It aims to answer questions like:
What will happen?
How can we make it happen?
Key characteristics of data science:
Predictive and Prescriptive: It uses machine learning, statistical modeling, and AI to predict future trends and prescribe optimal actions.
Unstructured and Structured Data: It can handle both structured and unstructured data from various sources.
Technical Proficiency: Strong programming skills (Python, R) and a deep understanding of machine learning algorithms are essential.
Tools: Data scientists use programming languages, machine learning libraries, and big data technologies.
Focus: Developing innovative solutions, building AI-powered products, and driving long-term strategic initiatives.
Key Differences Summarized:

Which Path is Right for You?
Choose Business Analytics if:
You are interested in analyzing past data to improve current business operations.
You have a strong understanding of a specific business domain.
You prefer working with structured data and using visualization tools.
Choose Data Science if:
You are passionate about building predictive models and developing AI-powered solutions.
You have a strong interest in programming and machine learning.
You enjoy working with both structured and unstructured data.
Xaltius Academy's Data Science & AI Course:
If you're leaning towards data science and want to delve into machine learning and AI, Xaltius Academy's Data Science & AI course is an excellent choice. This program equips you with the necessary skills and knowledge to become a proficient data scientist, covering essential topics like:
Python programming
Machine learning algorithms
Data visualization
And much more!
By understanding the distinct roles of business analytics and data science, you can make an informed decision about your career path and leverage the power of data to drive success.
2 notes
·
View notes
Text
Why Tableau is Essential in Data Science: Transforming Raw Data into Insights
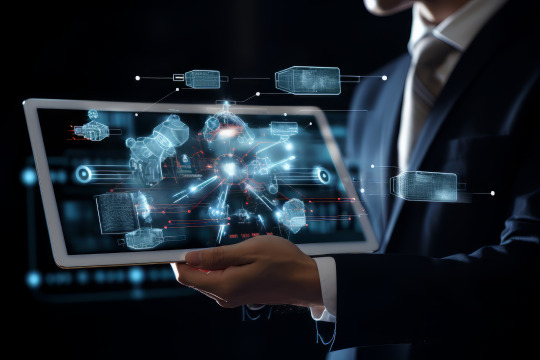
Data science is all about turning raw data into valuable insights. But numbers and statistics alone don’t tell the full story—they need to be visualized to make sense. That’s where Tableau comes in.
Tableau is a powerful tool that helps data scientists, analysts, and businesses see and understand data better. It simplifies complex datasets, making them interactive and easy to interpret. But with so many tools available, why is Tableau a must-have for data science? Let’s explore.
1. The Importance of Data Visualization in Data Science
Imagine you’re working with millions of data points from customer purchases, social media interactions, or financial transactions. Analyzing raw numbers manually would be overwhelming.
That’s why visualization is crucial in data science:
Identifies trends and patterns – Instead of sifting through spreadsheets, you can quickly spot trends in a visual format.
Makes complex data understandable – Graphs, heatmaps, and dashboards simplify the interpretation of large datasets.
Enhances decision-making – Stakeholders can easily grasp insights and make data-driven decisions faster.
Saves time and effort – Instead of writing lengthy reports, an interactive dashboard tells the story in seconds.
Without tools like Tableau, data science would be limited to experts who can code and run statistical models. With Tableau, insights become accessible to everyone—from data scientists to business executives.
2. Why Tableau Stands Out in Data Science
A. User-Friendly and Requires No Coding
One of the biggest advantages of Tableau is its drag-and-drop interface. Unlike Python or R, which require programming skills, Tableau allows users to create visualizations without writing a single line of code.
Even if you’re a beginner, you can:
✅ Upload data from multiple sources
✅ Create interactive dashboards in minutes
✅ Share insights with teams easily
This no-code approach makes Tableau ideal for both technical and non-technical professionals in data science.
B. Handles Large Datasets Efficiently
Data scientists often work with massive datasets—whether it’s financial transactions, customer behavior, or healthcare records. Traditional tools like Excel struggle with large volumes of data.
Tableau, on the other hand:
Can process millions of rows without slowing down
Optimizes performance using advanced data engine technology
Supports real-time data streaming for up-to-date analysis
This makes it a go-to tool for businesses that need fast, data-driven insights.
C. Connects with Multiple Data Sources
A major challenge in data science is bringing together data from different platforms. Tableau seamlessly integrates with a variety of sources, including:
Databases: MySQL, PostgreSQL, Microsoft SQL Server
Cloud platforms: AWS, Google BigQuery, Snowflake
Spreadsheets and APIs: Excel, Google Sheets, web-based data sources
This flexibility allows data scientists to combine datasets from multiple sources without needing complex SQL queries or scripts.
D. Real-Time Data Analysis
Industries like finance, healthcare, and e-commerce rely on real-time data to make quick decisions. Tableau’s live data connection allows users to:
Track stock market trends as they happen
Monitor website traffic and customer interactions in real time
Detect fraudulent transactions instantly
Instead of waiting for reports to be generated manually, Tableau delivers insights as events unfold.
E. Advanced Analytics Without Complexity
While Tableau is known for its visualizations, it also supports advanced analytics. You can:
Forecast trends based on historical data
Perform clustering and segmentation to identify patterns
Integrate with Python and R for machine learning and predictive modeling
This means data scientists can combine deep analytics with intuitive visualization, making Tableau a versatile tool.
3. How Tableau Helps Data Scientists in Real Life
Tableau has been adopted by the majority of industries to make data science more impactful and accessible. This is applied in the following real-life scenarios:
A. Analytics for Health Care
Tableau is deployed by hospitals and research institutions for the following purposes:
Monitor patient recovery rates and predict outbreaks of diseases
Analyze hospital occupancy and resource allocation
Identify trends in patient demographics and treatment results
B. Finance and Banking
Banks and investment firms rely on Tableau for the following purposes:
✅ Detect fraud by analyzing transaction patterns
✅ Track stock market fluctuations and make informed investment decisions
✅ Assess credit risk and loan performance
C. Marketing and Customer Insights
Companies use Tableau to:
✅ Track customer buying behavior and personalize recommendations
✅ Analyze social media engagement and campaign effectiveness
✅ Optimize ad spend by identifying high-performing channels
D. Retail and Supply Chain Management
Retailers leverage Tableau to:
✅ Forecast product demand and adjust inventory levels
✅ Identify regional sales trends and adjust marketing strategies
✅ Optimize supply chain logistics and reduce delivery delays
These applications show why Tableau is a must-have for data-driven decision-making.
4. Tableau vs. Other Data Visualization Tools
There are many visualization tools available, but Tableau consistently ranks as one of the best. Here’s why:
Tableau vs. Excel – Excel struggles with big data and lacks interactivity; Tableau handles large datasets effortlessly.
Tableau vs. Power BI – Power BI is great for Microsoft users, but Tableau offers more flexibility across different data sources.
Tableau vs. Python (Matplotlib, Seaborn) – Python libraries require coding skills, while Tableau simplifies visualization for all users.
This makes Tableau the go-to tool for both beginners and experienced professionals in data science.
5. Conclusion
Tableau has become an essential tool in data science because it simplifies data visualization, handles large datasets, and integrates seamlessly with various data sources. It enables professionals to analyze, interpret, and present data interactively, making insights accessible to everyone—from data scientists to business leaders.
If you’re looking to build a strong foundation in data science, learning Tableau is a smart career move. Many data science courses now include Tableau as a key skill, as companies increasingly demand professionals who can transform raw data into meaningful insights.
In a world where data is the driving force behind decision-making, Tableau ensures that the insights you uncover are not just accurate—but also clear, impactful, and easy to act upon.
#data science course#top data science course online#top data science institute online#artificial intelligence course#deepseek#tableau
3 notes
·
View notes
Text
What Are the Qualifications for a Data Scientist?
In today's data-driven world, the role of a data scientist has become one of the most coveted career paths. With businesses relying on data for decision-making, understanding customer behavior, and improving products, the demand for skilled professionals who can analyze, interpret, and extract value from data is at an all-time high. If you're wondering what qualifications are needed to become a successful data scientist, how DataCouncil can help you get there, and why a data science course in Pune is a great option, this blog has the answers.
The Key Qualifications for a Data Scientist
To succeed as a data scientist, a mix of technical skills, education, and hands-on experience is essential. Here are the core qualifications required:
1. Educational Background
A strong foundation in mathematics, statistics, or computer science is typically expected. Most data scientists hold at least a bachelor’s degree in one of these fields, with many pursuing higher education such as a master's or a Ph.D. A data science course in Pune with DataCouncil can bridge this gap, offering the academic and practical knowledge required for a strong start in the industry.
2. Proficiency in Programming Languages
Programming is at the heart of data science. You need to be comfortable with languages like Python, R, and SQL, which are widely used for data analysis, machine learning, and database management. A comprehensive data science course in Pune will teach these programming skills from scratch, ensuring you become proficient in coding for data science tasks.
3. Understanding of Machine Learning
Data scientists must have a solid grasp of machine learning techniques and algorithms such as regression, clustering, and decision trees. By enrolling in a DataCouncil course, you'll learn how to implement machine learning models to analyze data and make predictions, an essential qualification for landing a data science job.
4. Data Wrangling Skills
Raw data is often messy and unstructured, and a good data scientist needs to be adept at cleaning and processing data before it can be analyzed. DataCouncil's data science course in Pune includes practical training in tools like Pandas and Numpy for effective data wrangling, helping you develop a strong skill set in this critical area.
5. Statistical Knowledge
Statistical analysis forms the backbone of data science. Knowledge of probability, hypothesis testing, and statistical modeling allows data scientists to draw meaningful insights from data. A structured data science course in Pune offers the theoretical and practical aspects of statistics required to excel.
6. Communication and Data Visualization Skills
Being able to explain your findings in a clear and concise manner is crucial. Data scientists often need to communicate with non-technical stakeholders, making tools like Tableau, Power BI, and Matplotlib essential for creating insightful visualizations. DataCouncil’s data science course in Pune includes modules on data visualization, which can help you present data in a way that’s easy to understand.
7. Domain Knowledge
Apart from technical skills, understanding the industry you work in is a major asset. Whether it’s healthcare, finance, or e-commerce, knowing how data applies within your industry will set you apart from the competition. DataCouncil's data science course in Pune is designed to offer case studies from multiple industries, helping students gain domain-specific insights.
Why Choose DataCouncil for a Data Science Course in Pune?
If you're looking to build a successful career as a data scientist, enrolling in a data science course in Pune with DataCouncil can be your first step toward reaching your goals. Here’s why DataCouncil is the ideal choice:
Comprehensive Curriculum: The course covers everything from the basics of data science to advanced machine learning techniques.
Hands-On Projects: You'll work on real-world projects that mimic the challenges faced by data scientists in various industries.
Experienced Faculty: Learn from industry professionals who have years of experience in data science and analytics.
100% Placement Support: DataCouncil provides job assistance to help you land a data science job in Pune or anywhere else, making it a great investment in your future.
Flexible Learning Options: With both weekday and weekend batches, DataCouncil ensures that you can learn at your own pace without compromising your current commitments.
Conclusion
Becoming a data scientist requires a combination of technical expertise, analytical skills, and industry knowledge. By enrolling in a data science course in Pune with DataCouncil, you can gain all the qualifications you need to thrive in this exciting field. Whether you're a fresher looking to start your career or a professional wanting to upskill, this course will equip you with the knowledge, skills, and practical experience to succeed as a data scientist.
Explore DataCouncil’s offerings today and take the first step toward unlocking a rewarding career in data science! Looking for the best data science course in Pune? DataCouncil offers comprehensive data science classes in Pune, designed to equip you with the skills to excel in this booming field. Our data science course in Pune covers everything from data analysis to machine learning, with competitive data science course fees in Pune. We provide job-oriented programs, making us the best institute for data science in Pune with placement support. Explore online data science training in Pune and take your career to new heights!
#In today's data-driven world#the role of a data scientist has become one of the most coveted career paths. With businesses relying on data for decision-making#understanding customer behavior#and improving products#the demand for skilled professionals who can analyze#interpret#and extract value from data is at an all-time high. If you're wondering what qualifications are needed to become a successful data scientis#how DataCouncil can help you get there#and why a data science course in Pune is a great option#this blog has the answers.#The Key Qualifications for a Data Scientist#To succeed as a data scientist#a mix of technical skills#education#and hands-on experience is essential. Here are the core qualifications required:#1. Educational Background#A strong foundation in mathematics#statistics#or computer science is typically expected. Most data scientists hold at least a bachelor’s degree in one of these fields#with many pursuing higher education such as a master's or a Ph.D. A data science course in Pune with DataCouncil can bridge this gap#offering the academic and practical knowledge required for a strong start in the industry.#2. Proficiency in Programming Languages#Programming is at the heart of data science. You need to be comfortable with languages like Python#R#and SQL#which are widely used for data analysis#machine learning#and database management. A comprehensive data science course in Pune will teach these programming skills from scratch#ensuring you become proficient in coding for data science tasks.#3. Understanding of Machine Learning
3 notes
·
View notes
Text
The Skills I Acquired on My Path to Becoming a Data Scientist
Data science has emerged as one of the most sought-after fields in recent years, and my journey into this exciting discipline has been nothing short of transformative. As someone with a deep curiosity for extracting insights from data, I was naturally drawn to the world of data science. In this blog post, I will share the skills I acquired on my path to becoming a data scientist, highlighting the importance of a diverse skill set in this field.
The Foundation — Mathematics and Statistics
At the core of data science lies a strong foundation in mathematics and statistics. Concepts such as probability, linear algebra, and statistical inference form the building blocks of data analysis and modeling. Understanding these principles is crucial for making informed decisions and drawing meaningful conclusions from data. Throughout my learning journey, I immersed myself in these mathematical concepts, applying them to real-world problems and honing my analytical skills.
Programming Proficiency
Proficiency in programming languages like Python or R is indispensable for a data scientist. These languages provide the tools and frameworks necessary for data manipulation, analysis, and modeling. I embarked on a journey to learn these languages, starting with the basics and gradually advancing to more complex concepts. Writing efficient and elegant code became second nature to me, enabling me to tackle large datasets and build sophisticated models.
Data Handling and Preprocessing
Working with real-world data is often messy and requires careful handling and preprocessing. This involves techniques such as data cleaning, transformation, and feature engineering. I gained valuable experience in navigating the intricacies of data preprocessing, learning how to deal with missing values, outliers, and inconsistent data formats. These skills allowed me to extract valuable insights from raw data and lay the groundwork for subsequent analysis.
Data Visualization and Communication
Data visualization plays a pivotal role in conveying insights to stakeholders and decision-makers. I realized the power of effective visualizations in telling compelling stories and making complex information accessible. I explored various tools and libraries, such as Matplotlib and Tableau, to create visually appealing and informative visualizations. Sharing these visualizations with others enhanced my ability to communicate data-driven insights effectively.
Machine Learning and Predictive Modeling
Machine learning is a cornerstone of data science, enabling us to build predictive models and make data-driven predictions. I delved into the realm of supervised and unsupervised learning, exploring algorithms such as linear regression, decision trees, and clustering techniques. Through hands-on projects, I gained practical experience in building models, fine-tuning their parameters, and evaluating their performance.
Database Management and SQL
Data science often involves working with large datasets stored in databases. Understanding database management and SQL (Structured Query Language) is essential for extracting valuable information from these repositories. I embarked on a journey to learn SQL, mastering the art of querying databases, joining tables, and aggregating data. These skills allowed me to harness the power of databases and efficiently retrieve the data required for analysis.
Domain Knowledge and Specialization
While technical skills are crucial, domain knowledge adds a unique dimension to data science projects. By specializing in specific industries or domains, data scientists can better understand the context and nuances of the problems they are solving. I explored various domains and acquired specialized knowledge, whether it be healthcare, finance, or marketing. This expertise complemented my technical skills, enabling me to provide insights that were not only data-driven but also tailored to the specific industry.
Soft Skills — Communication and Problem-Solving
In addition to technical skills, soft skills play a vital role in the success of a data scientist. Effective communication allows us to articulate complex ideas and findings to non-technical stakeholders, bridging the gap between data science and business. Problem-solving skills help us navigate challenges and find innovative solutions in a rapidly evolving field. Throughout my journey, I honed these skills, collaborating with teams, presenting findings, and adapting my approach to different audiences.
Continuous Learning and Adaptation
Data science is a field that is constantly evolving, with new tools, technologies, and trends emerging regularly. To stay at the forefront of this ever-changing landscape, continuous learning is essential. I dedicated myself to staying updated by following industry blogs, attending conferences, and participating in courses. This commitment to lifelong learning allowed me to adapt to new challenges, acquire new skills, and remain competitive in the field.
In conclusion, the journey to becoming a data scientist is an exciting and dynamic one, requiring a diverse set of skills. From mathematics and programming to data handling and communication, each skill plays a crucial role in unlocking the potential of data. Aspiring data scientists should embrace this multidimensional nature of the field and embark on their own learning journey. If you want to learn more about Data science, I highly recommend that you contact ACTE Technologies because they offer Data Science courses and job placement opportunities. Experienced teachers can help you learn better. You can find these services both online and offline. Take things step by step and consider enrolling in a course if you’re interested. By acquiring these skills and continuously adapting to new developments, they can make a meaningful impact in the world of data science.
#data science#data visualization#education#information#technology#machine learning#database#sql#predictive analytics#r programming#python#big data#statistics
14 notes
·
View notes
Text
From Zero to Hero: Grow Your Data Science Skills
Understanding the Foundations of Data Science
We produce around 2.5 quintillion bytes of data worldwide, which is enough to fill 10 million DVDs! That huge amount of data is more like a goldmine for data scientists, they use different tools and complex algorithms to find valuable insights.
Here's the deal: data science is all about finding valuable insights from the raw data. It's more like playing a jigsaw puzzle with a thousand parts and figuring out how they all go together. Begin with the basics, Learn how to gather, clean, analyze, and present data in a straightforward and easy-to-understand way.
Here Are The Skill Needed For A Data Scientists
Okay, let’s talk about the skills you’ll need to be a pro in data science. First up: programming. Python is your new best friend, it is powerful and surprisingly easy to learn. By using the libraries like Pandas and NumPy, you can manage the data like a pro.
Statistics is another tool you must have a good knowledge of, as a toolkit that will help you make sense of all the numbers and patterns you deal with. Next is machine learning, and here you train the data model by using a huge amount of data and make predictions out of it.
Once you analyze and have insights from the data, and next is to share this valuable information with others by creating simple and interactive data visualizations by using charts and graphs.
The Programming Language Every Data Scientist Must Know
Python is the language every data scientist must know, but there are some other languages also that are worth your time. R is another language known for its statistical solid power if you are going to deal with more numbers and data, then R might be the best tool for you.
SQL is one of the essential tools, it is the language that is used for managing the database, and if you know how to query the database effectively, then it will make your data capturing and processing very easy.
Exploring Data Science Tools and Technologies
Alright, so you’ve got your programming languages down. Now, let’s talk about tools. Jupyter Notebooks are fantastic for writing and sharing your code. They let you combine code, visualizations, and explanations in one place, making it easier to document your work and collaborate with others.
To create a meaningful dashboard Tableau is the tool most commonly used by data scientists. It is a tool that can create interactive dashboards and visualizations that will help you share valuable insights with people who do not have an excellent technical background.
Building a Strong Mathematical Foundation
Math might not be everyone’s favorite subject, but it’s a crucial part of data science. You’ll need a good grasp of statistics for analyzing data and drawing conclusions. Linear algebra is important for understanding how the algorithms work, specifically in machine learning. Calculus helps optimize algorithms, while probability theory lets you handle uncertainty in your data. You need to create a mathematical model that helps you represent and analyze real-world problems. So it is essential to sharpen your mathematical skills which will give you a solid upper hand in dealing with complex data science challenges.
Do Not Forget the Data Cleaning and Processing Skills
Before you can dive into analysis, you need to clean the data and preprocess the data. This step can feel like a bit of a grind, but it’s essential. You’ll deal with missing data and decide whether to fill in the gaps or remove them. Data transformation normalizing and standardizing the data to maintain consistency in the data sets. Feature engineering is all about creating a new feature from the existing data to improve the models. Knowing this data processing technique will help you perform a successful analysis and gain better insights.
Diving into Machine Learning and AI
Machine learning and AI are where the magic happens. Supervised learning involves training models using labeled data to predict the outcomes. On the other hand, unsupervised learning assists in identifying patterns in data without using predetermined labels. Deep learning comes into play when dealing with complicated patterns and producing correct predictions, which employs neural networks. Learn how to use AI in data science to do tasks more efficiently.
How Data Science Helps To Solve The Real-world Problems
Knowing the theory is great, but applying what you’ve learned to real-world problems is where you see the impact. Participate in data science projects to gain practical exposure and create a good portfolio. Look into case studies to see how others have tackled similar issues. Explore how data science is used in various industries from healthcare to finance—and apply your skills to solve real-world challenges.
Always Follow Data Science Ethics and Privacy
Handling data responsibly is a big part of being a data scientist. Understanding the ethical practices and privacy concerns associated with your work is crucial. Data privacy regulations, such as GDPR, set guidelines for collecting and using data. Responsible AI practices ensure that your models are fair and unbiased. Being transparent about your methods and accountable for your results helps build trust and credibility. These ethical standards will help you maintain integrity in your data science practice.
Building Your Data Science Portfolio and Career
Let’s talk about careers. Building a solid portfolio is important for showcasing your skills and projects. Include a variety of projects that showcase your skills to tackle real-world problems. The data science job market is competitive, so make sure your portfolio is unique. Earning certifications can also boost your profile and show your dedication in this field. Networking with other data professionals through events, forums, and social media can be incredibly valuable. When you are facing job interviews, preparation is critical. Practice commonly asked questions to showcase your expertise effectively.
To Sum-up
Now you have a helpful guideline to begin your journey in data science. Always keep yourself updated in this field to stand out if you are just starting or want to improve. Check this blog to find the best data science course in Kolkata. You are good to go on this excellent career if you build a solid foundation to improve your skills and apply what you have learned in real life.
2 notes
·
View notes
Text
Essential Skills for Aspiring Data Scientists in 2024
Welcome to another edition of Tech Insights! Today, we're diving into the essential skills that aspiring data scientists need to master in 2024. As the field of data science continues to evolve, staying updated with the latest skills and tools is crucial for success. Here are the key areas to focus on:
1. Programming Proficiency
Proficiency in programming languages like Python and R is foundational. Python, in particular, is widely used for data manipulation, analysis, and building machine learning models thanks to its rich ecosystem of libraries such as Pandas, NumPy, and Scikit-learn.
2. Statistical Analysis
A strong understanding of statistics is essential for data analysis and interpretation. Key concepts include probability distributions, hypothesis testing, and regression analysis, which help in making informed decisions based on data.
3. Machine Learning Mastery
Knowledge of machine learning algorithms and frameworks like TensorFlow, Keras, and PyTorch is critical. Understanding supervised and unsupervised learning, neural networks, and deep learning will set you apart in the field.
4. Data Wrangling Skills
The ability to clean, process, and transform data is crucial. Skills in using libraries like Pandas and tools like SQL for database management are highly valuable for preparing data for analysis.
5. Data Visualization
Effective communication of your findings through data visualization is important. Tools like Tableau, Power BI, and libraries like Matplotlib and Seaborn in Python can help you create impactful visualizations.
6. Big Data Technologies
Familiarity with big data tools like Hadoop, Spark, and NoSQL databases is beneficial, especially for handling large datasets. These tools help in processing and analyzing big data efficiently.
7. Domain Knowledge
Understanding the specific domain you are working in (e.g., finance, healthcare, e-commerce) can significantly enhance your analytical insights and make your solutions more relevant and impactful.
8. Soft Skills
Strong communication skills, problem-solving abilities, and teamwork are essential for collaborating with stakeholders and effectively conveying your findings.
Final Thoughts
The field of data science is ever-changing, and staying ahead requires continuous learning and adaptation. By focusing on these key skills, you'll be well-equipped to navigate the challenges and opportunities that 2024 brings.
If you're looking for more in-depth resources, tips, and articles on data science and machine learning, be sure to follow Tech Insights for regular updates. Let's continue to explore the fascinating world of technology together!
#artificial intelligence#programming#coding#python#success#economy#career#education#employment#opportunity#working#jobs
2 notes
·
View notes
Text
How to write a paper with ai
mbrace the Future of Research: The Advantages of Using AI Websites for Writing Academic Papers
The landscape of academic writing is evolving with the incorporation of artificial intelligence (AI). AI-powered websites have become a valuable asset in the arsenal of students, researchers, and academics. I highlight the benefits of using AI websites for writing papers and provide recommendations for those looking to optimize their writing process.
Why Use AI Websites for Academic Writing?
1. Efficiency in Research: Tools like Google Scholar and arXiv provide AI-enhanced search functionalities enabling you to quickly find relevant and credible academic sources, thereby accelerating the research process.
2. Streamlined Writing Process: AI writing assistants, such as Jasper AI, offer to help you compose text based on provided prompts or outlines. They can assist in creating drafts more rapidly than traditional methods.
3. Enhanced Organization: Note-taking and outlining AI tools like Evernote or Notion AI can categorize your research, create sophisticated outlines, and keep all your ideas and references neatly organized.
4. High-Quality Drafts: AI websites such as WriteGo.ai generate comprehensive essay drafts, including complex financial analysis and data interpretation, which can significantly improve the initial quality of your paper.
5. Advanced Editing Assistance: Editing platforms like Grammarly use AI to detect grammatical errors, suggest style improvements, and ensure your paper reads naturally and adheres to professional writing standards.
6. Plagiarism Detection: AI-based tools like Turnitin and Copyscape scan your document against a vast database to check for originality and prevent any instances of plagiarism.
7. Data Analysis and Visualization: AI-driven data tools like Tableau can sift through and visualize large datasets, which is particularly beneficial for data-intensive disciplines like finance and sciences.
Recommendations for AI Websites:
Here are some of the top AI websites I recommend for writing academic papers:
Jasper AI for generating written content.
Evernote or Notion AI for organizing your research and notes.
Grammarly or ProWritingAid for editing and refining drafts.
Google Scholar for conducting an AI-enhanced literature search.
Turnitin for plagiarism checks.
Conclusion:
Using AI to assist in writing academic papers is an innovative approach that combines cutting-edge technology with scholarly rigor. The fusion of AI with your own analytical skills can vastly improve the quality of your work, making the process more efficient and leading to higher caliber research outputs.
Whether you are writing a comprehensive review, an empirical paper, or a thesis, AI websites have the potential to complement your intellect and to push the boundaries of what you can achieve in the academic realm. As we move further into the digital era, embracing these tools can help maintain a competitive edge and ensure your academic writing is as impactful and effective as possible.
writego
2 notes
·
View notes
Text

Day 3/100 days of productivity | Wed 21 Feb, 2024
Long day at work, chatted with colleagues about career paths in data science, met with my boss for a performance evaluation (it went well!). Worked on writing some Quarto documentation for how to build one of my Tableau dashboards, since it keeps breaking and I keep referring to my notes 😳
In non-work productivity, I completed the ‘Logistic Regression as a Neural Network’ module of Andrew Ng’s Deep Learning course on Coursera and refreshed my understanding of backpropagation using the calculus chain rule. Fun!
#100 days of productivity#data science#deep learning#neural network#quarto#tableau#note taking#chain rule
3 notes
·
View notes
Text
Cracking the Code: A Beginner's Roadmap to Mastering Data Science
Embarking on the journey into data science as a complete novice is an exciting venture. While the world of data science may seem daunting at first, breaking down the learning process into manageable steps can make the endeavor both enjoyable and rewarding. Choosing the best Data Science Institute can further accelerate your journey into this thriving industry.
In this comprehensive guide, we'll outline a roadmap for beginners to get started with data science, from understanding the basics to building a portfolio of projects.
1. Understanding the Basics: Laying the Foundation
The journey begins with a solid understanding of the fundamentals of data science. Start by familiarizing yourself with key concepts such as data types, variables, and basic statistics. Platforms like Khan Academy, Coursera, and edX offer introductory courses in statistics and data science, providing a solid foundation for your learning journey.
2. Learn Programming Languages: The Language of Data Science
Programming is a crucial skill in data science, and Python is one of the most widely used languages in the field. Platforms like Codecademy, DataCamp, and freeCodeCamp offer interactive lessons and projects to help beginners get hands-on experience with Python. Additionally, learning R, another popular language in data science, can broaden your skill set.
3. Explore Data Visualization: Bringing Data to Life
Data visualization is a powerful tool for understanding and communicating data. Explore tools like Tableau for creating interactive visualizations or dive into Python libraries like Matplotlib and Seaborn. Understanding how to present data visually enhances your ability to derive insights and convey information effectively.
4. Master Data Manipulation: Unlocking Data's Potential
Data manipulation is a fundamental aspect of data science. Learn how to manipulate and analyze data using libraries like Pandas in Python. The official Pandas website provides tutorials and documentation to guide you through the basics of data manipulation, a skill that is essential for any data scientist.
5. Delve into Machine Learning Basics: The Heart of Data Science
Machine learning is a core component of data science. Start exploring the fundamentals of machine learning on platforms like Kaggle, which offers beginner-friendly datasets and competitions. Participating in Kaggle competitions allows you to apply your knowledge, learn from others, and gain practical experience in machine learning.
6. Take Online Courses: Structured Learning Paths
Enroll in online courses that provide structured learning paths in data science. Platforms like Coursera (e.g., "Data Science and Machine Learning Bootcamp with R" or "Applied Data Science with Python") and edX (e.g., "Harvard's Data Science Professional Certificate") offer comprehensive courses taught by experts in the field.
7. Read Books and Blogs: Supplementing Your Knowledge
Books and blogs can provide additional insights and practical tips. "Python for Data Analysis" by Wes McKinney is a highly recommended book, and blogs like Towards Data Science on Medium offer a wealth of articles covering various data science topics. These resources can deepen your understanding and offer different perspectives on the subject.
8. Join Online Communities: Learning Through Connection
Engage with the data science community by joining online platforms like Stack Overflow, Reddit (e.g., r/datascience), and LinkedIn. Participate in discussions, ask questions, and learn from the experiences of others. Being part of a community provides valuable support and insights.
9. Work on Real Projects: Applying Your Skills
Apply your skills by working on real-world projects. Identify a problem or area of interest, find a dataset, and start working on analysis and predictions. Whether it's predicting housing prices, analyzing social media sentiment, or exploring healthcare data, hands-on projects are crucial for developing practical skills.
10. Attend Webinars and Conferences: Staying Updated
Stay updated on the latest trends and advancements in data science by attending webinars and conferences. Platforms like Data Science Central and conferences like the Data Science Conference provide opportunities to learn from experts, discover new technologies, and connect with the wider data science community.
11. Build a Portfolio: Showcasing Your Journey
Create a portfolio showcasing your projects and skills. This can be a GitHub repository or a personal website where you document and present your work. A portfolio is a powerful tool for demonstrating your capabilities to potential employers and collaborators.
12. Practice Regularly: The Path to Mastery
Consistent practice is key to mastering data science. Dedicate regular time to coding, explore new datasets, and challenge yourself with increasingly complex projects. As you progress, you'll find that your skills evolve, and you become more confident in tackling advanced data science challenges.
Embarking on the path of data science as a beginner may seem like a formidable task, but with the right resources and a structured approach, it becomes an exciting and achievable endeavor. From understanding the basics to building a portfolio of real-world projects, each step contributes to your growth as a data scientist. Embrace the learning process, stay curious, and celebrate the milestones along the way. The world of data science is vast and dynamic, and your journey is just beginning. Choosing the best Data Science courses in Chennai is a crucial step in acquiring the necessary expertise for a successful career in the evolving landscape of data science.
3 notes
·
View notes
Text
What Does It Take to Be a Successful Data Scientist in India?
So, you want to dive into the exciting world of Data Science in India? Well, grab your thinking cap and get ready for a rollercoaster ride through the data jungle! Here's the lowdown on what it takes to succeed:
1. Get Your Math Game On: First things first, you need to be buddies with math and stats. It's like the secret handshake of the data club.
2. Learning is a Lifestyle: Data Science is like that ever-changing friend who always has something new up their sleeve. So, be prepared to be a lifelong learner. Online courses are your BFFs.
3. Code Like a Pro: Learn Python and R – they're like the cool tools in your data toolbox. You'll use them to cook up amazing data dishes.
4. Sherlock Holmes Mode: Develop a superpower for problem-solving. You'll be the Sherlock Holmes of data mysteries.
5. Dive into a Domain: Choose a domain – like finance, healthcare, or e-commerce – that tickles your fancy. It's like picking your favorite ice cream flavor in the world of data.
6. Picasso of Data: Learn to paint pretty pictures with data. Tools like Tableau or Power BI will help you create data masterpieces that everyone can understand.
7. Pet Projects: Get your hands dirty with personal data projects. It's like gardening but with data. Showcase your green thumb in a portfolio.
8. Make Data Friends: Network with data nerds. Attend meetups, conferences, or just chat with fellow enthusiasts. You might find your data soulmate.
9. Mu Sigma Magic: Mu Sigma is like Willy Wonka's Chocolate Factory of Decision Science. They offer golden tickets to data lovers. Check out job openings at Mu Sigma and see if it's your golden ticket.
10. Soft Skills: Your charm matters too! You need to explain data stuff to folks who don't speak binary. Communication and teamwork are your secret weapons.
11. Be a Chameleon: The data world changes faster than a chameleon's colors. Be ready to adapt and embrace new challenges and tech like a pro.
In India, the data science scene is sizzling hot! There are plenty of juicy job opportunities, whether you're fresh out of college or a seasoned pro. So, gear up because it's time to rock the data world and make some sense out of the chaos, one byte at a time!
2 notes
·
View notes
Text
Data Engineering Concepts, Tools, and Projects
All the associations in the world have large amounts of data. If not worked upon and anatomized, this data does not amount to anything. Data masterminds are the ones. who make this data pure for consideration. Data Engineering can nominate the process of developing, operating, and maintaining software systems that collect, dissect, and store the association’s data. In modern data analytics, data masterminds produce data channels, which are the structure armature.
How to become a data engineer:
While there is no specific degree requirement for data engineering, a bachelor's or master's degree in computer science, software engineering, information systems, or a related field can provide a solid foundation. Courses in databases, programming, data structures, algorithms, and statistics are particularly beneficial. Data engineers should have strong programming skills. Focus on languages commonly used in data engineering, such as Python, SQL, and Scala. Learn the basics of data manipulation, scripting, and querying databases.
Familiarize yourself with various database systems like MySQL, PostgreSQL, and NoSQL databases such as MongoDB or Apache Cassandra.Knowledge of data warehousing concepts, including schema design, indexing, and optimization techniques.
Data engineering tools recommendations:
Data Engineering makes sure to use a variety of languages and tools to negotiate its objects. These tools allow data masterminds to apply tasks like creating channels and algorithms in a much easier as well as effective manner.
1. Amazon Redshift: A widely used cloud data warehouse built by Amazon, Redshift is the go-to choice for many teams and businesses. It is a comprehensive tool that enables the setup and scaling of data warehouses, making it incredibly easy to use.
One of the most popular tools used for businesses purpose is Amazon Redshift, which provides a powerful platform for managing large amounts of data. It allows users to quickly analyze complex datasets, build models that can be used for predictive analytics, and create visualizations that make it easier to interpret results. With its scalability and flexibility, Amazon Redshift has become one of the go-to solutions when it comes to data engineering tasks.
2. Big Query: Just like Redshift, Big Query is a cloud data warehouse fully managed by Google. It's especially favored by companies that have experience with the Google Cloud Platform. BigQuery not only can scale but also has robust machine learning features that make data analysis much easier. 3. Tableau: A powerful BI tool, Tableau is the second most popular one from our survey. It helps extract and gather data stored in multiple locations and comes with an intuitive drag-and-drop interface. Tableau makes data across departments readily available for data engineers and managers to create useful dashboards. 4. Looker: An essential BI software, Looker helps visualize data more effectively. Unlike traditional BI tools, Looker has developed a LookML layer, which is a language for explaining data, aggregates, calculations, and relationships in a SQL database. A spectacle is a newly-released tool that assists in deploying the LookML layer, ensuring non-technical personnel have a much simpler time when utilizing company data.
5. Apache Spark: An open-source unified analytics engine, Apache Spark is excellent for processing large data sets. It also offers great distribution and runs easily alongside other distributed computing programs, making it essential for data mining and machine learning. 6. Airflow: With Airflow, programming, and scheduling can be done quickly and accurately, and users can keep an eye on it through the built-in UI. It is the most used workflow solution, as 25% of data teams reported using it. 7. Apache Hive: Another data warehouse project on Apache Hadoop, Hive simplifies data queries and analysis with its SQL-like interface. This language enables MapReduce tasks to be executed on Hadoop and is mainly used for data summarization, analysis, and query. 8. Segment: An efficient and comprehensive tool, Segment assists in collecting and using data from digital properties. It transforms, sends, and archives customer data, and also makes the entire process much more manageable. 9. Snowflake: This cloud data warehouse has become very popular lately due to its capabilities in storing and computing data. Snowflake’s unique shared data architecture allows for a wide range of applications, making it an ideal choice for large-scale data storage, data engineering, and data science. 10. DBT: A command-line tool that uses SQL to transform data, DBT is the perfect choice for data engineers and analysts. DBT streamlines the entire transformation process and is highly praised by many data engineers.
Data Engineering Projects:
Data engineering is an important process for businesses to understand and utilize to gain insights from their data. It involves designing, constructing, maintaining, and troubleshooting databases to ensure they are running optimally. There are many tools available for data engineers to use in their work such as My SQL, SQL server, oracle RDBMS, Open Refine, TRIFACTA, Data Ladder, Keras, Watson, TensorFlow, etc. Each tool has its strengths and weaknesses so it’s important to research each one thoroughly before making recommendations about which ones should be used for specific tasks or projects.
Smart IoT Infrastructure:
As the IoT continues to develop, the measure of data consumed with high haste is growing at an intimidating rate. It creates challenges for companies regarding storehouses, analysis, and visualization.
Data Ingestion:
Data ingestion is moving data from one or further sources to a target point for further preparation and analysis. This target point is generally a data storehouse, a unique database designed for effective reporting.
Data Quality and Testing:
Understand the importance of data quality and testing in data engineering projects. Learn about techniques and tools to ensure data accuracy and consistency.
Streaming Data:
Familiarize yourself with real-time data processing and streaming frameworks like Apache Kafka and Apache Flink. Develop your problem-solving skills through practical exercises and challenges.
Conclusion:
Data engineers are using these tools for building data systems. My SQL, SQL server and Oracle RDBMS involve collecting, storing, managing, transforming, and analyzing large amounts of data to gain insights. Data engineers are responsible for designing efficient solutions that can handle high volumes of data while ensuring accuracy and reliability. They use a variety of technologies including databases, programming languages, machine learning algorithms, and more to create powerful applications that help businesses make better decisions based on their collected data.
2 notes
·
View notes
Text
Master Data Analytics with DICS: The Leading Institute in Laxmi Nagar

In today’s digital age, data is the new oil—and the ability to analyze it is one of the most in-demand skills across industries. If you're searching for the best data analytics institute in Laxmi Nagar, your journey ends here. Our institute stands out as a leader in data analytics training, offering students not only world-class education but also the tools, confidence, and career support needed to thrive in the data-driven world.
Our carefully curated curriculum is designed with input from industry professionals to ensure it meets the evolving demands of employers. Whether you’re a recent graduate, working professional, or someone looking to switch careers, our programs are flexible and practical, equipping you with the latest techniques and technologies used by top data analysts today.
Why We Are the Best Data Analytics Institute in Laxmi Nagar
What sets us apart is our student-first approach. From day one, you’re not just attending classes—you’re stepping into a career-focused journey. Our faculty consists of experienced professionals with years of real-world experience in data analytics, business intelligence, and data science. Each module is taught with practical application in mind, reinforced by hands-on projects, case studies, and regular assessments.
We provide in-depth training in tools such as Excel, SQL, Python, Tableau, Power BI, R, and advanced analytics techniques including data visualization, predictive modeling, and machine learning. Students graduate with not only theoretical knowledge but also the confidence to apply it to real business problems.
Enroll in the Best Data Analytics Course in Laxmi Nagar
Choosing the best data analytics course in Laxmi Nagar is a critical step for anyone looking to build a strong foundation in analytics. Our course structure balances core concepts with practical implementation. It’s not just about learning tools—it's about solving problems, understanding data-driven decision making, and becoming a professional who can turn raw data into actionable insights.
Whether you’re interested in business analytics, marketing analytics, or big data technologies, our comprehensive course is designed to cover every essential area. You’ll work on real-time projects and receive one-on-one mentorship, allowing you to build a portfolio that impresses employers.
100% Placement Assistance and Career Support
We don’t just train—you’re supported every step of the way, from resume building and mock interviews to connecting you with recruiters from top companies. Many of our alumni now work at leading organizations, a testament to the quality of training and support we provide.
Start Your Data Analytics Journey Today
If you're ready to step into one of the most promising careers of the future, then don’t settle for anything less than the best data analytics institute in Laxmi Nagar. With the best data analytics course in Laxmi Nagar, hands-on training, expert mentors, and strong placement support, we make sure your investment turns into long-term success.
#DataAnalytics#DataScience#AnalyticsTraining#BigData#DataVisualization#DataDriven#DataAnalyticsCourse#DataAnalyticsTraining#DataAnalyticsInstitute
0 notes
Text
Top In-Demand Data Analyst Jobs in 2025 & How an "Data Analyst Certification Course" Can Help, 100% Job in MNC, Excel, VBA, SQL, Power BI, Tableau Projects, "Data Analyst Certification Course" in Delhi, 110005 - Free Python Data Science Certification, By SLA Consultants India,
In 2025, the explosion of data across all industries has created an urgent demand for skilled professionals who can make sense of it. Data analysts are no longer limited to just one sector—they are needed everywhere, from tech startups to financial giants and healthcare institutions. As companies shift to data-driven strategies, roles in data analytics have diversified, giving rise to several high-paying and high-impact job profiles.
Enrolling in a comprehensive Data Analyst Course in Delhi, by SLA Consultants India, is the first step to preparing for these roles. Among the top in-demand data analyst jobs in 2025 are Business Intelligence Analyst, Financial Data Analyst, Marketing Analyst, Healthcare Analyst, and Data Quality Analyst. These roles require proficiency in tools like Excel, VBA, SQL, Power BI, and Tableau, which are essential for handling large datasets, automation, reporting, and data visualization. For example, a Business Intelligence Analyst uses Power BI to create dashboards for executive teams, while a Financial Analyst leverages Excel and SQL to build forecasting models. SLA Consultants India's course curriculum is designed to cover these critical tools with hands-on project experience, preparing students to meet the requirements of diverse industries.
What makes this Data Analyst Training Course in Delhi stand out is its strong focus on real-world projects and 100% job assistance in MNCs. The training includes practical assignments using actual business datasets, helping students gain industry-relevant experience. Whether it’s analyzing marketing campaign effectiveness or streamlining supply chain data, learners build a portfolio that employers can trust. The course also emphasizes soft skills like analytical thinking and communication—skills that are essential for presenting insights and making strategic recommendations in professional environments.
Adding more value to the program, SLA Consultants India provides a Free Python Data Science Certification, which is especially useful for those aspiring to move into more advanced roles such as Data Scientist or AI Analyst. Python is a must-have skill in today’s analytics landscape, allowing you to build predictive models, automate repetitive tasks, and work with big data more efficiently. Learning Python alongside Excel, SQL, and BI tools not only boosts your profile but also broadens your job prospects across international markets where hybrid and remote data roles are booming.
Data Analytics Training Course Module 1 – Basic and Advanced Excel With Dashboard and Excel Analytics Module 2 – VBA / Macros – Automation Reporting, User Form and Dashboard Module 3 – SQL and MS Access – Data Manipulation, Queries, Scripts and Server Connection – MIS and Data Analytics Module 4 – Tableau | MS Power BI BI & Data Visualization Module 5 – Python | R Programing BI & Data Visualization Module 6 – Python Data Science and Machine Learning – 100% Free in Offer – by IIT/NIT Alumni Trainer
In conclusion, the data analytics field in 2025 is full of opportunity, with job roles evolving to meet the dynamic needs of a data-centric world. The Data Analyst Certification Course in Delhi by SLA Consultants India gives you the skills, tools, and certifications necessary to thrive in this high-demand field. With hands-on training in Excel, Power BI, Tableau, and more—plus a free Python certification—this course is your launchpad to a successful career in one of the most future-proof industries today. To get more details Call: +91-8700575874 or Email: [email protected]
0 notes
Text
How Much Time Does It Take to Learn Data Science?
Hi everyone. Data science is one of the most exciting and in-demand careers today. From predicting trends to helping companies make better decisions, data scientists play a big role in our data-driven world. But many people wonder, how long does it take to learn data science?
The answer depends on a few things—your background, how much time you can commit, and what level you want to reach.
If You’re Starting from Scratch
If you’re completely new—no coding, no math background—learning data science may take about 12 to 18 months. This includes learning Python or R, basic statistics, data visualization, and machine learning. You’d also need to understand how to work with data using tools like Pandas, NumPy, and SQL. If you want to advance your career at the Data Science Course in Chennai, you need to take a systematic approach and join up for a course that best suits your interests and will greatly expand your learning path.
Spending 10–15 hours a week studying can help you reach a beginner-to-intermediate level within a year.
If you already know programming or statistics, you can learn faster. Many people with a tech background can pick up data science in 6 to 9 months, especially if they focus on real-world projects and hands-on practice.
Key Areas to Learn
To become a data scientist, you'll need to cover topics such as
Programming: Usually Python or R
Math & Statistics: For understanding patterns and building models
Data Analysis: Using tools like Excel, Pandas, and SQL
Machine Learning: Algorithms like decision trees and regression
Data Visualization: Tools like Matplotlib, Seaborn, or Tableau

With the aid of Best Online Training & Placement programs, which offer comprehensive training and job placement support to anyone looking to develop their talents, it’s easier to learn this tool and advance your career. Learning Paths
There are many ways to learn data science:
Online Courses: Websites like Coursera, edX, and Udemy
Bootcamps: Fast-paced programs (3–6 months full-time)
University Degrees: More in-depth but takes 2–4 year
Self-study: Flexible, but requires discipline
Conclusion
Learning data science is a journey, not a race. It can take anywhere from 6 months to 2 years, depending on your pace. The best way to speed up your learning is to build projects, join communities, and stay curious.
So don’t worry about how long it takes. Focus on steady progress, and you’ll get there before you know it!
0 notes