#parallel corpora
Explore tagged Tumblr posts
Text
ty @perotovar for the tag you cutie pie. I love silly little questions (I'm procrastinating making dinner and writing, so I'd love anything more than those things rn tbh)
me yapping below, if you'd like to know silly little answers to some questions
Do you make your bed?: a vague attempt at flapping my sheets is made most days, but it's never neatly made.
Favorite number?: 7! I can't tell you why it just has always been that way. when I found out my sun sign is in my 7th house I had an "of course 😌" moment even though I don't really even know what that means but it feels right.
What's your job?: books! and data!
If you could go back to school, would you?: there was a time I seriously considered a masters so I could go more ham with using corpora to study trends in language, but I didn't. now I literally work with data and databases and analysis of that data, and books have words in them so 🤷♀️
Can you parallel park?: I don't know what I'm capable of (I got my full license a little over a decade ago and can legally go buy a car and drive it all by myself even though honestly I have no clue if I can even drive any more and I'd probably be a major hazard. I've never driven a car with a full license, not as a learner)
Do you think aliens are real?: with how impossibly massive the universe is? duh. as long as they stay away though pls and thank you.
Can you drive a manual car?: I am licensed to, though once again, who knows what I am capable of (this sounds like a threat and maybe it is)
What's your guilty pleasure?: no guilty pleasures here!
Tattoos?: none! I've thought of various ideas for them for years and years and never committed to anything because I am so painfully indecisive. I will probably get one soon though, it is perfect but the idea makes me devastatingly sad. (a little print of my dogs paw on the top of my right foot - she always stands on it and always leaves a little indent and I'd like to walk with her forever)
Favorite color?: pink or yellow
Do you like puzzles?: fuck yes! we have one on the go called pumpkin patch right now. I'm very tempted by a colourful mushroom one too.
Any phobias?: yes, and I'm not typing it out because that makes me feel Worse™ and feeds into my OCD in ways I do not need at any time, let alone at nighttime.
Favorite childhood sport?: I hated team sports as a kid and still do now. I played rugby for a little while when I was 15, and liked when we did tennis or hockey during PE, but outside of that sport was not for me. turns out I'm a solo exercise bitch though.
Do you talk to yourself?: I am fortunate enough to have a dog who I can direct most of my chronic yapping towards, so I look less insane and feel less compelled to talk to myself. I do do the standard "what the fuck am I doing" and the like when I enter a room and immediately forget why I went there in the first place
no clue who has done this or not but @milla-frenchy @jolapeno @strang3lov3 @beefrobeefcal
and, finally, puppy tax for getting this far

18 notes
·
View notes
Text
fun facts about horse eyes:
the eyes rotate so the pupil is always parallel-ish to the ground
the pupil is kind of dark blue-grey
their pupils have their own lashes! XD

see how the pupil has those flaps overhanging it. it's called corpora nigra, apparently.
7 notes
·
View notes
Text
i think that paper that claims all the 17 languages it tested transmit information at the same bitrate is probably kinda bogus. it comes down to their way of estimating bitrate
In parallel, from independently available written corpora in these languages, we estimated each language’s information density (ID) as the syllable conditional entropy to take word-internal syllable-bigram dependencies into account.
this is a bad way of thinking about the information transmitted by language! its totally meaningless! i mean, its an interesting result, but i dont think this tells us about the different rates at which languages convey, yknow...meaning!
they defend it with this:
As a preliminary analysis, we checked whether our definition of ID provides a relevant measure of linguistic ID, using the syntagmatic density of information ratio (SDIR), defined in (9), as a control. SDIR quantifies the relative informational density of language L compared to a reference language, based on the semantic information expressed in the context of a limited oral corpus (see Materials and Methods below for more details). It thus provides the ground truth on the semantic information conveyed by the sentences in the spoken corpus. Following (9), we used Vietnamese as a reference, such that a language L with a ratio bigger than one (or, respectively, less than one) is denser (respectively, less dense) than Vietnamese in terms of semantic information. By contrast, being estimated from a very large written lexical database, ID subsumes an overall syllable usage disregarding any semantic consideration. The preliminary analysis nevertheless shows that the two information quantification approaches are connected; we obtain, for our data, a very high correlation between ID and SDIR (Pearson’s r = 0.91, P = 3.4 × 10−7 and Spearman’s ρ = 0.80, P = 0.00011), which suggests that, despite differences in material (heterogeneous and written corpus versus parallel and spoken corpus) and nature (an entropy measured on a large lexicon versus a normalized ratio derived from small texts), our ID is a good estimate of the average amount of information per syllable.
anyway the really bizarre thing about this study is they have this whole system where they have speakers read some pre-written text aloud to get the syllable rate, which they then combine with the syllable information density to get the total information rate, but surely the more natural measure is just...the time people take to say the same statement? a statement should have a fixed amount of semantic content! i mean, then you get into the ambiguities of translation. but that seems less bad than this silly definition of information
anyway the other study they cite is more in that vein, but considers information density as per syllable instead of syllable per time, but this one says that their per-time measurement *correlates* with the per-syllable measurement, but the per-time measurement already divided by syllables per second! which means their information-per-syllable measure has to be wrong, right? because they explicitly measure significant differences in syllables per second!
idk. it seems like a weird messy kind of poorly designed study. also both studies use read-aloud speech instead of produced speech and i know you kind of need to do that for standardization but also like come on man. you know that totally bungles your results. reading aloud is totally unlike thinking from your brain
29 notes
·
View notes
Text
June 12, 2023
QUESTION 5:
Give me information on the translation capabilities of OpenAI GPT-3
ChatGPT’s Response:
As of my knowledge cutoff in September 2021, OpenAI GPT-3, while primarily designed as a language model rather than a dedicated machine translation (MT) system, has demonstrated some translation capabilities. However, it's worth noting that GPT-3's performance in translation may not be on par with specialized MT systems. Here are some key points regarding GPT-3's translation capabilities:
Language Generation: GPT-3 is proficient in generating coherent and contextually appropriate text, which includes generating translations for given prompts or text inputs.
Multilingual Capabilities: GPT-3 has the ability to understand and generate text in multiple languages. It can be used for translation tasks involving various language pairs.
Limitations: While GPT-3 can generate translations, it is important to note that its translation capabilities may not match those of dedicated MT systems, especially for complex or specialized domains. GPT-3 may lack domain-specific knowledge, specific terminology, or fine-grained control over translation quality.
Fine-Tuning: GPT-3 can be further fine-tuned on translation-specific datasets to improve its performance in translation tasks. Fine-tuning helps adapt the model to a specific domain or improve its accuracy in generating translations.
Post-Editing: Like other MT systems, translations generated by GPT-3 may require post-editing by human translators to ensure accuracy, fluency, and adherence to specific requirements. Human intervention can help refine the output and address any linguistic or cultural nuances that may have been missed by the model.
It's worth mentioning that the primary focus of GPT-3 is on language generation, and specialized MT systems like Google Translate, Microsoft Translator, or DeepL are typically more widely used and have dedicated resources for translation tasks. These specialized systems undergo extensive training on large amounts of parallel corpora and utilize specific algorithms and techniques to optimize translation quality.
While GPT-3 can provide useful translations in certain scenarios, it is important to evaluate its performance based on specific requirements and consider specialized MT systems for critical or high-quality translation needs. Additionally, advancements and updates may have been made in GPT-3's translation capabilities since my knowledge cutoff, so it's advisable to refer to the latest information from OpenAI for the most up-to-date insights.
2 notes
·
View notes
Text
Bite-Sized Brilliance: Small Plates, Big Impressions

In the world of catering, size doesn’t always matter—especially when it comes to small plates. At The Sole Ingredient Catering, we know that when done right, bite-sized dishes can deliver bold flavor, stunning presentation, and unforgettable guest experiences. Whether you’re planning a chic cocktail hour, a corporate mixer, or a stylish reception, small plates can pack a serious punch.
Let’s dive into why going small might be the smartest move you make for your next event.
1. First Impressions—Delivered in One Bite
Small plates are a visual and flavorful statement. When guests walk into your event and are greeted with carefully crafted mini dishes, their eyes light up—and so do their appetites. These tiny creations are often beautifully plated, garnished with precision, and bursting with flavor, creating an instant wow factor.
They set the tone. These dishes whisper (or sometimes shout), “This event is something special.”

2. Built for Mingling, Networking & Movement
Small plates are designed with flexibility in mind. At standing events or cocktail hours, they make it easy for guests to eat, drink, and network without juggling full plates or needing to sit down. The portion size encourages sampling and conversation—perfect for keeping the vibe energetic and interactive.
Whether it’s a mini crab cake with aioli or a petite caprese skewer, guests can enjoy a full culinary experience in just a few bites—no formal seating required.
3. A Tasting Tour Without Leaving the Room
One of the biggest benefits of bite-sized dishes? Variety.
Instead of committing to one entrée, guests get to enjoy a tasting journey. You can explore different cuisines, textures, and presentations all within one event. From Southern staples to global flavors, small plates allow you to showcase the diversity and creativity of your menu.
At The Sole Ingredient, we love designing small plate menus that reflect your event’s theme and personality while giving your guests options that surprise and satisfy.
4. Perfect Pairings with Cocktails or Mocktails
Small bites and drinks go hand-in-hand. These elegant portions are ideal for pairing with signature cocktails or wine selections. A mini lamb lollipop pairs beautifully with a bold red wine. A spicy shrimp tostada? Try it with a jalapeño-infused margarita. The magic is in the match.
Even non-alcoholic options get elevated—think fresh fruit shooters with herb-infused sparkling water or mini cheesecakes with espresso shots.
5. Elegant Control Over Portions & Presentation
Small plates give chefs creative control. Every plate is individually composed, garnished, and presented with intention. This level of detail elevates the aesthetic of your event and gives your guests something to remember (and photograph!).
From edible flowers to handcrafted sauces, we take pride in plating each bite with artistry. This attention to detail is part of what makes our service stand out.

6. Customizable to Dietary Needs
Hosting a group with mixed dietary preferences or restrictions? Small plates make it easy to cater to everyone.
Vegetarian, vegan, gluten-free, dairy-free—there’s no need to compromise. Instead of reworking one big menu item for the whole crowd, small plates let you offer specialized options in parallel. It’s inclusive, it’s thoughtful, and it ensures every guest feels seen and satisfied.
We label everything clearly and work with you to make sure no detail is missed.
7. Less Waste, More Experience
With traditional buffets or oversized entrees, food waste can be a concern. Small plates encourage mindful eating. Guests take what they want, try more variety, and often waste less. Plus, since everything is portioned and served with care, leftovers are easier to manage and your event stays clean and polished.
At The Sole Ingredient, we care about sustainability, and this service style supports that effort beautifully.
8. Great for Brand or Theme Integration
Planning a corporate event or product launch? Small plates are a clever way to integrate your brand into the menu. We can design custom dishes that reflect your company’s color palette, seasonal theme, or launch concept. Think: gold-dusted desserts for a luxury brand or vibrant veggie skewers for a wellness campaign.
Even at weddings and milestone parties, you can weave your story into the plate—literally.
Conclusion: Big Impact in Every Bite
Small plates don’t mean small impact. In fact, they do just the opposite. They give you the power to wow guests from the moment they arrive, offer culinary variety, and maintain an elevated, interactive experience throughout your event.
At The Sole Ingredient Catering, we bring flavor, flair, and function together in every plate—big or small. Let us help you design a bite-sized menu that speaks volumes about your style and taste.
0 notes
Text
A Modern Approach to English to Odia Translation
Translation from one language to another has become a significant concern in this world. Millions of Odia speakers exist everywhere on the globe, and they need information and communication in their mother tongue. Here is the role that English to Odia translation plays. The article below deals with modern approaches to this translation process, considering the movements as well as the challenges in it.
The Translation Revolution
Traditionally, translation was laborious, depending upon mere bilingualism and dictionary use. However, technology has dramatically altered the situation of translation in many ways. Modern translation bears no resemblance whatsoever to what it was done before. Today, it forms part of the translation landscape to incorporate machine translation systems that work on complex algorithms and giant data banks.
Contingent Modern Methods of Translating from English to Odia
Various contingent modern methods are followed for translating from English into Odia, with different merits and demerits associated with each method.
1. SMT or Statistical Machine Translation: This relies on statistical models learned from large parallel corpora, which, in this case, means texts in both Odia and English, to predict the most probable translation of an English text given. It is effective at translating routine phrases and sentences but becomes weaker with more complex grammatical structures and idiomatic expressions.
2. NMT or Neural Machine Translation: The newest approach with artificial neural networks, especially the deep learning model, is to learn about the complex relationships between languages. NMTs have proved to be excellent systems in improvement with fluently natural-sounding translations over SMTs. Contextual is better with sensitivity towards language nuances.
3. Hybrid Approaches: Hybrid approaches combine the best of SMT and NMT, using statistical models for specific tasks and neural networks for others. This may result in more efficient and accurate translation outcomes.
4. RBMT: Not so popular these days, RBMT systems rely on linguistic rules and grammar to translate from one language to another. Although they produce an extremely accurate translation in a specific domain, they still require extensive manual work to develop and maintain the rules.
MT has come a long way, but human translators still play an essential role in maintaining quality. Their linguistic and cultural backgrounds describe nuances and produce accurate translations that sound natural as if they were born from the native speaker's vocabulary. Besides, they furnish the necessary post-editing of MT output, error correction, and enhancement of the quality of translation.
Future of English to Odia Translation
The future of English to Odia translation seems bright with continuous research and development in MT and other related technologies. Improvements in deep learning, natural language processing, and data collection will take the MT systems forward toward high accuracy and fluency. Further, increased digital resources and online platforms have been helping translators and researchers collaborate and share knowledge.
New ways of translating English into Odia, especially NMT, have significantly increased efficiency and quality. However, bottom-line problems persist as humans dominate and command this sector. Combining the best from the technology bag and human skills handbags, we can ensure effective communication between the English-speaking or Odia-speaking populations for better interaction and cooperation among people moving around in this increasingly interdependent world.
#Odia Translation Solution#English to Odia Translation#Modern Translation Solutions#Language Translation India#Odia Language Solutions#Translation Technology#AI Powered Translation#Odia Global Connect#Breaking Language Barriers
0 notes
Text
What Are the Technical Requirements for Private LLM Development Services in 2025?

The demand for private Large Language Model (LLM) development services is set to skyrocket in 2025, as businesses increasingly leverage AI for customized applications. These models offer transformative capabilities, ranging from automating complex workflows to enabling sophisticated natural language understanding. However, building private LLMs comes with its own set of technical requirements that organizations must carefully address. This blog explores the key aspects that define the technical requirements for private LLM development services in 2025.
1. Hardware Infrastructure
Private LLM development demands robust and scalable hardware infrastructure to handle intensive computations, vast datasets, and real-time inference capabilities. The following components form the backbone of hardware requirements:
a. GPUs and TPUs Modern LLMs, such as GPT-4 and its successors, require specialized hardware like Graphics Processing Units (GPUs) and Tensor Processing Units (TPUs). These processors accelerate training by handling parallel computations and matrix operations. NVIDIA’s H100 GPUs and Google’s TPU v5 are leading choices in 2025, providing higher memory bandwidth and faster throughput.
b. High-Performance Storage Efficient storage solutions are critical for managing terabytes or even petabytes of data required for LLM training. NVMe SSDs and parallel storage systems ensure faster data retrieval and reduced bottlenecks during training.
c. Network Bandwidth Distributed training requires high-speed interconnects to synchronize parameters across multiple nodes. Technologies like NVIDIA’s NVLink and InfiniBand are essential to reduce latency and maximize throughput.
2. Data Requirements
The quality and diversity of data directly influence the performance of private LLMs. Organizations must address several data-related requirements:
a. Dataset Collection Acquiring domain-specific, high-quality data is paramount. Companies often need proprietary datasets supplemented with publicly available corpora to train their models effectively.
b. Data Preprocessing Before feeding data into LLMs, extensive preprocessing is necessary. This includes cleaning, deduplication, tokenization, and normalizing text. Tools like spaCy and Hugging Face’s Transformers library provide essential preprocessing utilities.
c. Data Privacy and Compliance Privacy regulations such as GDPR, CCPA, and sector-specific laws like HIPAA (for healthcare) demand strict data governance. Techniques such as differential privacy and data anonymization are indispensable for ensuring compliance.
3. Model Architecture and Customization
Private LLMs must align with specific business objectives, necessitating tailored architectures and training methodologies:
a. Custom Architectures While foundational models like GPT and T5 provide a base, customization is required to adapt these architectures for specific use cases. Adding domain-specific embeddings, fine-tuning on niche tasks, or developing hybrid architectures (e.g., integrating vision and language) enhances functionality.
b. Transfer Learning Transfer learning minimizes computational costs by leveraging pre-trained models and fine-tuning them on domain-specific datasets. This method has proven highly effective for deploying private LLMs in specialized fields like legal, finance, and healthcare.
c. Parameter Optimization Large models with billions of parameters require careful optimization to balance performance and computational cost. Techniques such as parameter pruning, quantization, and distillation play crucial roles in reducing model size while maintaining accuracy.
4. Development Frameworks and Tools
Developers require advanced frameworks and tools to build, train, and deploy private LLMs efficiently. Popular choices in 2025 include:
a. TensorFlow and PyTorch These frameworks remain the go-to for deep learning development. PyTorch’s dynamic computation graph and TensorFlow’s extensive ecosystem make them ideal for building and experimenting with LLM architectures.
b. Hugging Face Transformers This library simplifies the process of training and fine-tuning transformer models. It provides pre-trained checkpoints, tokenizers, and utilities that reduce development time.
c. MLOps Platforms Platforms like Weights & Biases, MLflow, and SageMaker are essential for managing the end-to-end lifecycle of LLM development—from version control to deployment monitoring.
5. Scalability and Distributed Training
LLM training is computationally intensive and often exceeds the capacity of a single machine. Distributed training techniques and infrastructure ensure scalability:
a. Model Parallelism Splitting a model across multiple GPUs or nodes helps manage memory limitations and enables training of larger models.
b. Data Parallelism This approach distributes data across nodes, allowing each to train on a subset of the data while synchronizing gradients periodically.
c. Federated Learning For organizations handling sensitive data, federated learning allows model training across decentralized datasets without transferring sensitive data to a central location.
6. Security and Access Control
Security is a cornerstone of private LLM development, especially for sensitive applications in sectors like healthcare, finance, and defense:
a. Encrypted Data Pipelines Data transmission during training and inference should be encrypted using protocols like TLS 1.3 and AES-256.
b. Role-Based Access Control (RBAC) Fine-grained access control ensures that only authorized personnel can access specific parts of the system or data.
c. Secure Model Hosting Models should be hosted on secure platforms with robust firewalls, intrusion detection systems, and regular security audits.
7. Ethical AI and Bias Mitigation
Private LLMs must adhere to ethical AI principles to avoid unintended consequences:
a. Bias Detection and Correction Pre-trained models often inherit biases from training data. Post-training evaluation and debiasing techniques help mitigate these issues.
b. Explainability Organizations must implement tools to make LLMs’ predictions interpretable. Techniques like SHAP (SHapley Additive exPlanations) and LIME (Local Interpretable Model-agnostic Explanations) are valuable.
c. Adherence to AI Ethics Guidelines Adopting frameworks such as the EU’s AI Act or NIST’s AI Risk Management Framework ensures responsible AI deployment.
8. Deployment and Inference Optimization
Once trained, LLMs need to be deployed efficiently to ensure low latency and scalability during inference:
a. Edge Computing Deploying LLMs on edge devices reduces latency and ensures real-time processing, particularly for IoT applications.
b. Containerization and Orchestration Technologies like Docker and Kubernetes simplify model deployment, enabling scalable and reliable inference environments.
c. Optimization Techniques Dynamic quantization, mixed precision inference, and hardware acceleration (e.g., using NVIDIA TensorRT) improve performance and reduce costs.
9. Monitoring and Maintenance
Continuous monitoring and maintenance are critical to ensure that private LLMs remain accurate and relevant:
a. Performance Metrics Metrics like BLEU, ROUGE, and perplexity help evaluate model performance and guide iterative improvements.
b. Drift Detection Regularly checking for data and model drift ensures the model remains aligned with evolving requirements and user expectations.
c. Automated Updates Implementing pipelines for automated re-training and updates helps keep the model up-to-date with minimal manual intervention.
Conclusion
Private LLM development in 2025 requires a comprehensive approach that encompasses cutting-edge hardware, high-quality data, sophisticated architectures, and stringent security measures. By addressing these technical requirements, organizations can unlock the full potential of LLMs while ensuring compliance, scalability, and ethical AI practices. As the AI landscape continues to evolve, staying ahead of these requirements will be crucial for businesses aiming to harness the power of private LLMs.
#Private LLM Development Services#Private LLM Development#Private LLM#LLM Development Services#LLM Development#LLM
0 notes
Text
Have a feeling it's about time to leave Duolingo. With AI translations, it's likely to have lots of errors introduced into lessons.
I also wonder if this is related to the cancellation of a lot of minority language courses. AI cannot handle translations of smaller languages because the parallel corpora don't exist.
@ duolingo users
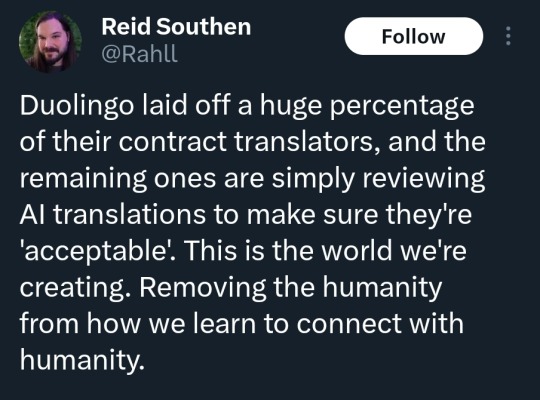

More ai shit taking jobs
21K notes
·
View notes
Text
Transformer Impact: Has Machine Translation Been Solved?
New Post has been published on https://thedigitalinsider.com/transformer-impact-has-machine-translation-been-solved/
Transformer Impact: Has Machine Translation Been Solved?
Google recently announced their release of 110 new languages on Google Translate as part of their 1000 languages initiative launched in 2022. In 2022, at the start they added 24 languages. With the latest 110 more, it’s now 243 languages. This quick expansion was possible thanks to the Zero-Shot Machine Translation, a technology where machine learning models learn to translate into another language without prior examples. But in the future we will see together if this advancement can be the ultimate solution to the challenge of machine translation, and in the meanwhile we can explore the ways it can happen. But first its story.
How Was it Before?
Statistical Machine Translation (SMT)
This was the original method that Google Translate used. It relied on statistical models. They analyzed large parallel corpora, collections of aligned sentence translations, to determine the most likely translations. First the system translated text into English as a middle step before converting it into the target language, and it needed to cross-reference phrases with extensive datasets from United Nations and European Parliament transcripts. It’s different to traditional approaches that necessitated compiling exhaustive grammatical rules. And its statistical approach let it adapt and learn from data without relying on static linguistic frameworks that could quickly become completely unnecessary.
But there are some disadvantages to this approach, too. First Google Translate used phrase-based translation where the system broke down sentences into phrases and translated them individually. This was an improvement over word-for-word translation but still had limitations like awkward phrasing and context errors. It just didn’t fully understand the nuances as we do. Also, SMT heavily relies on having parallel corpora, and any relatively rare language would be hard to translate because it doesn’t have enough parallel data.
Neural Machine Translation (NMT)
In 2016, Google made the switch to Neural Machine Translation. It uses deep learning models to translate entire sentences as a whole and at once, giving more fluent and accurate translations. NMT operates similarly to having a sophisticated multilingual assistant within your computer. Using a sequence-to-sequence (seq2seq) architecture NMT processes a sentence in one language to understand its meaning. Then – generates a corresponding sentence in another language. This method uses huge datasets for learning, in contrast to Statistical Machine Translation which relies on statistical models analyzing large parallel corpora to determine the most probable translations. Unlike SMT, which focused on phrase-based translation and needed a lot of manual effort to develop and maintain linguistic rules and dictionaries, NMT’s power to process entire sequences of words lets it capture the nuanced context of language more effectively. So it has improved translation quality across various language pairs, often getting to levels of fluency and accuracy comparable to human translators.
In fact, traditional NMT models used Recurrent Neural Networks – RNNs – as the core architecture, since they are designed to process sequential data by maintaining a hidden state that evolves as each new input (word or token) is processed. This hidden state serves as a sort of a memory that captures the context of the preceding inputs, letting the model learn dependencies over time. But, RNNs were computationally expensive and difficult to parallelize effectively, which was limiting how scalable they are.
Introduction of Transformers
In 2017, Google Research published the paper titled “Attention is All You Need,” introducing transformers to the world and marking a pivotal shift away from RNNs in neural network architecture.
Transformers rely only on the attention mechanism, – self-attention, which allows neural machine translation models to focus selectively on the most critical parts of input sequences. Unlike RNNs, which process words in a sequence within sentences, self-attention evaluates each token across the entire text, determining which others are crucial for understanding its context. This simultaneous computation of all words enables transformers to effectively capture both short and long-range dependencies without relying on recurrent connections or convolutional filters.
So by eliminating recurrence, transformers offer several key benefits:
Parallelizability: Attention mechanisms can compute in parallel across different segments of the sequence, which accelerates training on modern hardware such as GPUs.
Training Efficiency: They also require significantly less training time compared to traditional RNN-based or CNN-based models, delivering better performance in tasks like machine translation.
Zero-Shot Machine Translation and PaLM 2
In 2022, Google released support for 24 new languages using Zero-Shot Machine Translation, marking a significant milestone in machine translation technology. They also announced the 1,000 Languages Initiative, aimed at supporting the world’s 1,000 most spoken languages. They have now rolled out 110 more languages. Zero-shot machine translation enables translation without parallel data between source and target languages, eliminating the need to create training data for each language pair — a process previously costly and time-consuming, and for some pair languages also impossible.
This advancement became possible because of the architecture and self-attention mechanisms of transformers. Thetransformer model’s capability to learn contextual relationships across languages, as a combo with its scalability to handle multiple languages simultaneously, enabled the development of more efficient and effective multilingual translation systems. However, zero-shot models generally show lower quality than those trained on parallel data.
Then, building on the progress of transformers, Google introduced PaLM 2 in 2023, which made the way for the release of 110 new languages in 2024. PaLM 2 significantly enhanced Translate’s ability to learn closely related languages such as Awadhi and Marwadi (related to Hindi) and French creoles like Seychellois and Mauritian Creole. The improvements in PaLM 2’s, such as compute-optimal scaling, enhanced datasets, and refined design—enabled more efficient language learning and supported Google’s ongoing efforts to make language support better and bigger and accommodate diverse linguistic nuances.
Can we claim that the challenge of machine translation has been fully tackled with transformers?
The evolution we are talking about took 18 years from Google’s adoption of SMT to the recent 110 additional languages using Zero-Shot Machine Translation. This represents a huge leap that can potentially reduce the need for extensive parallel corpus collection—a historically and very labor-extensive task the industry has pursued for over two decades. But, asserting that machine translation is completely addressed would be premature, considering both technical and ethical considerations.
Current models still struggle with context and coherence and make subtle mistakes that can change the meaning you intended for a text. These issues are very present in longer, more complex sentences where maintaining the logical flow and understanding nuances is needed for results. Also, cultural nuances and idiomatic expressions too often get lost or lose meaning, causing translations that may be grammatically correct but don’t have the intended impact or sound unnatural.
Data for Pre-training: PaLM 2 and similar models are pre trained on a diverse multilingual text corpus, surpassing its predecessor PaLM. This enhancement equips PaLM 2 to excel in multilingual tasks, underscoring the continued importance of traditional datasets for improving translation quality.
Domain-specific or Rare Languages: In specialized domains like legal, medical, or technical fields, parallel corpora ensures models encounter specific terminologies and language nuances. Advanced models may struggle with domain-specific jargon or evolving language trends, posing challenges for Zero-Shot Machine Translation. Also Low-Resource Languages are still poorly translated, because they do not have the data they need to train accurate models
Benchmarking: Parallel corpora remain essential for evaluating and benchmarking translation model performance, particularly challenging for languages lacking sufficient parallel corpus data.The automated metrics like BLEU, BLERT, and METEOR have limitations assessing nuance in translation quality apart from grammar. But then, we humans are hindered by our biases. Also, there are not too many qualified evaluators out there, and finding the perfect bilingual evaluator for each pair of languages to catch subtle errors.
Resource Intensity: The resource-intensive nature of training and deploying LLMs remains a barrier, limiting accessibility for some applications or organizations.
Cultural preservation. The ethical dimension is profound. As Isaac Caswell, a Google Translate Research Scientist, describes Zero-Shot Machine Translation: “You can think of it as a polyglot that knows lots of languages. But then additionally, it gets to see text in 1,000 more languages that isn’t translated. You can imagine if you’re some big polyglot, and then you just start reading novels in another language, you can start to piece together what it could mean based on your knowledge of language in general.” Yet, it’s crucial to consider the long-term impact on minor languages lacking parallel corpora, potentially affecting cultural preservation when reliance shifts away from the languages themselves.
#000#2022#2023#2024#Accessibility#applications#approach#architecture#attention#attention mechanism#barrier#benchmarking#Building#Capture#challenge#change#CNN#Collections#computation#computer#data#datasets#Deep Learning#deploying#Design#development#domains#efficiency#English#european parliament
0 notes
Text
What is ChatGpt
Introduction: Unraveling the Essence of ChatGPT
At its core, ChatGPT epitomizes the culmination of cutting-edge advancements in natural language processing (NLP) and machine learning. Developed by OpenAI, ChatGPT is an exemplar of Generative Pre-trained Transformer technology, specifically engineered to engage in human-like conversations seamlessly. Its prowess lies in its ability to comprehend, generate, and respond to text inputs with remarkable coherence and contextuality.
Understanding the Architecture
ChatGPT's architecture is anchored in the Transformer model, a paradigm-shifting architecture that has redefined the NLP landscape. At its essence, the Transformer model employs self-attention mechanisms to process input sequences in parallel, enabling ChatGPT to grasp the nuances of language with unprecedented finesse. Through a process of pre-training on vast corpora of text data, ChatGPT develops a nuanced understanding of language patterns, semantics, and contextual cues, empowering it to generate contextually relevant responses in real-time.
Unveiling the Capabilities
The versatility of ChatGPT knows no bounds, manifesting across a myriad of domains and applications. From customer service chatbots to virtual assistants and content generation tools, ChatGPT seamlessly integrates into various platforms, enriching user experiences and streamlining operations. Its adaptability allows it to cater to diverse use cases, ranging from educational assistance to creative writing and beyond.
Harnessing the Power of Conversational AI
In an era dominated by digital interactions, the significance of conversational AI cannot be overstated. ChatGPT serves as a cornerstone in this paradigm shift, bridging the gap between humans and machines with unparalleled sophistication. By imbuing machines with the ability to comprehend and generate natural language, ChatGPT fosters more intuitive and immersive user experiences across a spectrum of applications.
For more information visit us :
1 note
·
View note
Text
Natural Language Processing: A Machine Learning Perspective
Natural Language Processing (NLP) stands at the intersection of computer science, artificial intelligence, and linguistics, aiming to bridge the gap between human communication and machines. In recent years, NLP has witnessed remarkable advancements, primarily driven by machine learning techniques. This article explores NLP from a machine learning perspective, delving into key concepts, challenges, and the transformative potential of this dynamic field.
Understanding Natural Language Processing
NLP involves the development of algorithms and models that enable machines to understand, interpret, and generate human language. It encompasses a wide range of tasks, from basic ones like language translation and sentiment analysis to more complex challenges like text summarization and question answering. The ultimate goal of NLP is to equip machines with the ability to comprehend and interact with human language in a way that is both meaningful and contextually relevant.
The Role of Machine Learning in NLP
Machine learning plays a pivotal role in the evolution of NLP, providing the tools and techniques necessary for computers to learn patterns and relationships within language data. Supervised learning, unsupervised learning, and reinforcement learning are common paradigms employed in NLP. Supervised learning involves training models on labeled datasets, while unsupervised learning explores patterns in unlabeled data. Reinforcement learning, on the other hand, emphasizes learning through interaction with an environment, receiving feedback in the form of rewards or penalties.
Tokenization and Word Embeddings
Tokenization is a fundamental preprocessing step in NLP, involving the segmentation of text into smaller units, such as words or subwords. Machine learning models often operate on tokenized representations of text. Word embeddings, another crucial concept, represent words as dense vectors in a continuous vector space, capturing semantic relationships between words. Popular word embedding techniques, like Word2Vec and GloVe, contribute significantly to enhancing the performance of NLP models.
Sentiment Analysis: Unveiling Emotions in Text
Sentiment analysis, a prominent NLP application, involves determining the emotional tone expressed in a piece of text. Machine learning models trained on labeled sentiment datasets can classify text as positive, negative, or neutral. This capability finds applications in business, marketing, and social media monitoring, enabling organizations to gauge public opinion and customer sentiment.
Named Entity Recognition: Extracting Meaningful Information
Named Entity Recognition (NER) is a key task in NLP that focuses on identifying and classifying entities such as names of people, organizations, locations, and dates within text. Machine learning models, particularly those based on recurrent neural networks (RNNs) and transformers, have demonstrated high accuracy in extracting meaningful information from unstructured text, facilitating tasks like information retrieval and knowledge extraction.
Machine Translation: Breaking Language Barriers
Machine translation, a classic problem in NLP, involves automatically translating text from one language to another. With the advent of neural machine translation models, powered by deep learning architectures like transformers, the accuracy and fluency of machine translation have significantly improved. These models leverage large parallel corpora to learn the intricate relationships between languages, breaking down language barriers and fostering global communication.
Challenges in Natural Language Processing
While NLP has made tremendous strides, it faces several challenges. Ambiguity in language, cultural nuances, and evolving linguistic trends pose obstacles to accurate language understanding. Additionally, biases present in training data can result in biased models, reinforcing societal stereotypes. Ethical considerations in NLP, including fairness and transparency, remain critical as these technologies become increasingly integrated into various aspects of our lives.
The Future of NLP: Multimodal Approaches and Beyond
The future of NLP holds exciting possibilities, with the integration of multimodal approaches that combine text, images, and other forms of data. This evolution allows machines to understand and generate content across multiple modalities, paving the way for more immersive and context-aware applications. As technology advances, NLP will likely extend its capabilities to simulate natural conversations, enabling machines to engage in more human-like interactions.
Natural Language Processing, propelled by machine learning, has revolutionised the way machines interact with human language. From sentiment analysis to machine translation, NLP applications continue to permeate various industries, offering solutions to complex linguistic challenges. As we navigate the future, ethical considerations and a commitment to minimising biases will be integral to the responsible development and deployment of NLP technologies. Embracing the machine learning perspective in NLP ensures that we harness the power of algorithms to enhance communication, understanding, and collaboration between humans and machines
#elearning#onlinetraining#career#learning#programming#technology#automation#online courses#security#startups
0 notes
Text
The Last Course for Humans of this term--Chanyuan Corpus and Xuefeng Corpus

The vast and eternal universe is populated by countless varieties of LIVES at many different levels, the earth where humans live is a transit station through which LIFE beings at higher and lower spaces can pass. For countless millennia, people have been searching and reincarnating endlessly in darkness and suffering, however they still fail to have ideal lives or enter into higher LIFE spaces. Jesus Christ and Buddha Sakyamuni were mercifully born into the world; Jesus sacrificed his LIFE to point out the way and Buddha devoted his heart and soul to create a vessel heading toward heaven.
Having struggled and waited in ignorance and suffering for two thousand years, humans finally have Xuefeng, an angel who came to harvest “ripe crops”. Xuefeng spent more than twenty years writing “Chanyuan Corpus” and “Xuefeng Corpus” and has devoted all his possessions toward the founding of Lifechanyuan. He brought the last course for humans to this term and has led those ripe crops (Chanyuan celestials) to copy the lifestyle of the Thousand-year World here on earth and created an ideal new life model for mankind.
Chanyuan Corpus and Xuefeng Corpus have proven with forty examples with eight forms of logic the existence of the Greatest Creator, the creator of LIFE in the universe; they have illustrated eight features of the Greatest Creator and the relationship between the Greatest Creator and us. They have also explained the eight features of Tao and the meaning of “the way of the Greatest Creator”.
Here are six things that those Corpora have brought to us:
1.They have described the origins of the Greatest Creator, the universe, LIFE, and of humans, have explained the structure of the universe and the secrets of time and space, and have revealed the secret of latitudinal time and space.
2.They first revealed the existence of the non-material world and have given brief descriptions of thirty-six (36) dimensional spaces and twenty (20) parallel worlds. They have described real scenes in heaven - the Thousand-year World, the Ten-thousand-year World, and the Celestial Islands Continent of the Elysium World.
3.They have thoroughly dissected the essence of LIFE, illustrated the levels of all LIFE in the universe, and explained the meaning of LIFE's existence. They also stated the truth and meaning of life and death and explained the principle of how LIFE reincarnates and evolves in different spaces.
4.They have illustrated humanity’s role and the meaning of our existence in great detail. They have pointed out that the root causes of human suffering are the existences of families, countries, religions, and political parties, and that the crisis of the soul is the core of all crises that we face. They have given a complete and specific solution to the elimination of all suffering and crises and paved a path through which mankind can enter into a completely new era.
5.They have dissected the root causes of human misery and have pointed out the value and meaning of our short lives. Not only have they indicated how to escape from the sea of bitterness so as to enjoy happy and blessed lives, but they have also shown us a detailed map that we could ascend to become celestials and enter higher levels of LIFE spaces which pave a perfect path for one’s life and LIFE.
6.They have disclosed the secrets of our thinking and unfolded its eight ladders. They have also revealed the truths of love and sex and the real meaning of predestined relationships. They have also dissected the secrets of the three key elements of the universe: consciousness, structure, and energy, and have shown the mystery of Thirty-Six mazes; they have given answers to much of our confusion and many of our riddles from ancient times.
Chanyuan Corpus and Xuefeng Corpus are deep and profound, all-encompassing, unendingly miraculous, and they integrate the essence of science and philosophy, and teachings of Gods, Buddhas, Celestials, and saints. They reveal the truth of the universe and of LIFE, and confirm the ultimate belief of the unity of mankind, and eliminate the defects and confusions of human consciousness from ancient times. They pave a bright road to heaven for humans, and are an invaluable treasure and a ray of hope. They are the messages from Gods and Buddhas to save humans, the grace from the Greatest Creator, and an unbelievable fortune and opportunity.
People have committed many sins throughout their long history, and since the Great Flood, another catastrophe has been escalating on the earth in a stepped way, however a great soul purification is also happening simultaneously. All consciousnesses that fail to resonate with the frequency of the new era will be eliminated gradually and our traditional lifestyle will be replaced by a new one; an unprecedented civilized paradisiacal era is coming. All those who want to survive the catastrophe and live in the new era, and all who want to escape the sea of bitterness and live in this eternal paradise must study the last course for humans of this term as soon as possible.
0 notes
Text
October 30, 2023
QUESTION 73:
What are some ways that LSP's can leverage AI and machine learning advancements to enhance the accuracy and efficiency of their translation memory systems?
Chat GPT's Response:
Language Service Providers (LSPs) can leverage AI and machine learning advancements to enhance the accuracy and efficiency of their translation memory systems in several ways:
Machine Translation Integration:
Integrate machine translation engines into your TM systems to pre-translate content automatically. Modern machine translation models, like neural machine translation, can provide high-quality suggestions that can be used as a starting point for human translators.
Quality Estimation Models:
Implement quality estimation models that use AI and machine learning to predict the quality of a translation. These models can help identify segments that may require additional human review, saving time and improving translation accuracy.
Customized Machine Learning Models:
Train custom machine learning models specific to your domain and clients. This can improve the relevancy of translation memory suggestions and make them more aligned with your specific content needs.
Continuous Learning and Improvement:
Develop systems that learn and adapt over time. This involves continuously updating your translation memory with the latest translations and improving the algorithms used for matching and suggestion ranking.
Data Augmentation:
Use machine learning techniques to augment your translation memory with publicly available parallel corpora. This can help fill gaps and improve the quality of suggestions, especially for less common language pairs.
Post-Editing Automation:
Implement post-editing automation using machine learning models. These models can help automate the post-editing process by suggesting edits to machine-generated translations, making the work of human translators more efficient.
Data Cleaning and Maintenance:
Use machine learning to identify and clean up outdated or low-quality translation memories. Regularly review and update your translation memory data to ensure its accuracy and relevance.
By effectively integrating AI and machine learning advancements into their translation memory systems, LSPs can significantly enhance the accuracy and efficiency of their translation processes, ultimately providing higher-quality translations and more cost-effective services to their clients.
0 notes
Text
TOC: International Journal of Corpus Linguistics Vol. 28, No. 4 (2023)
ICYMI: 2023. iii, 136 pp. Table of Contents Articles When loanwords are not lone words: Using networks and hypergraphs to explore Māori loanwords in New Zealand English David Trye, Andreea S. Calude, Te Taka Keegan & Julia Falconer pp. 461–499 A proposal for the inductive categorisation of parenthetical discourse markers in Spanish using parallel corpora Hernán Robledo & Rogelio Nazar pp. 500–527 “You betcha I’m a ’Merican”: The rise of YOU BET as a pragmatic marker Tomoharu Hirota & Laurel J. Br http://dlvr.it/Stn0dB
0 notes
Link
An interesting article about the social process of creating machine translation datasets in Khoekhoegowab. Excerpt:
On the surface, Wilhelmina Ndapewa Onyothi Nekoto and Elfriede Gowases seem like a mismatched pair. Nekoto is a 26-year-old data scientist. Gowases is a retired English teacher in her late 60s. Nekoto, who used to play rugby in Namibia’s national league, stands about a head taller than Gowases, who is short and slight. Like nearly half of Namibians, Nekoto speaks Oshiwambo, while Gowases is one of the country’s roughly 200,000 native speakers of Khoekhoegowab.
But the women grew close over a series of working visits starting last October. At Gowases’s home, they translated sentences from Khoekhoegowab to English. Each sentence pair became another entry in a budding database of translations, which Nekoto hopes will one day power AI tools that can automatically translate between Namibia’s languages, bolstering communication and commerce within the country.
“If we can design applications that are able to translate what we’re saying in real time,” Nekoto says, “then that’s one step closer toward economic [development].” That’s one of the goals of the Masakhane project, which organizes natural language processing researchers like Nekoto to work on low-resource African languages.
Compiling a dataset to train an AI model is often a dry, technical task. But Nekoto’s self-driven project, rooted in hours of close conversation with Gowases, is anything but. Each datapoint contains fragments of cultural knowledge preserved in the stories, songs, and recipes that Gowases has translated. This information is as crucial for the success of a machine translation algorithm as the grammar and syntax embedded in the training data.
Read the whole thing.
#linguistics#khoekhoegowab#namibia#khoekhoe#masakhane#natural language processing#nlp#nlproc#machine translation#corpora#parallel corpora#ai#data#low-resource languages#low resource languages#digitally disadvantaged languages#data science
169 notes
·
View notes
Link
via ACM Transactions on Computer-Human Interaction (TOCHI). Vol 29, Issue 2. https://doi.org/10.1145/3487193
8 notes
·
View notes