#AI-driven data analysis
Explore tagged Tumblr posts
Text
Scientists from India, UK join hands to research healthy brain aging
The Centre for Brain Research (CBR) at the Indian Institute of Science (IISc) and the UK Dementia Research Institute (UK DRI) on Friday launched an international partnership that will create an interconnected research ecosystem to accelerate scientific understanding and innovation in brain health. According to CBR, by integrating expertise and cutting-edge technologies including blood-based…
#AI-driven data analysis#and digital cognitive monitoring tools#blood-based biomarkers#Centre for Brain Research (CBR) at the Indian Institute of Science (IISc) and the UK Dementia Research Institute (UK DRI)#interconnected research ecosystem to accelerate scientific understanding and innovation in brain health#Scientists from India#UK join hands to research healthy brain aging
0 notes
Text
What Makes a Great Agronomist? Unpacking the Traits of Agricultural Excellence
Agronomists are the unsung heroes shaping the future of farming. They’re the bridge between science and soil, the architects of abundance in a world hungry for both food and sustainability. Over the years, after sifting through hundreds of agronomist resumes and meeting countless professionals in this field, I’ve come to realize that greatness in agronomy isn’t just about a degree or a title.…
#agricultural excellence#Agricultural Research#agriculture#agronomist#agronomist traits#agronomy skills#AI soil analysis#collaboration#community impact#crop yield#data-driven farming#drones in farming#eco-friendly farming#Farmer Support#farming innovation#kenya#kenyan farmers#lifelong learning#precision agriculture#regenerative agriculture#soil health#Sustainability#sustainable productivity#tech pioneers#technology in agriculture
7 notes
·
View notes
Text
🚀 Explore how AI can transform your B2B marketing strategy! Discover actionable tactics to enhance buyer engagement and create personalized experiences. Dive into AI-driven buyer-centric strategies today! #B2BMarketing #AI #BuyerEngagement #DigitalMarketing
#account-based marketing#AI#AI-driven marketing#automated nurturing#B2B marketing#brand awareness#buyer enablement#buyer experiences#buyer journeys#buyer-centric strategies#buying groups#campaign effectiveness#content distribution#conversion rate optimization#customer engagement#data analysis#demand intelligence#digital marketing#engagement#lead generation#marketing automation#marketing insights#multi-touch attribution#omnichannel experience#performance insights#personalization#resource optimization
4 notes
·
View notes
Text
How DeepSeek AI Revolutionizes Data Analysis
1. Introduction: The Data Analysis Crisis and AI’s Role2. What Is DeepSeek AI?3. Key Features of DeepSeek AI for Data Analysis4. How DeepSeek AI Outperforms Traditional Tools5. Real-World Applications Across Industries6. Step-by-Step: Implementing DeepSeek AI in Your Workflow7. FAQs About DeepSeek AI8. Conclusion 1. Introduction: The Data Analysis Crisis and AI’s Role Businesses today generate…
#AI automation trends#AI data analysis#AI for finance#AI in healthcare#AI-driven business intelligence#big data solutions#business intelligence trends#data-driven decisions#DeepSeek AI#ethical AI#ethical AI compliance#Future of AI#generative AI tools#machine learning applications#predictive modeling 2024#real-time analytics#retail AI optimization
3 notes
·
View notes
Text
Elon Musk’s Ally Pushes for ‘AI-First’ Strategy in Government Agency
Elon Musk’s Ally Pushes for ‘AI-First’ Strategy in Government Agency In a groundbreaking statement, a close ally of Elon Musk has revealed that embracing an “AI-first” approach is the future for a key government agency. This bold vision outlines how artificial intelligence (AI) will shape the operations and policies of government entities, especially those tied to technology and national…
#AI adoption#AI collaboration#AI in defense#AI in government#AI strategy#AI technology#AI-driven government#AI-first#artificial intelligence#cybersecurity#data analysis#Elon Musk#future of AI#government agency#government reform#Musk&039;s vision#national security#public sector#public services#tech industry#technology innovation
2 notes
·
View notes
Text
AI in Lead Generation: Who Does It Better, B2B or B2C?
As AI continues to shape the digital landscape, businesses are turning to intelligent systems to enhance their lead generation efforts. But which model—B2B or B2C—leverages AI best?
1. B2B Lead Generation: Precision is Key
In B2B, AI helps generate highly targeted leads. Key benefits include:
Personalized Outreach: AI automates tailored emails, boosting conversion rates.
Predicting Intent: AI analyzes data to identify when a client is ready for engagement.
Lead Scoring: Machine learning ranks leads, saving time and focusing efforts on high-potential prospects.
B2B lead gen is about quality, and AI helps businesses zero in on the best opportunities.
2. B2C Lead Generation: Speed and Scale
B2C businesses benefit from AI's ability to scale quickly. AI enables:
Customer Insights: Real-time data analysis helps understand consumer behavior.
Personalization at Scale: AI powers dynamic ads and product recommendations.
Automated Nurturing: AI-driven content and email campaigns keep prospects engaged.
For B2C, AI is a game-changer in reaching large audiences efficiently.
Who Wins?
B2B and B2C each win in different ways. B2B thrives on AI's precision, while B2C excels with AI's scalability. The ultimate winner is the business that adapts AI to its specific needs.
About US: AI Technology Insights (AITin) is the fastest-growing global community of thought leaders, influencers, and researchers specializing in AI, Big Data, Analytics, Robotics, Cloud Computing, and related technologies. Through its platform, AITin offers valuable insights from industry executives and pioneers who share their journeys, expertise, success stories, and strategies for building profitable, forward-thinking businesses.
Contact Us :
Call Us
+1 (520) 350-7212
Email Address
Local Address
1846 E Innovation Park DR Site 100 ORO Valley AZ 85755
0 notes
Text
How are Investors using AI in Stock Market Trading to Drive Powerful Results?

AI in Stock Trading has quietly become Wall Street’s most trusted partner, a digital oracle guiding decisions with data, not emotion.
From detecting trends before they go viral to executing trades in the blink of an eye, it’s transforming how investors and CEOs conquer the markets.
This isn’t just about automation. It’s a revolution in intelligence, strategy, and results.
Why is AI becoming the secret weapon of modern-day traders and investors?
Let’s peel back the curtain and explore why AI in Stock Trading is quietly reshaping the way investors, analysts, and decision-makers approach the market with more precision and power than ever before.
Because it’s no longer just a buzzword, it’s Wall Street’s new brain
Once seen as a futuristic concept reserved for tech geeks and hedge funds, AI in Stock Trading has now entered the mainstream. It’s quietly disrupting age-old trading strategies and replacing gut-feel decisions with precision-based automation.
And it’s doing so with alarming efficiency.
AI is doing to traditional stock trading what GPS did to printed maps which is rendering them obsolete, one algorithm at a time.
From real-time sentiment analysis to predictive forecasting, AI is taking over not just how trades are executed, but why they’re made.
If you're a CEO, CTO, investor, or portfolio manager, the message is clear: Get ahead of the AI curve or get left behind.
The evolution from human intuition to machine intelligence
Not long ago, a good trader needed a sixth sense; a mix of experience, instinct, and maybe a little caffeine-induced luck. But now, success hinges on data accuracy, speed, and pattern recognition, which AI does exponentially better.
AI doesn't sleep
AI doesn’t panic in volatile markets
AI sees patterns humans simply can’t
It digests billions of data points in real-time, identifies anomalies, and executes trades at the speed of thought or faster.
So, what does this mean for modern-day investors?
It means the edge is no longer emotional intelligence, it’s algorithmic intelligence. It’s about integrating a system that can think, learn, and act all while sipping your morning coffee.
Let’s break down how to harness this edge, what tools you’ll need, and what pitfalls to avoid in your AI in Stock Trading journey.
How does AI actually work in stock trading behind the scenes?
To understand the true power of AI in Stock Trading, we need to look beneath the surface and follow the data trail that fuels every intelligent decision.
It all starts with data. And lots of it.
At the heart of every AI-powered trading strategy is data. Tons of it. We’re talking about:
Market price history
Trading volumes
Social media sentiment
News headlines
Financial reports
Macroeconomic indicators
AI uses this to train models, spot patterns, and make informed predictions.
Think of AI like a trader with 100,000 eyes, scanning markets, news, and trends simultaneously.
Key AI techniques used in trading today:
These aren’t just buzzwords from a tech conference. They’re the engines driving today’s most powerful AI trading systems, each with their own roles in turning raw data into real-time decisions.
1: Machine Learning (ML):
Uses historical data to forecast future prices and trends
Learns from past trades and adapts without manual input
2: Natural Language Processing (NLP):
Analyzes news articles, tweets, and even Reddit threads to measure market sentiment
Detects shifts in investor mood before markets react
3: Deep Learning (Neural Networks):
Mimics human brain functions to find hidden patterns
Effective in predicting price volatility and automating high-frequency trading
4: Reinforcement Learning:
A trial-and-error approach where the algorithm learns strategies over time, improving with every trade
"Machine learning is the only way to discover exploitable inefficiencies in modern markets." - Dr. Marcos López de Prado (AI expert, author of Advances in Financial Machine Learning)
Real-world application of AI in trading:
While theory shows us the potential, these real-world applications prove just how deeply AI in Stock Trading is already woven into the strategies of global financial powerhouses.
JP Morgan’s LOXM: Executes trades with minimal market impact
BlackRock’s Aladdin: Manages over $21 trillion in assets using AI risk analysis
JP Morgan’s LOXM
JP Morgan developed an AI-powered trading engine called LOXM, designed to execute large trades with minimal market disruption. Instead of pushing large orders into the market all at once (which can move prices), LOXM smartly breaks them down and times each part to get better pricing. It’s like having a trader who never gets tired, never second-guesses, and always aims for the most efficient result.
BlackRock’s Aladdin
BlackRock, the world’s largest asset manager, runs its operations using an AI-driven platform called Aladdin. This system helps manage risk, analyze portfolios, and make data-backed investment decisions across more than $21 trillion in assets. From scanning market changes to stress-testing portfolios, Aladdin acts like a digital brain behind BlackRock’s global investment machine.
The takeaway? This isn't theory, this is practice.
How to use AI in stock market trading the smart way?
Understanding the strategy is only half the battle. To truly unlock the potential of AI in Stock Trading, you need a clear roadmap that turns ideas into intelligent action.
Step-by-step: From concept to execution
There’s a misconception that AI in Stock Trading is only for billion-dollar hedge funds. Not true. Whether you're an individual trader, financial startup, or mid-size enterprise, implementing AI is possible and profitable if you follow the right framework.
Let’s break it down in simple, actionable steps.
A Step-by-Step Guide to Implementing AI in Stock Trading Operations:
Building an AI-powered trading system involves defining clear objectives, collecting and preparing quality data, choosing the right tech stack, training and validating models, running thorough backtests, and gradually deploying into live markets with continuous monitoring and refinement.
Define Your Objective:
Are you building a predictive model? Risk management tool? A sentiment analyzer?
Clear goals help narrow your AI approach.
Gather High-Quality Data:
This includes structured data (prices, indicators) and unstructured data (news, social posts).
Garbage in = garbage out.
Choose the Right Tech Stack:
Python, TensorFlow, PyTorch, Scikit-learn
Consider cloud platforms like AWS or Azure for scalability
Build & Train Your Model:
Supervised or unsupervised? Regression or classification? Choose based on your trading logic.
Validate the model against historical data.
Backtest Like Crazy:
Test your AI model using past data to simulate real-world scenarios.
Refine based on success metrics like Sharpe Ratio and ROI.
Deploy in a Sandbox Environment:
Monitor your AI’s performance before going live.
Protect your capital while the model learns in real-time.
Go Live & Scale:
Start with small volumes.
Monitor trades and make iterative updates.
The smarter the model, the longer it takes to train, but the more powerful the payoff.
What’s the real ROI of AI in stock trading?
To truly evaluate the value of AI in Stock Trading, you need to move beyond the hype and look at the measurable impact it delivers in real-world operations.
Spoiler alert: It can be massive if done right
When implemented strategically, AI can unlock impressive returns and drastically reduce trading risks.
Higher accuracy in forecasting
Faster trade execution
Lower transaction costs
24/7 market monitoring
Firms using AI have reported:
AI in stock trading is already delivering real results, with firms reporting major gains in performance and efficiency.
Up to 30% improvement in portfolio performance
40% reduction in operational costs
Real-time fraud detection and prevention
In the race of trading efficiency, AI doesn’t just run faster, it predicts the finish line.
Want to dive deeper into AI tools, implementation models, and real-world examples?
Don’t miss our in-depth post: AI in Stock Trading: The Complete Guide
It’s a must-read if you’re serious about understanding how to use AI in stock market trading effectively, securely, and profitably.
What the future holds for AI in stock trading
The future of AI in stock trading isn’t just promising. It’s already unfolding. As the technology evolves, it’s unlocking smarter, faster, and more personalized ways to invest and it’s only going to get better.
1. AI and Blockchain Will Bring New Levels of Trust
The next generation of trading will combine AI with blockchain, creating systems that are not only powerful but also fully transparent. Every trade can be tracked, verified, and trusted, making automated strategies even more secure and reliable.
2. Quantum Computing Will Supercharge Performance
With quantum computing on the horizon, AI models will be able to process and learn from data at speeds we’ve never seen before. That means better forecasts, quicker decisions, and stronger results for both individual investors and large institutions.
3. Hyper-Personalized Trading Experiences
AI will no longer just track market trends. It will learn how you invest, what risks you’re comfortable with, and how to tailor strategies to match your goals. Imagine having a smart advisor that adjusts your strategy in real time based on your unique profile.
4. More Accessible AI for Everyone
AI in stock trading is becoming more user-friendly and accessible. Thanks to open platforms and low-code tools, more startups, independent investors, and financial advisors can now tap into the same powerful tools once reserved for major firms.
5. Built-In Intelligence for Compliance and Stability
AI will help keep trading environments safer and more compliant. Future systems will include real-time monitoring and automatic checks, making sure trades follow regulations while reducing risk, all without slowing you down.
The takeaway: AI in stock trading is not just the future. It’s a smarter, more reliable, and more inclusive way forward. Whether you’re managing billions or just getting started, AI is creating opportunities for everyone to trade with more confidence, clarity, and control.
"AI is the defining technology of our time. It will augment human capability and help us do more." - Satya Nadella (CEO, Microsoft)
Conclusion: The future of trading is already here, and it’s powered by AI
The message is loud and clear: AI in Stock Trading is no longer the future, it’s the present.
From hedge funds to home offices, algorithms are analyzing markets, identifying patterns, and executing trades with precision that human brains simply can't replicate. But the real power lies not just in adopting AI but in implementing it strategically, ethically, and intelligently.
Whether you're a CEO exploring digital transformation, a fintech founder building a next-gen platform, or an investor looking to scale smarter, AI isn’t just an option.
It’s your competitive advantage.
Ready to leverage AI for strategic market dominance?
Let’s make the market work for you, not against you.
#AI in Stock Trading#AI Market Analysis#Stock Trading Tools#AI Implementation#Fintech Innovation#Data Driven Trading#Machine Learning Finance#Investment Strategies#Trading Technology#AI For Investors
0 notes
Text
AI-Powered Business Analytics: Make Smarter Decisions, Faster
AI-Powered Business Analytics Make Smarter Decisions, Faster 💡 AI-powered analytics give you instant insights into what’s working and what’s not. Learn how to use AI to optimize business decisions. The Problem: Are You Guessing or Growing? Let’s be real—making business decisions based on gut feelings is like throwing darts blindfolded. Sure, you might hit the target occasionally, but most of…
#AI automation for business#AI business analytics#AI business optimization tools#AI customer insights#AI data analysis#AI data visualization#AI for advertising performance#AI for audience segmentation#AI for business intelligence#AI for business scalability#AI for customer retention#AI for customer segmentation#AI for demand prediction#AI for eCommerce analytics#AI for financial forecasting#AI for operational decision-making#AI for process optimization#AI for revenue optimization#AI for ROI maximization#AI for sales forecasting#AI for sales optimization#AI for small business growth#AI in corporate decision-making#AI in digital marketing#AI in marketing analytics#AI operational efficiency#AI performance tracking#AI predictive analytics#AI-driven business strategy#AI-driven competitive analysis
0 notes
Text
Automate, Optimize, and Succeed AI in Call Centers

Introduction
The call center industry has undergone a significant transformation with the integration of artificial intelligence (AI). Businesses worldwide are adopting AI-powered call center solutions to enhance customer service, improve efficiency, and reduce operational costs. AI-driven automation helps optimize workflows and ensures superior customer experiences. This article explores how AI is revolutionizing call centers, focusing on automation, optimization, and overall business success.
The Rise of AI in Call Centers
AI technology is reshaping the traditional call center model by enabling automated customer interactions, predictive analytics, and enhanced customer service strategies. Key advancements such as Natural Language Processing (NLP), machine learning, and sentiment analysis have led to the creation of intelligent virtual assistants and chatbots that streamline communication between businesses and customers.
Key Benefits of AI in Call Centers
Automation of Repetitive Tasks
AI-driven chatbots and virtual assistants handle routine customer inquiries, freeing up human agents to focus on more complex issues.
AI automates call routing, ensuring customers reach the right agent or department quickly.
Improved Customer Experience
AI-powered systems provide personalized responses based on customer history and preferences.
AI reduces wait times and improves first-call resolution rates, leading to higher customer satisfaction.
Optimized Workforce Management
AI-based analytics predict call volumes and optimize staffing schedules to prevent overstaffing or understaffing.
AI assists in real-time monitoring and coaching of agents, improving overall productivity.
Enhanced Data Analysis and Insights
AI tools analyze customer interactions to identify trends, allowing businesses to improve services and make data-driven decisions.
Sentiment analysis helps understand customer emotions and tailor responses accordingly.
Cost Efficiency and Scalability
AI reduces the need for large call center teams, cutting operational costs.
Businesses can scale AI-powered solutions effortlessly without hiring additional staff.
AI-Powered Call Center Technologies
Chatbots and Virtual Assistants
These AI-driven tools handle basic inquiries, appointment scheduling, FAQs, and troubleshooting.
They operate 24/7, ensuring customers receive support even outside business hours.
Speech Recognition and NLP
NLP enables AI to understand and respond to human language naturally.
Speech recognition tools convert spoken words into text, enhancing agent productivity and improving accessibility.
Sentiment Analysis
AI detects customer emotions in real time, helping agents adjust their approach accordingly.
Businesses can use sentiment analysis to track customer satisfaction and identify areas for improvement.
Predictive Analytics and Call Routing
AI anticipates customer needs based on past interactions, directing them to the most suitable agent.
Predictive analytics help businesses forecast trends and plan proactive customer engagement strategies.
AI-Powered Quality Monitoring
AI analyzes call recordings and agent interactions to assess performance and compliance.
Businesses can provide data-driven training to improve agent efficiency and customer service.
Challenges and Considerations in AI Implementation
While AI offers numerous benefits, businesses must address several challenges to ensure successful implementation:
Data Privacy and Security
AI systems process vast amounts of customer data, making security a top priority.
Businesses must comply with regulations such as GDPR and CCPA to protect customer information.
Human Touch vs. Automation
Over-reliance on AI can make interactions feel impersonal.
A hybrid approach, where AI supports human agents rather than replacing them, ensures a balance between efficiency and empathy.
Implementation Costs
AI integration requires an initial investment in technology and training.
However, long-term benefits such as cost savings and increased productivity outweigh the initial expenses.
Continuous Learning and Improvement
AI models require regular updates and training to adapt to changing customer needs and market trends.
Businesses must monitor AI performance and refine algorithms to maintain efficiency.
Future of AI in Call Centers
The future of AI in call centers is promising, with continued advancements in automation and machine learning. Here are some trends to watch for:
AI-Driven Omnichannel Support
AI will integrate seamlessly across multiple communication channels, including voice, chat, email, and social media.
Hyper-Personalization
AI will use real-time data to deliver highly personalized customer interactions, improving engagement and satisfaction.
Autonomous Call Centers
AI-powered solutions may lead to fully automated call centers with minimal human intervention.
Enhanced AI and Human Collaboration
AI will complement human agents by providing real-time insights and recommendations, improving decision-making and service quality.
Conclusion
AI is transforming call centers by automating processes, optimizing operations, and driving business success. Companies that embrace AI-powered solutions can enhance customer service, increase efficiency, and gain a competitive edge in the market. However, successful implementation requires balancing automation with the human touch to maintain meaningful customer relationships. By continuously refining AI strategies, businesses can unlock new opportunities for growth and innovation in the call center industry.
#AI in call centers#Call center automation#AI-powered customer service#Virtual assistants in call centers#Chatbots for customer support#Natural Language Processing (NLP)#Sentiment analysis in call centers#Predictive analytics in customer service#AI-driven workforce optimization#Speech recognition in call centers#AI-powered quality monitoring#Customer experience optimization#Data analysis in call centers#Call center efficiency#AI and human collaboration#Future of AI in call centers#AI-driven omnichannel support#Hyper-personalization in customer service#Autonomous call centers#AI security and compliance
0 notes
Text
Discover how AI-powered analytics, real-time competitor monitoring, and predictive insights are revolutionizing marketplace intelligence. Learn how businesses can leverage dynamic pricing, demand forecasting, and external data integration to stay competitive and maximize revenue. Stay ahead in the fast-evolving eCommerce landscape with cutting-edge intelligence strategies!
#competitor price tracking#product matching#price monitoring#price intelligence#price matching#Marketplace Intelligence#competitor pricing#competitor tracking#AI-driven intelligence tools#Monitoring competitor prices#Data Analysis#Dynamic Pricing#AI-Driven Competitive Analysis#Business intelligence#Pricing Intelligence#smart pricing#pricing strategies
0 notes
Text
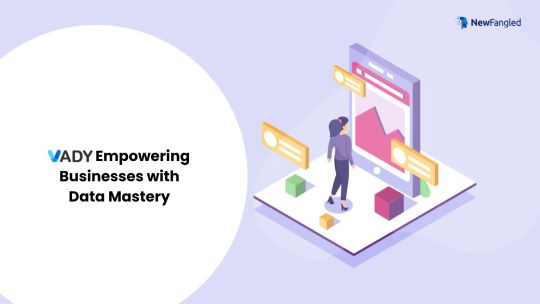
VADY – Empowering Businesses with Data Mastery
In today’s fast-paced world, mastering data is essential for business success. VADY empowers organizations to harness the true potential of their data, turning it into actionable intelligence that drives performance and growth. With our AI-powered tools and advanced analytics, we enable businesses to make informed decisions, improve operational efficiencies, and discover new market opportunities. From cleaning and structuring data to deriving meaningful insights, VADY ensures that businesses gain a mastery over their data. Our solutions simplify complex data, enabling businesses to stay ahead of trends, optimize strategies, and achieve sustainable growth, regardless of industry or size.
#vady#newfangled#data democratization#big data#data analytics#machine learning#data at fingertip#etl#nlp#ai to generate dashboard#ai enabled dashboard#generativebi#generativeai#artificialintelligence#data visualization#data mining#data analysis#data privacy#data driven decisions
0 notes
Text
Jade Ann Byrne 🩸 Blood Pressure Chronicles: What 92/45 and 96/57 Say About My Post Home Alone Midnight Journey
Jade Ann Byrne 🩸 Blood Pressure Chronicles: What 92/45 and 96/57 Say About My Post Home Alone Midnight Journey Life is measured in moments, and health is written in numbers. Today’s check-in? A rollercoaster of 92/45 mmHg followed by a slight rebound to 96/57 mmHg, with a steady pulse of 85 bpm. Let’s break it down and see what the body is whispering. 📊 Breaking It Down: 🔹 Systolic (92 → 96…
#adrenaline and BP correlation#AI-driven BP tracking#ambulatory blood pressure#arm circumference BP adjustments#arterial stiffness index#automated BP cuff#autonomic dysfunction#baroreflex#blood circulation#blood pressure#blood pressure monitor#blood pressure variability#blood volume pulse#BP accuracy factors#BP artifacts#BP cuff calibration#BP logging#BP rebound effect#BP telemetry#BP trends analysis#caffeine effects on BP#capillary refill time#cardiovascular health#chronic illness#circulatory efficiency#cuff inflation technology#cuff positioning#daily vitals#data tracking#diastolic pressure
0 notes
Text
3 Ways to Boost Sales with Artificial Intelligence
Delivering Hyper-Targeted Ecommerce Experiences The era of generic, one-size-fits-all ecommerce experiences is fading fast. In its place, we’re witnessing the rise of hyper-personalization, driven by the power of Artificial Intelligence (AI). Gone are the days of basic product recommendations. Today, AI algorithms can analyze vast amounts of customer data – browsing history, purchase history,…
#AI#AI-driven content personalization#Artificial Intelligence#Customer data analysis#Customer needs anticipation#Dynamic content adjustments#Ecommerce experiences#Inventory optimization#Personalized marketing messages#Predictive Analytics#Product recommendations
0 notes
Text
Transforming real-time monitoring with AI-enhanced digital twins
New Post has been published on https://thedigitalinsider.com/transforming-real-time-monitoring-with-ai-enhanced-digital-twins/
Transforming real-time monitoring with AI-enhanced digital twins


A recent McKinsey report found that 75% of large enterprises are investing in digital twins to scale their AI solutions. Combining digital twins with AI has the potential to enhance the effectiveness of large language models and enable new applications for AI in real-time monitoring, offering significant business and operational benefits.
What are digital twins?
Digital twins, originally developed to aid in the design of complex machinery have evolved significantly over the last two decades. They track and analyse live systems in real-time by processing device telemetry, detecting shifting conditions, and enhancing situational awareness for operational managers. Powered by in-memory computing, they enable fast, actionable alerts. Beyond real-time monitoring, digital twins also can simulate intricate systems like those for use in airlines and logistics, supporting strategic planning and operational decisions through predictive analytics.
Integrating digital twins with generative AI creates new opportunities for both technologies: The synergy can boost the prediction accuracy of generative AI, and can enhance the value of digital twins for system monitoring and development.
Proactively identifying anomalies with AI-powered digital twins
Continuous, real-time monitoring is a strategic necessity for organisations that manage complex live systems, like transportation networks, cybersecurity systems, and smart cities. Emerging problems must never be overlooked because delayed responses can cause small problems to become large ones.
Enhancing digital twins with generative AI reshapes how real-time monitoring interprets massive volumes of live data, enabling the reliable and immediate detection of anomalies that impact operations. Generative AI can continuously examine analytics results produced by digital twins to uncover emerging trends and mitigate disruptions before they escalate. While AI enhances situational awareness for managers, it can also pinpoint new opportunities for optimising operations and boosting efficiency.
At the same time, real-time data supplied by digital twins constrains the output of generative AI to avoid erratic results, like hallucinations. In a process called retrieval augmented generation, AI always uses the most up-to-date information about a live system to analyse behaviour and create recommendations.
Transforming data interaction with AI-driven visualisations
Unlocking insights from digital twin analytics should be intuitive, not technical. Generative AI is redefining how teams interact with massive datasets by enabling natural language-driven queries and visualisations. Instead of manually constructing intricate queries, users can simply describe their needs, and generative AI immediately visualises relevant charts and query results that provide new insights. This capability simplifies interactions and gives decision-makers the data they need. As organisations handle increasingly complex live systems, AI-powered intelligence allows them to efficiently sift through vast data pools, extract meaningful trends, and optimise operations with greater precision. It eliminates technical barriers, enabling faster, data-driven decisions that have a strategic impact.
Incorporating machine learning with automatic retraining
Digital twins can track numerous individual data streams and look for issues with the corresponding physical data sources. Working together, thousands or even millions of digital twins can monitor very large, complex systems. As messages flow in, each digital twin combines them with known information about a particular data source and analyses the data in a few milliseconds. It can incorporate a machine learning algorithm to assist in the analysis and find subtle issues that would be difficult to describe in hand-coded algorithms. After training with data from live operations, ML algorithms can identify anomalies and generate alerts for operational managers immediately.
Once deployed to analyse live telemetry, an ML algorithm will likely encounter new situations not covered by its initial training set. It may either fail to detect anomalies or generate false positives. Automatic retraining lets the algorithm learn as it gains experience so it can improve its performance and adapt to changing conditions. Digital twins can work together to detect invalid ML responses and build new training sets that feed automatic retraining. By incorporating automatic retraining, businesses gain a competitive edge with real-time monitoring that reliably delivers actionable insights as it learns over time.
Looking forward
Integrating digital twin technology with generative AI and ML can transform how industries monitor complex, live systems by empowering better real-time insights and enabling managers to make faster, more informed decisions. ScaleOut Software’s newly-released Digital Twins™ Version 4 adds generative AI using OpenAI’s large language model and automatic ML retraining to move real-time monitoring towards the goal of fully-autonomous operations.
(Image source: Unsplash)
#ai#AI-powered#alerts#algorithm#Algorithms#analyses#Analysis#Analytics#anomalies#applications#Artificial Intelligence#autonomous#awareness#Business#charts#cities#computing#continuous#cybersecurity#data#data sources#Data Streams#data-driven#data-driven decisions#datasets#decision-makers#Design#detection#development#digital twins
0 notes
Text
Wawasan Pelanggan Berbasis AI untuk Pemasaran yang Tepat Sasaran
Di era digital yang semakin kompleks, memahami pelanggan menjadi kunci keberhasilan pemasaran. Perusahaan yang dapat mengidentifikasi kebutuhan, preferensi, dan perilaku pelanggan memiliki keunggulan kompetitif yang signifikan. Namun, dengan jumlah data yang terus bertambah, menganalisis informasi secara manual menjadi tantangan besar. Di sinilah kecerdasan buatan (AI) memainkan peran penting. AI…
#AI in small businesses#AI marketing#AI-driven campaigns#customer insights#customer loyalty#data ethics#future of AI marketing#hyper-personalization#machine learning tools#marketing automation#omnichannel marketing#personalized marketing#predictive analytics#ROI improvement#sentiment analysis
0 notes
Text
Why AI is SEO Friendly for Writing?
Today, where content reigns supreme, mastering Search Engine Optimization (SEO) is essential for anyone looking to increase their online visibility. With the advent of Artificial Intelligence (AI), the writing process has undergone a significant transformation, making it easier to produce SEO-friendly content. This article delves into how AI enhances SEO writing, supported by examples and…
View On WordPress
#AI#AI content generation#AI for content marketing#AI-driven SEO strategies#AI-powered keyword analysis#automated content creation#competitive analysis with AI#content personalization AI#data-driven SEO techniques#keyword research AI#machine learning for SEO#natural language processing SEO#predictive analytics in SEO#semantic search optimization#SEO#SEO optimization tools#voice search optimization
0 notes